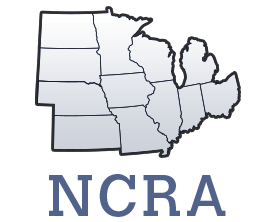
NCCC170: Research Advances in Agricultural Statistics
(Multistate Research Coordinating Committee and Information Exchange Group)
Status: Active
NCCC170: Research Advances in Agricultural Statistics
Duration: 10/01/2021 to 09/30/2026
Administrative Advisor(s):
NIFA Reps:
Non-Technical Summary
Statement of Issues and Justification
Statisticians who consult and do research in an Agricultural Experiment Station (AES) environment have a unique relationship with their university. Their multi-disciplinary, collaborative role is often more extensive than that of faculty members in domain-specific disciplines as well as that of most faculty in Statistics departments. Indeed, the role of statisticians who consult and do AES research frequently cuts across several departments or units. As a result, AES statisticians must have a broad understanding of statistical methodology that goes well beyond their specific research areas. This multi-state coordinating committee provides a mechanism to bring together AES statisticians from academia, research stations, government and industries and to create critical mass for continued professional development that is specifically targeted to the unique role of AES statisticians. The resulting exchange of ideas and the sharing of knowledge is of benefit to all project members and to the institutions they represent.
Collaboration of such statisticians and scientists allows land grant institutions to perform their agricultural research missions more effectively and efficiently than would otherwise be possible. As State and Federal appropriations continue to fall short of levels needed for research, and as grants become more competitive, it becomes even more imperative that research dollars extend as far as possible. This requires AES statisticians to keep abreast of the latest statistical research, as well as to work to develop methodology appropriate to their consulting roles. Advances in the complexity of designed experiments and observational studies, along with a broader range of qualitative and quantitative types of data being collected in increasingly larger quantities (i.e., Big Data), require new methodologies in both design and analysis that ensure both the quality of the data and the inferences drawn. Unfortunately, it is also not unusual for statisticians who do not have AES responsibilities to misunderstand these essential scholarly contributions of AES statisticians.
Never has the charge to remain current in the statistical profession been more challenging. For statisticians, this requires assessing the utility of new statistical methods and their associated software tools and routines, many of which are computationally intensive. Rapid changes in our understanding of best statistical practices also means that agricultural researchers face an unprecedented need for viable mechanisms to stay current as consumers of statistics. Unfortunately, it is not unusual for agricultural researchers who use state of the art statistical methodology to experience difficulty during their institutional review process because the review infrastructure consists primarily of subject-matter experts who are not familiar with current advances in statistical practice. Dissemination of information on these statistical advances to agricultural researchers, and those who review agricultural research, must be made available in a timely manner.
Thus, it is critical that AES statisticians work cooperatively to determine the best current approaches to common statistical problems, to help guide future directions of statistical research and software development, to educate researchers and reviewers in new developments in statistical methodology, and to articulate the scholarship of their contributions to non-AES statisticians and to administrators involved in the evaluation, tenure and promotion processes.
In this respect, AES statisticians and their counterparts in government and industry must work together to provide more effective assistance to the agricultural researchers of their respective states. This NCCC committee plays an extremely important role by 1) serving as a focal point of critical mass for the development and implementation of sound statistical practice to food and agriculture research programs and 2) providing a support network of direct relevance to AES statisticians, both on technical issues as well as on matters of professional mentorship and development. Through the NCCC committee, members have direct access to and are able to contact other members as a resource about specific statistical matters relevant to the many facets of the agricultural sciences. Perhaps even more important, this NCCC network facilitates timely mentorship to its members in dealing with the unique nature of responsibilities of an AES statistician at its home institution. This uniqueness and specificity of the NCCC committee as a professional network raises its efficacy well above that offered by other, more general, collaborative venues, such as professional meetings for statisticians or for agricultural scientists. In fact, the NCCC annual meeting provides a unique platform for confluence of discussion of issues in agricultural statistics, the relevance of which is not matched by professional conferences either in statistics on in any of the agricultural sciences. As a result, this NCCC committee improves the quality of research in the agricultural sciences at its participating institutions through educational outreach within and beyond its NCCC membership.
Thus, this committee has an educational support component that ultimately assists agricultural researchers in other disciplines in their efforts to accomplish national research priorities. The members of this NCCC are committed to this goal.
