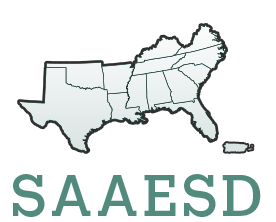
S1090: AI in Agroecosystems: Big Data and Smart Technology-Driven Sustainable Production
(Multistate Research Project)
Status: Active
S1090: AI in Agroecosystems: Big Data and Smart Technology-Driven Sustainable Production
Duration: 10/01/2021 to 09/30/2026
Administrative Advisor(s):
NIFA Reps:
Non-Technical Summary
Statement of Issues and Justification
The need as indicated by stakeholders
The competitive nature of modern agriculture demands agribusiness firms innovate and adapt quickly to capture benefits from advances in new technology such as artificial intelligence (AI). Throughout the value chain, there is a critical need to increase efficiency and protect the bottom line. Although currently lagging behind other industries, agriculture is forecasted to experience a “digital revolution” over the next decade. Global growth of AI applications in agriculture is expected to average 22.5% per year, reaching $186 billion by 2025, according to a recent report by Adroit Market Research. Growers, for example, are constantly exploring the best opportunities to increase yield and profit as expressed by Iowa corn and soybean producers at the recent NSF Convergence Accelerator Workshop for Digital and Precision Agriculture. Participants strongly voiced the need for new technology to answer “what is my best opportunity?” to enhance crop productivity and strengthen their bottom line.
Based on the National Science & Technology Council (2019) report, the American Artificial Intelligence Initiative was established in 2019 to maintain American leadership in AI and ensure AI benefits to the American people. The initiative set up long-term investments in AI research as one of the priorities. Following the initiative, USDA/NIFA invests in many AI-related programs such as the Food and Agriculture Cyberinformatics and Tools (FACT) and other programs for crop and soil monitoring systems, autonomous robots, computer vision algorithms, and intelligent decision support systems.
AI allows computers and machines to carry out tasks without human cognition. AI includes machine learning (ML) and deep learning (DL). Machine learning is a data analysis method to imitate human learning. Examples of using ML in agriculture include crop production (machine vision, yield prediction, pest and disease detection, and phenotyping) and agricultural robotics (navigation, self-leaning, man-machine synergy, and optimization). Deep learning is a subset of machine learning and consists of artificial neural networks to mimic human brain functions. Recently, deep learning has driven huge improvements in various computer vision problems, such as object detection, motion tracking, action recognition, pose estimation, and semantic segmentation (Voulodimos et al., 2018). Internet of Things (IoT) is a technology that enables data acquisition and exchange using sensors and devices through a network connection. IoT tends to generate big data, which requires AI to make valuable inferences.
Crop growth is a complex and risky process and difficult for producers to analyze in isolation. The emergence of information technology has developed a large amount of data, i.e., big data, that can be analyzed utilizing AI to provide valuable decision support to producers, particularly large-scale operations. AI can provide predictive analytics for growers to better manage risks through improved preparation and response for unexpected events such as severe flooding and drought. The Colorado-based company, aWhere, uses machine learning algorithms in connection with satellites and 1.9 million weather stations (virtual) to predict daily weather patterns for clientele, including farmers, crop consultants, and researchers. Improved crop and irrigation planning assist a global network of growers in reducing water usage with a particular focus on the impacts of climate change.
Robotics and visual machine learning platforms enable automation of critical labor activities such as field scouting and harvesting. Pest control companies have begun using aerial drone technology to cut costs in the labor-intensive practice of scouting pests and diseases. According to Brian Lunsford of the Georgia-based Inspect-All company, “In 2016, we performed our first paid drone inspection after months of testing. Most importantly, we wanted to make sure our flight operators could safely fly our drones and provide our customers with substantial value, while at the same time being mindful of privacy concerns.” As reported by Protein Industries Canada, AI-assisted pest and disease monitoring systems can save pesticide use up to 95% and reduce costs by $52 per acre.
Emerging AI technologies are expected to reach a broader spectrum of the value chain. While prior technologies focused primarily on grain commodities, machine learning and advanced visual machine learning algorithms will enable stakeholders from fruits and vegetables to improve harvest efficiency using platforms such as flying robot pickers. Early adopters such as John White of Marom Orchards support the use of AI in response to the need “to pay wages, organize visas, housing, food, healthcare and transportation” for a large number of workers and to address critical labor shortages due to “hard, seasonal work and other crops can pay higher wages. Young people all over the world are abandoning agricultural work in favor of higher paying, full-time urban jobs.” Small, labor-intensive farm operations can thus expand production opportunities by reducing harvest losses by 10% while reallocating freed-up labor to alternative enterprises, alleviating concerns over expected labor shortages that are expected to reach $5 million by 2050, according to Marom Orchards.
Downstream on the value chain, AI is also expected to have a strong demand over the coming decade. A recent article in Food Online lists three new types of AI technology to improve supply chain management, including: (1) food safety monitoring and testing of product along the supply chain; (2) improved marketing analysis of price and inventory; and (3) a comprehensive “farm to fork” tracking of product. In the food processing industry, visually based AI algorithms combined with machine learning are used by companies such as TOMRA Sorting Food to “view food in the same way that consumers do” and sort products based on consumer preferences and quality. AI reduces labor time compared to manual sorting, reduces wasted product, and enhances product quality delivered to consumers. To fine-tune product development and optimally satisfy consumer preferences, startup companies such as Gastrograph AI use machine learning to assist their clientele in fine-tuning product development. According to their website, their services ``interpret and predict flavor preferences for over a billion unique consumer groups. This technology empowers companies to look beyond trends, and formulate unique, novel and successful food and beverage products based on custom sensory intelligence.” Visually based AI and machine learning are also expected to be in high demand to improve hygiene in both manufacturing plants and restaurants. The use of AI in the cleaning of manufacturing equipment is projected by the University of Nottingham researchers to reduce cleaning costs by up to 40%.
The importance of the work, and what the consequences are if it is not done
There are approximately 1 billion people who have no access to clean water or sanitation. The same number have insufficient food to prevent them from chronic hunger, and ultimately, starvation. To tackle those challenges, improvements in agricultural production systems must be achieved, which requires a better understanding of the complex agricultural ecosystems. AI is projected to be one of the primary drivers to help study complex systems. Recent advances in AI, including big data, machine learning, and robotics, have helped achieve breakthroughs in various areas, such as healthcare, medicine, marketing, manufacturing, autonomous driving, etc. The field of agroecosystem has seen increasing applications of AI in recent years as well. However, more research must be done to transfer the general AI technologies into agroecosystem-specific AI technologies.
The importance of this project lies in that it will help tackle multiple pressing challenges that we are currently facing in the agroecosystem. The current AI technologies are not explicitly tuned for agroecosystem, which causes problems such as low accuracy in prediction, inefficiency in using computer resources, inefficiency in data management, and not being cost-effective for most agricultural crop productions. Also, the lack of next-generation farmers and workers in this area will be a major bottleneck for adopting and applying AI technologies. Included in this project, multiple AI-centered projects will be conducted at multiple states in the southeast U.S. to develop AI tools suitable for specific applications important to the improvement of production and sustainability of the agroecosystem. The project will also assess the feasibility of different AI technologies and showcase the value of those technologies to stakeholders to improve AI adoption. Additionally, this work will help develop the workforce for the future agricultural production system. Tasks in this project should be completed quickly and efficiently to ensure that production in agriculture meets the global needs in the near future and ensures the sustainability of the agroecosystem. It is also essential that technologies in agriculture must keep up with technologies in other fields to attract more talented people to ensure workforce sustainability.
The shift of agriculture to intensive production over the past several decades has led to a dramatic increase in the use of chemical inputs, particularly fertilizer. Although necessary to feed a growing world population, agriculture is a significant contributor to climate change. In a typical year, agriculture’s carbon and related environmental footprints account for 10% to 15% of the world’s greenhouse gas (GHG) emissions. Fertilizer is particularly problematic, contributing about 2.5% of the world’s GHG emissions. Broadcast applications of fertilizer are inefficient, with large portions remaining unused. Subsequent erosion and runoff pollute local watersheds resulting in algae blooms and related environmental problems. Fertilizer GHG emissions are often the most severe: nitrous oxide, for example, has been found to warm the atmosphere about 300 times more compared to CO2.
Artificial intelligence is expected to greatly assist in reducing agriculture's environmental footprint and contributions to climate change while continuing to increase productivity and input efficiency. Our project will develop a suite of AI-enabled technologies using sensors, actuators, and robotic platforms to increase the precision and efficiency of farm and ranch management practices, reducing overall input use and GHG emissions. Such precision-based agriculture is expected to play a critical role in mitigating agriculture's contribution to climate change. Projections from the World Economic Forum indicate that GHG emissions and water use could be reduced by 10% and 20% if precision agriculture were adopted on upwards of 25% of farms worldwide.
Livestock agri-food systems are at the crossroads of human, animal, and environmental health, and animal welfare is a priority in all livestock systems globally (FAO, 2018). Good animal welfare requires disease prevention, veterinary treatment, appropriate management, nutrition, and humane slaughter of livestock. Increasing standards for animal welfare has led to considerable research activities into ways to monitor and measure a wide range of traits that can be used for management (Bell & Tzimiropoulos, 2018). Due to the high labor cost and inaccuracy of traditional methods of expert evaluation, there is increasing awareness that the monitoring of animals requires the adoption of innovative AI technologies (Nasirahmadi, Edwards, & Sturm, 2017).
The technical feasibility of the research
The technical feasibility of the research can be summarized in three aspects. Firstly, the applications of the most recent AI technologies have been proven effective and helped improve various areas in the agroecosystem. As presented in the literature reviews of this proposal, AI has helped in areas such as yield prediction, crop quality assessment, environmental monitoring, phenotyping, genotyping, etc., in the past decade. Furthermore, an extensive number of studies have shown the power of AI and its potential in improving production and sustainability in most, if not all, aspects of the agroecosystem.
Secondly, the research activities proposed in this project are based on substantial preliminary studies. Team members on this project have expertise in remote sensing, machine vision, robotics, automation, UAVs, AI models, water management, hydrology, soil spectroscopy, natural resource economics and policy, and ecosystem services evaluation. All members have had substantial experience in conducting research, teaching, and extension activities in their respective focus areas. Proposed activities stem from the team’s preliminary studies, which ensures the technical feasibility and success of this project.
Thirdly, the team proposed a closed-loop method to balance the impact and feasibility of the research. Feedbacks gathered from extension activities will be communicated to the team through regular meetings to help refine objectives and approaches throughout the entire duration of the project. In addition, information such as newly discovered constraints, changes in specific operations in each production system will be shared and discussed to facilitate evaluating the technical feasibility of each sub-objective.
The advantages for doing the work as a multistate effort
Each state has different experts in different research areas. Also, different crops are grown in different states, and their cropping conditions are not the same. Therefore, working as a multistate team creates more opportunities for collaborating in various disciplines from other states.
From the survey completed in May 2021 at SAASED Institutions (SAASED, 2021), only 46% of the respondents have developed a partnership with other institutions. The survey also found that it is less likely that researchers know what other institutions are doing, and there is not much coordination among them. Therefore, this multistate project will facilitate more productive collaboration through organized coordination to complete the tasks proposed in this project.
During the proposal development stage in May-July 2021, we had meetings every one or two weeks, and there were 15-20 participants for most of the meetings. As a group, we came up with the title, defined objectives, and established the writing team for each specific objective. For each objective, a leader was chosen to lead the writing activities. All members were committed to accomplishing their assigned tasks of writing an introduction, literature review, and detailed activities, along with outputs, outcomes, and milestones, which resulted in this proposal.
During the project period, all participants from multiple institutions will meet once a year to discuss their research activities, major findings, current issues, funding opportunities, potential collaborations, and future directions. A listserv of emails will be created to facilitate efficient communication among the participants. An online cloud folder will be created to share and maintain data and information. By the end of this project, we anticipate multiple co-authored journal publications, funded research projects, outreach events, and Extension publications among the participants.
What the likely impacts will be from successfully completing the work
The likely impacts of this project include better preparation for harvest and storage with improved yield prediction, the timely intervention of control measures to address pest infestation, and reduced loss associated with low-quality produce in the supply chain.
Another potential impact is that farmers are able to maximize their return through timely harvesting, application of treatments to reduce loss, and consumers’ confidence through assurance of high-quality products. For fruit crops, automated fruit detection systems will enhance site-specific crop management practices to increase yield and profit.
For scouting and monitoring natural resources, the developed calibration models in this project can serve various stakeholders, including farmers and USDA-NRCS, to rapidly derive soil properties, which will reduce the cost and time of their projects. In addition, new technological improvements will be introduced, which can be utilized to develop new in situ sensors for the rapid estimation of soil properties.
This research will provide future scenarios projecting economic and social impacts from the adoption and use of AI technology. It is expected that results will be disseminated to producers, policymakers, and stakeholders and will be used as a key input in decision-making. This will provide more informed and improved choices resulting in more streamlined and socially optimal agricultural practices and policy outcomes.
AI-based algorithms depend heavily on the existence of large, clean, and information-rich databases. By combining multiple datasets from multiple states, the users will see a dramatic increase in their algorithms’ predictive measures and pattern recognition ability.
Related, Current and Previous Work
AI for yield prediction of crops
Agricultural production aims to improve the productivity of crops with reduced inputs while minimizing the impact of production on natural resources and the environment. Accurate yield estimation in a growing season is essential for managing production inputs, planning harvest and storage requirements, crop insurance purposes, and marketing (Chlingaryan et al., 2018; Kim et al., 2019). Several factors, including management, climate change, water availability, crop genetics, and the physical and chemical properties of soils, pests, diseases, and weed pressures, cause variability in crop yield (Liu et al., 2001). Despite the developments in weather forecasting, crop modeling, crop monitoring techniques, satellite remote sensing, and increased use of UAVs, developing reliable and efficient in-season yield prediction models is challenging. AI tools such as machine learning and deep neural networks provide various approaches in handling large amounts of data in developing yield prediction models for various crops. A systematic literature review on crop yield prediction using machine learning was published by von Klompenburg et al. (2020). They reported that the models that used more features did not provide the best performance for yield prediction. It was also reported that random forest, neural networks, linear regression, and gradient boosting tree were used more than the other methods. Among the neural network models, convolutional neural networks (CNNs), DNN, and long-short term memory were the most widely used models (van Klompenburg et al., 2020). Applications of AI techniques for yield prediction of other crops include wheat (Pantazi et al., 2016), sorghum (Zannou & Houndji, 2019), soybean, and corn (Maimaitijiang et al., 2020; T. Drummond, A. Sudduth, Joshi, J. Birrell, & R. Kitchen, 2003), and rice (Gandhi, Petkar, & Armstrong, 2016).
Yield prediction is essential for the planning and logistics of specialty crops. Unlike grains, the geometrical parameters of fruits and vegetables vary. These crops also do not reach maturity simultaneously, so multiple harvesting within a few weeks to a month is needed. Pre-harvest prediction of the yield of these crops provides opportunities for the producers to plan their harvesting and logistics operations and increase their profitability by achieving an early market competitive pricing (Braddock, Roth, Bulanon, Allen, & Bulanon, 2019; Chen et al., 2019a). The current method of estimating fruit yield is based on historical yield data or sampling several trees or plants and counting the number of fruits ((Braddock et al., 2019; Chen et al., 2019a; Cheng, Damerow, Sun, & Blanke, 2017). This method is time-consuming, labor-intensive, and may not be accurate. Using AI, early yield prediction using image processing and neural networks for apples developed. Cheng et al. (2017) used fruit features and tree canopy features for early yield estimation of apples using a backpropagation neural network algorithm. Chen et al. (2019a) used a deep neural network and predicted strawberry yield using the number of strawberry flowers extracted from UAV orthomosaics images.
Artificial neural networks have also been used for pepper fruit yield estimation with high accuracy (R2 = 0.97) based on the response to traits, plant height, canopy width, number of fruits per plant, fruit water content, and reproductive stage duration (Gholipoor & Nadali, 2019). Image processing and machine learning algorithms were applied for fruit detection and yield prediction for tomatoes (Yamamoto, Guo, Yoshioka, & Ninomiya, 2014), apricot (Blagojević, Blagojević, & Ličina, 2016), apple (Ji et al., 2021), and eggplant (Naroui Rad, Ghalandarzehi, & Koohpaygani, 2017).
