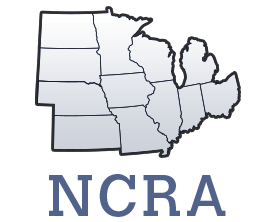
NC_temp1210: Frontiers in On-Farm Experimentation
(Multistate Research Project)
Status: Draft Project
NC_temp1210: Frontiers in On-Farm Experimentation
Duration: 10/01/2025 to 09/30/2030
Administrative Advisor(s):
NIFA Reps:
Non-Technical Summary
The problem NC-1210 addresses is the worldwide, systemic inefficient application of crop production inputs on farm fields, which leads to worldwide economic waste and environmental damage. A principal cause of inefficient crop production management is simple ignorance due to data scarcity. Farmer production strategies, whether developed with the help of public- or private-sector advisors and institutions, as well as government policies aimed at influencing those strategies for social good are currently determined in data-scarce environments. NC-1210’s activities are justified because they are turning that decision-making environment from data-scarce to data-rich, and so leading to new crop production management strategies that enhance the economic and environmental sustainability of commercial crop production.
During NC-1210’s first five years members worked to design and run hundreds of OFPEs, then analyzed and reported the data’s real-world implications for farm management. NC-1210 members worked as part of the Data-Intensive Farm Management Project to develop a cyber-infrastructure to facilitate the expansion of OFPE into use by its target audience, which is made up of private and public-sector agriculture scientists and practitioners (farmers). In its second five-years (2025-2030), NC-1210’s goal will be to facilitate the collaboration of researchers, extension personnel, private-sector crop advisors and farmers, which will result in the running of at least one thousand OFPEs during the time period.
Statement of Issues and Justification
Identification and Significance of a Problem or Opportunity
The problem we propose to address is the worldwide, systemic inefficient application of crop inputs on farm fields. A principal focus will be on the chronic mismanagement of nitrogen fertilizer. Our society manipulates the nitrogen (N) cycle to great benefit, but chronic inefficient use of nitrogen fertilizer has led to the hypoxic “dead zone” in the Gulf of Mexico and the leaching of nitrates into groundwater. The National Academy of Engineering (2012) has declared managing the nitrogen cycle a “Grand Challenge,” and the N cycle has been labeled a “planetary boundary that has been transgressed” (Rockström, et al. 2009). But currently agricultural science is far from understanding the processes determining crop yields, and therefore from discovering how efficient input management practices vary over time and space. Reimer, et al. (2017, p. 6A) described the problem well:
The incredible complexities of the biophysical systems alone are still not well understood by researchers, advisors, or farmers. There are a myriad of variables involved in a decision about how much N to apply in a given growing season … This makes providing reliable recommendations inherently difficult, both for researchers trying to distill complex science into useable information and for advisors trying to craft recommendations for individual farms and fields. (Reimer, et al., 2017, p. 6A)
Unless scientists can gain a better understanding of these complexities, farmers will continue applying fertilizer in ways that send chemicals that everyone wants to go into crops actually go down rivers. The objective of the proposed project is to develop a research infrastructure and encourage the development of a commercial infrastructure that will generate the data needed for this better understanding. If successful, the research will increase farm income and enhance the quality of the nations’ waters.
Of late, great hope has been placed in using the components of digital agriculture, including precision agriculturetechnology, agricultural Big Data, remote and proximal sensing, and on-farm experimentation to increase farm management efficiency. Schlam (2019) offered a typical quote:
The use of analytics will be crucial to enabling smarter agriculture, with farmers leveraging data on soil, local diseases and pests, climate, and other environmental factors to optimize yields and seed selection. ... Soil sensors, aerial drones, GPS-enabled tractors, and more will generate the data upon which analytics solutions will rely … .
But, however impressive these technologies and techniques are individually, and despite public and commercial enthusiasm about them, academic agriculturists looking to the future are expressing frustration with the current state of their use, and have recognized a need to bring them together systematically:
Digital agriculture … has been trying to attract customers before the ecosystem has been properly constructed. What we believe is missing is a standardized way to gather and interpret data, and then translate actionable insights to commercial users—insights which then, in turn, can deliver value to growers. (Zuckerberg, 2017)
In the following, we propose an integrated project to drive the creation of the system Zuckerberg calls for above. We will argue that the key impediment to the development of digital agriculture is lack of information about crop responses to factors of production, but that components of digital agriculture can be used to generate that same information.
A Conceptual Framework and the Fundamental Question
We conceptualize the problem of crop input management in terms of four types of variables. The first variable, y, is crop yield. The second type we represent by a multi-element vector c, of unmanaged, spatially distributed “field characteristics.” The third is a multi-element vector z, of unmanaged and temporally stochastic variables (principally, weather). The fourth is a multi-element vector x of “managed input variables,” (e.g., fertilizer application rate, seeding rate). We conceptualize yield, y, as resulting from a natural process described by a function f, which depends on farmer choices, field characteristics, and weather: y = f(x, c, z).
The fundamental research question for crop input management is, “What is f?” Agricultural scientists have been attempting to generate data to estimate ffor major row crops for almost two hundred years (Odell, et al. 1984). Indeed, estimating f was motivated the advance of modern statistical theory, as R.A. Fischer (1935) developed his pioneering statistical research on randomization in experimental design to address estimating f using data from agronomic experiments (Antle 2019). The extraordinary efforts to learn about f have been made because f reveals how crop yields respond to producer choices, and how yield responses change with growing conditions. Better estimates of f are key to better scientific support of farm management and all the social benefits that follow. Figure 1 provides the simplest of illustrations. Versions of figure 1 and explanations of the implications for producer management choices appear in most introductory microeconomics textbooks (e.g., Pindyck and Rubinfeld 2013, pp. 204-208), and are typically presented in the first few weeks of introductory microeconomics courses. It is assumed in figure 1 that there is only one managed input, x. The characteristics of a site A on which the crop is grown are cA. For simplicity, it is assumed that when a value of x is chosen, weather is assumed known and given as the vector of constant values z2019. The input price and output price are assumed constant at levels w and p. Elementary calculus shows that the profit-maximizing input application rate, x*A,19(p, w), is that value at which the slope of the yield response curve is equal to the price ratio, w/p. When multiple inputs are chosen, similar mathematical rules apply, optimizing over more than two dimensions. Space limitations prevent us from illustrating more complicated situations.