Objectives
-
To identify, foster and coordinate educational and research efforts in statistics among statisticians serving food and agriculture research programs, thus enhancing their relevance to collaborative research teams at their home institutions.
-
To mentor new faculty interested in pursuing a career in agricultural statistics and to educate other statisticians and administrators involved in the faculty evaluation process about the scholarship contributions of AES statisticians and their role in the scientific community.
-
To provide continuing statistical education to the scientific community through workshops and short courses, thus empowering scientists to conduct and evaluate research through the review and editorial processes.
-
To address technical concerns associated with the development of modern statistical methodology and its software implementation, as motivated by problems in food and agricultural research, including but not limited to: - Hierarchical models, including Bayesian models and generalized linear mixed models; - Meta-analyses characterized by multi-location, multi-investigator projects including those in which study treatments and/or designs may differ by location; - Big data and data science; - Interface between statistics, machine learning, and other predictive modeling strategies.
Procedures and Activities
Since its inception in 1990 as NCR-170, NCCC-170 has addressed a series of statistical topics using a common general approach. At each annual meeting, the members discuss current research projects at their university or research station and the statistical issues associated with them. These topics are compiled and when appropriate, the members use this information to decide on a statistical topic to be addressed by the group. Once that decision has been made, a multi-year cycle begins.
The first and, if necessary, second year of the cycle is devoted to educating the membership on the chosen topic. This is accomplished primarily through the annual meeting technical programs presented by knowledgeable project members and, on occasion, by nonmembers (usually located at that year's meeting site). Once this phase has been completed, materials are developed for members to use in their research, education and outreach activities. These may include workshop materials for subject matter audiences, software and usage instructions for data analysis, and statistical material for members own continuing education.
Material development is carried out by a subcommittee of volunteers from the project membership. A draft of their work (e.g., a dry-run of a workshop) is presented at the next annual meeting to obtain feedback from the entire group. During this meeting, a decision is made regarding whether or not a final version needs to be presented at the annual meeting the following year.
Over the past 30 years, project members have dealt with statistical aspects of on-farm trials, experimental design, mixed models, spatial statistics, -omics data and bioinformatics, meta-analysis, and generalized linear mixed models. They have developed specialized workshops to educate both statistical and subject matter audiences on topics such as mixed models, spatial statistics, Bayesian statistics, graphical models, meta-analysis, and generalized linear mixed models.
A most noteworthy outcome is the mixed models workshop, which has been offered approximately every other year since 1999 to the American Society of Animal Sciences and/or to the American Dairy Science Association. This workshop has been successful and continues to be updated and offered upon request. Also, a workshop focused on the use of generalized linear mixed models for non-normal data was offered for the first time at the American Society of Agronomy, Soil Science Society of America, and the Crop Science Society annual meeting in 2010. A similar workshop was presented at the American Phytopathological Society.
Other workshops have been presented at the annual meetings of various professional societies, including the American Phytopathological Society, the American Society of Animal Sciences, the American Dairy Science Association, the Association of Veterinary Epidemiology and Preventive Medicine, the American Society of Horticultural Sciences, Aquaculture America, the Latin American Conference of Statistical Societies (Argentina), the International Horticulture Congress (Australia), and the Tropical Agronomic Center for Research and Teaching (Costa Rica) as well as at meetings of several local chapters of the American Statistical Association (ASA) and the International Biometrics Society. Some of these workshops were also presented as full day workshops prior to the Conference on Applied Statistics in Agriculture for multiple years. Several members have presented workshops at their home institutions.
A book on applications of generalized linear mixed models to the agricultural sciences was published by group members and sponsored by the American Society of Agronomy in 2012. This book, authored by E.E. Gbur, , W.W. Stroup, K.S. McCarter, S. Durham, L.J. Young, M. Christman, M. West and M. Kramer, is titled “Analysis of Generalized Linear Mixed Models in the Agricultural and Natural Resources Sciences”. A year later CRC Press published “Generalized Linear Mixed Models: Modern concepts, Methods and Applications” by group member W.W. Stroup. Both books continue to be used by practitioners and statisticians. Copies of both books are currently available on Amazon.com. Individual chapters of the former book may be purchased directly from the American Society of Agronomy.