AI for animal health and welfare monitoring
Recent advances in machine learning algorithms made it possible for individual animal analysis. For example, unsupervised machine learning methods such as Otsu’s (Nilsson et al., 2014) and K-means (Nahari et al., 2017) were used for segmenting pigs and cows from their background. More advanced analyses such as posture and behavior recognition typically require supervised machine learning methods. For example, Guo et al. (2016) applied a support vector machine (SVM) algorithm to data from 3D cameras to analyze the gaits of pigs. Tsai & Huang (2014) also applied SVM on top-view 2d images to detect estrus and mating behaviors in cattle. Viazzi et al. (2012) developed a machine vision system to detect the back posture of dairy cows and used decision tree classifiers for the early detection of lameness. Nasirahmadi et al. (2017) used a multilayer perceptron (MLP) neural network to automatically classify the group lying behavior of pigs into three thermal categories: cool, ideal, or warm temperatures. Oczak et al. (2014) applied a multilayer feed-forward neural network to classify aggressive behaviors in pigs. The majority of the studies focused on large animals in confined settings that body parts can be captured relatively easily by cameras. There were few studies focusing on poultry behavior analysis, however, only under lab settings. For example, Zhuang et al. (2018) conducted a series of experiments to develop a machine vision system for sick broiler detection. They utilized K-means to segment single broilers from a black background and applied SVM to classify sick and healthy broilers based on posture features. The method achieved an accuracy of 99.5% in classifying the broilers using their test data. de Alencar Nääs et al. (2020) designed a lab platform with a blue background and recorded videos of a single broiler walking on the platform. They applied computer vision algorithms to automatically detect the broiler’s speed and acceleration. Then, they were combined with each broiler’s genetic strain and sex information and manually labeled gait scores to train a decision tree model for gait score estimation. Using the 3-point gait score (GS0 is a sound bird, and GS2 is a lame bird), the team obtained a model with an accuracy of 78%.
AI for improved robotic systems
There are a number of distinct sub-domains within agricultural robotics where AI/ML have direct applications. The following research topics routinely use AI/ML to solve problems, such as 1) scene interpretation, object detection and localization, 2) mapping, planning, and navigation, 3) vision-based control and task execution, and 4) Robotic fleet management and self-diagnostics. These topics incorporate a significant body of work and are the subject of complete textbooks in academia. We will only briefly touch on some of their background applications in agroecosystems.
Recent advances in machine learning have paved the way for the development of superior object detection algorithms using deep learning (DL) techniques. Fruit detection and localization methods are at the core of automated yield estimation and robotic harvesting. The accuracy and efficiency of these methods can significantly impact the economic viability of robotic harvesting solutions. To improve the fruit detection efficiency, various DL architectures have been investigated, Rahnemoonfar and Sheppard (2017), Mureşan and Oltean (2018), and Zhang et al. (2019). Sa et al. (2016) adopted the Faster R-CNN architecture for sweet pepper detection using multi-modal input data consisting of RGB and Near-Infrared (NIR) images and later for strawberry, apple, avocado, mango, and orange detection. Ganesh et al. (2019) used a Mask R-CNN (He et al., 2017) network, named Deep Orange, for detection and segmentation of oranges to obtain a pixel-wise mask for each detected fruit in an image. One of the barriers in the use of DL is the need for large training datasets (Kamilaris and Prenafeta-Boldu, 2018), which also increase the training time since data annotation is required in the majority of the cases. To decrease the size of the network and thereby decrease the need for large amounts of training data, (Volle et al., 2020) presented a segmentation approach that uses a small version of the U-Net (Ronneberger et al., 2015) architecture to generate masks to identify and localize oranges in an image.
Navigation in orchards is considered more challenging than in the open field of most agronomic crops. Over the years, many methods have been created to navigate ground robots in orchards. Machine vision and laser scanner-based methods were used most frequently. Subramanian et al. (2006) developed a machine vision and laser radar (LiDAR) based guidance system for citrus grove navigation and achieved average positioning errors of 28 mm using machine vision and 25 mm using the laser radar in a straight citrus grove alleyway. Barawid et al. (2007) developed a navigation system for an orchard using a two-dimensional laser scanner. They applied Hough transform to fit lines along with detected tree canopies and provided lateral offset and heading measurements. Similarly, Bayar et al. (2015) developed a model-based control method in which a laser scanner was used to detect the relative positions of fruit trees for central line calculation. Sharifi and Chen (2015) classified RGB images taken from a mobile robot in an orchard row into classes based on graph partitioning theory and then applied Hough transform to determine the central path in a row for navigation. All these works presented satisfactory results in cases of the single row following using machine vision or laser scanning techniques. Multi-sensor fusion is another technique used for robot navigation in orchards. Kise et al. (2002) used an RTK-GPS and an IMU to develop a steering controlling algorithm for an autonomous tractor. Iida and Burks (2002) combined DGPS and ultrasonic sensors to provide navigation of a tractor in orchards. Hansen et al. (2011) fused odometry and gyro measurements with line features created by 2D laser scanner data using derivative-free Kalman filters and navigated a tractor in orchards. In orchards navigation, methods using multi-sensor fusion, especially, GPS-based sensor fusion methods, were not studied as much as machine vision and LiDAR-based methods. However, GPS-based navigation solutions are still used frequently in practice due to their simplicity and robustness to environmental noises.
Vision-based control is the most popular control technique used in robotic harvesting, as well as in vehicle navigation. The objective of vision-based control in robotic harvesting is to autonomously position the robot in relation to fruit for successful detachment using measurements provided by the vision system. A comprehensive overview of robotic systems and vision-based control in agriculture can be found in Bac et al. (2014) and Zhao et al. (2016). Vision-based control approaches in robotic harvesting can be defined as either open-loop or closed-loop control. Although open-loop control systems are simple, they may suffer from excessive positioning errors in outdoor agricultural environments since continuous image feedback is not provided to correct robot position with respect to the fruit. Closed-loop vision systems employ continuous image feedback as reported by Harrell et al. (1985) and then direct visual servo control that tracks fruit centroids in Harrell et al. (1989). Edan et al. (2000) used a cooperative sensing framework consisting of two monochromatic cameras serving as a far-vision sensor and a near-vision sensor. Bulanon et al. (2005) considered an end-effector mounted camera and laser ranging sensor for an apple harvesting robot. Although the stability of the closed-loop control system is critical in achieving high harvesting efficiency, most of these results paid little or no attention to rigorous controller formulation and stability analysis. To guarantee and improve the stability of robotic harvesters, Mehta and Burks (2014) presented a hybrid control framework to control the 3D translation of the camera. The controller in Mehta and Burks (2014) guarantees exponential regulation of the robot to a target fruit. Subsequently, the hybrid control design was extended to develop robust and adaptive visual servo controllers in Mehta et al. (2016) and Mehta and Burks (2016), respectively, to compensate for the unknown fruit motion that may arise due to environmental disturbances. Recent results in Chen et al. (2019b) developed a sliding mode controller for apple harvesting based on fuzzy neural networks to reduce chattering that is commonly associated with sliding mode control and proved to be asymptotically stable, which is a weaker notion than exponential stability.
A robotic harvester system can be considered as a collection of components (e.g., fruit detection, fruit localization, manipulation, gripper, servo control, mobile platform, material handling, etc.) whose individual efficiencies dictate the overall economics of harvesting. The same concepts apply on a broader scale to fleets of autonomous robotic systems. Not only can AI be useful in fleet task planning and optimization, in collision avoidance, but also in fleet diagnostic. By diagnosing the various components of a system or fleet, and servicing the components operating at subpar efficiencies, any loss in the value of production can be reduced and the economic outcomes enhanced. Artificial intelligence is already being studied and applied in a broad range of fleet applications in the general industry, while being explored in application to agricultural robotic fleets.
AI for natural resources scouting and monitoring
Increased availability of soil data that can be acquired remotely and proximally and freely available algorithms have accelerated the adoption of machine learning to analyze soil data (Padarian et al., 2020). This has permeated into soil carbon and health as well. The application of AI in soil carbon has been demonstrated in digital mapping of carbon fractions (Keskin et al., 2019), carbon stock estimation using satellite images (Pham et al., 2021), climate-sensitive soil carbon stock mapping using field samples (Bui et al., 2009; McNicol et al., 2019), modeling organic carbon change (Heuvelink et al., 2020), and estimating soil health indicators and properties using spectroscopic data (Morellos et al., 2016; Ng et al., 2019; Sanderman et al., 2019).
Deep learning algorithms, particularly various deep neural networks (DNN), have been recently investigated for the prediction of WQ parameters and HABs based on RS data. Pyo et al. (2019) trained a CNN with two parallel fully connected regression heads to predict phycocyanin (PC) and chlorophyll-a (Chl) concentrations from airborne hyperspectral imagery. Yim et al. (2020) investigated a fully connected DNN model to predict PC from airborne hyperspectral imagery. They found that unsupervised pre-training of each DNN layer to predict the previous layer led to a 3% improvement in prediction accuracy compared to that without pretraining. Peterson et al. (2020) compared DNN with three conventional machine learning (ML) methods (MLR, SVR, and ELR) for estimation of six WQ parameters from Landsat-8/Sentinel-2 satellite imagery in the Midwestern US. The same team conducted a more comprehensive comparison (Sagan et al., 2020) where proximal spectral, proximal hyperspectral, and satellite spectral data were utilized. Both studies showed that deep learning models such as DNN and LSTM outperformed conventional ML for optically active WQ parameters estimation. However, estimation of non-optically active WQ remains a challenging task. The DNN-based multimodal spatiotemporal analysis could be a promising solution. Hill et al. (2020) developed the HABNet that combined CNN with LSTM to detect HAB events near the west Florida coastline from 12 time-series satellite RS products. The DNN model resulted in detection accuracy of 91%, showing the great feature-learning capability of DNN in multimodal spatiotemporal data.
AI for plant phenotyping and genotyping
Deep learning has significantly advanced the image-based characterization of plant phenotypes in the last five years. Specifically, deep CNNs enabled a wide range of image-based plant phenotyping tasks, which were often challenging to solve with conventional image processing and machine learning algorithms. The tasks that deep CNNs can solve include image classification, regression, semantic segmentation, object detection, and instance segmentation. CNN-based classification has been studied for identification of plant species (Dyrmann et al., 2016; Barré, 2017), rosette mutants (Ubbens & Stavness, 2017), leaf disease (Mohanty et al., 2016; Ghosal et al., 2018), wheat shoot and root features (Pound et al., 2017), and wheat lodging (Zhao et al., 2020). The network architecture for classification typically involved a backbone CNN as a feature extractor followed by a multilayer perceptron network. Similar network architecture is normally used for regression, except that the output of the network is a numeric value instead of class probabilities. CNN-based regression models were developed for tasks such as rosette leaf counting and age estimation (Ubbens & Stavness, 2017), maize tassel counting (Lu et al., 2017), soybean seed-per-pod estimation (Uzal et al., 2018), and wheat awn morphology and flowering scoring (Wang et al., 2019). CNN-based semantic segmentation (i.e., pixel-level classification) was also used for phenotyping tasks such as plant organ counting. Pound et al. (2017) used an hour-glass network architecture to localize wheat spike and spikelet pixels. Malambo et al. (2019) adapted a VGG-16 model (Simonyan & Zisserman, 2014) for semantic segmentation of sorghum panicles from UAV images. Lin and Guo (2020) used a U-Net for the same application. Wu et al. (2021) developed SegNet for semantic segmentation of rice culms in micro-CT slice, which further enabled 3D reconstruction of culms for rice lodging resistance characterization
Compared to the semantic segmentation approach, CNN-based objection detection provides a more end-to-end solution for plant and plant component identification and localization. Jin et al. (2018) trained a Faster R-CNN model to detect in-field maize stems from 2D images converted from 3D point clouds acquired by a terrestrial LiDAR. Baweja et al. (2018) used the Faster R-CNN developed by Ren et al. (2015) coupled with an hour-glass network to detect and segment sorghum stalks, respectively, from RGB stereo images collected on a ground robot. The results were further processed for in-field sorghum stalk counting and stalk width estimation. Madec et al. (2019) found that Faster R-CNN was more robust than the CNN-based regression method for wheat ear counting in UAV images. Yu et al. (2020) employed Faster R-CNN to detect and count flowers on individual cotton plants from close-range multi-view RGB images collected with a high-clearance ground vehicle.
Deep CNNs have been mostly used on RGB plant images. Analysis of plant imagery of modalities other than RGB can also benefit from deep CNNs. Han and Gao (2019) investigated deep CNNs to detect pixel-level aflatoxin contamination on peanut kernels from hyperspectral imagery. Jin et al. (2020) developed a 3D voxel-based CNN model with an encoder-decoder structure to segment leaves and stems from terrestrial LiDAR-scanned 3D point clouds of in-field maize plants.
The lack of open-source, public data is one of the identified bottlenecks in the fast prototyping and evaluation of AI algorithms for various agricultural management tasks (e.g., weed control and harvesting) (Lu and Young, 2020). For example, a common computer vision-based precision agriculture task is presumably the goal of detecting the objects of interest (e.g., crop, weed, or fruit) and discriminating them from the rest of the scene.
Turning to AI applications will allow researchers to develop new tools for farmers, extension agents, and researchers that will help them make better use of all data collected throughout the farming operations. A big bottleneck in the use of these new applications is the non-traditional aspect they propose, which is not explained in any statistics-oriented program (Samek et al., 2017). Hence most of the time, the algorithms are considered a high-level black box by the researchers who thus hesitate to use them (Linardatos et al., 2021). Educational-informational programs on AI will thus be among the priorities of this multistate project.
Standardization and testbed development
World and U.S. agriculture production systems face daunting challenges from the changing climate, as well as reductions in the amount and quality of available soil and water. These challenges threaten the resilience, environmental and economic sustainability of current and future food supply systems (Andersen et al., 2018). Agriculture is keeping pace with climate change, but innovations will be needed to ensure its adaptability in the future (Hatfield et al., 2014). More than ever, scientific and technological advances in agriculture are needed to deal with the challenges mentioned above and increase agriculture productivity. Traditional research has resulted in the availability of large volumes of information from different components within the agricultural system.
Economic analysis of technology adoption in agroecosystems
Studies have found that current digital or precision technologies generally increase crop yield and farm profitability. This includes variable rate technology (VRT), the coupling of soil and yield sensors with VRT applicators, which enables farmers to apply optimal quantities of input applications such as seeds, fertilizers, and pesticides at finer levels of resolution than conventional farming, e.g., at sub-field or even plant-level (Faulkner and Cebul 2014; Calabi-Floody et al. 2018). European Parliament (2014) found that about 68% of adoption cases reported increased profitability. Similar results were found by OECD (2016). For soybeans, potatoes, and wheat, the corresponding numbers are 100%, 75%, and 52%. In terms of the magnitude of increase in net returns due to precision technologies, Schimmelpfennig (2016) documented that corn farms that adopted some precision technologies had net returns only about 1% to 2% higher than corn farms that did not adopt any precision technologies. A literature review conducted by Griffin and Lowenberg-DeBoer (2005) found that about 73% of reviewed studies focusing on corn reported profit increase from using precision agricultural technologies.
A significant gap in the literature exists since most applications of new technology have been narrowly focused on their technical merits rather than more complete economic, social, and environmental assessments. Economic surplus methods are used when evaluating the downstream impacts of new technology on both producers and consumers through welfare measures that summarize expected gains (or losses) following the introduction of new technology (Alston et al.). This type of approach has been used in numerous studies to assess the economic impacts of introducing new agricultural technology in crop production (Moschini et al., 1999; Traxler and Falck-Zepeda, 1999; Falck-Zepeda et al., 2000; Elbehri and MacDonald, 2004; Huang et al., 2004; Jefferson-Moore and Traxler, G., 2005; Frisvold et al., 2006; Langyintuo and Lowenberg-DeBoer, 2006). The economic surplus method estimates how new technology would affect market supply using a supply-demand framework that details how markets respond to price movements (MacCarl). Risk modeling analyzes the production, price, and financial risks that pervade producers' decision-making environment, including procedures to characterize risk attitudes of farmers (Warren; Hardaker, Huirne, and Anderson; King and Robinson; Hardaker et al.; Richardson). Environmental impact analysis relies extensively on biophysical simulation models such as EPIC (Sharpley and Williams) or SWAT (Srinivasan et al.). Soil and Water Assessment Tool (SWAT) model was developed to simulate the hydrological implications (sediment, chemical, and nutrient loading) of farm management practices on adjacent waterways, including groundwater, lakes, rivers, and streams over long periods of time. Simulation models are expected to be integrated into big data formats, and future versions could be expanded to include machine learning algorithms to greatly enhance modeling accuracy and power.