Technical Feasibility of the Work
The focus of the proposed project is on-farm precision experimentation (OFPE) and the analysis of OFPE data, which are illustrated in figure 2. The first panel of figure 2 shows the randomized design of a nitrogen rate OFPE on corn. In form, the design is like those of traditional small-plot trials, but covers a much wider area. The field in figure 2 is 37 ha in size. The second panel shows the trial being “put in the ground” using variable input rate application is accomplished by using GPS-based computer software to pre-program a variable application rate “plan” into a computer aboard farm machinery. That program “instructs” application equipment to apply inputs at the planned rates on the designated plots as the farmer just drives the farm equipment through the field in the usual manner. The final figure shows the resultant data. Led by PI David Bullock, the Data-Intensive Farm Management (DIFM) project has now worked with participating farmers to conduct over one hundred large-scale OFPEs in eight US states, Argentina, Brazil, and South Africa. Trials have been run on cotton, corn, soybeans, and wheat. DIFM has collected, processed, and analyzed the data, communicated the analytical results to the participating farmers, and published journal articles describing the techniques and results. The basic elements of OFPE research, then, are clearly feasible. Those techniques inexpensively provide excellent data, and one very legitimate aim of the proposed multistate project will simply be to pass the proven techniques to scientists currently unfamiliar with them, so that they might work with farmers to conduct OFPEs. But the project goals are much more ambitious than simply teaching other researchers OFPE techniques. The aim is to build a research infrastructure and encourage the development of a commercial infrastructure that will permit tens of thousands of OFPEs to be designed and conducted farmers and their crop consultants annually, as well as the handling, processing, and analysis of the resulting data. The system would annually generate vastly more field trial data than has been generated since the first agronomic field trials were conducted in the first half of the nineteenth century.
The main elements of the strategy to scale up data generation involve the creation of a cloud-based, “on-farm precision experiment design” software system. That system will allow crop consultants, who have received training from Extension personnel or others, to upload basic information about a farmer’s field, such as a geo-referenced file of the field’s border, and the sizes of the farmer’s machinery, and then, with some “pointing and clicking,” design a statistically legitimate agronomic experiment over the farmer’s entire field. All data would be transferred wireless to and from farm machinery. Writers of this proposal have already developed parts of this automated trial design system, and are confident that developing the rest will take hard work, but is feasible.
The principal element of scaling up OFPE data analysis will be an automated “analytical engine,” which can import OFPE data, and then with minimal “human-in-the-loop” effort, employ econometric analysis and a variety of machine learning methods to develop management recommendations. For example, methods based on reinforcement learning can be used to optimize prescriptions for seeding or applying fertilizers or herbicides. Learning algorithms such as those based on deep learning (e.g., convolutional networks or long short-term memories), locally (spatial or temporal) random forests, or Gaussian processes can be used to capture spatial and temporal properties in fields. Other techniques based on a variety of heuristics (local search) and metaheuristics (evolutionary and swarm-based search) in constraint satisfaction, Markov random fields, and even natural language models to describe agricultural processes can be applied to derive data-based farm management support.
The statistical and machine learning models will be accompanied by responsive, interactive statistical visualizations to explain which data have the most impact on the predictions (the “why” of the statistical models), helping build the trust of farmers and consultants in the “black box” models used for prediction. In addition, OFPE data will be used to improve the calibrations of existing crop growth models, which in turn can be used to model yield response and estimate optimal management strategies. Automating OFPE data analysis will be a major challenge. We envision a future in which significant parts of crop science and agricultural economics academia devote themselves to this very task. But it will be feasible, and fascinating, to make a convincing start to this research endeavor in the five years of the proposed project.
Automating the communication of the implications of a farm’s OFPE data will involve the creation of a cloud-based “decision tool” software system, which displays in user-friendly, visual ways, OFPE data results and implications. Several such “decision tools” are currently available on commercial markets. Several of the 135 interviews of farmers, professional crop consultants, and extension personnel conducted by two of the writers of this proposal (Montesdeoca, et al. 2018) made clear that many farmers and consultants have limited faith in their effectiveness. The crop science and agricultural economics literature impels skepticism of current commercial decision tool software packages. They are based on long debunked yield-based input application algorithms (Rodriguez, et al. 2019) and crop growth models with parameters that in general cannot be well enough calibrated to allow the models to provide adequate management advice (Antle 2018).
The DIFM project has proved that conducting trials and analyzing the data is feasible. We believe that the scaling-up of that work is also feasible within the five-year timeline. But, certainly, the project faces risks. Data analysis is difficult, and to develop automated techniques for it that work well enough to provide farmers with profitable management recommendations will be a challenge. But this is why a multistate project is needed--we plan to put dozens of very skilled, highly talented researchers to the tasks at hand, for five years. We are confident that we can develop a workable infrastructure by then, and that the next generation of agronomic science and farm management rely upon, and continue to advance that infrastructure.
The Importance of the Work, and Consequences of It not Being Done
“Site-specific” agriculture technology appeared on the market in the 1990s. But, as Reimer describes in the quote above, very little about the yield response process is known. As a result, little is known about how to use precision agriculture technology well. Farmers complain that they do not know what to do with their data. In truth, in many ways neither does anyone else. If the proposed work is not done, the ignorance that Reimer describes will continue for longer than necessary, and farmers will continue having to make input application decisions that depress their incomes and are bad for the environment.
Evidence for Stakeholder Identification
Evidence for stakeholder identification of the need for the proposed project’s outputs was provided in abundance during the 135 interviews of farmers, professional crop consultants, and extension personnel, mentioned above. That study concluded that many farmers are already experimenters. Certified Crop Advisors (CCAs) and farmers told us repeatedly that they were placing various types of informal “test plots” in their fields, to see how alternative management strategies fared against their actual strategies. But CCAs also told us that carrying out field trials was a great deal of work, and that they realized that the statistical designs of their trials were technically flawed.
Another principal conclusion from the interviews is that many farmers are frustrated that they now possess multitudes of data that they do not know how to analyze. A few representative quotes follow:
“Once you get all this data, you need to hire someone to analyze it. If you can trust them. … Lots of people are just trying to sell you something.” -western Illinois corn grower
“Our seed rates? Pretty much a shot in the dark.” -three south Texas cotton growers
“It would be wonderful if my decision-making were more analytical instead of just off-the-hip SWAG (‘Scientific Wild-A** Guesses’)!” –northern Illinois corn grower
The interviews also showed that neither farmers nor their CCAs want “black-box” management recommendations from decision tool. They want intuitive agronomic explanations of how field characteristics determine the responses of yields to managed variables.
The Advantages of Doing the Work as a Multistate Effort
The proposed project’s collaborators come from all over the US. Including multiple geographically dispersed universities allows trials in crops from different regions: maize, soybeans, white winter wheat, red spring wheat, barley, cotton, canola, sugarcane, and sorghum, under varied topography, soil characteristics, and climates. The multiple-university team of researchers and extension personnel is comprised of personnel with significant experience already working together, and its professionals are capable of providing the considerable needed expertise.
Likely Impacts of Successfully Completing the Work
The proposed project’s principal goal is ambitious: we want to revolutionize agronomic research and how the information from that research is transferred to those who actually make farming decisions. It will make farmers central to the research process. The data will allow crop consultants to provide farmers management advice derived from data from the same fields for which that advice needs to be applied, instead of data conducted by researchers somewhere else. Farmers will improve their input management strategies, basing them on data, not speculation. Farm income will be increased, and environmental damage from agricultural chemicals will decrease. In addition, the commercial crop consultancy industry will change, providing advantages to those who understand experimental design and statistics. It will attract highly skilled workers into rural areas, which will have multiple positive social and economic impacts in those regions.
