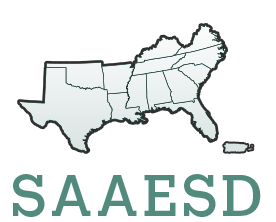
S1090: AI in Agroecosystems: Big Data and Smart Technology-Driven Sustainable Production
(Multistate Research Project)
Status: Active
Homepage
The need as indicated by stakeholders
The competitive nature of modern agriculture demands agribusiness firms innovate and adapt quickly to capture benefits from advances in new technology such as artificial intelligence (AI). Throughout the value chain, there is a critical need to increase efficiency and protect the bottom line. Although currently lagging behind other industries, agriculture is forecasted to experience a “digital revolution” over the next decade. Global growth of AI applications in agriculture is expected to average 22.5% per year, reaching $186 billion by 2025, according to a recent report by Adroit Market Research. Growers, for example, are constantly exploring the best opportunities to increase yield and profit as expressed by Iowa corn and soybean producers at the recent NSF Convergence Accelerator Workshop for Digital and Precision Agriculture. Participants strongly voiced the need for new technology to answer “what is my best opportunity?” to enhance crop productivity and strengthen their bottom line.
Based on the National Science & Technology Council (2019) report, the American Artificial Intelligence Initiative was established in 2019 to maintain American leadership in AI and ensure AI benefits to the American people. The initiative set up long-term investments in AI research as one of the priorities. Following the initiative, USDA/NIFA invests in many AI-related programs such as the Food and Agriculture Cyberinformatics and Tools (FACT) and other programs for crop and soil monitoring systems, autonomous robots, computer vision algorithms, and intelligent decision support systems.
AI allows computers and machines to carry out tasks without human cognition. AI includes machine learning (ML) and deep learning (DL). Machine learning is a data analysis method to imitate human learning. Examples of using ML in agriculture include crop production (machine vision, yield prediction, pest and disease detection, and phenotyping) and agricultural robotics (navigation, self-leaning, man-machine synergy, and optimization). Deep learning is a subset of machine learning and consists of artificial neural networks to mimic human brain functions. Recently, deep learning has driven huge improvements in various computer vision problems, such as object detection, motion tracking, action recognition, pose estimation, and semantic segmentation (Voulodimos et al., 2018). Internet of Things (IoT) is a technology that enables data acquisition and exchange using sensors and devices through a network connection. IoT tends to generate big data, which requires AI to make valuable inferences.
Crop growth is a complex and risky process and difficult for producers to analyze in isolation. The emergence of information technology has developed a large amount of data, i.e., big data, that can be analyzed utilizing AI to provide valuable decision support to producers, particularly large-scale operations. AI can provide predictive analytics for growers to better manage risks through improved preparation and response for unexpected events such as severe flooding and drought. The Colorado-based company, aWhere, uses machine learning algorithms in connection with satellites and 1.9 million weather stations (virtual) to predict daily weather patterns for clientele, including farmers, crop consultants, and researchers. Improved crop and irrigation planning assist a global network of growers in reducing water usage with a particular focus on the impacts of climate change.
Robotics and visual machine learning platforms enable automation of critical labor activities such as field scouting and harvesting. Pest control companies have begun using aerial drone technology to cut costs in the labor-intensive practice of scouting pests and diseases. According to Brian Lunsford of the Georgia-based Inspect-All company, “In 2016, we performed our first paid drone inspection after months of testing. Most importantly, we wanted to make sure our flight operators could safely fly our drones and provide our customers with substantial value, while at the same time being mindful of privacy concerns.” As reported by Protein Industries Canada, AI-assisted pest and disease monitoring systems can save pesticide use up to 95% and reduce costs by $52 per acre.
Emerging AI technologies are expected to reach a broader spectrum of the value chain. While prior technologies focused primarily on grain commodities, machine learning and advanced visual machine learning algorithms will enable stakeholders from fruits and vegetables to improve harvest efficiency using platforms such as flying robot pickers. Early adopters such as John White of Marom Orchards support the use of AI in response to the need “to pay wages, organize visas, housing, food, healthcare and transportation” for a large number of workers and to address critical labor shortages due to “hard, seasonal work and other crops can pay higher wages. Young people all over the world are abandoning agricultural work in favor of higher paying, full-time urban jobs.” Small, labor-intensive farm operations can thus expand production opportunities by reducing harvest losses by 10% while reallocating freed-up labor to alternative enterprises, alleviating concerns over expected labor shortages that are expected to reach $5 million by 2050, according to Marom Orchards.