Objectives
-
Introductory remark
Comments: While the foregoing review covers substantial breadth in the application of AI to agricultural and environmental issues, coordinated efforts are only beginning. Three key areas are the focus of coordinated efforts in this multistate project: (1) applying AI techniques to agricultural and environmental problems; (2) developing methods for applying AI that enable broad, efficient, and appropriate use for agricultural and environmental problems, and (3) extending the knowledge developed in these efforts to the public. Based on these three areas, we have developed the following three main objectives, in which detailed sub-objectives indicate the diversity of scientific interests among the research team. -
Obj. 1. Develop AI-based approaches for agroecosystems production, processing, & monitoring
Comments: Sub-objectives: a. AI tools for crop and animal production b. AI tools for autonomous system perception, localization, manipulation, and planning for agroecosystems c. Natural resources scouting and monitoring d. Socioeconomic sustainability e. Phenotyping and genotyping -
Obj. 2. Data curation, management, accessibility, security, and ethics
Comments: Sub-objectives: a. Develop open-source, public agricultural datasets for benchmarking AI algorithms with a focus on explainability b. Standardization and testbed development -
Obj. 3. AI adoption (technology transfer) and workforce development
Methods
Objective 1: Develop AI-based approaches for agroecosystems production, processing, & monitoring.
The intent of this multistate project is to approach each objective in an integrated fashion in which multistate teams address each, with the expectation that the overall multistate team will share progress and new knowledge across subobjectives. Furthermore, the five subobjectives in Objective 1 are naturally interdependent. For example, crop production (subobjective 1a) has significant effects on environmental conditions (1d) and natural resources (1c). Moreover, breeding and phenomics (1e) and autonomous systems (1b) have significant effects on crop production (1a). Therefore, these subobjectives should be viewed and will be approached not as individual and separate objectives but as interdependent subobjectives in an overall objective focused on AI approaches for agroecosystems.
Obj. 1a. AI tools for crop and animal production.
A. Introduction
Crop yield depends on several factors that vary from field to field. A yield prediction model developed for a particular crop on a specific field will likely not work in another field. As the amount of data collected from satellites, aerial and ground sensors, weather forecasting, and plant genomics increase, new and more accurate, reliable, and robust yield prediction and quality evaluation tools are needed.
The advancements in AI tools and the improved computational power make it easier to handle and derive meaning from large amounts of agricultural data. The ability to process large amounts of data in real-time or near-real-time would help improve the productivity of agricultural crops with precise management of agricultural operations.
AI tools can also be used for various aspects of crop production, including crop status/growth monitoring, nutrient and water stress detection, pest/disease detection and management, weed detection and management, precision spraying, and postharvest processing and quality evaluation.
It is critical to developing methods for yield prediction that incorporate data that are likely to be available to farmers, such as historical yield, images from satellites and drones, surveys of soil fertility and electrical conductivity and topography data, and historical and current weather.
B. Detailed activities/procedures
The team will conduct research in the following areas: crop and animal status/growth monitoring; yield estimation/prediction; stress detection; and quality evaluation. Various imaging and non-imaging sensors will be used to collect data on crop status and growth. Data will be acquired with stationary sensors, ground robots, and UAVs.
A major difficulty in predicting crop yield is the fact that the data used are collected at specific, different times. For example, an instance of remote-sensing data may be taken during the flowering period in the current season, while a set of historical yield data may have been taken at the end of the season two years ago. Data collected at different times can be represented as a sequence, and an approach being used at Mississippi State University involves AI based on sequence learning. This method involves (1) a network into sequences of arbitrary length that can be fed one element of the sequence per time step, and (2) a network that can remember important events that happened many time steps in the past. A variety of different recurrent neural networks will be considered.
For example, color images will be acquired to monitor various crop growth statuses and detect crop nutrients, yield, disease, and weeds. Thermal images will be used for detecting crop diseases and water stress in the field. Hyperspectral imaging is costly and not suitable for implementation in crop fields. Thus, it will be used to identify important wavelengths toward the development of multispectral sensors.
While foundational AI research is not the intent of this project, these types of data will be used to apply AI algorithms for detecting and monitoring crop status. Various AI algorithms will be used, including convolutional neural networks (CNN), region-based CNNs, ResNet, You Only Look Once (YOLO), and single shot multibox detector (SSD).
A concern with outdoor image data is that it is heavily affected by varying illumination. We will train AI algorithms with images of varying illumination to explore whether AI algorithms can handle them. If not, AI algorithms will be trained with different illumination conditions and used separately for individual illumination conditions.
Quality evaluation, grading, and sorting of agricultural products are critical tasks at packing facilities to ensure the separation of high-quality, marketable grades of products and remove culls (low-quality, defective products) that are inferior or unmarkable. Furthermore, in the age of precision agriculture and traceability, commodity quality can conceivably be traced back to field position to enhance spatially variably crop management. Human inspection is routinely used for grading and sorting food products (e.g., sweet potato), especially when sorting for defects. This process is the most labor-intensive during postharvest handling. In addition, the human inspection may suffer from inconsistency and variability in selection induced by human subjectivity and other physiological factors. Moreover, packinghouses face a significant challenge of retaining well-trained workers, especially during a crisis such as the COVID-19 pandemic. Hence there is a pressing need to develop automated quality evaluation, grading, and sorting systems. AI-based machine vision (MV) will be investigated as a means to automate postharvest grading and sorting on production lines (Blasco et al., 2017) to offer improved efficiency, objectivity, and accuracy.
In addition to post-harvest quality evaluation, non-destructive testing of foods is needed for pest detection in produce, adulterant detection, etc. Near-infrared sensing and other sensing technologies like acoustic sensors are being developed to improve AI deployment for solving problems related to food quality assessment and safety assurance (Adedeji et al., 2020; Rady and Adedeji, 2020; Al Khaled, Parrish & Adedeji, 2021). Activities in these areas will include developing multispectral models that are based on AI tools like machine learning and deep learning from near-infrared (HSI, CV, etc.), acoustic, and other sensors.
Data expected to be commonly available to farmers will be collected on a cotton field, including soil electrical conductivity, soil type from a soil survey, historical yield data, satellite data, UAV data, historical and predicted weather data, etc. AI-based models will be developed to use these data in an attempt to predict yield with high spatial precision.
The team will also conduct research in animal health and welfare assessment using AI-based MV. Detailed activities may include estimation of cattle body weight and detection of broiler behaviors associated with key welfare indicators. Bodyweight is an important factor associated with many management practices in cattle production and a good indicator of cattle health (Uluta & Saat, 2001). Previous studies used cattle’s body measurements such as chest girth, body length, and wither height to predict body weight and achieved good results (Ozkaya & Bozkurt, 2009). In this study, the vision system will automatically capture the body measurements for bodyweight prediction. The 2D and 3D images will be combined to accurately extract each animal’s chest girth, hip-width, body length, and wither height. Models will be trained to associate the body measurements with body weight.
Obj. 1b. AI tools for autonomous system perception, localization, manipulation, and planning for agroecosystems.
A. Introduction
It is not possible to implement robotics and autonomous systems in unstructured environments such as production agriculture without some form of AI that can interpret the environment and make and effect decisions required for navigation, manipulation, and planning when coupled with sensors. Economic, agronomic, workforce, and environmental pressures, coupled with the need for complex robotic operations within limited equipment budgets, have resulted in a growing demand for AI approaches. The application of AI requires tremendous amounts of computations, and a new breed of embedded processors and GPU-enabled servers provide the potential for Edge computational capacity that can run complex models without cloud service (due to limited internet access in farm fields) to support robotic field operations.
B. Detailed activities/procedures
Our research activities will be concentrated on applying AI tools that will improve robotic perception, localization, manipulation, and planning for agroecosystems. We will derive a closed-loop system based on large datasets that first need to be collected and efficiently processed before the corresponding control actions can be derived. The information will come from sensors attached to unmanned platforms to support quick and accurate decisions.
Generally, a closed-loop system’s goal is to measure, monitor, and control a process by monitoring its output and comparing it to the desired output to reduce the error. The error, which is the difference between the input and feedback, is fed to the controller to reduce the system's error and bring the output back to the desired state. Model estimations are carried out similarly by estimating the values of parameters based on measured empirical data. Although this is based on known principles and processes, the models require extensive data (Cai et al., 2017). Moreover, this is often difficult to calibrate due to the complexity of the processes, limited availability of data across a wide range of environments, and a huge number of uncertain input parameters (Lobell & Burke, 2010). Artificial Neural Network (ANN) used feedback loops to learn from their mistakes (Nord, 2020). Machine Learning (ML) models aim to build empirical predictive algorithms using historical ground truth records and predict for the future. Predictions derived from these approaches are not directly based on physiological mechanisms, which have the advantage of forecasting without relying on specific parameters (Medar & Rajpurohit, 2014; Crane-Droesch, 2018). Although different ML have been explored specifically for agriculture applications (Bolton & Friedl, 2013; Kaul et al., 2005; Zhang et al., 2019), these methods may fail when directly applied to complex and big data. Thus, it is critical to developing effective feature extraction strategies to reduce dimensionality and use appropriate features as input for building the predictive models. We will develop nonlinear feature extraction and supervised feature extraction approaches for data reduction. Specifically, CNNs will be used to discover the most relevant information which is best for the model development and improve prediction accuracy.
The technological approaches being proposed require algorithms that are computationally expensive to train, implement and tune. As a result, we also plan to explore, develop and implement approaches that will use various forms of edge processing either with systems like smart cameras or AI-enhanced embedded processors like Nvidia Jetson Nano or Xavier or with GPU enabled Edge servers on a local network in the field.
To develop and validate the predictive models and algorithms, we will conduct field trials at multiple environments and various geographical areas for their applicability. The field experiments will be conducted over multiple years. Different sensors (Lidar [2D & 3D], imaging, distance, rotary, GPS, IMU, etc.) and actuators will be used for the field trials. Research on the use of different protocols will be conducted through best practices from ongoing AI projects from multistate members. Pertinent results will be disseminated through peer-reviewed publications and presentations at national and international conferences.
Obj. 1c. Natural resources scouting and monitoring.
A. Introduction
The potential of soils to sequester carbon and consequently regulate atmospheric CO2 to mitigate climate change is widely recognized and has been an active research area for decades. Conventional methods of estimating soil carbon and health indicators involve intensive field and laboratory work that require money, time, and labor. Recent developments in sensing have created large volumes of soil data which can potentially be used for soil carbon and health measurements to complement or substitute for conventional laboratory methods. AI-based approaches can assist in identifying relationships between big data and soil carbon/health for real-world applications.
Water resources is another critical global issue. Nutrients (e.g., nitrogen and phosphorus) from agricultural run-off coupled with climate change (e.g., warmer temperature and rainfall anomalies) have led to an increase in algal blooms around the globe. Efficiently monitoring water quality with high spatial and temporal resolutions remains a challenge. Remote sensing has been researched to develop predictive models for water quality parameters, but not all water quality variables such as off-flavors and toxins cause changes in the spectral reflectance of surface water. The spatiotemporal dynamics of multimodal remote sensing data, weather data, and geographic data could all contribute to accurate predictions of the non-optically active water quality parameters and forecasting of HAB outbreaks. Deep learning has great potential to harness high spatiotemporal multimodal data and enable predictive models that can improve the decision-making of water resources managers and policymakers.
B. Detailed activities/procedures
The intended research activities under this objective will focus on the development of AI-based approaches for soil carbon stock estimation, monitoring, mapping, soil health evaluations, water quality and quantity parameter monitoring and estimation, and harmful algal bloom (HAB) forecasting. These approaches can use the available data sources such as satellite images, soil databases, water quality and quantity databases, and spectral libraries to produce accurate estimations of soil carbon and soil health indicators, water quality and quantity parameters, and HAB outbreaks required for farmers, water resources managers, and other stakeholders. The use of available data sources can drastically reduce the cost and time associated with the task. However, the application of such data in local or regional conditions can increase the uncertainty of estimates due to inherent global to local variations. AI-based approaches can potentially be used to either sub-sample the data to match the local conditions or implement smart algorithms to enable calibration transfer from global to local/regional scale. Also, expansion to a finer scale can be achieved by the incorporation of data from different sensors onboard sensor networks, machinery, ground vehicles, UAVs and Unmanned Ground Vehicles (UGVs). AI-based approaches can effectively be used to build models to incorporate such fine-scale data with global scale to improve the accuracy and uncertainty of the predictions.
Obj. 1d. Socioeconomic sustainability.
A. Introduction
Adopting AI to develop causal relationships among variables of interest is an emerging area of research in the production economics field. A recent study identified causal inferences based on the conditional average treatment effects (CATE) approach using the causal random forest algorithm (Athey and Wager, 2019). The least absolute shrinkage and selection operator (LASSO) analysis has also been popularly used and applied based on the causal random forest method (Ludwig et al., 2015). Agricultural production, agroecosystem services, and supply chain analysis are also sub-disciplines where AI and machine learning will continue to extract valuable information from data obtained from a variety of sensor and robotics-based platforms. Collaborating multi-disciplinary teams in this project will work together to develop econometric models based on AI and machine learning to identify causal linkages among variables, enabling more accurate predictions and forecasts of future production and environmental outcomes.
Advances in agricultural production from the adoption of new technology, while generating substantial overall societal gains, have often had negative impacts on other groups through various externalities and unintended consequences. Carolan’s (2016) inventory of overall “costs” and “benefits” to the community from the introduction of new technology lists far more negatives than positives, including greater community inequality and population decline from rural exodus, higher unemployment, and social disruption. The introduction of AI is expected to result in a further concentration of agricultural production and marketing towards big business and away from small family-owned farms and agribusinesses. The emergence of hi-tech internet firms is likely to occur with the introduction of AI as information-based new technology generates new sources of income. There is likely to be a concentration of critical technology in large-scale information providers leading to concerns over monopoly and monopsony market power. The large-scale shift of pork production from the family farm to CAFOs provides a cautionary tale for the likely transformation that AI could render on the US agricultural community. CAFOs have been responsible for welfare losses in rural communities, including environmental quality, lost public services as changes in retail trade drive old businesses out of the community, increased health concerns (upper respiratory, digestive tract disorders), reduction in outdoor activities, and community involvement, and increased energy demands. Hence, there is a need to consider a wider scope of impacts beyond net returns to producers and technology providers by considering welfare impacts on rural communities, consumers, and the environment.
The overall purpose of this sub-objective is to identify new AI technologies and techniques that would best enhance the profitability of US agriculture while maintaining a proper balance with environmental concerns and socio-economic equity. Given the complexities involved in the adoption and extension processes, this research will conduct comprehensive economic evaluations of AI developments within the US agricultural sector. This is anticipated to include both ex-post and ex-ante analysis on the impact of AI on agricultural production, farm labor, marketing, consumption, rural communities, and the environment.
B. Detailed activities/procedures
Evaluations will include the impact of each AI technology on expected net returns, economic risk, compatibility with existing production systems, resource endowments, and institutional constraints. Wherever appropriate, adoption surveys will be conducted to develop profiles of anticipated adoption rates and to identify constraints to adoption. The impact of AI technology is also expected to include producer surveys to obtain necessary data to operationalize the welfare models discussed above. This project will include but not be limited to the development of large-scale information systems, particularly robotic and UAV platforms.
Assessments will include the impacts of each AI technology on enhancing the environment through improved application of technology and farm practices. Such impacts are expected to reduce erosion and subsequent runoff and nutrient loading into watersheds, increase the efficiency of irrigated water use, and better sustain soil nutrient levels over the long run. The assessments will be conducted under the appropriate type of environmental compliance, voluntary or otherwise, which often place constraints and limits on producer’s choices. The analysis would also include weighing the tradeoffs between the economic and environmental impacts, to assess the costs of mitigating environmental damage. The analysis is expected to include complementary environmental models such as SWAT, EPIC, and similar bio-physical models discussed above.
There are methods that are well suited to studying the potential application of AI to varying farming situations. The larger scope of AI impacts will be analyzed using complementary models such as IMPLAN and CHANS that provide spillover effects into the rural economy, including multipliers. Survey interview data will be collected from rural communities to assist in decisions about which AI will best serve communities. Given the global importance of US agriculture, such applications will be extended to foreign markets, including developing countries.
The economic team will develop data integration approaches for various data sources generated by big data, machine learning, and AA. The research will focus on utilizing machine learning and AI techniques that can assist in identifying causal inferences in the economics framework. Such approaches generate much stronger and meaningful relationships and are substantial improvements over statistical models based on causal inferences rooted in correlation analysis.