Proposed Project Members
Bullock, David
dsbulloc@illinois.edu
Boerngen, Maria
maboern@ilstu.edu
Hawkins, Elizabeth
hawkins.301@osu.edu
Griffin, Terry
twgriffin@ksu.edu
Vanderplas, Susan
svanderplas2@unl.edu
Jung, Jinha
jinha@purdue.edu
Miao, Yuxin
ymiao@umn.edu
Chowdhary, Girish
girishc@illinois.edu
Miguez, Fernando
femiguez@iastate.edu
Mitchell, Paul D
pdmitchell@wisc.edu
Brorsen, Wade
wade.brorsen@okstate.edu
Ashworth, Amanda
Amanda.Ashworth@ars.usda.gov
Kharel, Tulsi
Tulsi.Kharel@usda.gov
Li, Xiaofei
xiaofei.li@msstate.edu
Ketterings, Quirine
qmk2@cornell.edu
Sheppard, John
john.sheppard@montana.edu
Daccache, Andre
adaccache@ucdavis.edu
Sun, Xin
xin.sun@ndsu.edu
Dhillon, Jagmandeep
jagman.dhillon@msstate.edu
Allen, Cody
allencm@illinois.edu
Baath, Gurjinder
gurjinder.baath@ag.tamu.edu
Reed, Vaughn
vr401@msstate.edu
Guo, Wenxuan
wenxuan.guo@ag.tamu.edu
Tao, Haiying
haiying.tao@uconn.edu
Froes de Borja Reis, Andre
areis@missouri.edu
Mieno, Taro
tmieno2@unl.edu
Longchamps, Louis
ll928@cornell.edu
Jha, Gaurav
gjha@ksu.edu
Ransom, Curtis
Curtis.Ransom@usda.gov
Enciso, Juan
Juan.Enciso@ag.tamu.edu
Shajahan, Sunoj
sunoj@illinois.edu
Nugent, Paul
paul.nugent@montana.edu
Sellars, Sarah
sarah.sellars@sdstate.edu
Kaur, Upinder
kauru@purdue.edu
Bagavathiannan, Muthukumar
muthu@tamu.edu
Proulx, Rob
rob.proulx@ndsu.edu
Mizuta, Katsutoshi
toshi.m@uky.edu
Pinto, Ricardo
ricardo.pinto@montana.edu
Yost, Matt
matt.yost@usu.edu
Ghatrehsamani, Shirin
spg5994@psu.edu
Becker, Talon
tbecker2@illinois.edu
Diss, Meagan
mcdiss@illinois.edu
Jones, John D.
jones86@illinois.edu
Kumar, Hemendra
hemendra@umd.edu
Mendes Bastos, Leonardo
lmbastos@uga.edu
Related, Current and Previous Work
The following is a list of the major accomplishment of the NC-1210 project during its first five years:
- Wrote and submitted a grant proposal to the NRCS Conservation Innovation Grant On-Farm Trials (CIGOFT) project, and was awarded $4,000,000.
- Used CIGOFT funding to develop the Data-Intensive Farm Management Project’s Cyber-Infrastructure, which can be freely accessed at https://difm.farm , and can be used to do the following in a “user-friendly” environment:
- create trial design files that can be loaded into variable rate application machinery to put the field trial designs “in the ground”;
- upload as-applied input data, yield data, and other spatial data into a well organized, secure database on the Oracle cloud, where is can be processed and managed efficiently;
- conduct statistic and machine-learning analyses of the practical implementation of the data for farm management; and
- write reports that present the agronomic and economic implications of the data analysis.
- Ran over 200 on-farm precision experiments in the following states: Idaho, Illinois, Indiana, Iowa, Kansas, Minnesota, Missouri, Montana, Nebraska, North Dakota, Ohio, Oklahoma, Oregon, South Dakota, Texas, and Washington.
- Wrote numerous academic journal articles and presented scores of presentations, a list of which can found attached.
- Won a NIFA Conference Grant funding to put on the 2024 International Conference for On-Farm Precision Experimentation, which was attended in-person by over 90 farmers, crop consultants, and professional research. The main accomplishment of the conference was to provider training in the use of early versions of the difm.farm cyber-infrastructure.
- Wrote and submitted a grant proposal to the NIFA-AFRI Competitive Grants Program’s Education and Workforce Development Program. Funding is being used to organize and run a class on on-farm precision experimentation, which is being attended by students at seven cooperating community colleges. $750,000.
- Wrote and submitted a grant proposal to the Illinois Nutrient Research and Education Council. Project uses OFPE to run “checkerboard” cover-crop trials. $670,000.
- Organized the 2025 “Opportunities for Extension in On-farm Precision Experimentation.” Approximately 60 university Extension faculty and specialists have registered and will attend in-person in January 2025 to receive training in the use of the difm.farm cyber-infrastructure.
Further investigation is needed in numerous branches of OFPE. The cyber-infrastructure needs to be advanced to be more flexible in its data analysis algorithms. Given that the difm.farm cyber-infrastructure is up and running, it should be possible to conduct and analyze at least a thousand OFPEs over the five years of the project, and all the data thus produced will need to be analyzed and reported on.
Related Multistate Projects
In the following, we present short comparisons between the proposed project and the active multistate projects that have key elements in common with those of the proposed project. Should the proposed project be funded, there will be no more than minimal duplication of the efforts of existing projects.
NCERA 180: Precision Agriculture for Technologies for Food, Fiber, and Energy Production
The NCERA 180 projects states that its principle expected outcome “is an improvement in the understanding of how precision and prescriptive agricultural brings value to the farm and agriculture overall.” The proposed multistate project will focus itself on a narrower topic than does form NCERA 180, examining not so much on the use of precision technology “overall,” but rather more narrowly focusing on the idea of using precision technology to generate information about yield response functions. We believe that the principal bottleneck to progress in precision agriculture is ignorance about yield response functions. The proposed project will focus not so much on what is currently working well in digital agriculture, but rather on fixing what is working poorly.
S1090: AI in Agroecosystems: Big Data and Smart Technology-Driven Sustainable Production
The S1090 project and NC1210 both involve the use of artificial intelligence to increase the sustainability of crop production. But NC1210’s use of AI is more narrowly focused. NC1210 uses AI to analyze data from on-farm precision experiments. S1090 uses AI far more generally, examining issues in animal health, robotics systems, the scouting and monitoring of natural resources, plant phenotyping and genotyping.
S1098: Autonomy for Agricultural Production, Processing, and Research to Advance Food Security through Sustainable and Climate-Smart Methods
The S1098 project focuses on autonomous systems, such as self-driving farm machinery. This topic is tangentially related to NC1210 in that both project seek to guide producers in implementing more sustainable crop production methods. But the projects’ basic methods, autonomous machinery and on-farm research, are quite different.
SERA46: Framework for Nutrient Reduction Strategy Collaboration: The Role of Land Grant Universities
NC1210 shares certain goals with SERA46, which is to encourage the development of more efficient fertilizer management strategies. But, the proposed project focuses on the narrower topic of yield response function estimation, and will apply to a broader geographical region than the twelve-states in the Mississippi River Basin that are developing ERA-requested Nutrient Loss Reduction Strategies.
