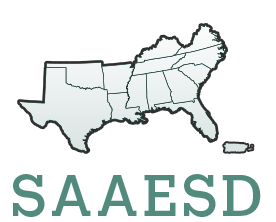
S1090: AI in Agroecosystems: Big Data and Smart Technology-Driven Sustainable Production
(Multistate Research Project)
Status: Active
SAES-422 Reports
08/31/2022
Alabama: Aubrun University
Citation of the conference proceedings paper: Oliveira, M.F., F.M. Carneiro, M. Thurmond, M.D. Del val, L.P. Oliveira, B. Ortiz, A. Sanz-saez, D. Tedesco. 2022. Predicting Below and Above Ground Peanut Biomass and Maturity Using Multi-target Regression. In Proceedings of the 2022 International Conference of Precision Agriculture. June 26-29, 2022 Minneapolis.
Florida: University of Florida
Yuan, W., Choi, D., Bolkas, D., Heinemann, P.H. and He, L., 2022. Sensitivity examination of YOLOv4 regarding test image distortion and training dataset attribute for apple flower bud classification. International Journal of Remote Sensing, 43(8), pp.3106-3130.
Yuan, W., Choi, D., & Bolkas, D. (2022). GNSS-IMU-assisted colored ICP for UAV-LiDAR point cloud registration of peach trees. Computers and Electronics in Agriculture, 197, 106966.
Zahid, A., Mahmud, M.S., He, L., Schupp, J., Choi, D. and Heinemann, P., 2022. An Apple Tree Branch Pruning Analysis. HortTechnology, 32(2), pp.90-98.
Zhang, H., He, L., Di Gioia, F., Choi, D., Elia, A. and Heinemann, P., 2022. LoRaWAN based internet of things (IoT) system for precision irrigation in plasticulture fresh-market tomato. Smart Agricultural Technology, 2, p.100053.
Mahmud, M.S., Zahid, A., He, L., Choi, D., Krawczyk, G. and Zhu, H., 2021. LiDAR-sensed tree canopy correction in uneven terrain conditions using a sensor fusion approach for precision sprayers. Computers and Electronics in Agriculture, 191, p.106565.
Patel, A., W. S. Lee, N. A. Peres, and C. W. Fraisse. 2021. Strawberry plant wetness detection using computer vision and deep learning. Smart Agricultural Technology 1, 2021, 100013, ISSN 2772-3755, https://doi.org/10.1016/j.atech.2021.100013
Yun, C., H.-J. Kim, C.-W. Jeon, M. Gang, W. S. Lee, and J. G. Han. 2021. Stereovision-based ridge-furrow detection and tracking for auto-guided cultivator. Computers and Electronics in Agriculture 191, 2021, 106490, ISSN 0168-1699, https://doi.org/10.1016/j.compag.2021.106490.
Puranik, P., W. S. Lee, N. Peres, F. Wu, A. Abd-Elrahman, and S. Agehara. 2021. Strawberry flower and fruit detection using deep learning for developing yield prediction models. In the Proceedings of the 13th European Conference on Precision Agriculture (ECPA), July 19-22, 2021, Budapest, Hungary.
Zhou, X., W. S. Lee, Y. Ampatzidis, Y. Chen, N. Peres, and C. Fraisse. 2021. Strawberry maturity classification from UAV and near-ground imaging using deep learning. Smart Agricultural Technology 1, 2021, 100001, ISSN 2772-3755, https://doi.org/10.1016/j.atech.2021.10000
Costa L., McBreen J., Ampatzidis Y., Guo J., Reisi Gahrooei M., Babar A., 2021. Using UAV-based hyperspectral imaging and functional regression to assist in predicting grain yield and related traits in wheat under heat-related stress environments for the purpose of stable yielding genotypes. Precision Agriculture, 23 (2), 622-642.
Costa L., Ampatzidis Y., Rohla C., Maness N., Cheary B., Zhang L., 2021. Measuring pecan nut growth utilizing machine vision and deep learning for the better understanding of the fruit growth curve. Computers and Electronics in Agriculture, 181, 105964, doi.org/10.1016/j.compag.2020.105964.
Costa L., Archer L., Ampatzidis Y., Casteluci L., Caurin G.A.P., Albrecht U., 2021. Determining leaf stomatal properties in citrus trees utilizing machine vision and artificial intelligence. Precision Agriculture 22, 1107-1119, https://doi.org/10.1007/s11119-020-09771-x.
Nunes L., Ampatzidis Y., Costa L., Wallau M., 2021. Horse foraging behavior detection using sound recognition techniques and artificial intelligence. Computers and Electronics in Agriculture, 183, 106080, doi.org/10.1016/j.compag.2021.106080.
Vijayakumar V., Costa L., Ampatzidis Y., 2021. Prediction of citrus yield with AI using ground-based fruit detection and UAV imagery. 2021 Virtual ASABE Annual International Meeting, July 11-14, 2021, 2100493, doi:10.13031/aim.202100493.