The data collected and generated in other objectives in this project will provide data that will be processed on a finer resolution than previous research conducted using data sources such as the National Resource Inventories. This will include filling in missing observations, updating model parameters for simulation models, and providing new sources of research hypotheses based on new and more refined variables. This will enable the project to proceed with economic valuations of a more complete and meaningful set of variables to analyze social welfare changes.
Obj. 1e. Phenotyping and genotyping.
A. Introduction
In recent decades, plant genetics research has focused on developing crop varieties with enhanced traits such as high yield, environmental stress tolerance, and disease resistance (Cuenca et al., 2013; Rambla et al., 2014). Current breeding methods require many years to develop, select, and release new cultivars (Sahin-Cevik et al., 2012). New breeding methods, such as genomic selection, incorporate genomics, statistical and computational tools, and accelerate cultivar development (Vardi et al., 2008; Zheng et al., 2014; Albrecht et al., 2016). A key requirement for implementing these new breeding methods is creating a large and genetically diverse training population (Aleza et al., 2012). Hence, large-scale experiments in plant phenotyping are critical because the accurate and rapid acquisition of phenotypic data is important for exploring the correlation between genomic and phenotypic information. Traditional sensing technologies for evaluating field phenotypes rely on manual sampling and are often labor-intensive and time-consuming, especially when covering large areas (Mahlein, 2016; Shakoor et al., 2017). Additionally, field surveys for weed and disease detection to create plant inventory and assess plant health status are expensive, labor-intensive, and time-consuming too (Luvisi et al., 2016; Cruz et al., 2017; Cruz et al., 2019). Small unmanned aerial vehicles (UAVs) equipped with various sensors have recently become flexible and cost-effective solutions for fast, precise, and non-destructive high-throughput phenotyping (Pajares et al., 2015; Singh et al., 2016).
UAVs allow growers to constantly monitor crop health status, estimate plant water needs, and even detect diseases (Abdullahi et al., 2015; Abdulridha et al., 2018; Abdulridha et al., 2019). They represent a low-cost method for image acquisition in high-resolution settings and have been increasingly studied for precision agricultural applications and high throughput phenotyping. UAVs and machine learning, an application of AI, have been increasingly used in remote sensing for genotype selection in breeding programs (Ampatzidis and Partel, 2019; Ampatzidis et al., 2019; Costa et al., 2021). These methods have achieved dramatic improvements in many domains and have attracted considerable interest from both academic and industrial communities (LeCun et al., 2015). For example, deep convolutional neural networks (CNNs) are the most widely used deep learning approach for image recognition. These networks require a large amount of data to create hierarchical features to provide semantic information at the output (Cruz et al., 2017; Krizhevsky et al., 2012; Simonyan and Zisserman, 2015). With the increasing access to large amounts of aerial images from UAVs and satellites, CNNs can play an important role in processing all these data to obtain valuable information for breeding programs. Since labor shortage is a major issue, remote sensing and machine learning can simplify the surveying procedure, reduce labor costs, decrease data collection time, and produce critical and practical information for breeding programs.
B. Detailed activities/procedures
AI algorithms will be developed to analyze spatiotemporal multimodal sensor data at multiple scales for plant breeding programs in the Southern States. Specific crops include peanut and southern pine. Traits of interest will be focused on yield, yield components, drought tolerance, and disease resistance.
Proximal high-resolution RGB and depth imagery data will be collected using ground-based platforms (handheld, pushcart, tractor, and UGV) for plant- and organ-level phenotyping. Remote RGB, multispectral, hyperspectral, thermal, and LiDAR data will be collected using aerial platforms (UAV and satellite) for canopy-level phenotyping. Data collection campaigns will be carried out multiple times during each growing season.
Deep CNN-based instance segmentation algorithms will be used to detect and segment plant organs from proximal RGB/RGB-D imagery data. For peanut breeding, pre-trained Mask R-CNN models will be fine-tuned to detect infield peanut pods after digging from multi-view close-range RGB images. The detection results in conjunction with the yield data will be used to develop yield estimation models for peanut yield trials. The same approach will be used to detect leaf spot disease lesions to quantify disease resistance among the advanced breeding lines. For loblolly pine breeding, instance segmentation will be performed to detect and segment branches and trunks from RGB stereo images of loblolly pine trees in progeny tests. The segmentation results will be used to estimate tree architectural traits such as branch angle, branch diameter, and trunk diameter via stereo 3D reconstruction and point cloud analysis.
Deep CNN and RNN algorithms will be developed to process the time-series canopy-level RGB, multispectral, thermal, and hyperspectral imagery data to predict peanut, cotton yield, maturity, and quantification of leaf spot disease severity and drought stress. Deep CNNs will be used to extract hierarchical features from the imagery data in a supervised, semi-supervised, or unsupervised manner. The features maps will be concatenated and fed into RNN models for regression of ground truth measurements of yield, disease grading, and physiological parameters (stomatal conductance, photosynthesis, etc.).
Aerial image data collected with UAVs has become central to many plant-breeding research operations. The sheer volume of data collected in these operations lends itself to AI methods for predicting the performance of specific genotypes relative to vastly variable environmental and climatic conditions. AI methods require large volumes of data, but in any model, there is a tradeoff between the accuracy of the data (i.e., signal to noise ratio) and the amount of data required (i.e., number of replications) to determine a trend. Some recent research has focused on how to efficiently improve the accuracy of the input data to the AI models used to predict genotype performance (Han et al., 2018; 2019; 2020). Future research will develop AI models for phenomics that consider the value of improved data accuracy in UAV-based plant phenotyping. An example of appropriate AI analyses is saliency mapping, which tends to indicate key variables and relationships in explainable AI.
Objective 2: Data curation, management, and accessibility, and security, ethics
Obj. 2a. Develop open-source, public agricultural datasets for benchmarking AI algorithms with a focus on explainability
A. Introduction
Unlike traditional techniques that are typically applied to specific cases, artificial intelligence (AI) techniques utilize large quantities of data from various modalities and different types of environments, finding patterns in data and pointing to combinations of farming practices that cannot be easily understood by simple analysis. Large-scale datasets are the cornerstone requirement for any AI-based agricultural applications to be viable. Furthermore, in many fields of precision agriculture, there are already datasets in development that can greatly enhance the AI applications of the future. Those datasets are currently left unused due to the lack of coordination between the various land grant universities.
In Objective 1, the team has identified and has already started working on many AI-based projects, and we anticipate the creation of various information-rich datasets. This Multistate project aims to bring together all these datasets into a common, sharable, and standardized environment.
Such a high-quality, large-scale dataset is of vital importance to the performance of the developed data analysis pipeline and the success of the tasks at hand. Preparation of such dataset though is not trivial because of the efforts and costs required for acquisition, categorization, standardization, and annotation, as well as the encryption, de-identification, and in general, the secure handling of them in a sharable environment.
This data sharing, which is seen to have a vast potential for fostering scientific progress, provides an effective way of addressing the difficulty with data preparation for precision agriculture tasks. Making datasets publicly available will save significant resources associated with data collection and curation and also enable benchmarking and evaluation of machine learning algorithms developed among different research groups. The land grant universities can be the gatekeepers of these datasets, making sure that all researchers involved have access to high quality, multidimensional data from various sources, are aware of the current successes and failures of various AI-based methodologies, and are able to compare their findings with similar results of partnering entities.
B. Detailed activities/procedures
Our first priority will be to launch an inquiry to various land grant universities to identify publicly available databases that they maintain. We will extend that to private companies affiliated with those universities and more. This way, we will compile a list of participating units and their capabilities of sharing or exchanging information. A standardized survey/questionnaire will be sent to all unit heads of extension programs, and the relevant research will be identified.
The group will also identify publicly available datasets and create an easy to search catalog. Our researchers will test the accessibility of USDA-established databases and identify databases that will include free satellite imagery, soil information, as well as weather data, economic data, and more. Multiple modalities will be included in those datasets, and they will be as de-identified as possible. Standardization will be the key element, and for that effort, a thorough literature review will be conducted to identify established practices in the field. We will be building upon standardizations coming from the Precision Agriculture field, but with an emphasis on AI. The questionnaire will also be sent to the various companies working in the field of data management that have expressed an interest to participate like Oracle, Microsoft (Azure), as well as Digital Agriculture companies like Mothive, Ag-Analytics, Agri-Data, and more.
After combining the feedback from the questionnaires above, the group will develop new publicly accessible, large-scale image datasets designated for agricultural vision tasks (e.g., weed detection and control) with image- and pixel-level annotations and will benchmark the state-of-art deep learning algorithms for the datasets. Many small-scale datasets exist at this stage in various universities, so bringing them together will be a challenge but also a straightforward process after a few affiliated universities create the first venues of collaboration. The main source of these datasets will be the initial core researchers in this Multistate proposal, as explained in Objective 1.
The group will create and share de-identified datasets from participating farmers, extension agents, and other partners. This will include obtaining new samples and laboratory data to create datasets. These automated processes are already in place and some of the co-PI’s have already been extending them (LSU’s connection with Ag Analytics is such a platform that is now being explored further).
The group will share benchmark attempts and the corresponding standardized datasets. All de-identified information, including transfer protocols, de-identification protocols, and final results, will be available to participating universities.
Finally, we propose the creation of various educational programs for students and extension agents, but also to interested researchers in the field, whose goal would be to provide a robust understanding of the theoretical underpinnings of the basic AI models, in an Agricultural setting.
Yearly meetings and workshops on applications of AI with an emphasis on hands-on learning, especially for students in agriculture as well as forums and yearly talks about the ethical issues in AI and the effect they may have on various stakeholders, will be established. The program will be connecting our experts to specialists from Computer Science who will then participate in various workshops and presentations about the ethical issues with the use of AI and the better distribution of results.
Obj. 2b. Standardization and testbed development
A. Introduction
Besides the integration and standardization of the resulting datasets proposed in Objective 1, the creation of dedicated testbeds presents an opportunity for a continuous data generating process that will serve as a fixed point in the data creation and accumulation.
A testbed is a platform for conducting rigorous, transparent, and replicable testing of scientific theories, computational tools, and new technologies. We suggest adopting a digital portal or data hub with a user-friendly interface to integrate tools, implement algorithms, and facilitate data sharing and collaboration to process and manage data collected in agriculture research plots or commercial fields.
Currently, perhaps one of the biggest issues slowing the adoption of Artificial Intelligence technology in agricultural applications is the lack of standardized data and integrated software available to the end-user specifically designed to manage big data for agriculture applications purposes. Visualizing, analyzing, interpreting, and communicating/sharing data in a timely manner is critical in agriculture research, but little focus has been placed on this topic so far.
To address this challenge, Texas A&M AgriLife developed a data portal denominated UASHub in the Oracle cloud environment to facilitate data communications. The UASHub integrates electronic field notes, raw and post-processed UAS imagery data for sharing, visualization, analysis, and interpretation. To leverage current efforts, we plan to develop additional tools to enable data management, analysis, and interpretation of large volumes of UAS-derived data. The UASHub includes tools to integrate online data management and access tools to the UASHub such that research scientists can download both raw and processed geospatial data products to their workstations for further analysis. Data analysis tools are implemented to extract various phenotypic features, including canopy height, canopy cover, canopy volume, NDVI, EVI, and canopy surface temperature over a user-specified region on the fly.
B. Detailed activities/procedures
Geospatial data product generation: Images acquired from the UAS platforms is processed using the Structure from Motion (SfM) algorithm to generate geospatial data products such as high-density 3-D point cloud data, orthomosaic images, and digital surface models (DSMs) The orthomosaic images from the multispectral sensor are radiometrically calibrated using a solar spectrometer and radiometric calibration method with known reflectance so that spectral measurements can be compared without bias throughout the growing season. The ground control targets are used in this process to ensure high geo-referencing accuracy of the geospatial data products. Crop height model generation: A DSM (Digital Surface Model), which represents the surface elevation of objects on the ground, is generated from the 3-D point cloud data. To estimate plant height for each flight date, the Crop Height Model (CHM) is then generated by subtracting the DTM (Digital Terrain Model) from the DSM. The DTM will be generated from UAS data acquired before planting.
Grid structure and plot boundary: 1-m² or more for commercial fields. Grids will be used to extract high-density crop data. The grid structure also enables detailed analysis such as the ability to remove from statistical tests grids with no plants, and even its surrounding neighbors that may also be affected due to the lack of plant competition. Likewise, plants on both ends of the plots (i.e., grids 1 and 11, 10 and 20) usually tend to grow and yield more due to lack of plant competition. Those grids could also be removed from statistical analysis if so desired. Plot size and intended use of the data will determine the number and size of grids. From each individual grid, information such as plant height, growth rate, canopy volume, canopy volume progression rate, canopy cover, and canopy cover progression rate may be extracted for analysis.
Crop growth pattern analysis: Time course of crop height measurements within each grid will be extracted from CHM time series layers, and measurements will be fitted to a non-linear sigmoidal model to create a crop growth curve. The first derivative of the growth curve will be calculated as a growth rate curve. The growth rate curve will be used to extract features related to crop growth characteristics, including maximum growth rate, time of maximum growth rate, and duration of half maximum growth rate. These features will be calculated for each grid and summarized by genotype to be used not only to understand the growth characteristics of individual genotypes but also to estimate harvest yield for high yielding genotype selection (i.e., breeding).
Canopy cover progression analysis: A classification algorithm to delineate crop canopy from other non-canopy objects (background) will be developed to calculate canopy cover from the orthomosaic images. The classification algorithm will use four spectral bands (Blue, Green, Red, and Near-Infrared) of orthomosaic images, and various ratios (Red/Green, Blue/Green, 2 x Green – Red – Blue, and NDVI) will be tested to design the best classifier for cotton. The same grid structure designed for the crop growth pattern analysis will be used to calculate canopy cover from the binary classification map. Canopy cover progression will be estimated by fitting a non-linear function to a series of the weekly canopy cover fraction measurements, and the first derivative of the canopy cover progression curve will be computed as a canopy cover expansion rate curve. Features related to the canopy cover expansion pattern will also be extracted, including maximum canopy cover expansion rate, time of the maximum canopy cover expansion rate, and duration of half maximum canopy cover expansion rate. These features will be used to understand phenological characteristics
Crop canopy volume: Crop canopy volume should provide an estimate of plant biomass. Canopy cover for individual grids will be calculated as the sum of pixels classified as canopy times the individual pixel height.
Crop canopy efficiency: Plant canopies function much like a solar panel in that they capture solar radiation and convert it into usable energy. Canopy energy conversion efficiency depends on several factors, including, but not limited to canopy greenness, and stress severity at the canopy level, which may be measured using multispectral and thermal sensors, respectively. Vegetation indices such as normalized difference vegetation index (NDVI) measurement provide useful information on crop growth rates, canopy cover, and ultimately, crop photosynthetic efficiency. Additionally, NDVI is also a good indicator of plant biomass. We are also proposing to use an Excess Greenness Index (ExG) derived from a regular RGB sensor to assess plant canopy efficiency. In combination with other popular vegetation indices such as NDVI and Enhanced Vegetation Index (EVI), canopy efficiency-related phenotypic features including maximum greenness (ExG/EVI/NDVI), the timing of maximum greenness, early/late slopes and their duration will be extracted. Once temporal measurements are plotted over time (NDVI, EVI, ExG, etc.), we find that fitting a model through the data points and then using the model for data interpretation works well since it addresses the normal variability found between sampling dates. Preliminary results collected by our group in several crops (sorghum, cotton, tomato, potato, and corn) seem to indicate that ExG measurements are, for the most part, unaffected by environmental conditions and thus more stable.
Crop maturity monitoring: Some genotypes have markedly distinct maturities, while others may have subtle differences. To rank genotypes regarding differences in maturity, we will use an automated algorithm to classify orthomosaic images. Genotype maturity may be quantified in three different ways: 1) by the number of flowers, 2) by the area covered by flowers, or 3) a combination of number and area covered by flowers. A summary of the classification may be presented ‘per plot’ or ‘per grid’. Preliminary results from tests conducted in cotton showed the great potential of this algorithm to also identify cotton bolls. This algorithm shows a 90% classification accuracy on a per pixel basis. This means that counts should not be affected, although the size and area estimates may affect accuracy.