Downstream on the value chain, AI is also expected to have a strong demand over the coming decade. A recent article in Food Online lists three new types of AI technology to improve supply chain management, including: (1) food safety monitoring and testing of product along the supply chain; (2) improved marketing analysis of price and inventory; and (3) a comprehensive “farm to fork” tracking of product. In the food processing industry, visually based AI algorithms combined with machine learning are used by companies such as TOMRA Sorting Food to “view food in the same way that consumers do” and sort products based on consumer preferences and quality. AI reduces labor time compared to manual sorting, reduces wasted product, and enhances product quality delivered to consumers. To fine-tune product development and optimally satisfy consumer preferences, startup companies such as Gastrograph AI use machine learning to assist their clientele in fine-tuning product development. According to their website, their services ``interpret and predict flavor preferences for over a billion unique consumer groups. This technology empowers companies to look beyond trends, and formulate unique, novel and successful food and beverage products based on custom sensory intelligence.” Visually based AI and machine learning are also expected to be in high demand to improve hygiene in both manufacturing plants and restaurants. The use of AI in the cleaning of manufacturing equipment is projected by the University of Nottingham researchers to reduce cleaning costs by up to 40%.
The importance of the work, and what the consequences are if it is not done
There are approximately 1 billion people who have no access to clean water or sanitation. The same number have insufficient food to prevent them from chronic hunger, and ultimately, starvation. To tackle those challenges, improvements in agricultural production systems must be achieved, which requires a better understanding of the complex agricultural ecosystems. AI is projected to be one of the primary drivers to help study complex systems. Recent advances in AI, including big data, machine learning, and robotics, have helped achieve breakthroughs in various areas, such as healthcare, medicine, marketing, manufacturing, autonomous driving, etc. The field of agroecosystem has seen increasing applications of AI in recent years as well. However, more research must be done to transfer the general AI technologies into agroecosystem-specific AI technologies.
The importance of this project lies in that it will help tackle multiple pressing challenges that we are currently facing in the agroecosystem. The current AI technologies are not explicitly tuned for agroecosystem, which causes problems such as low accuracy in prediction, inefficiency in using computer resources, inefficiency in data management, and not being cost-effective for most agricultural crop productions. Also, the lack of next-generation farmers and workers in this area will be a major bottleneck for adopting and applying AI technologies. Included in this project, multiple AI-centered projects will be conducted at multiple states in the southeast U.S. to develop AI tools suitable for specific applications important to the improvement of production and sustainability of the agroecosystem. The project will also assess the feasibility of different AI technologies and showcase the value of those technologies to stakeholders to improve AI adoption. Additionally, this work will help develop the workforce for the future agricultural production system. Tasks in this project should be completed quickly and efficiently to ensure that production in agriculture meets the global needs in the near future and ensures the sustainability of the agroecosystem. It is also essential that technologies in agriculture must keep up with technologies in other fields to attract more talented people to ensure workforce sustainability.
The shift of agriculture to intensive production over the past several decades has led to a dramatic increase in the use of chemical inputs, particularly fertilizer. Although necessary to feed a growing world population, agriculture is a significant contributor to climate change. In a typical year, agriculture’s carbon and related environmental footprints account for 10% to 15% of the world’s greenhouse gas (GHG) emissions. Fertilizer is particularly problematic, contributing about 2.5% of the world’s GHG emissions. Broadcast applications of fertilizer are inefficient, with large portions remaining unused. Subsequent erosion and runoff pollute local watersheds resulting in algae blooms and related environmental problems. Fertilizer GHG emissions are often the most severe: nitrous oxide, for example, has been found to warm the atmosphere about 300 times more compared to CO2.
Artificial intelligence is expected to greatly assist in reducing agriculture's environmental footprint and contributions to climate change while continuing to increase productivity and input efficiency. Our project will develop a suite of AI-enabled technologies using sensors, actuators, and robotic platforms to increase the precision and efficiency of farm and ranch management practices, reducing overall input use and GHG emissions. Such precision-based agriculture is expected to play a critical role in mitigating agriculture's contribution to climate change. Projections from the World Economic Forum indicate that GHG emissions and water use could be reduced by 10% and 20% if precision agriculture were adopted on upwards of 25% of farms worldwide.