Groups consisting of various combinations of project members have written scientific articles on translational statistics addressing domain-specific disciplines on how to tailor modern concepts in experimental design to specific problems in the agricultural sciences. These articles are published in peer-review scientific journals. A few examples are listed next (names of project members are underlined):
- Bello NM, Kramer M, Tempelman RJ, Stroup WW, St-Pierre NR, Craig, BA, Young LJ, Gbur, EE. Short communication: On recognizing the proper experimental unit in animal studies in the dairy sciences. J Dairy Sci. 2016;99(11):8871-8879. doi:10.3168/jds.2016-11516
- Bello NM, Renter DG. Invited review: Reproducible research from noisy data: Revisiting key statistical principles for the animal sciences. J Dairy Sci. 2018;101(7):5679-5701. doi:10.3168/jds.2017-13978
- Stroup, W.W. Rethinking the Analysis of Non-Normal Data in Plant and Soil Science. Agronomy Journal 2015 v. 107, no.2 pp. 811-827
- A selection of articles in Glaz, B. and M. Yeater (ed.) 2018. Applied Statistics in Agricultural, Biological, and Environmental Sciences. ASA, CSSA, and SSSA, Madison, WI. doi:10.2134/appliedstatistics
- Madden, L.V., Piepho, H.-P., and Paul, P.A. 2016. Statistical models and methods for network meta-analysis. Phytopathology 106: 792-806.
- Stroup W.W., Milliken, G.A, Claasen E.A. 2018 “SAS for Mixed Models” SAS Institute. 3rd
In addition to these group outputs, NCCC-170 discussions have also impacted project members' collaborations at their own institutions and have led to numerous refereed and non-refereed publications, participation in competitive grants, and presentations at professional meetings. These outputs are listed in detail in the corresponding annual project reports, available at https://nccc170.uark.edu/. Highlights from the 2020 report are listed below.
Finally, during the latest iteration of NCCC170, the project members came together to critically address the scholarship component of statistical collaboration for increasingly important team-based interdisciplinary research. The document produced provided a conceptual framework and practical guidelines for evaluating scholarship by project statisticians working in collaboration with domain-specific sciences. The intended audience for the document was all parties involved in the faculty evaluation process and especially administrators. In addition, the intent of this document was to assist young statistical scientists in their negotiation of faculty positions with a strongly collaborative team-based role. This document evolved into a position paper that was formally endorsed by the board of the American Statistical Association (ASA) in 2018. The position paper currently can be accessed as an official statement on the ASA website: https://www.amstat.org/asa/files/pdfs/POL-Statistics-as-a-Scientific-Discipline.pdf
Expected Outcomes and Impacts
- Continuing education of NCCC members leading to improved research collaborations of individual members at their home institutions.
- Cooperative research efforts among some members and information exchange on new developments among all members in the areas of modern modeling, including hierarchical Bayesian models, generalized linear mixed models, predictive modeling and machine learning.
- Continued offering of workshops and, where appropriate, alternative means of continuing education and support for subject matter scientists, reviewers, and technical editors on valid design and statistical analysis of studies.
- Growth of the group by recruitment of new membership from academia, government and industry.
Projected Participation
View Appendix E: ParticipationEducational Plan
Members will educate their individual clientele of subject matter scientists and students in one-on-one and group settings as they deem appropriate. Workshops will continue to be offered to regional and national subject matter groups as well as journal editorial boards who may be interested in specific topics.
Organization/Governance
The Chair and Secretary are selected by the members present at the annual meeting. The meeting Local Arrangement Chair for the next year is the member from the state in which the meeting will be held. The meeting Program Chair for the next year is a volunteer from among the members present at the annual meeting.
Literature Cited
This literature section contains selected publications from the Project's 2020 Annual Report as examples of the diversity of disciplines with which Project members interact. Names of project members are underlined.
- Chitakasempornkul, K., A. Jager, J.M. Rosa and N.M. Bello (2020). Hierarchical modeling of structural coefficients for heterogeneous networks, with an application to animal production systems. Journal of Agricultural, Biological and Environmental Statistics. DOI: 10.1007/ s13253-020-00389-0.
- Horvath, D.P., S.A. Clay, S.A. Bruggeman, J.V. Anderson, W.S. Chao, and Yeater (2019). Varying Weed Densities Alter the Corn Transcriptome, Highlighting a Core Set of Weed-Induced Genes and Processes with Potential for Manipulating Weed Tolerance. The Plant Genome 12 (3), 1-9.