As many as 60 parameters can be extracted from the above techniques (e.g., growth, canopy efficiency, bloom, and boll counts), which will be correlated to plant growth and lint yield. Features (i.e., data) will be extracted from the images using the methods previously described. Further, environmental conditions constantly change from year to year, which affects genotype responses at the field level (models developed in a particular year may need adjustments to be applicable to others). Equipment (UAS platform, sensors, computers, etc.) failure is also of concern; however, these could possibly be resolved within a relatively short period of time. At this time, the major limitation of the proposed procedures is the computation time required to pre- and post-process all the imagery collected by the UAS platforms. Depending on field size and flight altitude, as many as 500 to 1000 images may be required to produce an appropriate Level 1 data product. Thus, the computation time required for the Level 1 data product generation may be as much as 12–15 hours for each data collection date.
Objective 3: AI adoption (technology transfer) and workforce development
A. Introduction
Extension and outreach activities that present and explain complex topics has historically been approached from a deficit model following the assumption that people would accept and adopt scientific innovations or emerging technologies if they had more information (Nisbet and Scheufele, 2009). However, this model has been found lacking since information alone could not motivate people on adopting a new technology (or scientific innovation); particularly when political or social issues are involved on a specific topic (Knowles, 1984; Kolb et al., 2014). Other models that include active collaboration between scientists and stakeholders can improve technology transfer and adoption. For example, field days that include active engagement of extension personnel and other stakeholders (e.g., growers) have proven to be effective tools in promoting experiential learning (Knowles, 1984; Kolb et al., 2014), which increase interest and willingness in adopting new technology (Rogers, 2003).
In agriculture, extension, outreach, and education components are critically important to develop and implement AI-based tools and technologies. The degree to which a technology is adopted by the end-user is highly dependent on several factors such as the characteristics of producers, size of the farm, availability of resources, age of the farmers, level of knowledge/education, and need for capital investments (National Research Council, 2002; Schimmelpfennig, 2016). Paudel et al. (2021) highlighted the heterogeneity in the adoption of Precision Farming Technologies by farmers and found that the farmers who acquire large farms get higher income from farming, use computers to manage their farms, and who foresee the value of technologies are more likely to adopt new technologies. Thus, to address the heterogeneous producers and farm operations, it is important to perform a need assessment and link major stakeholders and end-users during the technology development processes.
A good understanding of how agriculture data must be collected, processed and how this data can be used to generate solutions based on AI is crucial for efficient technology transfer. For extension and outreach, public and private partnerships, field days, and annual conferences in Digital Agriculture are the main channels to deliver information. A trend is envisioned to have more virtual field days saving farmers time, but not replacing in-person field visits and more hands-on training and case studies type extension meetings. Main efforts in the teaching portion are the development of a robust curriculum in digital agriculture that includes multi-departmental course work and prepare students for the jobs in a digital era that require more skills in computer science and analytics than before. Additionally, it is important to train researchers, supporting staff, and faculties on AI technologies and the ways to obtain quality data to implement AI tools in their research programs.
The major focus areas under this objective are to (i) support AI algorithms development team during the development of user-friendly digital tools/platforms by engaging stakeholders through User-Centered Design (UCD) (Parker, 1999) process, (ii) train consultants, extension specialists, county agents, producers and allied industry on the use of digital and AI-based tools/platforms for precision farm management (technology transfer), (iii) develop next-generation experts on AI and digital agriculture tools development and use. The detailed procedure described below illustrates the method that will follow to achieve this objective. We believe that this model will serve as a foundation for AI technology transfer, adoption, and workforce development. The experience and methods will be transferable and scalable to other states according to their needs and resource availability.
B. Detailed activities/procedures
We propose to develop properly customized education programs for undergraduate/graduate, extension personnel, and other stakeholders. This project is envisioned to be a national resource and a nexus point for innovative education and workforce development programs focused on AI applications for agriculture and natural resources. In addition to leveraging recent programmatic and infrastructural initiatives in AI at our institutions, we will explore new collaborations with colleges serving minority and under-represented minority, and industry partners to train and engage diverse populations, including Ph.D. students, early career professionals, teachers, extension agents, growers, allied industry, and public. For example, in Texas, Institutions such as Texas A&M University-Kingsville, West Texas A&M University, Texas A&M University-Corpus Christi, and Prairie View A&M University train and educate underrepresented and underserved minority citizens. Similar collaborations will be developed between land-grant universities and minority-serving universities in other states too (e.g., Florida). In partnership with these system universities, we propose to develop curriculums (modules) to train graduate and undergraduate students in the use of remote sensing and data processing procedures for precision agriculture with AI technologies. The educational program supports the common goal of preparing the next generation of agricultural extension workers for the demands and expectations that are continually changing as the industry evolves. We will develop three modules on: 1) high-quality data collection, 2) data processing, extraction, and analysis, and 3) artificial intelligence (AI) application in agriculture (Jung et al., 2021), to cover a higher educational pipeline into AI-based digital agriculture. Undergraduates and graduate students will have an opportunity to experience and participate in practical projects in digital agriculture. We believe that this involvement will encourage the students to pursue their careers in the digital agriculture space. This course will be a model course introducing undergraduates and graduate students to the applications of science, technology, and data analytics to develop modern agricultural production systems. This should be offered later in the academic career of the students, and in its initial form, it can be a combined undergraduate-graduate class. This should be a project-based learning system that will help prepare our students for the ever-changing demands of the labor market. We envision covering a wide range of topics in which we will present enough theory and tools so that the students have a working understanding of the area and know the important aspects of it. It will also serve as a starting point in any of these areas and a repository for initial knowledge for those students that want to specialize in them. The course does not aim to replace relevant courses in other departments.
On the outreach and extension side, we plan to train county agents, consultants, producers, and allied industries on the use of AI tools in their farm operations. Training county agents will have a significant impact on technology transfer as they are the primary source of information to assist farmers with several crop production issues related to agronomy, disease, or pests (Hall et al., 2003). For example, Texas A&M AgriLife Extension service currently has an extensive network of county agriculture & natural resources Extension Agents (CEAs) and Integrated Pest Management (IPM) agents throughout the State of Texas in 250 out of 254 counties. Due to their important role in supporting our growers, they have to be updated on new issues and options. Therefore, we propose to develop a network of extension crop specialists from three key locations across the state, in the Texas High Plains (Lubbock-Amarillo-Vernon), Central Texas (Temple, College Station), and south Texas (Warton, Corpus Christi, Weslaco). Extension Specialists from each region will train and involve approximately 75 CEA and IPM agents in the design, evaluation, and dissemination of AI-based tools for crop management developed here. Additional stakeholders will include the agriculture industry, crop consultants, and obviously, our producers.
User-Centered Design is an iterative design process that involves end-users in all phases of product development and addresses their needs (Barnum et al., 2020). In this project, we envision involving major stakeholders throughout all stages of AI tools development, testing, validation, and implementation. An advisory board that includes researchers, crop consultants, producers (large, medium, and small scale), extension agents, industry representatives, and socio-economic scientists will be formed. The major role of the advisory board will be to provide input and evaluate technology development and project progress toward the development of functional, well-designed, user-friendly AI-based digital tools. Inputs from the advisory board members will be vital in making sure the technology and methodologies developed during this project are easily scalable and readily adoptable by producers. Project findings will be disseminated to stakeholders through field days, presentations (at the county and regional levels), and during meetings and commodities conferences (at the national level), where feedback from additional stakeholders is expected. We expect direct collaboration of the advisory board through UCD process and support the project in the following sectors:
- Feasibility and reliability of proposed methodologies to assist crop producers.
- Flexibility of transferring and adapting the AI technology to the production stakeholders.
- Usefulness of digital platform to producers.
Examples of extension and outreach activities/venues include: (i) development of digital communication and education materials; (ii) podcast series to present the developed AI-based technologies, designed to reach a boarder audience; (iii) webinars that will focus on new developments; (iv) in-service training to teach extension agents (in-person and via the eXtension network to train agents across the U.S.); (v) field days; (vi) technology show and expo; (vii) extension publications, infographics, and fact sheets.
The long-term impact of this objective is to develop a program that is a leading resource for AI technology in agriculture and can develop (1) a new generation of trained workforce in the use of AI technology applied to agriculture and natural resources, (2) stakeholders who can utilize and adopt AI technology, and (3) consumers who understand the role of AI in agriculture and natural resources.
Measurement of Progress and Results
Outputs
- Obj. 1a: Major findings will be published, which validates the importance of AI and specific methodology for particular agriculture applications. Trained deep learning algorithms will be available for estimating crop yield, inputs and monitoring crop status in the field and postharvest quality. High resolution remote sensing database will be created. Standardized big-data and data management system will be available to facilitate communication among scientist in conducting rigorous, transparent, and replicable testing of scientific theories, computational tools, and new technologies. Algorithms will be developed to automate the workflow of multi-scale remote sensing data analysis, information extraction, information scaling and synthesis for generating plot or field crop characteristics maps.
- Obj. 1b: New AI methods will be developed for: (1) advanced perception, localization, and manipulation for robotic production and harvesting tasks, (2) fruit detection and dynamic mapping during harvest, (3) harvest planning and obstacle avoidance approaches, and (4) edge computing approaches for perception, localization and planning of fruit harvest.
- Obj. 1c: Soil samples will be obtained from southern states of the US along with spectra, and laboratory measured properties. Trained deep learning models will be developed: (1) to predict soil carbon, hydrological properties, and health parameters using spectra (visible and near infrared, mid infrared), and (2) to predict water quality parameters and HAB events from UAV and satellite imagery data in the Southeast.
- Obj. 1d: This objective will produce economic, environmental, and social models assessing the impacts of AI technology on producers, consumers, and industries. This is expected to include surveys and other instruments that directly measure how AI has affected communities.
- Obj. 1e: A comprehensive dataset will be available including multi-scale, multi-modal imagery, and manual measurements of plant architecture, yield, yield-related traits, and disease rating at multiple time points and environments. A set of AI-based image analysis tools will be developed for predicting crop yield, drought response and disease. A prototype platform will be generated to use AI tools and sensor and image processing for variety selection and high throughput phenotyping. Data analysis and visualization support system will be available to assist plant breeders selecting elite genotypes based on growth parameters and yield potential.
- Obj. 2a: A shareable database schema with specific access capabilities will be created as well as a methodology to connect to it safely with both adding and downloading capabilities. Recurring workshops discussing the theoretical underpinnings of AI models in agriculture as well as the ethical use of AI and various security issues.
- Obj. 2b: The primary end product from this objective will be a database management system: (1) a UAS-based platform to collect detailed and high quality HTP data for research plots or commercial fields, (2) automated procedures for data processing, analysis, and growth parameter extraction, to analyze, visualize and interpret collected data, and (3) web-based algorithms for data management and communication for all project scientists.
- Obj. 3: Recurring workshops, technology expo, seminars, webinar, podcast series, field days, and in-service training will be established that target primarily extension agents, farmers and allied industry focusing on the uses of AI in agriculture. Extension publications, infographics, and fact sheets will be developed to present advancements on AI-based technologies. Classes with a focus on AI applications in agriculture and natural resources will be added and evaluated on a yearly basis. Certifications and Specialization programs will be added to already established Minors in relative fields.
Outcomes or Projected Impacts
- Obj. 1a: The outcome includes a better preparation for harvest and storage with improved prediction of yield, timely intervention of control measures to address pest invasion and reduced loss associated with low quality produce entrainment in the supply chain. Farmers and stakeholders can be benefited from more sophisticated algorithms for yield prediction and variable rate inputs on their operations. The outcomes of animal health and welfare assessment may include automated tools that can assist farmers in achieving more efficient farm management and improving animal welfare, farm productivity, and profit.
- Obj. 1b: Automated fruit detection and harvesting systems using AI to improve performance and efficiency will enhance site-specific crop management practices to increase yield, reduce cost, and improve grower profit. Implementation of AI-based edge processing will improve execution speed and perception effectiveness in lower cost embedded vision controllers.
- Obj. 1c: The calibrated model can serve different stakeholders including farmers and USDA-NRCS to rapidly derive soil properties. This will introduce new technological improvements for the development of new in situ sensors for rapid estimation of soil properties. The deep learning models can serve as a decision support system for water managers and policy makers to make early interventions and reduce economic and environmental losses in natural water bodies.
- Obj. 1d: This research will provide future scenarios projecting economic and social impacts from the adoption and use of AI technology. It is expected that results will be disseminated to producers, policy makers, and stakeholders and will be used as key input in decision-making. This will provide more informed and improved choices resulting in more streamlined and socially optimal agricultural practices and policy outcomes.
- Obj. 1e: We will obtain new knowledge on how state-of-the-art deep learning models trained on massive color images can be efficiently applied to solve crop phenotyping problems with limited data and diverse sensor modalities. We will facilitate HTP through transdisciplinary collaboration between scientists with various expertise from multiple institutions to assist breeders and agriculture scientists with selecting elite genotypes or the interpretation of experimental treatments.
- Obj. 2a: AI based algorithms depend heavily on the existence of large, clean and information rich databases. By combining multiple datasets, the users will see a dramatic increase in their algorithms’ predictive percentages, and pattern recognition ability.
- Obj. 2b: This objective will enable transdisciplinary scientists to communicate and exchange information and accelerate the development of agricultural applications for crop management. The data portal has the potential to deliver tools and methodologies for UAS-based HTP, enabling the development of AI-based cognitive tools. Data gathered using the proposed framework would provide users a high level of both spatial and temporal details of crops at a scale.
- Obj. 3: An outcome of this Objective is to increase acceptability, awareness,and trust of AI by stakeholders in the agriculture industry. We envision this project to become a nexus point for education and training, building capacity for the U.S. workforce to meet the demands of AI within the food system. Activities of this Objective aim to increase knowledge of available careers in AI for underrepresented groups including minorities, women, rural residents, and other disadvantaged groups. Every year, we plan to educate more than 1,000 students, faculty, and other stakeholders on AI developments for precision agriculture applications via our education, extension, and outreach activities.
Milestones
(2022):Obj. 1b: In year 1, multiple multistate research teams will be formed to focus on advanced perception, localization, and manipulation for robotic production and harvesting tasks. Obj. 1c: A water quality and aerial imagery dataset will be assembled in Year 1. Obj. 1e: Milestones for year 1 include selection of proximal sensors and process standardization; partnership with other Universities to replicate trials as possible; a common database for algorithm training; test plots for data collection at various locations, algorithms and platforms for data upload, analysis and visualization; algorithms to extract plant parameters and analyze genotypes performance; and training technical support personnel in remote sensing data collection. Obj. 2b: During year 1, we will select and develop testbed fields at various locations and also a cloud based “Data Portal” and software to upload, analyze and visualize remote sensing data. Various educational workshops will be organized to train users of the portal and get feedback. Obj. 3: In year 1, we will be testing the new proposed classes and establishing certifications. We will develop extension and outreach programs. A collaboration among extension programs from every location/state will be established.(2023):Obj. 1b: In year 2-3, there will be a minimum of one funded project for collaborative research. In year 3-4, there will be at least two submissions of multi-university research proposals. Obj. 1d: The economic team, in coordination with other team members, aspires to develop a comprehensive set of socio-economic and environmental models by early 2023. Obj. 1e: In year 2, algorithms will be trained with database collected. Workshops will be conducted to discuss year 1 data collection and refine objectives for year 2. The algorithms developed in year 1 will be validated and tested for generalization across locations and years. Obj. 2a: Within the first two years of the project, a common repository will be formed and the necessary transfer protocols, safety protocols and access protocols will be established. Obj. 2b: In year 2, we will continue collecting data, expand testbed locations, add more users, and improve the analytical and visualization algorithms. We will continue and expand the educational workshops on uses of the system and receive feedback from participants. Obj. 3: In years 2, an online multi-institutional program will be developed to train consultants and service providers on how to include AI tools in their portfolio.
(2024):Obj. 1c: DL models will be developed by Year 3. Obj. 1e: In years 3-5, all items listed in Year 2 will be refined and improved. Obj. 2b: In years 3-5, we will expand and refine the activities listed in year 2, and add many surveys to determine the use of the resulting portal and extend its capabilities. Obj. 3: In years 3, an online multi-institutional program will be developed to train consultants and service providers on how to include AI tools in their portfolio.
(2025):Obj. 1e: In years 3-5, all items listed in Year 2 will be refined and improved. Obj. 2a: Connection with existing databases will be established on the 4th year mark Obj. 2b: In years 3-5, we will expand and refine the activities listed in year 2, and add many surveys to determine the use of the resulting portal and extend its capabilities. Obj. 3: Within years 2-5, programs in applications of AI in agriculture will be established and compared. Every year, extension and outreach events (e.g., webinars, seminars, field days, in-service training, extension publications, etc.) will focus on new project developments and serve as a combined public information sharing and training and education opportunity with anticipated attendance at 1,000 people annually.