NCCC_temp211: Cover Crops to Improve Agricultural Sustainability and Environmental Quality in the Upper Midwest
NC1210 plans to conduct significant research in cover crop on-farm precision experimentation. NCCC_temp211 is also conducting field research on cover crops. But the methodologies of the two projects are quite different. NC1210 will be conducting multi-state, multi-year, multi-field large-scale on-farm precision experiments, and run them under common protocols using the difm.farm cyber-infrastructure. NCCC_temp211 works on a much broader spectrum of field trials, with members designing and running generally smaller strip trials under differing protocols. Also, NC1210 will be running cover crop trials over a wider geographic area than is covered by the five states in which NCCC_temp211 trials will be run.
S1085: Cover Crops for Sustainable Southern Agroecosystems
NC1210 plans to conduct significant research in cover crop on-farm precision experimentation. S1085 is also conducting field research on cover crops. But the methodologies of the two projects are quite different. NC1210 will be conducting multi-state, multi-year, multi-field large-scale on-farm precision experiments, and run them under common protocols using the difm.farm cyber-infrastructure. S1085 works on a much broader spectrum of field trials, with members designing and running generally smaller strip trials under differing protocols. Also, NC1210 will be running cover crop trials over a wider geographic area than is covered by the S1085 trials.
Other Related Previous and Current Work
Several on-farm research networks are currently active in the US. Of these, only the Data-Intensive Farm Management project is conducting OFPEs. But other networks are currently running other kinds of field trials with participating farmers, with whom they have developed longer-term relationships. These relationships develop only over time, and therefore the other on-farm research networks are well situated to begin running OFPEs. Researchers and Extension personnel from DIFM and five other on-farm research networks will participate in the proposed multistate project, as described below. Having representatives from these different networks and projects at the same meetings on a regular basis will help them establish common trial design, data processing, and data analysis protocols, discuss challenges in OFPE research, and move OFPE research and outreach activities as a whole, and in common directions.
The Data-Intensive Farm Management Project
The NIFA-funded Data-Intensive Farm Management project has lain much of the foundation for the proposed project. Bullock, et al. (2019), describe that project in detail, summarized here. DIFM works with farmers, using precision technology to inexpensively design and conduct OFPEs that provide data-based, site-specific management guidance for farm inputs. DIFM’s data and the agricultural “Big Data” currently being collected with remote and proximal sensors are complementary; that is, more of each increases the value of the other. In 2019, DIFM and affiliates are conducting over one hundred OFPEs, ranging from 10 to 100 ha in size, on maize, wheat, soybeans, cotton, and barley in eight US states, Argentina, Brazil, and South Africa. DIFM has developed the beginnings of an OFPE cyberinfrastructure, which must be “scaled up” to permit researchers and crop consultants worldwide to work with farmers to conduct trials, then process and manage the data. In addition, DIFM is in the early stages of developing a software system for semi-automatic data analytics, and a cloud-based farm management aid, the purpose of which is to facilitate conversations between crop consultants and their farmer-clients about implementing data-driven input management decisions.
On-farm Experimentation Networks and Projects
On-farm experimentation (though not necessarily on-farm precision experimentation) is currently being organized and conducted by at several U.S. universities. The Nebraska On-Farm Research Network (NOFRN) is a statewide program that addresses critical farmer production, profitability and natural resource questions. Growers take an active role in the project, which is sponsored by Nebraska Extension in partnership with the Nebraska Corn Growers Association, Nebraska Corn Board, Nebraska Soybean Checkoff and Nebraska Dry Bean Commission. (Nebraska On-Farm Research Network 2019). Farmers have conducted research as part of the NOFRN since 1990; annually 60-100 on-farm research projects are conducted and meetings are held to share the local, reliable research-based information. Among its many on-farm research pursuits, NOFRN has cooperated with DIFM project, working with participating farmers to run “checkboard trials” since 2016. Laura Thompson is a Co-coordinator of NOFRN, and will play an important role in the proposed multistate project.
Ohio State University’s eFields On-Farm Research program (E-Fields On-Farm Research 2019) utilizes modern technologies and information to conduct on-farm studies with an educational and demonstration component used to help farmers and advisors understand how new practices and techniques can improve farm efficiency and profitability. The program is also dedicated to delivering timely and relevant, data-driven, actionable information. Current projects are focused on precision nutrient management strategies and technologies to improve efficiency of fertilizer placement, enable on-farm evaluation, automate machine functionality, enhance placement of pesticides and seed, and to develop analytical tools for digital agriculture. Though eFields does not currently conduct OFPE research, it has the institutional backing along with the experienced research and extension personnel to do so, having run ninety-five on-farm, full-field strip trials in 2019. Ag Technologies Extension Field Specialist Dr. Elizabeth Hawkins is the Coordinator of eFields, and will play an important role in the proposed multistate project.
Washington State University’s Farmers Network (WSU Farmers Network 2019) advances soil and nutrient management, crop productivity, farm sustainability and profit through collaborative research, extension and on-farm participatory learning. By directly participating in the research on their own farms, farmers learn first-hand which practices are better choices and how to fine-tune their own nutrient management systems. Along with other research activities, in 2019 WSFN began cooperating with the DIFM project to conduct OFPE research. Dr. Haiying Tao is the Director of WSFN, and will play a key role in the proposed multistate project.
At the University of Wisconsin, Dr. Paul Mitchell is the lead on the NIFA-funded project “Applications of Reinforcement Learning Algorithms to Improve Crop Input Use” (grant: number 2019-67023-29418). He has assembled a multi-disciplinary research team and a small group of cooperating grain and commercial vegetable farmers to field test algorithms developed by project research assistant Yuji Saikai (who will graduate in May 2020). An important focus of their research is to use Bayesian optimization and other machine learning techniques to improve plot placement and other farm management in on-farm field trials (Sakai et al. 2018, Saikai and Mitchell 2019, Saikai et al. 2019).
The Iowa Soybean Association’s On-Farm Network® (Iowa Soybean Association 2019) runs field-length strip trials, including soybean and corn seed rate trials and corn N rate trials. Working with participating farmers, ISA completed over 200 trials in 2018. The ISA strip trials are simpler than typical OFPEs, to help farmers successfully implement trials on their own. Because ISA runs hundreds of trials annually, farmers need to be able to implement them with minimal guidance and less disturbance to their common operations. An important objective of the proposed multistate project is to design software that will simplify and automate the design and implementation of OFPEs, enabling ISA and other organizations to run them on hundreds of farms annually without increasing their costs for trial implementation and technical support.
OFPE Data Analysis Activities
Anselin, et al. (2004) used spatial econometrics to analyze yield monitor data from a full-field N strip trial in Argentina. Their principal result that the estimates of the profitability of site-specific N management depended on model specification, and that spatial econometric methods predicted profitability of site-specific management, whereas non-spatial econometric analysis did not. Ruffo, et al. (2006) estimated yield response functions using data from early OFPEs on eight Illinois cornfields, and analyzed the data using geospatial techniques. They concluded that terrain attributes could be used as surrogates for soil water content, and that results of the Illinois Soil Nitrogen Test could be used to proxy soil mineralizable nitrogen in estimated yield response to nitrogen fertilizer. Bullock, et al. (2009) also used OFPE data from Illinois fields to measure the value of information in nitrogen management. They concluded that, excluding consideration of the costs of precision technology equipment, variable rate nitrogen application would have been profitable on six of the eight fields on which the OFPEs were conducted.