- Tempelman, R.J. and Y. Lu. (2020). Genetic relationships between different measures of feed efficiency and the implications for dairy cattle selection indexes. Journal of Dairy Science 103: 5327-5345.
- Larson, D.L., M. Faisal, J. Tempelman, H. Yu, and K.T. Scribner. (2020). Effects of hatchery rearing density, handling, and nutrition on Renibacterium salmoninarum infection prevalence in juvenile Chinook salmon (Oncorhynchus tshawytscha). Journal of Aquatic Animal Health. https://doi.org/10.1002/aah.10103
- Lang, B.J., Dixon, P.M., Klaver, R.W, Thompson, J., and Widrlechner, M.P., (2019). Characterizing urban butterfly populations: The case for purposive point-count surveys. Urban Ecosystems 22(6):1083-1096.
- Bartel, C.A., Archontoulis, S.V., Lenssen, A.W., Moore, K.J., Huber, I.L., Laird, D.A., Fei, S., and Dixon, P.M. (2020). Modeling perennial groundcover effects on annual maize grain crop growth with the Agricultural Production Systems sIMulator. Agronomy Journal 112(3):1895-1910.
- Lang, B.J., Widrlechner, M.P., Dixon, P.M., and Thompson, J. (2020). Can climatic variables improve phenological predictions for butterfly species? Journal of Insect Conservation 24(2): 375-383.
- Ramos, P.M., Wright, S.A., Delgado, E.F., Santen, E. van, Johnson, D.D., Scheffler, J. ., Elzo, M.A., Carr, C.C., and Scheffler, T.L. (2020). Resistance to pH decline and slower calpain-1 autolysis are associated with higher energy availability early postmortem in Bos taurus indicus Meat Science, 159, 107925. doi: 10.1016/j.meatsci.2019.107925.
- Lopes, F., Magnabosco, C. U., Passafaro, T, L., Brunes, L. C., Costa, M. F. O., Eifert, E. C., Narciso, M. G., Rosa, G. J. M., Lobo, R. B. and Baldi, F. (2020) Improving genomic prediction accuracy for meat tenderness in Nellore cattle using artificial neural networks. Journal of Animal Breeding and Genetics 2020. https://doi.org/10.1111/jbg.12468.
- Koltes, J. E., Cole, J. B., Clemmens, R., Dilger, R. N., Kramer, L. M., Lunney, J. K., McCue, M. E., McKay, S. D., Mateescu, R. G., Murdoch, B. M., Reuter, R., Rexroad, C.E., Rosa, G. J. M., Serão, N. V. L., White, S. N., Woodward-Greene, M. J., Worku, M., Zhang, H. and Reecy, J. M. (2019). A vision for development and utilization of high-throughput phenotyping and big data analytics in livestock. Frontiers in Genetics 10: 1197.
- Fernandes, A. F. A., Turra, E. M., Alvarenga, E. R., Passafaro, T. L., Lopes, F. B., Alves, G. F. O., Singh, V. and Rosa, G. J. M. (2020). Deep Learning image segmentation for extraction of fish body measurements and prediction of body weight and carcass traits in Nile tilapia. Computers and Electronics in Agriculture 170: 105274.
- Passafaro, T.L., Fernandes, A. F. A., Valente, B. D., Williams, N. H. and Rosa, G. J. M. (2020). Network analysis of swine movements in a multi-site pig production system in Iowa, USA. Preventive Veterinary Medicine 174: 104856.
- Preiss, G., J.K. Norsworthy, T.N. Spurlock, T.L. Roberts and E. Gbur (2020). Soybean growth and incidence of soil-borne fungi as influenced by Metribuzin™. Agronomy Journal. doi:10.1002/agi2.20396.