(2026):Obj. 1a: At the end of the five year project, milestones include development of AI models and tools with high accuracy in predicting yield and detecting quality of in-field and postharvest agricultural products. An organized database linked with proper procedures for data acquisition is needed before the exploration using AI approaches. For animal production, milestones include (1) development of computer vision systems to estimate cattle bodyweight in real-time and to detect broiler behaviors, and (2) commercialization and deployment of the computer vision systems in cattle and broiler farms. Obj. 1b: At the end of the project, AI-based edge processing approaches will be implemented for various robotic tasks improving precision, throughput, and economics. Publications in refereed journals and opportunity for future collaborations and multistate funded projects will be achieved. Obj. 1c: At the end of 5 years, a soil spectral library including acquired spectra and laboratory measured properties for Mississippi State is expected to be developed. An algorithm to enable application of models calibrated on laboratory collected spectra on field soil spectra is expected to be developed. A decision support system will be developed by year 5. Obj. 1d: This research will include the development of survey instruments to assess the adoption potential of key AI technologies being developed buy other team members, including robotics, UAV, and machine learning technology. Obj. 2a: The dissemination of information will begin in earnest in year five. Obj. 2b: In years 3-5, we will expand and refine the activities listed in year 2, and add many surveys to determine the use of the resulting portal and extend its capabilities.
Projected Participation
View Appendix E: ParticipationOutreach Plan
On the outreach and extension side, we plan to train county agents, consultants, producers, and allied industries on the use of AI tools in their farm operations. Training county agents will have a significant impact on technology transfer as they are the primary source of information to assist farmers for several crop production issues related to agronomy, disease, or pests (Hall et al., 2003). For example, Texas A&M AgriLife Extension service currently has an extensive network of county agriculture & natural resources Extension Agents (CEAs) and Integrated Pest Management (IPM) agents throughout the State of Texas in 250 out of 254 counties. Due to their important role in supporting our growers, they have to be updated on new issues and options. Therefore, we propose to develop a network of extension crop specialists from three key locations across the state, in the Texas High Plains (Lubbock-Amarillo-Vernon), Central Texas (Temple, College Station), and south Texas (Warton, Corpus Christi, Weslaco). Extension Specialists from each region will train and involve approximately 75 CEA and IPM agents in the design, evaluation, and dissemination of AI-based tools for crop management developed here. Additional stakeholders will include the agriculture industry, crop consultants, and obviously, our producers.
Examples of extension and outreach activities/venues include: (i) development of digital communication and education materials; (ii) podcast series to present the developed AI-based technologies, designed to reach a boarder audience; (iii) webinars that will focus on new developments; (iv) in-service training to teach extension agents (in-person and via the eXtension network to train agents across the U.S.); (v) field days; (vi) technology show and expo; (vii) extension publications, infographics, and fact sheets.
Organization/Governance
In this multistate research project, there will be three officer positions, i.e., a Chair, a Vice Chair, and a Secretary. These three officers will make up the project Executive Committee. The Executive Committee will oversee activities of the project, help coordinate among participants, and facilitate annual meetings.
All officers are to be elected for a two-year term to provide continuity. At the initiation of the project, the officers will be elected. Their terms will end at the end of the second annual meeting. At the end of the term, the Vice Chair will become Chair, the Secretary will become Vice Chair, and a new Secretary will be elected.
Administrative guidance will be provided by an assigned Administrative Advisor and a NIFA Representative.
Literature Cited
Abcouwer, N., Daftry, S., Venkatraman, S., del Sesto, T., Toupet, O., Lanka, R., … Ono, M. (2020). Machine Learning Based Path Planning for Improved Rover Navigation (Pre-Print Version). Retrieved from http://arxiv.org/abs/2011.06022
Abdulridha, J.; Ehsani, R.; Abd-Elrahman, A.; Ampatzidis, Y. A Remote Sensing technique for detecting laurel wilt disease in avocado in presence of other biotic and abiotic stresses. Computers and Electronics in Agriculture 2019, 156, 549-557.
Abdullahi, H.S.; Mahieddine, F.; Sheriff, R.E. Technology impact on agricultural productivity: A review of precision agriculture using unmanned aerial vehicles. International Conference on Wireless and Satellite Systems 2015, 388-400.
Adedeji, A. A., Ekramirad, N., Rady, A., Hamidisepehr, A., Donohue, K., Villanueva, R., Parrish, C.A., and Li, M. (2020). Non-destructive technologies for detecting insect infestation in fruits and vegetables under postharvest conditions: A critical review. Foods 9(7), 927.
Ahmed, N., E. Sample, and M. Campbell. (2013) Bayesian multi-categorical soft data fusion for human-robot collaboration. Robotics, IEEE Transactions,29(1):189-206.
Albrecht, U.; Fiehn, O.; Bowman, K.D. Metabolic variations in different citrus rootstock cultivars associated with different responses to Huanglongbing. Plant Physiology and Biochemistry 2016, 107, 33-44.
Aleza, P.; Juarez, J.; Hernandez, M.; Ollitrault, P.; Navarro, L. Implementation of extensive citrus triploid breeding programs based on 4x x 2x sexual hybridisations. Tree Genet Genomes 2012, 8, 1293–1306.
Al Khaled, Y.A., Parrish, C. and Adedeji, A.A. (2021). Emerging non-destructive approaches for meat quality and safety evaluation. Comprehensive Reviews in Food Science and Food Safety. In press.
Ampatzidis Y., and Partel V., 2019. UAV-based high throughput phenotyping in citrus utilizing multispectral imaging and artificial intelligence. Remote Sensing, 11(4), 410; doi: 10.3390/rs11040410.
Ampatzidis Y., Partel V., Meyering B., and Albrecht U., 2019. Citrus rootstock evaluation utilizing UAV-based remote sensing and artificial intelligence. Computers and Electronics in Agriculture, 164, 104900, doi.org/10.1016/j.compag.2019.104900.
Andersen MA, Alston JM, Pardey PG, Smith A: A century of U.S. productivity growth: A surge then a slowdown. Am J Agr Econ 2018, 93:1257-1277.
Ashapure A, Jung J, Yeom J, Chang A, Maeda M, Maeda A, Landivar J: 2019. A novel framework to detect conventional tillage and no-tillage cropping system effect on cotton growth and development using multi-temporal UAS data. ISPRS-J Photogramm Remote Sens, 152:49-64.
Athey, S. and Wager, S., 2019. Estimating treatment effects with causal forests: An application. Observational Studies, 5(2), pp.37-51.
Bac, C., E. Henten, J. Hemming, and Y. Edan. (2014) Harvesting robots for high-value crops: State-of- the-art review and challenges ahead. Journal of Field Robotics, 31(6):888–911.
Bargoti, S., & Underwood, J. (2016). Deep fruit detection in orchards. ArXiv Preprint ArXiv:1610.03677. https://doi.org/10.1109/ICRA.2017.7989417
Barnum, C. M. (2020). Usability testing essentials: ready, set... test!. Morgan Kaufmann.
Barré, P., Stöver, B. C., Müller, K. F., & Steinhage, V. (2017). LeafNet: A computer vision system for automatic plant species identification. Ecological Informatics, 40, 50-56.
Baweja, H. S., Parhar, T., Mirbod, O., & Nuske, S. (2018). Stalknet: A deep learning pipeline for high-throughput measurement of plant stalk count and stalk width. In Field and service robotics (pp. 271-284). Springer, Cham.
Bell, M. J., & Tzimiropoulos, G. (2018). Novel Monitoring Systems to Obtain Dairy Cattle Phenotypes Associated With Sustainable Production. Frontiers in Sustainable Food Systems, 2, 31. https://doi.org/10.3389/fsufs.2018.00031
Blagojević, M., Blagojević, M., & Ličina, V. (2016). Web-based intelligent system for predicting apricot yields using artificial neural networks. Scientia Horticulturae, 213, 125-131. doi:https://doi.org/10.1016/j.scienta.2016.10.032
Blasco, J., Munera, S., Aleixos, N., Cubero, S. and Molto, E. 2017. Machine vision-based measurement systems for fruit and vegetable quality control in postharvest. In: Hitzmann B. (eds), Measurement, Modeling and Automation in Advanced Food Processing. Advances in Biochemical Engineering/Biotechnology, vol 161. Springer, Cham, pp 71-91. https://doi.org/10.1007/10_2016_51
Bolton, D. K., & Friedl, M. A. 2013. Forecasting crop yield using remotely sensed vegetation indices and crop phenology metrics. Agricultural and Forest Meteorology, 173, 74-84.
Braddock, T., Roth, S., Bulanon, J. I., Allen, B., & Bulanon, D. M. (2019). Fruit Yield Prediction Using Artificial Intelligence. Paper presented at the 2019 ASABE Annual International Meeting, St. Joseph, MI. https://elibrary.asabe.org/abstract.asp?aid=50793&t=5
Bulanon, D., H. Okamoto, and S. Hata. (2005) Feedback control of manipulator using machine vision for robotic apple harvesting. In 2005 ASAE Annual Meeting, page 1. American Society of Agricultural and Biological Engineers.
Bui, E., Henderson, B., & Viergever, K. (2009). Using knowledge discovery with data mining from the Australian Soil Resource Information System database to inform soil carbon mapping in Australia. Global Biogeochemical Cycles, 23(4). https://doi.org/https://doi.org/10.1029/2009GB003506
Busch, Lawrence. "Big data, big questions| A dozen ways to get lost in translation: Inherent challenges in large scale data sets." International Journal of Communication 8 (2014): 18.
Calabi-Floody M, Medina J, Rumpel C, Condron LM, Hernandez M, Dumont M, Luz Mora MDL. 2018. Smart fertilizers as a strategy for sustainable agriculture. Advances in Agronomy 147:119-157.
Chen, Y., Lee, W. S., Gan, H., Peres, N., Fraisse, C., Zhang, Y., & He, Y. (2019a). Strawberry Yield Prediction Based on a Deep Neural Network Using High-Resolution Aerial Orthoimages. Remote Sensing, 11(13), 1584. Retrieved from https://www.mdpi.com/2072-4292/11/13/1584
Chen, W., T. Xu, J. Liu, M. Wang, and D. Zhao. (2019b) Picking robot visual servo control based on modified fuzzy neural network sliding mode algorithms. Electronics, 8(6):605.
Cheng, H., Damerow, L., Sun, Y., & Blanke, M. (2017). Early Yield Prediction Using Image Analysis of Apple Fruit and Tree Canopy Features with Neural Networks. Journal of Imaging, 3(1), 6. Retrieved from https://www.mdpi.com/2313-433X/3/1/6
Chlingaryan, A., Sukkarieh, S., & Whelan, B. (2018). Machine learning approaches for crop yield prediction and nitrogen status estimation in precision agriculture: A review. Computers and Electronics in Agriculture, 151, 61-69. doi:https://doi.org/10.1016/j.compag.2018.05.012
Coeckelbergh, Mark. AI ethics. MIT Press, 2020.
Costa L., McBreen J., Ampatzidis Y., Guo J., Reisi G.M., Babar A., 2021. Predicting grain yield and related traits in wheat under heat-related stress environments using UAV-based hyperspectral imaging and fuctional regression. Precision Agriculture.
Crane-Droesch, A. 2018. Machine learning methods for crop yield prediction and climate change impact assessment in agriculture. Environmental Research Letters, 13(11), 114003.
Cruz, A.C.; Luvisi, A.; De Bellis, L.; Ampatzidis, Y. X-FIDO: An Effective Application for Detecting Olive Quick Decline Syndrome with Novel Deep Learning Methods. Frontiers, Plant Sci. 2017, 8, 1741.
Cruz, A.; Ampatzidis, Y.; Pierro, R.; Materazzi, A.; Panattoni, A.; De Bellis, L.; Luvisi, A. Detection of Grapevine Yellows Symptoms in Vitis vinifera L. with Artificial Intelligence. Computers and Electronics in Agriculture 2019, 157, 63-76.
Cuenca, J.; Aleza, P.; Vicent, A.; Brunel, D.; Ollitrault, P.; Navarro, L. Genetically based location from triploid populations and gene ontology of a 3.3-Mb genome region linked to Alternaria brown spot resistance in citrus reveal clusters of resistance genes. PLoS ONE 2013, 8(10), e767553.
Daniya, T., & Vigneshwari, S. (2019). A review on machine learning techniques for rice plant disease detection in agricultural research. International Journal of Advanced Science and Technology, 28(13), 49–62.
de Alencar Nääs, I., da Silva Lima, N. D., Gonçalves, R. F., de Lima, L. A., Ungaro, H., & Abe, J. M. (2020). Lameness prediction in broiler chicken using a machine learning technique. Information Processing in Agriculture. https://doi.org/10.1016/j.inpa.2020.10.003
Dhillon, A., & Verma, G. K. (2020). Convolutional neural network: a review of models, methodologies and applications to object detection. Progress in Artificial Intelligence, 9(2), 85–112. https://doi.org/10.1007/s13748-019-00203-0.
Drummond, T., S., A. Sudduth, K., Joshi, A., J. Birrell, S., & R. Kitchen, N. (2003). Statistical and neural methods for site–specific yield prediction. Transactions of the ASAE, 46(1), 5. doi:https://doi.org/10.13031/2013.12541.
Duckett, T., Pearson, S., Blackmore, S., Grieve, B., Chen, W.-H., Cielniak, G., … Yang, G.-Z. (2018). Agricultural Robotics: The Future of Robotic Agriculture. Retrieved from http://arxiv.org/abs/1806.06762
Dyrmann, M., Karstoft, H., & Midtiby, H. S. (2016). Plant species classification using deep convolutional neural network. Biosystems Engineering, 151, 72-80.
Edan, Y., D. Rogozin, T. Flash, and G. Miles. (2000) Robotic melon harvesting. IEEE Transactions on Robotics and Automation, 16(6):831–835.
Esposito, M., Crimaldi, M., Cirillo, V., Sarghini, F., & Maggio, A. (2021). Drone and sensor technology for sustainable weed management: a review. Chemical and Biological Technologies in Agriculture, 8(1), 1–11. https://doi.org/10.1186/s40538-021-00217-8
Eli-Chukwu, Ngozi Clara. "Applications of artificial intelligence in agriculture: A review." Engineering, Technology & Applied Science Research 9.4 (2019): 4377-4383.
European Parliament, 2014. Precision Agriculture: An Opportunity for EU Farmers – Potential support with the CAP 2014-2020.
FAO. (2018). Shaping the future of livestock sustainably, responsibly, efficiently. 10th Global Forum for Food and Agriculture, (January), 20. Retrieved from http://www.fao.org/3/i8384en/I8384EN.pdf
Faulkner A, Cebul K. 2014. Agriculture gets smart: The rise of data and robotics. Cleantech Agriculture Report. Cleantech Group.
Finger R., S.M. Swinton, N. El Benni, and A. Walter, 2019. “Precision Farming at the Nexus of Agricultural Production and the Environment.” Annual Review of Resource Economics 11:313-335.
Fuentes, S.; Gonzalez Viejo, C.; Cullen, B.; Tongson, E.; Chauhan, S.S.; Dunshea, F.R. Artificial Intelligence Applied to a Robotic Dairy Farm to Model Milk Productivity and Quality based on Cow Data and Daily Environmental Parameters. Sensors 2020, 20, 2975. https://doi.org/10.3390/s20102975.
Gan, H., Lee, W.S., Alchanatis, V., & El-Rahman, A. (2020). Active thermal imaging for immature citrus fruit detection. Biosystems Engineering, 198(2020), 291-303. https://doi.org/10.1016/j.biosystemseng.2020.08.015.
Gandhi, N., Petkar, O., & Armstrong, L. J. (2016, 15-16 July 2016). Rice crop yield prediction using artificial neural networks. Paper presented at the 2016 IEEE Technological Innovations in ICT for Agriculture and Rural Development (TIAR).
Ganesh, P., Volle, K., Burks, T. F., & Mehta, S. S. (2019). Deep Orange: Mask R-CNN based Orange Detection and Segmentation. IFAC-PapersOnLine, 52(30), 70–75. https://doi.org/10.1016/j.ifacol.2019.12.499.