Zhou, C., W. S. Lee, O. E. Liburd, I. Aygun, J. K. Schueller, and I. Ampatzidis. 2021. Smartphone-based tool for two-spotted spider mite detection in strawberry. ASABE Paper No. 2100558. St. Joseph, MI.: ASABE.
Zhou, X., Y. Ampatzidis, W. S. Lee, and S. Agehara. 2021. Postharvest strawberry bruise detection using deep learning. ASABE Paper No. 2100458. St. Joseph, MI.: ASABE.
Influence of Planting Date, Maturity Group, and Harvest Timing on Soybean (Glycine max (L.) Yield and Seed Quality, PRISCILA CAMPOS, DONNIE MILLER, JOSH COPES, MELANIE NETTERVILLE, SEBE BROWN, TREY PRICE, DAVID MOSELEY, THANOS GENTIMIS, PETERS EGBEDI, RASEL PARVEJ3 (Accepted by Crop, Forage, & Turfgrass Management, Summer 2022). In this paper, modern methodologies were implemented in the analysis of the results, as well as more traditional statistical techniques.
The Time of Day Is Key to Discriminate Cultivars of Sugarcane upon Imagery Data from Unmanned Aerial Vehicle, BARBOSA JÚNIOR, M.R.; TEDESCO, D.; CARREIRA, V.S.; PINTO, A.A.; MOREIRA, B.R.A.; SHIRATSUCHI, L.S.; ZERBATO, C.; SILVA, R.P., Drones 2022, 6, 112. https://doi.org/10.3390/drones6050112
UAVs to Monitor and Manage Sugarcane: Integrative Review, BARBOSA JÚNIOR, M.R.; MOREIRA, B.R.A.; BRITO FILHO, A.L.; TEDESCO, D.; SHIRATSUCHI, L.S.; SILVA, R.P., Agronomy 2022, 12, 661. https://doi.org/10.3390/agronomy12030661
Predicting Days to Maturity, Plant Height, and Grain Yield in Soybean: A Machine and Deep Learning Approach Using Multispectral Data, TEODORO, P. E.; TEODORO, L. P. R.; BAIO, F. H. R.; SILVA JUNIOR, C. A.; SANTOS, R. G.; RAMOS, A. P. M.; PINHEIRO, M. M. F.; OSCO, L. P.; GONCALVES, W. N.; CARNEIRO, A. M.; MARCATO JUNIOR, J.; PISTORI, H.; SHIRATSUCHI, L. S., Remote Sensing, v. 13, p. 4632, 2021
Comparison of Machine Learning Techniques in Cotton Yield Prediction Using Satellite Remote Sensing, MORELLI-FERREIRA, F.; MAIA, N.J.C.; TEDESCO, D.; KAZAMA, E.H.; MORLIN CARNEIRO, F.; SANTOS, L.B.; SEBEN JUNIOR, G.F.; ROLIM, G.S.; SHIRATSUCHI, L.S.; SILVA, R.P. Preprints 2021, 2021120138 (doi: 10.20944/preprints202112.0138.v2). Published and in preparation for Remote Sensing.
Kentucky: University of Kentucky
Ekramirad, N., Khaled, Y.A., Doyle, L., Loeb, J., Donohue, K.D., Villanueva, R., and Adedeji, A.A. (2022). Nondestructive detection of codling moth infestation in apples using pixel-based NIR hyperspectral imaging with machine learning and feature selection. Foods 11(8), 1 - 16. (Citation: 2)
Rady, A., Watson, N., and Adedeji, A.A. (2021). Color imaging and machine learning for adulteration detection in minced meat. Journal of Agriculture and Food Research 6(100251), 1-11. (Citation: 1)
Watson, N.J., Bowler, A.L., Rady, A., Fisher, O.J., Simeone, A., Escrig, J., Woolley, E., and Adedeji, A.A. (2021). Intelligent sensors for sustainable food and drink manufacturing. Frontiers in Sustainable Food Systems. 5, 642786. (Citation: 5)
Ekramirad, N., Al Khaled, Y.A., Donohue, K., Villanueva, R., Parrish, C.A., and Adedeji, A. (2021). Development of pattern recognition and classification models for the detection of vibro-acoustic emissions from codling moth infested apples. Postharvest Biology and Technology 181, 111633. (Citation: 1)
Khaled, Y.A., Parrish, C. and *Adedeji, A.A. (2021). Emerging nondestructive approaches for meat quality and safety evaluation. Comprehensive Reviews in Food Science and Food Safety. 20(4): 3438-3463. (Citation: 15)
Mississppi: Mississippi State University
Chen, D., Lu, Y., Li, Z., and Young, S. 2022. Performance evaluation of deep transfer learning on multi-class identification of common weed species in cotton production systems. Computers and Electronics in Agriculture.
Yadav, P.K., Thomasson, J.A., Hardin, R.G., Searcy, S.W., Braga-Neto, U., Popescu, S.C., Martin, D.E., Rodriguez III, R., Meza, K., Enciso, J. and Solorzano, J. 2022. Volunteer cotton plant detection in corn field with deep learning. In Proc. Autonomous Air and Ground Sensing Systems for Agricultural Optimization and Phenotyping VII (Vol. 12114, pp. 15-22). SPIE.