- Torres, Norma, Vilda L. Rivera, Ingrid Y. Padilla, Raul E. Macchiavelli, David Kaeli, Akram N. Alshawabkeh (2019). Effect of hydrogeological and anthropogenic factors on the spatial and temporal distribution of CVOCs in the karst system of northern Puerto Rico. Environmental Earth Sciences 78:594. https://doi.org/10.1007/s12665-019-8611-7
- Giannini Kurina. F., S. Hang, Macchiavelli, M. Balzarini (2019). Spatial predictive modelling essential to assess the environmental impacts of herbicides. Geoderma 354: 113874. https://doi.org/10.1016/j.geoderma.2019.07.032
- Wolfe, B., Macchiavelli, S. Van Bloem (2019). Seed rain along a gradient of degradation in a Caribbean dry forest: Effect of dispersal limitation on the trajectory of forest recovery. Applied Vegetation Science 22(3):423-434. DOI: 10.1111/avsc.12444
- Kuruwita, D., Jiang, X., Darby, D., Sharp, J.L. and Fraser, A. (2020). Persistence of Escherichia coli O157:H7 and Listeria monocytogenes on the exterior of common food packaging materials. Journal of Food Control, 112.
- Evans, S., Sharp, J.L., and Vap, L. (2020). Optimizing the u411 automated urinalysis instrument for veterinary use. Veterinary Clinical Pathology, 49(1): 106-111.
- Martabano, B., Henriksen, M., Wang, Z. and Sharp, J.L. (2020). Effect of topical Diclofenac sodium 0.1% ophthalmic solution on intraocular pressure in healthy beagles when administered at four different frequencies (SID, BID, TID & QID). American Journal of Veterinary Research, 81(1): 41-46.
- Gauvin M.C., Pillai S.M., Reed S.A., Stevens J.R., Hoffman M.L., Jones A.K., Zinn S.A., and Govoni K.E. (2020). Poor maternal nutrition during gestation in sheep alters prenatal muscle growth and development in offspring. Journal of Animal Science 98(1):1-15.
- Mills, K.B., Madden, L.V., and Paul, P.A. (2020). Quantifying the effects of temperature and relative humidity on the development of wheat blast incited by the Lolium pathotype of Magnapothe oryzae. Plant Disease 104. doi.org/10.1094/PDIS-12-19-2709-RE.
- Madden, L.V., and Paul, P.A. (2020). Is disease intensity a good surrogate for yield loss or toxin contamination? A case study with Fusarium head blight of wheat. Phytopathology 110. doi.org/10.1094/PHYTO-11-19-0427-R
- Shannon, D.A., van Santen, E., Salmasi, S.Z., Murray, T.J., Duong, L.T., Greenfield, J.T., Gonzales, T., Foshee, W. (2019). Shade, Establishment Method, and Varietal Effects on Rhizome Yield and Curcumin Content in Turmeric in Alabama. Crop Science, 59(6), 2701-2710. doi:10.2135/cropsci2019.04.0262.
- 24. Hansen S. M., J. Gunnell. A. Whaley, X. Dai, C. Harding and B. L. Black (2020). Adaptability of Tree Species as Windbreaks for Urban Farms in the U.S. Intermountain West. Horticulturae 2020, 6(1), 17-25.
- Purdum, S., Eusebio, P., Hanford, K. (2020). The effects of two genetic lines on spatial distribution and use and preference of perch and nest area in an aviary system. Poultry Science. https://doi.org/10.1016/j.psj.2020.03.056
- Kramer M., Y. Guo, M. Pooler. (2020). Ranking Resistance of Buxus Cultivars to Boxwood Blight An Integrated Analysis. J. Environ. Hort. 38 (2): 50–55.
- Kramer M. (2019). Stats: how useful is the treatment? Nature 569, 192.
- Kramer, MH, Paparozz,i ET, and Stroup, WW. (2019). Best Practices for Presenting Statistical Informationin a Research Article. HortScience 54(9):1605–1609.
- Lockard, Cathy L., Chris J. Richards, Caleb G. Lockard, Maggie Youngers, Mariah A. Woolsoncroft, Taylor C. Husz, Blake K. Wilson, Carla L. Goad, Todd A. Jackson, Douglas L. Step, Bryan C. Bernhard, Marilyn J. Corbin, Clint R. Krehbiel (2019) Growth, performance, and carcass characteristics of feedlot Holstein steers fed ractopamine hydrochloride, Translational Animal Science, Volume 4, Issue 1. https://doi.org/10.1093/tas/txz157
- Fontanier, Charles, Justin Quetone Moss, Lakshmy Gopinath, Carla Goad, Kemin Su, Yanqi Wu (2020). Lipid Composition of Three Bermudagrasses in Response to Chilling Stress, Journal of the American Society of Horticultural Science, DOI: 10.21273/JASHS04815-19.