Gholipoor, M., & Nadali, F. (2019). Fruit yield prediction of pepper using artificial neural network. Scientia Horticulturae, 250, 249-253. doi:https://doi.org/10.1016/j.scienta.2019.02.040
Girshick, R. (2015). Fast R-CNN. Proceedings of the IEEE International Conference on Computer Vision, 2015 Inter, 1440–1448. https://doi.org/10.1109/ICCV.2015.169
Girshick, R., Donahue, J., Darrell, T., & Malik, J. (2014). Rich feature hierarchies for accurate object detection and semantic segmentation. Proceedings of the IEEE Computer Society Conference on Computer Vision and Pattern Recognition, 580–587. https://doi.org/10.1109/CVPR.2014.81
Ghosal, S., Blystone, D., Singh, A. K., Ganapathysubramanian, B., Singh, A., & Sarkar, S. (2018). An explainable deep machine vision framework for plant stress phenotyping. Proceedings of the National Academy of Sciences, 115(18), 4613-4618.
Goodfellow, I., Bengio, Y., & Courville, A. (2016). Deep Learning. MIT Press. https://doi.org/10.4258/hir.2016.22.4.351.
Griffin, T.W. and J. Lowenberg-DeBoer. 2005. Worldwide Adoption and Profitability of Precision Agriculture: Implications for Brazil. Revista de Politica Agricola, XIV (4):21-37
Grinblat, G. L., Uzal, L. C., Larese, M. G., & Granitto, P. M. (2016). Deep learning for plant identification using vein morphological patterns. Computers and Electronics in Agriculture, 127, 418–424. https://doi.org/10.1016/j.compag.2016.07.003.
Guo, Y., Zhu, W., Ma, C., & Chen, C. (2016). Top-view recognition of individual group-housed pig based on Isomap and SVM. Transactions of the Chinese Society of Agricultural Engineering, 32(3), 182-187.
Hall, L., Dunkelberger, J., Ferreira, W., Prevatt, J., & Martin, N. R. (2003). Diffusion-adoption of personal computers and the Internet in farm business decisions: Southeastern beef and peanut farmers. Journal of Extension, 41(3), 1-11.
Han, Z., & Gao, J. (2019). Pixel-level aflatoxin detecting based on deep learning and hyperspectral imaging. Computers and Electronics in Agriculture, 164, 104888.
Harrell, R., P. Adsit, and D. Slaughter. (1985) Real-time vision-servoing of a robotic tree fruit harvester. ASAE paper, pages 85–3550.
Harrell, R., D. Slaughter, and P. Adsit. (1989) A fruit- tracking system for robotic harvesting. Machine Vision and Applications, 2(2):69–80.
Harrell R., ,P. Adsit, T. Pool, and R.Hoffman. (1990) The Florida robotic grove-lab. Transactions of the ASAE, 33 (2):391–399.
Hatfield J, Takle G, Grotjahn R, Holden P, Izaurralde RC, Mader T, Marshall E, Liverman D: Ch.6: Agriculture. Climate change in the United States: The Third National Climate Assessment. Edited by Melillo JM, Richmond T, Yohe GW. U.S. Global Change Research Program; 2014:50-174.
He, K., Gkioxari, G., Dollár, P., & Girshick, R. (2017). Mask R-CNN. In In Proceedings of the IEEE international conference on computer vision (pp. 2961–2969). https://doi.org/10.1109/TPAMI.2018.2844175
He, K., Zhang, X., Ren, S., & Sun, J. (2016). Deep Residual Learning for Image Recognition. Proceedings of the IEEE Conference on Computer Vision and Pattern Recognition, 770–778. https://doi.org/10.1002/chin.200650130.
Hill, P. R., Kumar, A., Temimi, M., & Bull, D. R. (2020). HABNet: Machine Learning, Remote Sensing-Based Detection of Harmful Algal Blooms. IEEE Journal of Selected Topics in Applied Earth Observations and Remote Sensing, 13, 3229-3239.
Howard, A. G., Zhu, M., Chen, B., Kalenichenko, D., Wang, W., Weyand, T., … Adam, H. (2017). MobileNets: Efficient Convolutional Neural Networks for Mobile Vision Applications. ArXiv Preprint ArXiv:1704.04861 (2017).
Huang, G., Liu, Z., Van Der Maaten, L., & Weinberger, K. Q. (2017). Densely connected convolutional networks. In Proceedings of the IEEE conference on computer vision and pattern recognition (pp. 4700–4708).
Ji, J., Zhu, X., Ma, H., Wang, H., Jin, X., & Zhao, K. (2021). Apple Fruit Recognition Based on a Deep Learning Algorithm Using an Improved Lightweight Network. Applied Engineering in Agriculture, 37(1), 123-134. doi:https://doi.org/10.13031/aea.14041
Jiang, Y., Li, C., Xu, R., Sun, S., Robertson, J. S., & Paterson, A. H. (2020). DeepFlower: a deep learning-based approach to characterize flowering patterns of cotton plants in the field. Plant methods, 16(1), 1-17.
Jin, S., Su, Y., Gao, S., Wu, F., Hu, T., Liu, J., ... & Guo, Q. (2018). Deep learning: individual maize segmentation from terrestrial lidar data using faster R-CNN and regional growth algorithms. Frontiers in plant science, 9, 866.
Jin, S., Su, Y., Gao, S., Wu, F., Ma, Q., Xu, K., ... & Guo, Q. (2019). Separating the structural components of maize for field phenotyping using terrestrial lidar data and deep convolutional neural networks. IEEE Transactions on Geoscience and Remote Sensing, 58(4), 2644-2658.
Ji, W., Li, S., Chen, S., Shi, Z., Viscarra Rossel, R. A., & Mouazen, A. M. (2016). Prediction of soil attributes using the Chinese soil spectral library and standardized spectra recorded at field conditions. Soil and Tillage Research, 155, 492–500. https://doi.org/http://dx.doi.org/10.1016/j.still.2015.06.004
Johnson, D. M. (2014). An assessment of pre- and within-season remotely sensed variables for forecasting corn and soybean yields in the United States. Remote Sensing of Environment, 141, 116-128. doi:https://doi.org/10.1016/j.rse.2013.10.027
Jones, Meg Leta. "Silencing bad bots: Global, legal and political questions for mean machine communication." Communication Law and Policy 23.2 (2018): 159-195.
Jung, J., M. Maeda, A. Chang, M. Bhandari, A. Ashapure, J. Landivar, "The potential of remote sensing and artificial intelligence as tools to improve the resilience of agriculture production systems", Current Opinion in Biotechnology, vol. 70, pp. 15-22, 2021.
Kamilaris, A., Kartakoullis, A., & Prenafeta-Boldú, F. X. (2017). A review on the practice of big data analysis in agriculture. Computers and Electronics in Agriculture, 143(January), 23–37. https://doi.org/10.1016/j.compag.2017.09.037
Kamilaris, A., & Prenafeta-Boldu, F. X. (2018). Deep learning in agriculture: A survey. Computers and Electronics in Agriculture, 147, 70–90.
Kaul, M.; Hill, R. L.; Walthall, C. 2005. Artificial neural networks for corn and soybean yield prediction. Agricultural Systems, 85(1), 1-18.
Keskin, H., Grunwald, S., & Harris, W. G. (2019). Digital mapping of soil carbon fractions with machine learning. Geoderma, 339, 40–58. https://doi.org/https://doi.org/10.1016/j.geoderma.2018.12.037
Khanna M, Gramig BM, DeLucia EH, Cai X, Kumar P. 2019. Harnessing emerging technologies to reduce Gulf hypoxia. Nature Sustainability 2:889-891.
Knowles, M. (1984). The Adult Learner: A Neglected Species (3rd Ed.). Houston, TX: Gulf Publishing.
Koenig, N. and M. Matari. (2017) Robot life-long task learning from human demonstrations: a bayesian approach. Autonomous Robots, 41(5):1173-1188.
Kolb, D. A. (2014). Experiential learning: Experience as the source of learning and development. Upper Saddle River, NJ: Pearson Education.
Krizhevsky, A., Sutskever, I., & Hinton, G. E. (2012). ImageNet Classification with Deep Convolutional Neural Networks. Advances In Neural Information Processing Systems, 1–9. https://doi.org/http://dx.doi.org/10.1016/j.protcy.2014.09.007.
Kruseman, Gideon. "Workshop on data standardization and minimum data sets: setting the scene." (2018).
Kunisch, M. "Big Data in Agriculture—Perspectives for a Service Organization." Landtechnik 71.1 (2016): 1-3.
Lamichhane, S., Kumar, L., & Wilson, B. (2019). Digital soil mapping algorithms and covariates for soil organic carbon mapping and their implications: A review. Geoderma, 352(January), 395–413. https://doi.org/10.1016/j.geoderma.2019.05.031
Lawrence, S., Giles, C. L., Tsoi, A. C., & Back, A. D. (1997). Face recognition: A convolutional neural-network approach. IEEE Transactions on Neural Networks, 8(1), 98–113. https://doi.org/10.1109/72.554195.
LeCun, Y., Bottou, L., Bengio, Y., & Haffner, P. (1998). Gradient-based learning applied to document recognition. Proceedings of the IEEE, 86(11), 2278–2323. https://doi.org/10.1109/5.726791.
LeCun, Y.; Bengio, Y.; Hinton, G. Deep learning. Nature 2015, 521(7553), 436.
Li, Y., & Ren, F. (2019). Light-Weight RetinaNet for Object Detection. Retrieved from http://arxiv.org/abs/1905.10011.
Liakos, K. G., Busato, P., Moshou, D., Pearson, S., & Bochtis, D. (2018). Machine Learning in Agriculture: A Review. Sensors, 18(8), 2674. Retrieved from https://www.mdpi.com/1424-8220/18/8/2674
Linardatos P, Papastefanopoulos V, Kotsiantis S. (2020). Explainable AI: A Review of Machine Learning Interpretability Methods. Entropy (Basel). 2020;23(1):18. Published 2020 Dec 25. doi:10.3390/e23010018
Lin, Z., & Guo, W. (2020). Sorghum Panicle Detection and Counting Using Unmanned Aerial System Images and Deep Learning. Frontiers in Plant Science, 11, 1346.
Lin, Z., & Guo, W. (2020). Sorghum Panicle Detection and Counting Using Unmanned Aerial System Images and Deep Learning. Frontiers in Plant Science, 11, 1346.
Liu, B., & Bruch, R. (2020). Weed Detection for Selective Spraying: a Review. Current Robotics Reports, 1(1), 19–26. https://doi.org/10.1007/s43154-020-00001-w
Liu, Simon Y. "Artificial Intelligence (AI) in agriculture." IT Professional 22.3 (2020): 14-15.
Liu, J., E. Goering, C., & Tian, L. (2001). A NEURAL NETWORK FOR SETTING TARGET CORN YIELDS. Transactions of the ASAE, 44(3), 705. doi:https://doi.org/10.13031/2013.6097
Liu, W., Anguelov, D., Erhan, D., Szegedy, C., Reed, S., Fu, C. Y., & Berg, A. C. (2016). SSD: Single shot multibox detector. Lecture Notes in Computer Science (Including Subseries Lecture Notes in Artificial Intelligence and Lecture Notes in Bioinformatics), 9905 LNCS, 21–37. https://doi.org/10.1007/978-3-319-46448-0_2.
Lobell, D.B. & Burke, M. B. 2010. On the use of statistical models to predict crop yield responses to climate change. Agricultural and forest meteorology, 150(11), 1443-1452.
Lowenberg-deBoer J, Erickson B. 2019. Setting the record straight on precision technology adoption. Agronomy Journal 111(4):1-18.
Lu, H., Cao, Z., Xiao, Y., Zhuang, B., & Shen, C. (2017). TasselNet: counting maize tassels in the wild via local counts regression network. Plant methods, 13(1), 1-17.
Lu, Y., Young, S. (2020). A survey of public datasets for computer vision tasks in precision agriculture. Computers and Electronics in Agriculture 178, 105760. https://doi.org/10.1016/j.compag.2020.105760
Luvisi, A.; Ampatzidis, Y.; Bellis, L.D. Plant pathology and information technology: opportunity and uncertainty in pest management. Sustainability 2016, 8, 831.
Madec, S., Jin, X., Lu, H., De Solan, B., Liu, S., Duyme, F., ... & Baret, F. (2019). Ear density estimation from high resolution RGB imagery using deep learning technique. Agricultural and forest meteorology, 264, 225-234.
Mahlein, A.K. Plant disease detection by imaging sensors — parallels and specific demands for precision agriculture and plant phenotyping. Plant Dis 2016, 100, 241-251.
McNicol, G., Bulmer, C., D’Amore, D., Sanborn, P., Saunders, S., Giesbrecht, I., Arriola, S. G., Bidlack, A., Butman, D., & Buma, B. (2019). Large, climate-sensitive soil carbon stocks mapped with pedology-informed machine learning in the North Pacific coastal temperate rainforest. Environmental Research Letters, 14(1), 14004. https://doi.org/10.1088/1748-9326/aaed52
Medar, R., & Rajpurohit, V. (2014). A survey on data mining techniques for crop yield prediction. International Journal of Advance Research in Computer Science and Management Studies, 2(9), 59-64.
Mehta S. and T. Burks. (2014) Vision-based control of robotic manipulator for citrus harvesting. Computers and Electronics in Agriculture, 102:146–158.
Mehta, S. and T. Burks. (2016) Adaptive visual servo control of robotic harvesting systems. IFAC-Papers On Line, 49 (16):287–292.
Mehta, S., W. MacKunis, and T. Burks. (2016) Robust visual servo control in the presence of fruit motion for robotic citrus harvesting. Computers and Electronics in Agriculture, 123:362–375.
Morellos, A., Pantazi, X.-E., Moshou, D., Alexandridis, T., Whetton, R., Tziotzios, G., Wiebensohn, J., Bill, R., & Mouazen, A. M. (2016). Machine learning based prediction of soil total nitrogen, organic carbon and moisture content by using VIS-NIR spectroscopy. Biosystems Engineering, 152, 104–116. https://doi.org/https://doi.org/10.1016/j.biosystemseng.2016.04.018
Mureşan, H., & Oltean, M. (2018). Fruit recognition from images using deep learning. Acta Universitatis Sapientiae, Informatica, 10, 26–42.
Musser W.N., Shortle JS, Kreahling K, Roach B, Huang WC, Beegle DB, Fox RH. (1995). An economic analysis of the pre-sidedress nitrogen test for Pennsylvania corn production. Review of Agricultural Economics 17(1):25-35.
Nahari, R. V., Jauhari, A., Hidayat, R., & Alfita, R. (2017). Image Segmentation of Cows using Thresholding and K-Means Method. International Journal of Advanced Engineering, Management and Science, 3(9), 913–918. https://doi.org/10.24001/ijaems.3.9.2
Naroui Rad, M. R., Ghalandarzehi, A., & Koohpaygani, J. A. (2017). Predicting Eggplant Individual Fruit Weight Using an Artificial Neural Network. International Journal of Vegetable Science, 23(4), 331-339. doi:10.1080/19315260.2017.1290001
Nasirahmadi, A., Hensel, O., Edwards, S. A., & Sturm, B. (2017). A new approach for categorizing pig lying behaviour based on a Delaunay triangulation method. Animal, 11(1), 131–139. https://doi.org/10.1017/S1751731116001208
Nasirahmadi, A., Edwards, S. A., & Sturm, B. (2017). Implementation of machine vision for detecting behaviour of cattle and pigs. Livestock Science, 202, 25-38. https://doi.org/10.1016/j.livsci.2017.05.014
National Research Council. (2002). Structural implications of technology transfer and adoption. Publicly funded agricultural research and the changing structure of US agriculture, 52-68.
National Science & Technology Council. (2019). The National artificial intelligence research and development strategic plan: 2019 update.
Nemitz, Paul. "Constitutional democracy and technology in the age of artificial intelligence." Philosophical Transactions of the Royal Society A: Mathematical, Physical and Engineering Sciences 376.2133 (2018): 20180089.
Nicole Ludwig, Stefan Feuerriegel & Dirk Neumann (2015) Putting Big Data analytics to work: Feature selection for forecasting electricity prices using the LASSO and random forests, Journal of Decision Systems, 24:1, 19-36, DOI: 10.1080/12460125.2015.994290
Nilsson, M., Ardö, H., Åström, K., Herlin, A., Bergsten, C., & Guzhva, O. (2014). Learning Based Image Segmentation of Pigs in a Pen. Isual Observation and Analysis of Vertebrate and Insect Behavior–Workshop at the 22nd International Conference on Pattern Recognition (ICPR 2014), 24–28. Retrieved from http://homepages.inf.ed.ac.uk/rbf/vaib14.html
Nisbet, M. C., & Scheufele, D. A. (2009). What's next for science communication? Promising directions and lingering distractions. Botany, 96(10). 1767–1778. https://doi.org/10.3732/ajb.0900041.