Trevisan, Bullock, and Martín (2019) demonstrated the intriguing potential of geographically weighted regression in the analysis of on-farm precision experiment data. The method proposed revealed the spatial variability in corn response to nitrogen and seed rate in four corn fields in Illinois, USA. The within-field variation in the economically optimum seed and nitrogen rates varied from 10 to 20 kseeds ha-1 and from 20 to 60 kg ha-1, respectively. Applying the knowledge obtained with the OFPE results showed potential guiding profitable input reduction and yield improvement. s, increasing the profitability by an estimated US$ 71.00 ha-1. Whether the greatest benefits would come from adjusting the field’s uniform rate or from accounting for the spatial variability in crop response and implementing variable rate application strategies was field-specific.
Piepho, et al. (2017) review statistical issues arising in the design and analysis of on-farm experiments. They outline basic design principles, and consider both the classical set-up with either a single datum per randomization unit (plot) or subsampled data that are not geo-referenced, as well as the case of within-plot, geo-referenced data as arising in precsion farming. They emphasize the importance of replicating trials in several envi ronments, focusing on the implications for both design and analysis. Two OFPE case studies illustrate the concepts they review.
Kindred, et al. (2017) conduct OFPE experiments to examine variation in N requirements, and to develop and test systems for its prediction and predictability. Results demonstrated substantial within-field variability in fertilizer N requirements, typically greater than150 kg per ha. But only increases in profitability were modest (compared to the uniform average rate).
Activities Involving Development of the Analytical Engine
Considerable progress has been made in the fields of machine learning (especially deep learning) and real-time control (especially in the context of autonomous vehicles). From a computational standpoint, issues related to high-dimensional, noisy, dynamic optimization remain (Duan et al, 2016; Ho & Ermon, 2016). Within the context of precision agriculture in general, and data intensive agriculture in particular, fundamental problems to be addressed in this project range from crop and weed identification to field characterization to yield prediction and beyond. The work proposed here approaches these problems computationally by expanding upon new methods in multi-task reinforcement learning under uncertainty, transfer learning capitalizing on models trained from different fields, and high-dimensional, multi-/many-objective optimization (Li et al, 2009; van Moffaert & Nowe, 2014; Liu et al, 2015; Omishafiei et al., 2017; Devin et al. 2017).
To date, the vast majority of prior work done has either focused on limited experiments over limited time frames and fields, or has attempted to create models aggregating over a variety of field and crop types (Kaul et al., 2005; Panda et al., 2010; You et al., 2017). At the heart of these problems is scalability – how well can we develop methods and algorithms to scale to large feature spaces and large state spaces, especially in the presence of uncertain and dynamically changing environments? Furthermore, how well can we adapt our methods to exploit rather than ignore the unique characteristics of the specific farms and even plots on the farms being managed? Ultimately, the advances in the algorithms developed or expanded here will contribute to the larger goal by being integrated together to enable closed-loop operations in support of effective farm management.
Field and Plant Characterization
Over the past two decades, tremendous have been put into research on measuring and interpreting how field and plant characteristics affect yield response to managed inputs. But because this kind of c data is highly complemented by the x and y data that OFPEs generate, using these data together is far more valuable than using them apart. The proposed multistate project will indeed work with the latest technology for measurement and mapping of field characteristics. But the main part of the research that makes it differ from other projects measuring and mapping field characteristics is that it will combine the use of c data with its x and y data in the analyses.
Objectives
-
General Goal: Create Crop Management Information System
Comments: The general goal of the proposed project is to conduct the research necessary for the creation of a new digital crop management information system, which will increase profitability and sustainability in agriculture through improved productivity and input use efficiency. -
Supporting Objective: Continue Development of On-farm Precision Experimentation Cyber-infrastructure
Comments: Our first supporting objective is to lay the research groundwork needed to continue the development of an on-farm precision experimentation (OFPE) cyber-infrastructure, sufficiently automated to be scaled up to enable 1) the running of tens of thousands of OFPEs per year, worldwide; 2) the collection of field characteristics and weather data on the OFPE fields; 3) the processing and management of the resultant data, which will make it possible to apply advanced statistical and artificial intelligence methodologies to data analysis, thereby 4) providing farmers with management recommendations based on data from their own fields, as well as on data from other fields, worldwide. The resultant increase in input use efficiency will not only 5) increase farm income, but also 6) enhance the nation’s water quality, as more fertilizers enter plants as building blocks to growth and production instead of waterways. -
Supporting Objective: Lay Groundwork for Revolution in Private and Public Sector Crop Production Advising
Comments: The second supporting objective is to build upon the research groundwork discussed above to revolutionize how university Extension services and the private crop-consulting industry give crop management advice to farmers. The cyber-infrastructure developed will enable the generation of vast amounts of on-farm field trial data. The system’s automated data processing and analysis tools will make it possible for those who give professional drop management advice to base that advice on the rigorous analysis of high-quality, high-quantity data. -
Supporting Obj: Develop Cyber-infrastructure Modules that Coordinate Research and Policy Administration
Comments: These additions to the existing cyber-infrastructure will make it possible for government to encourage the organization and coordination of many-farm, multiple-state on-farm research projects and coordinate the administration of government programs that give incentives to farmers to implement sustainable crop production strategies.
Methods
The general goal of the proposed project is to conduct the research necessary for the creation of a new digital crop management information system, which will increase profitability and sustainability in agriculture through improved productivity and input use efficiency.
The principle objective of the proposed multistate project, which is to continue the development and use of an on-farm precision experimentation (OFPE) cyber-infrastructure, sufficiently automated to be scaled up to enable
1) the running of tens of thousands of OFPEs per year, worldwide;
2) the efficient collection of field characteristics and weather data on the OFPE fields;
3) the processing and management of the resultant data, which will make it possible to apply advanced statistical and artificial intelligence methodologies to data analysis, thereby
4) providing farmers with management recommendations based on data from their own fields, as well as on data from other fields, worldwide.
Methods to Allow the Running of Tens of Thousands of OFPEs per Year
Figure 1 (See Attachments) illustrates the current capabilities of the difm.farm cyber-infrastructure. Over the next five years, NC1210-affiliated researchers will continue to develop difm.farm so that it can be used by thousands of farmers to create OFPEs, analyze the data, and obtain management-improving information from the analyses.
Panel (a) depicts the design of an actual field trial recently conducted with a participating farmer on a 81-acre Illinois soybean field. The farmer designed the trial himself, using the tools publicly available at the difm.farm website (https://difm.farm), which was created through the collaboration of NC1210 and the Data-Intensive Farm Management Project (Bullock, et al. 2019). The difm.farm tool provided a shapefile with spatially-specific input application “instructions” to GPS-linked variable rate application equipment to apply an input at different randomized rates on the different plots (grid cells). The trial design shown in figure 1 was comprised of 112 plots, each 43.3 feet wide, between 223 and 250 long, and covering approximately 0.25 acres. The seed was planted with a 60-ft variable rate planter with by-row control, and was harvested with a combine 43.3 feet wide. Most importantly, because the machinery’s actual application rates were changed by an on-board computer and GPS-linked variable rate equipment as the machinery moved through the field, the implementation of the trial was simple and of little bother to the farmer—he simply drove through his field in the usual manner while the trial design file, on-board computer, variable rate planter and GPS “put the trial in the ground.” Yield data was gathered at harvest, using a GPS-linked yield monitor. As is shown in the right-hand panel of figure 1, this new experimental design and implementation technique allows very labor-extensive, inexpensive generation of large amounts of input and output data. The farmer then was able to use difm.farm to, at the “push of a button,” store, manage, process and analyze the data. difm.farm then created a report about the management implications of the data analysis. All of the panels of figure 1 we created within that report.