Livestock agri-food systems are at the crossroads of human, animal, and environmental health, and animal welfare is a priority in all livestock systems globally (FAO, 2018). Good animal welfare requires disease prevention, veterinary treatment, appropriate management, nutrition, and humane slaughter of livestock. Increasing standards for animal welfare has led to considerable research activities into ways to monitor and measure a wide range of traits that can be used for management (Bell & Tzimiropoulos, 2018). Due to the high labor cost and inaccuracy of traditional methods of expert evaluation, there is increasing awareness that the monitoring of animals requires the adoption of innovative AI technologies (Nasirahmadi, Edwards, & Sturm, 2017).
The technical feasibility of the research
The technical feasibility of the research can be summarized in three aspects. Firstly, the applications of the most recent AI technologies have been proven effective and helped improve various areas in the agroecosystem. As presented in the literature reviews of this proposal, AI has helped in areas such as yield prediction, crop quality assessment, environmental monitoring, phenotyping, genotyping, etc., in the past decade. Furthermore, an extensive number of studies have shown the power of AI and its potential in improving production and sustainability in most, if not all, aspects of the agroecosystem.
Secondly, the research activities proposed in this project are based on substantial preliminary studies. Team members on this project have expertise in remote sensing, machine vision, robotics, automation, UAVs, AI models, water management, hydrology, soil spectroscopy, natural resource economics and policy, and ecosystem services evaluation. All members have had substantial experience in conducting research, teaching, and extension activities in their respective focus areas. Proposed activities stem from the team’s preliminary studies, which ensures the technical feasibility and success of this project.
Thirdly, the team proposed a closed-loop method to balance the impact and feasibility of the research. Feedbacks gathered from extension activities will be communicated to the team through regular meetings to help refine objectives and approaches throughout the entire duration of the project. In addition, information such as newly discovered constraints, changes in specific operations in each production system will be shared and discussed to facilitate evaluating the technical feasibility of each sub-objective.
The advantages for doing the work as a multistate effort
Each state has different experts in different research areas. Also, different crops are grown in different states, and their cropping conditions are not the same. Therefore, working as a multistate team creates more opportunities for collaborating in various disciplines from other states.
From the survey completed in May 2021 at SAASED Institutions (SAASED, 2021), only 46% of the respondents have developed a partnership with other institutions. The survey also found that it is less likely that researchers know what other institutions are doing, and there is not much coordination among them. Therefore, this multistate project will facilitate more productive collaboration through organized coordination to complete the tasks proposed in this project.
During the proposal development stage in May-July 2021, we had meetings every one or two weeks, and there were 15-20 participants for most of the meetings. As a group, we came up with the title, defined objectives, and established the writing team for each specific objective. For each objective, a leader was chosen to lead the writing activities. All members were committed to accomplishing their assigned tasks of writing an introduction, literature review, and detailed activities, along with outputs, outcomes, and milestones, which resulted in this proposal.
During the project period, all participants from multiple institutions will meet once a year to discuss their research activities, major findings, current issues, funding opportunities, potential collaborations, and future directions. A listserv of emails will be created to facilitate efficient communication among the participants. An online cloud folder will be created to share and maintain data and information. By the end of this project, we anticipate multiple co-authored journal publications, funded research projects, outreach events, and Extension publications among the participants.
What the likely impacts will be from successfully completing the work
The likely impacts of this project include better preparation for harvest and storage with improved yield prediction, the timely intervention of control measures to address pest infestation, and reduced loss associated with low-quality produce in the supply chain.
Another potential impact is that farmers are able to maximize their return through timely harvesting, application of treatments to reduce loss, and consumers’ confidence through assurance of high-quality products. For fruit crops, automated fruit detection systems will enhance site-specific crop management practices to increase yield and profit.
For scouting and monitoring natural resources, the developed calibration models in this project can serve various stakeholders, including farmers and USDA-NRCS, to rapidly derive soil properties, which will reduce the cost and time of their projects. In addition, new technological improvements will be introduced, which can be utilized to develop new in situ sensors for the rapid estimation of soil properties.
This research will provide future scenarios projecting economic and social impacts from the adoption and use of AI technology. It is expected that results will be disseminated to producers, policymakers, and stakeholders and will be used as a key input in decision-making. This will provide more informed and improved choices resulting in more streamlined and socially optimal agricultural practices and policy outcomes.
AI-based algorithms depend heavily on the existence of large, clean, and information-rich databases. By combining multiple datasets from multiple states, the users will see a dramatic increase in their algorithms’ predictive measures and pattern recognition ability.