Ng, W., Minasny, B., Montazerolghaem, M., Padarian, J., Ferguson, R., Bailey, S., & McBratney, A. B. (2019). Convolutional neural network for simultaneous prediction of several soil properties using visible/near-infrared, mid-infrared, and their combined spectra. Geoderma, 352, 251–267. https://doi.org/https://doi.org/10.1016/j.geoderma.2019.06.016
Nord, Tina. 2021. What are Aritificial Nueral Networks? CXPulse, https://www.ultimate.ai/blog/ultimate-knowledge/what-are-artificial-neural-networks. Last Accessed: June 07, 2021.
Oczak, M., Viazzi, S., Ismayilova, G., Sonoda, L. T., Roulston, N., Fels, M., … Vranken, E. (2014). Classification of aggressive behaviour in pigs by activity index and multilayer feed forward neural network. Biosystems Engineering, 119, 89–97. https://doi.org/10.1016/j.biosystemseng.2014.01.005
OECD (Organization for Economic Cooperation and Development). 2016. Farm Management Practices to Foster Green Growth, OECD Publishing, Paris. http://dx.doi.org/10.1787/9789264238657-en
Padarian, J., Minasny, B., & McBratney, A. B. (2020). Machine learning and soil sciences: a review aided by machine learning tools. SOIL, 6(1), 35–52. https://doi.org/10.5194/soil-6-35-2020
Pajares, G. Overview and current status of remote sensing applications based on unmanned aerial vehicles (UAVs). Photogrammetric Engineering & Remote Sensing 2015, 81, 281-330.
Pane, Y. P., Nageshrao, S. P., Kober, J., & Babuška, R. (2019). Reinforcement learning based compensation methods for robot manipulators. Engineering Applications of Artificial Intelligence, 78(November 2018), 236–247. https://doi.org/10.1016/j.engappai.2018.11.006
Pantazi, X. E., Moshou, D., Alexandridis, T., Whetton, R. L., & Mouazen, A. M. (2016). Wheat yield prediction using machine learning and advanced sensing techniques. Computers and Electronics in Agriculture, 121, 57-65. doi:https://doi.org/10.1016/j.compag.2015.11.018
Parker, C. (1999, September). A user-centred design method for agricultural DSS. In EFITA-99: Proceedings of the Second European Conference for Information Technology in Agriculture. Bonn, Germany (pp. 27-30).
Paudel, K. P., Mishra, A. K., Pandit, M., & Segarra, E. (2021). Event dependence and heterogeneity in the adoption of precision farming technologies: A case of US cotton production. Computers and Electronics in Agriculture, 181, 105979.
Peterson, K. T., Sagan, V., & Sloan, J. J. (2020). Deep learning-based water quality estimation and anomaly detection using Landsat-8/Sentinel-2 virtual constellation and cloud computing. GIScience & Remote Sensing, 57(4), 510-525.
Pham, T. D., Yokoya, N., Nguyen, T. T. T., Le, N. N., Ha, N. T., Xia, J., Takeuchi, W., & Pham, T. D. (2021). Improvement of Mangrove Soil Carbon Stocks Estimation in North Vietnam Using Sentinel-2 Data and Machine Learning Approach. GIScience & Remote Sensing, 58(1), 68–87. https://doi.org/10.1080/15481603.2020.1857623
Pound, M. P., Atkinson, J. A., Townsend, A. J., Wilson, M. H., Griffiths, M., Jackson, A. S., ... & French, A. P. (2017). Deep machine learning provides state-of-the-art performance in image-based plant phenotyping. Gigascience, 6(10), gix083.
Priyanka, T., Soni, P., & Malathy, C. (2018). Agricultural Crop Yield Prediction Using Artificial Intelligence and Satellite Imagery. Eurasian Journal of Analytical Chemistry, 13(SP), 6-12. Retrieved from http://www.eurasianjournals.com/Agricultural-Crop-Yield-Prediction-Using-Artificial-Intelligence-and-Satellite-Imagery,105697,0,2.html
Pyo, J., Duan, H., Baek, S., Kim, M. S., Jeon, T., Kwon, Y. S., ... & Cho, K. H. (2019). A convolutional neural network regression for quantifying cyanobacteria using hyperspectral imagery. Remote Sensing of Environment, 233, 111350.
Rady, A., and Adedeji, A.A. (2020). Application of hyperspectral imaging to detect adulterants in minced meat. Food Analytical Methods 13(4), 970–981.
Rahnemoonfar, M., & Sheppard, C. (2017). Deep count: fruit counting based on deep simulated learning. Sensors, 17, 905.
Rambla, J.; Gonzalez-Mas, M.C.; Pons, C.; Bernet, G.; Asins, M.J.; Granell, A. Fruit volatile profiles of two citrus hybrids are dramatically different from their parents. J Agric Food Chem 2014, 62, 11312–11322.
Ren, S., He, K., Girshick, R., & Sun, J. (2015). Faster R-CNN: Towards Real-Time Object Detection with Region Proposal Networks. Advances in Neural Information Processing Systems, 91–99. https://doi.org/10.1109/TPAMI.2016.2577031.
Rogers, E. M. 2003. Diffusion of Innovations. 5th ed. New York: Free Press.
Ronneberger, O. F. (2015). U-net: Convolutional networks for biomedical image segmentation. International Conference on Medical image computing and computer-assisted intervention, (pp. 234-241).
Ronneberger, O., Fischer, P., & Brox, T. (2015). U-net: Convolutional networks for biomedical image segmentation. International Conference on Medical image computing and computer-assisted intervention, (pp. 234–241).
Rowe, E., Dawkins, M. S., & Gebhardt-Henrich, S. G. (2019). A systematic review of precision livestock farming in the poultry sector: Is technology focussed on improving bird welfare? Animals, 9(9), 1–18. https://doi.org/10.3390/ani9090614
Sa, I., Ge, Z., Dayoub, F., Upcroft, B., Perez, T., & McCool, C. (2016). DeepFruits: A Fruit Detection System Using Deep Neural Networks. Sensors, 16(8), 1222. https://doi.org/10.3390/s16081222.
Sagan, V., Peterson, K. T., Maimaitijiang, M., Sidike, P., Sloan, J., Greeling, B. A., ... & Adams, C. (2020). Monitoring inland water quality using remote sensing: potential and limitations of spectral indices, bio-optical simulations, machine learning, and cloud computing. Earth-Science Reviews, 103187.
Sahin-Çevik, M.; Moore, G.A. Quantitative trait loci analysis of morphological traits in citrus. Plant Biotechnol 2012, Rep 6, 47–57.
Saiz-Rubio, Verónica, and Francisco Rovira-Más. "From smart farming towards agriculture 5.0: A review on crop data management." Agronomy 10.2 (2020): 207.
Samek, Wojciech, Thomas Wiegand, and Klaus-Robert Müller. "Explainable artificial intelligence: Understanding, visualizing and interpreting deep learning models." arXiv preprint arXiv:1708.08296 (2017).
Sanderman, J., Savage, K., & Dangal, S. R. S. (2019). Mid-infrared spectroscopy for prediction of soil health indicators in the United States. Soil Science Society of America Journal, 84, 251–261. https://doi.org/10.1002/saj2.20009
Sandler, M., Howard, A., Zhu, M., Zhmoginov, A., & Chen, L. C. (2018). MobileNetV2: Inverted Residuals and Linear Bottlenecks. Proceedings of the IEEE Computer Society Conference on Computer Vision and Pattern Recognition, 4510–4520. https://doi.org/10.1109/CVPR.2018.00474
Schimmelpfennig, D. 2016. Farm Profits and Adoption of Precision Agriculture, ERR-217, U.S. Department of Agriculture, Economic Research Service, October.
Shakoor, N.; Lee, S.; Mockler, T.C. High throughput phenotyping to accelerate crop breeding and monitoring of diseases in the field. Current Opinion in Plant Biology 2017, 38, 184-192.
Sharma, Rohit, et al. "A systematic literature review on machine learning applications for sustainable agriculture supply chain performance." Computers & Operations Research 119 (2020): 104926.
Sheriff G. 2005. Efficient waste? Why farmers over-apply nutrients and the implications for policy design. Review of Agricultural Economics 27(4):542-557.
Simonyan, K., & Zisserman, A. (2014). Very Deep Convolutional Networks for Large-Scale Image Recognition. ImageNet Challenge, 1–10. https://doi.org/10.1016/j.infsof.2008.09.005.
Simonyan, K.; Zisserman, A. Very deep convolutional networks for large-scale image recognition. arXiv preprint arXiv 2015, 1409.1556.
Singh, A.; Ganapathysubramanian, B.; Singh, A.K.; Sarkar, S. Machine Learning for High-Throughput Stress Phenotyping in Plants. Trends in Plant Science 2016, 21, 110-124.
Sladojevic, S., Arsenovic, M., Anderla, A., Culibrk, D., & Stefanovic, D. (2016). Deep Neural Networks Based Recognition of Plant Diseases by Leaf Image Classification. Computational Intelligence and Neuroscience, 2016. https://doi.org/10.1155/2016/3289801.
Steen, K., Christiansen, P., Karstoft, H., & Jørgensen, R. (2016). Using Deep Learning to Challenge Safety Standard for Highly Autonomous Machines in Agriculture. Journal of Imaging, 2(1), 6. https://doi.org/10.3390/jimaging2010006.
Szegedy, C., Vanhoucke, V., Ioffe, S., Shlens, J., & Wojna, Z. (2015). Rethinking the Inception Architecture for Computer Vision. https://doi.org/10.1109/CVPR.2016.308.
Tan, M., & Le, Q. V. (2019). EfficientNet: Rethinking model scaling for convolutional neural networks. 36th International Conference on Machine Learning, ICML 2019, 2019-June, 10691–10700.
Tang, Y., Chen, M., Wang, C., Luo, L., Li, J., Lian, G., & Zou, X. (2020). Recognition and Localization Methods for Vision-Based Fruit Picking Robots: A Review. Frontiers in Plant Science, 11(May), 1–17. https://doi.org/10.3389/fpls.2020.00510
Tsai, D. M., & Huang, C. Y. (2014). A motion and image analysis method for automatic detection of estrus and mating behavior in cattle. Computers and Electronics in Agriculture, 104, 25–31. https://doi.org/10.1016/j.compag.2014.03.003
Ubbens, J. R., & Stavness, I. (2017). Deep plant phenomics: a deep learning platform for complex plant phenotyping tasks. Frontiers in plant science, 8, 1190.
Uzal, L. C., Grinblat, G. L., Namías, R., Larese, M. G., Bianchi, J. S., Morandi, E. N., & Granitto, P. M. (2018). Seed-per-pod estimation for plant breeding using deep learning. Computers and electronics in agriculture, 150, 196-204.
van Klompenburg, T., Kassahun, A., & Catal, C. (2020). Crop yield prediction using machine learning: A systematic literature review. Computers and Electronics in Agriculture, 177, 105709. doi:https://doi.org/10.1016/j.compag.2020.105709
Vardi, A.; Levin, I.; Carmi, N. Induction of seedlessness in citrus: from classical techniques to emerging biotechnological approaches. J Am Soc Hortic Sci 2008, 133, 117–126.
Viazzi, S., Bahr, C., Schlageter-Tello, A., Van Hertem, T., Romanini, C. E. B., Pluk, A., … Berckmans, D. (2012). Analysis of individual classification of lameness using automatic measurement of back posture in dairy cattle. Journal of Dairy Science, 96(1), 257–266. https://doi.org/10.3168/jds.2012-5806
Volle, K. P. Ganesh, T. Burks, S. Mehta, 2020. Semi-self-supervised sementation of oranges with small sample sizes, 2020 ASABE Annual International Meeting, Omaha, NE, July 12-15, 2020, Paper no. 1397.
Voulodimos, A., Doulamis, N., Doulamis, A., & Protopapadakis, E. (2018). Deep Learning for Computer Vision: A Brief Review. Computational Intelligence and Neuroscience, 2018. https://doi.org/10.1155/2018/7068349.
Wang, X., Xuan, H., Evers, B., Shrestha, S., Pless, R., & Poland, J. (2019). High-throughput phenotyping with deep learning gives insight into the genetic architecture of flowering time in wheat. GigaScience, 8(11), giz120.
Weersink A, Fraser E, Pannell D, Duncan E, and Rotz S. 2018. Opportunities and challenges for big data in agricultural and environmental analysis. Annual Review of Resource Economics 10:19-37.
Wolfert S, Ge L, Verdouw C, Bogaardt M. 2017. Big data in smart farming – a review. Agricultural Systems 153:69-80.
Wu, D., Wu, D., Feng, H., Duan, L., Dai, G., Liu, X., ... & Yang, W. (2021). A deep learning-integrated micro-CT image analysis pipeline for quantifying rice lodging resistance-related traits. Plant communications, 2(2), 100165.
Xie, S., Girshick, R., & Doll, P. (2017). Aggregated Residual Transformations for Deep Neural Networks http://arxiv.org/abs/1611.05431v2. Cvpr, 1492–1500.
Yamamoto, K., Guo, W., Yoshioka, Y., & Ninomiya, S. (2014). On Plant Detection of Intact Tomato Fruits Using Image Analysis and Machine Learning Methods. Sensors, 14(7), 12191-12206. Retrieved from https://www.mdpi.com/1424-8220/14/7/12191
Yeom J, J. Jung, A. Chang, A. Ashapure, M. Maeda, A. Maeda, J. Landivar. 2019. Comparison of Vegetation Indices Derived from UAV Data for Differentiation of Tillage Effects in Agriculture. Remote Sens, 11:1548.
Yim, I., Shin, J., Lee, H., Park, S., Nam, G., Kang, T., ... & Cha, Y. (2020). Deep learning-based retrieval of cyanobacteria pigment in inland water for in-situ and airborne hyperspectral data. Ecological Indicators, 110, 105879.
Yuan, Q., Shen, H., Li, T., Li, Z., Li, S., Jiang, Y., … Zhang, L. (2020). Deep learning in environmental remote sensing: Achievements and challenges. Remote Sensing of Environment, 241(March 2019), 111716. https://doi.org/10.1016/j.rse.2020.111716
Zannou, J. G. N., & Houndji, V. R. (2019, 24-26 April 2019). Sorghum Yield Prediction using Machine Learning. Paper presented at the 2019 3rd International Conference on Bio-engineering for Smart Technologies (BioSMART).
Zeiler, M. D., & Fergus, R. (2014). Visualizing and understanding convolutional networks. Lecture Notes in Computer Science (Including Subseries Lecture Notes in Artificial Intelligence and Lecture Notes in Bioinformatics), 8689 LNCS(PART 1), 818–833. https://doi.org/10.1007/978-3-319-10590-1_53.
Zhang, Y.-D., Dong, Z., Chen, X., Jia, W., Du, S., Muhammad, K., & Wang, S.-H. (2019). Image based fruit category classification by 13-layer deep convolutional neural network and data augmentation. Multimedia Tools and Applications, 78, 3613–3632.
Zhang, Naiqian, Maohua Wang, and Ning Wang. "Precision agriculture—a worldwide overview." Computers and electronics in agriculture 36.2-3 (2002): 113-132.
Zhang, Z.; Jin, Y.; Chen, B.; Brown, P. 2019. California Almond Yield Prediction at the Orchard Level with a Machine Learning Approach. Frontiers in Plant Science, 10, 809.
Zhao, B., Li, J., Baenziger, P. S., Belamkar, V., Ge, Y., Zhang, J., & Shi, Y. (2020). Automatic Wheat Lodging Detection and Mapping in Aerial Imagery to Support High-Throughput Phenotyping and In-Season Crop Management. Agronomy, 10(11), 1762.
Zhao, Y., L. Gong, Y. Huang, and C. Liu.(2016) A review of key techniques of vision-based control for harvesting robot. Computers and Electronics in Agriculture, 127:311–323.
Zheng, Q.M.; Tang, Z.; Xu, Q.; Deng, X.X. Isolation, phylogenetic relationship and expression profiling of sugar transporter genes in sweet orange (Citrus sinensis) plant cell tissue and organ. Culture 2014, 119, 609–624.
Zhuang, X., Bi, M., Guo, J., Wu, S., & Zhang, T. (2018). Development of an early warning algorithm to detect sick broilers. Computers and Electronics in Agriculture, 144(November 2017), 102–113. https://doi.org/10.1016/j.compag.2017.11.032
Zilberman D, Khanna M, Lipper L. 1997. Economics of new technologies for sustainable agriculture. Australian Journal of Agricultural and Resource Economics 41(1):63-80.