NC1210-affiliated researchers will continue to develop the difm.farm cyber-infrastructure, with the aim of allowing it to be useful for thousands of farmers every year. Running thousands of trials annually will require NC1210 to greatly expand its work with both public-sector extension personnel, private-sector certified crop advisors, farm management consultants at agricultural coops, and farm managers and farmers themselves. NC1210 will seek funding from various sources to hold virtual and in-person meetings, conferences, and training sessions where members of all of these groups will learn how to use the difm.farm cyber-infrastructure. A great deal of this work will be done by NC1210-affiliated extension personnel. They will create online training videos and virtual training sessions, continually promoting use of the cyber-infrastructure. NC1210 members will also create within the difm.farm cyber-infrastructure a way for members of the “OFPE community” to continually communicate with each other. “Ask the Expert” sessions and “Troubleshooting” sessions will be held at least monthly.
While promoting use of the difm.farm cyber-infrastructure will be necessary, continuing to the develop the cyber-infrastructure to handle thousands of trials per year will require a great deal of computer coding, database infrastructure development, and software user interface development. NC1210 members are already working to write grant proposals to fund the salaries of professionals who can work with NC1210 researchers to carry out these developments.
Increase Efficiency in Generating and Processing Data on Field and Plant Characteristics
Great efforts are currently being made by researchers in academic and commercial settings to generate characteristics data: drones, robots, satellites, and many kinds of proximal sensors have been or are being created and used. The current situation has a major shortcoming: while there exists a huge academic literature on how to use characteristics data to establish field “management zones” (there is very little discussion on how to manage said management zones. The multistate project will focus on not just gathering characteristics data, but learning if and how that data is most useful. Members will also work together to developing a common database structure, creating methods of cleaning, processing, and then “stacking and packing” the spatial characteristics data into common resolutions. The multistate project will organize the collection of the following types of field and plant characteristics data:
Electroconductivity Data
Efficient and accurate methods of measuring spatial variations in soil properties are important for precision agriculture and digital soil mapping. Mobile sensor systems that can collect dense datasets in situ provide several advantages over traditional measurement methods that involve soil sample collection, removal from the field, and lab analysis. Apparent soil electrical conductivity (EC), a widely used proximal soil sensing technology, is related to several important soil properties, including salinity, clay content, and bulk density (Suddoth, et al., 2013). Soil EC can serve as a proxy for soil physical properties such as organic matter (Jaynes, et al., 1994), clay content (Williams and Hoey, 1987), and cation exchange capacity (McBride, et al., 1990). These properties have a significant effect on water and nutrient-holding capacity, which are major drivers of yield (Jaynes, et al., 1995). The relationship between soil EC and yield has been reported and quantified by others (Kitchen, et al., 1996; Fleming, et al., 1998). The proposed multistate project will encourage the collection of EC data by all members conducting OFPEs. Discussions will be held about how to process and store that data under common protocols. How EC data can be used to guide management decisions will be a frequent topic of research and conversation.
Data from Unmanned Aircraft Systems.
There has been a pressing need to advance field-based plant phenotyping capabilities to match currently available genotyping tools. Recent advances in UAS (Unmanned Aircraft Systems) and sensor technology have made it possible to accurately assess overall crop growth and health status with fine spatial and high temporal resolutions previously unobtainable from traditional remote sensing platforms. These advanced platforms enable fast and accurate data collection throughout the growing season. UAS-based remote sensing technologies are becoming an important tool for agriculture scientists (Awika et al., 2019; Jung et al., 2018) and growers (Anderson et al., 2019; Pugh et al., 2018; Enciso et al., 2018) as they provide the capability to efficiently evaluate the performance of many experimental treatments under field conditions (Akash et al., 2019; Yeom et al., 2018; Pugh et al., 2017; Chang et al., 2017) at a relatively low cost. UAS based remote sensing platforms are expected to revolutionize on-farm research by providing means to collect fine spatial and high temporal resolution data. The data will be used to decipher complex interactions between genotypes and environments at field scales, which will eventually result in developing best farm management practices to increase input management efficiency. The proposed multistate project will encourage the collection of UAS data by all members conducting OFPEs. Discussions will be held about how to process and store that data under common protocols. How UAS data can be used to guide management decisions will be a frequent topic of research and conversation
Data from Robots
Collectively measuring different aspects of the plant, soil, and the surrounding microclimate is the key challenge facing scientists working to improve the yield and sustainability of productive agroforestry systems. Field experiments are necessary to advance both plant breeding and agricultural production systems research because it is well known that plants grow very differently inside greenhouses. However, scientists must account for the variability in the field environment to ensure that the results from their field experiments are statistically significant. The inability to collectively measure data that can capture this variability has led to the so-called phenotyping bottleneck: The inability to accurately understand the relationship between crop performance and crop genetics as a function of the plant’s local environment. This critical gap is significantly hampering agroforestry productivity by limiting yield potential, delaying detection of stressors, and precluding accurate predictions. But robotics is beginning to bridge said gap enabling plant phenotyping, including stem counts using side facing RGB cameras, plant height using vertically oriented LiDAR, plant width using RGB and side-facing LiDAR, and Leaf-Area index using vertically facing fish-eye cameras. A machine learning pipeline that could correlate visual data with data from other sensors could be developed. The proposed multistate project will encourage the collection of “agbot” data by all members conducting OFPEs. Discussions will be held about how to process and store that data under common protocols. How agbot data can be used to guide management decisions will be a frequent topic of research and conversation.
Publicly Available Remote Sensing Data
A great deal of remote sensing data is currently available, free of charge, for the purposes of academic research: Sentinel 2 satellite data (European Space Agency 2019) (in particular, vegetative index data that is now frequently used by agricultural scientists attempting to identify precision agricultural “management zones” (Mulla 2013)), LiDAR digital elevation model data (United States Geographic Survey 2019), USGS-POLARIS probabilistic soil data (Chaney et al. 2016), and WSS SSURGO soil-type data (United States Department of Agriculture 2019). Combining this publicly available agricultural “Big Data” with the (x, y) data from OFPEs increases the value of both types of data, leading to greater understanding of why yield responds differently to managed inputs at different locations. Without (x, y), c, and zdata, the yield response y = f(x,c,z) cannot be estimated, and this estimation is the key to data-intensive farm management. Discussions will be held about how to process and store this publicly available remote sensing data under common protocols. How said data can be used to guide management decisions will be a frequent topic of research and conversation.