North Carolina: NC State
Edward L. Kick, Laura McKinney, Steve McDonald, Andrew Jorgenson. “A Multiple-Network Analysis of the World System of Nations” is printed in Chinese and is used in what we hope will be a forthcoming publication in Sustainability.” That paper introduces the possibility of using artificial intelligence in farming around the world.
Edward L. Kick, Gregory Fulkerson, and Ahad Pezeshkpoor. “Agriculture Grains, and Beef Production: Remedies for food for Food Insecurity and the Ecological Footprint When the Cataclysm Comes?” Agricultural Research and Technology 23: 53-57.
Edward L. Kick “Cross-National Empirical Studies of Sustainability, Agriculture and the Environment: Cumulating Forward or Erring in an About Face?” Agricultural Research and Technology 25: 601-603.
Edward L. Kick. “Taking a World View”. College of Agriculture and Life Sciences Newsletter.
Edward L. Kick and Ahad Pezeshkpoor. “Biomes, World-System Positions, and National Characteristics as linked Precursors to Global Undernourishment and the Ecological Footprint” Under revision for publication in Sustainability.
South Carolina: Clemson University
Abenina MIA, Maja JM, Cutulle M, Melgar JC, Liu H. Prediction of Potassium in Peach Leaves Using Hyperspectral Imaging and Multivariate Analysis.
AgriEngineering.2022;4(2):400-413. https://doi.org/10.3390/agriengineering4020027
Tennessee; University of Tennessee
Nasiri, A., Yoder, J., Zhao, Y., Hawkins, S., Prado, M., & Gan, H. (2022). Pose estimation-based lameness recognition in broiler using CNN-LSTM network. Computers and Electronics in Agriculture, 197, 106931.
Texas: Texas A&M
Bhandari, M.; Baker, S.; Rudd, J. C.; Ibrahim, A. M. H.; Chang, A.; Xue, Q.; Jung, J.; Landivar, J.; Auvermann, B. Assessing the Effect of Drought on Winter Wheat Growth Using Unmanned Aerial System (Uas)-Based Phenotyping. Remote Sens. 2021, 13 (6). https://doi.org/10.3390/rs13061144.
W. Wu, S. Hague, J. Jung, A. Ashapure, M. Maeda, A. Maeda, A. Chang, D. Jones, J.A. Thomasson, J. Landivar, "Cotton row spacing and Unmanned Aerial Vehicle sensors," Agronomy Journal, https://doi.org/10.1002/agj2.20902, 2021
A. Chang, J. Jung, J. Landivar, J. Landivar, B. Barker, R. Ghosh, "Performance evaluation of parallel structure from motion (SfM) processing with public cloud computing and an on-premise cluster system for UAS images in agriculture," International Journal of Geo-Information, 10, 677, https://doi.org/10.3390/ijgi10100677, 2021
S. Oh, A. Chang, A. Ashapure, J. Jung, N. Dube, M. Maeda, D. Gonzalez, J. Landivar, "Plant Counting of Cotton from UAS Imagery Using Deep Learning-Based Object Detection Framework", Remote Sensing, 12(18):2981, DOI: 10.3390/rs12182981, 2020
M. Bhandari, A. Ibrahim, Q. Xue, J. Jung, A. Chang, J. Rudd, M. Maeda, N. Rajan, H. Neely, J. Landivar, "Assessing winter wheat foliage disease severity using aerial imagery acquired from small Unmanned Aerial Vehicle (UAV)", Computers and Electronics in Agriculture, 176:105665, DOI: 10.1016/j.compag.2020.105665, 2020
Landivar J., J. Jung, A. Ashapure, M. Bhandari, M. Maeda, J. Landivar, A. Chang and D. Gonzalez. 2021. In-Season Management of Cotton Crops Using “Digital Twins” Models. ASA-CSSA-SSSA International Annual Meeting, Salt Lake City, UT, November 9-11, 2021.
Landivar J, M. Maeda, A. Chang, J. Jung, J. McGinty, C. Bednarz, 2021. "Estimating the time and rate of harvest aid chemicals using an Unmanned Aircraft System," 2021 Beltwide Cotton Conferences, Online Conference, January 5 - 7, 2021
Ashapure A., J. Jung, A. Chang, S. Oh, J. Yeom, M. Maeda, A. Maeda, N. Dube, J. Landivar, S. Hague, W. Smith, 2020. "Developing a machine learning based cotton yield estimation framework using multi-temporal UAS data", ISPRS Journal of Photogrammetry and Remote Sensing, vol. 169, pp. 180-194.
J. Jung, M. Maeda, A. Chang, M. Bhandari, A. Ashapure, J. Landivar, "The potential of remote sensing and artificial intelligence as tools to improve the resilience of agriculture production systems", Current Opinion in Biotechnology, vol. 70, pp. 15-22, 2021
07/24/2023
09/05/2023