Establish Consistent Protocols
Especially in the early years of the proposed multistate project, it will also be important to discuss and use common OFPE protocols, such as establishing consistent data variable names and units, and consistency (though not necessarily complete uniformity) in trials design, data cleaning and processing, and spatial data resolutions. The proposed multistate project will not aim to make all the resultant research data available to all participants for analysis. But it will aim to build a highly secure, password-protected common dataset, with which researchers who generate data can grant access to that data to others, if desired. Creating such a dataset, with agreed on units, variable names, and protocols will greatly increase research efficiency over the long-run by allowing new OFPE projects to go from raw data collection to cleaned data analysis in relatively simple, well-established steps, instead of having to independently solve the same data cleaning and management problems that earlier OFPE projects have investigated and dealt with.
Enhance Soil-Crop Model Performance and Utility.
Process-based models offer a way to understand the underlying crop and soil processes driving yield and environmental outcomes, but this comes at the cost of extensive input data requirements (Basso et al., 2012; Puntel et al., 2018, refs). Successful yield forecasting approaches using crop models (Morell et al., 2016; Togliatti et al., 2017: Carberry et al., 2009) have shown potential to couple explanatory with predictive power to evaluate and deliver adaptive management strategies (Jones et al., 2017). We will enhance soil-crop model performance and utility within a cyber-infrastructure by using field-specific information, derived from the OFPEs, that generate high-resolution spatio-temporal data on crop response to field conditions and management. Although the algorithms behind crop models continue to be refined to better represent biophysical processes, knowledge gaps still limit their use (Rötter et al., 2018; Li et al., 2019). Continuous development and testing of field-scale agronomic crop models with OFPE data can therefore improve our understanding of fundamental science, identify gaps in model function, and improve current predictive methods to better account for extreme events (Peng et al., 2018). This research will use the Adapt-N and APSIM models, which are deterministic-stochastic models that simulate nitrogen in the soil-crop continuum. Adapt-N (Melkonian et al. 2008) was launched in 2008 and is already used by producers and researchers in commercial settings in the US and Canada, and APSIM (the Agricultural Production Systems sIMulator; Holzworth et al., 2014)) has been widely used by researchers in numerous countries and commercial applications (Yield Prophet). Both models have been extensively validated in controlled trials, including long-term studies, and on-farm strip trials (Sogbedji et al., 2006; Marjerison et al., 2016; Sela et al., 2017; Puntel et al., 2016).
Start up and Push forward a Literature on Statistical Analysis of OFPE Data.
There are many methods of regression analysis that might be applied to OFPE data to learn about yield response. Without doubt, an active literature will come about over coming decades examining and debating about which statistical methods work best in which situations. One important activity or the proposed product will be to start up that research, and that research discussion. We envision project meetings to feature presentations of statistical research, and discussions about that research. That research will eventually go into journals articles, and create and press forward a new lite4rature on statistical analysis and OFPEs. As more is learned about which analytical methods work best in which situations, it will be easier to design more automated statistical analysis techniques, which will be the primary inputs used for private companies and/or university research to begin to develop automated research analysis software.
Start up and Push forward a Literature on Machine Learning and OFPE Design
Machine learning is a broad term covering many types of algorithms (Coble et al. 2018), but at this time, their application to agricultural production contexts for decision making still remains rudimentary (Mishra et al. 2016; Shine et al. 2018; Sun et al. 2017; Ip et al. 2018). One impediment to the conduct of OFPEs is that is that they may involve short-run profit losses from either yield losses from substantially low input use for some treatments, and high costs for substantially high input use for others. Machine learning algorithms offer the opportunity to increase the efficiency of on-farm experimentation by targeting placement of “plots” within fields based on grid/zone sampling data. The traditional randomization and replication used for placement of small plot experiments is essentially a random “brute force” approach to identify the production function, while a more targeted approach can acquire the same information with fewer plots (Saikai and Mitchell 2018). Fewer plots would further reduce the cost of on-farm experimentation. Automated, easy-to-use, and low-cost systems are key to achieving wider adoption of on-farm experimentation to locally optimize crop management (Griffin 2018). In the proposed multistate project, researchers specializing in machine learning will work together to improve the efficiency of OFPE trial designs, through discussion, collaboration, and critique of each other’s reported research
Measurement of Progress and Results
Outputs
- Data Comments: Data. The proposed project will generate field trial data from thousands of OFPEs. The raw data from a typical OFPE will include tens of thousands of “as-applied”, and/or “as-planted” observations, and tens of thousands of yield observations. Those OFPEs will be designed to estimate yield response to inputs and other factors of production, including one or more of the following: randomized or stratified seed rate, N fertilizer rate, and P fertilizer rate data, for several crops, including corn, soy, cotton, barley, and various kinds of wheat. The proposed project will also help continue the development of the cyber-infrastructure needed to gather, process, and “stack and pack” many kinds of field characteristics data. The success of the data generation will be measured in numbers of OFPEs conducted and analyzed. The project goal is to generate data from 1500 OFPEs to be run over five years.
- Continued development of cyber-infrastructure Comments: The Intellectual Foundation for a Looped OFPE Cyber-infrastructure. The proposed project will provide the intellectual foundation needed for the continued development of the looped OFPE infrastructure shown in figure 3 (see Attachments). This foundation will facilitate the development of the infrastructure itself both in academia and in the private sector. The infrastructure will be made up of the following components, which will be either direct outputs of the project, or outputs developed in the private sector, but dependent on the proposed project’s research. Success in generating this infrastructure will be measured by numbers of consultants and farmers that use it to design OFPEs and develop data-intensive management strategies. The project goal after five years is for 400 farmers to be working with their consultants to manage their farmers data-intensively, using OFPEs. Component A: “Front End” Portal. Panel A in Figure 3 depicts a farmer and a consultant working at a laptop computer. Farmers and their consultants will have access, through a user-friendly “front end” portal, to a cloud-based software system that bookends the infrastructure’s loop. Component B: An Automated OFPE System. The portal will provide access to OFPE design software, allowing consultants/farmers to design and implement statistically sound field trials. For each field in an OFPE, the system will request basic information from the farmer/consultant, and use it to build a trial design, in the form of a polygonized geospatial file, assigning randomized input application rates to the “plots” of the trial design. Component C: Weather Data System. The proposed project will provide the intellectual foundation for a data system to store and manipulate daily weather data using the DAYMET platform. Component D: Characteristics Data System The cyber-infrastructure will provide a secure, centralized data system, in which researchers, consultants, and clients can store, access, and manipulate their OFPE characteristics data in proven, efficient ways. That data will be encrypted, and only accessible to those who own it. Component E: Automated Data Processing, Analysis and Management Recommendation System To scale up use of the cyber-infrastructure, the project will lay the intellectual foundation for the continued development of a (mostly) automated analytical engine, to provide practical, profitable management advice and data interpretation to farmers and their consultants through the front-end decision tool. Component F: Decision Tool. Panel F of figure 3 shows the end of the cyber-infrastructure’s loop, where the user interface gives the farmer/consultant access to input management “decision tool” software. Many commercial agricultural enterprises currently offer decision-tool software, but their effectiveness could be greatly enhanced by the development of the OFPE infrastructure. Component G: OFPE Community Discussion Forum. Area G of figure 3 shows how the web-based OFPE forum joins all OFPE participants in discussions about trial design and implementation, and the practical management implications of the data and resulting analyses. The forum will involve a global cyber-environment including all stakeholders, from university professors working in areas of complex mathematical observation to the farmers dealing with malfunctioning variable rate application machinery.
Outcomes or Projected Impacts
- Increased Farm Income from More Efficient Crop Input Management The proposed project will increase the efficiency of crop input management, enabling increased profitable use of site-specific crop input management. The economic benefits from precision farming come because a ‘‘compromise’’ in different areas’ input application rates need not then be made (Bullock and Bullock 2000). This increased efficiency, whether coming from lower costs of input application, higher yields, or both, increase farm income from their activities in private markets, but decrease the political incentives for government direct and indirect subsidization of agriculture, lowering taxpayer costs, and government debt.
- Improved Water Quality from More Efficient Crop Input Management A major purpose of agricultural research, education, and outreach is to increase the efficiency of input application management (“to grow two blades of grass, where only one grew before”). The most dramatic example of the need for increased input use efficiency is presented by the current environmental damage from inefficient nitrogen (N) fertilizer application. The proposed project will enable farmers to fertilize more where needed, and less where not needed; increasingly, N fertilizer will go into building plant structure, rather than the Gulf of Mexico, Puget Sound, Chesapeake Bay, the Great Lakes, and drinking water.
- High-skill Job Opportunities in Rural Economies The improvement in farm income and water quality discussed above needs to be achieved without neglecting the health of rural economies, which are already under considerable stress. Ideally, the benefits of better N management would be achieved in ways that encourage high-skilled job growth in rural areas. The system described here will provide high-skill job opportunities in rural communities, especially in the crop consulting industry, strengthening local economies. Numerous workshops, seminars, and symposia will communicate findings to a broad audience of academics and industry professionals, and will provide abundant training to further the advances in digital agriculture, including initiatives focusing on underrepresented farmer groups.
- High Social Returns from Increased Public Support for Agricultural Research It is well established by empirical research that there are high social returns to public agricultural research (Hurley, et al., 2014; Jin and Huffman 2016). The proposed project will involve farmers directly in research, changing the dominate research paradigm from one in which professional researchers run small-plot trials to gather data to one in which farmers and their consultants are the driving force behind agronomic research, which professional researchers “supervise” the process by providing the intellectual tools that underlay the on-farm research. This new system of public participation will not only increase the amount of agronomic research conducted, but also increase public support for government policies that encourage and support agronomic research.
Milestones
(2025):In 2025 two hundred OFPEs conducted, data processed and analyzed, reports written, aggregate report made publicly available.(2026):In 2026 four hundred OFPEs conducted, data processed and analyzed, reports written, aggregate report made publicly available.
(2027):In 2027 seven hundred and fifty OFPEs conducted, data processed and analyzed, reports written, aggregate report made publicly available.
(2028):In 2028 on thousand OFPEs conducted, data processed and analyzed, reports written, aggregate report made publicly available.
(2029):In 2029 one thousand five hundred OFPEs conducted, data processed and analyzed, reports written, aggregate report made publicly available.
(2026):Two components added to cyber-infrastructure. First additional component facilitates the organization and conduct of many-farm research projects, in which many experiments are run on fields over wide areas and multiple years. All experiments are run under similar protocols, and all data can be used in single analyses. Second additional component is a tool that government can use to implement policies that efficiently provide incentives to farmers to implement more sustainable crop production practices.
Projected Participation
View Appendix E: ParticipationOutreach Plan
We envision a world in which farmers run OFPEs on parts of every field every few years, similar to today’s soil sampling and chemical analyses. This will depend on a public education and outreach infrastructure to assist crop consultants and professional agronomists in running OFPEs, handling the data, and conducting statistical analyses. Of course, it will not be feasible to provide every crop consultant with advanced courses in data management and analysis. We envision public outreach that contains one more layer than does the traditional land grand university Extension framework, in which Extension Educators in many ways “compete” with commercial crop consultants and crop input suppliers in providing input management advice directly to farmers. Rather, Extension Educators will rely more on the private sector to reach out directly to farmers, and focus instead on educating commercial crop consultants, agronomists, and privately employed agronomists about how to use the OFPE infrastructure to the greatest advantage of their farmer-clients.
The public outreach objective of the proposed multistate will be to develop a program to teach professional crop consultants and agronomists to work with farmers at the beginning and end of the looped infrastructure illustrated by figure 2 in the Methods section. Panel A of that figure shows consultants using cloud-based software to work with farmers to design and conduct OFPEs. The DIFM project has designed early versions of the field trial design and implementation software. Extension Educators participating in the proposed multistate project will work together to develop common training session materials and presentations, to use the software in its current and later versions. Panel G also shows crop consultants using “decision tool” software with their farmer-clients, using “decision tool” software. A good deal of commercial “decision tool” software is currently available and used by crop consultants. But existing decision tools are based on data-extensive and intellectually dated analytical algorithms. The proposed project will develop data-intensive analytical algorithms, to serve as the “guts” of new commercial software packages, which will depend heavily on OFPE data.
Organization/Governance
Project Management Committee.
The Project Management Committee will be made up of the Chair, Vice-Chair, and Secretary. Each will serve a 5-year term. The Chair will be in charge of the general progress of the project, delegating responsibilities for meeting organization, logistics, and communication. The Vice Chair will assist the Chair, and fill the Chair position in the events of retirement or absence of the Chair. The Secretary will fill the Vice-Chair if needed, and is responsible for keeping minutes and organizing the writing of reports. Should the Secretary retire from office, the Chair will appoint a new Secretary from project membership, in consultation with the Vice Chair. The Project Management Committee will meet in person immediately before or after the annual project meeting, and will stay in regular contact via email and remote meetings. The Project Management Committee will be responsible for organizing the various research efforts of group members, working to make members’ research efforts complementary, where feasible. The Project Management Committee will also be responsible for organizing group efforts in obtaining outside research funding. Dr. David Bullock of the University of Illinois will serve as Chair, Dr. Taro Mieno of the University of Nebraska will serve as Vice Chair, and Dr. John Jones of the University of Illinois will serve as Secretary.
Groups
The project will be organized into five groups: the Field Trial Design Group, the Field Trial Implementation Group, the Characteristics Mapping Group, and the Empirical Analysis Group and the OFPE Systems Group. A project member will be welcome to participate in one or more groups. Each group will have a Group Leader, who will serve a five-year term. Group leaders will be approved by the Project Management Committee.
The Cyber-infrastructure Promotion Group will be responsible for promoting the use of the difm.farm cyber-infrastructure by researchers, extension personnel, crop advisors, farm managers, and farmers. This group will facilitate inter-group communication and data transfer. The group will be led by Dr. David Bullock of the University of Illinois.
The OFPE Cyber-infrastructure Development Group will be responsible for overseeing the computer coding and database development in the difm.farm cyber-infrastructure. Dr. Jinha Jung of Purdue University will lead this group.
The Field Trial Implementation and Farmer Communications Group will be made up of research and extension personnel who work with participating farmers to actually put the OFPEs “in the ground.” Representatives from several existing on-farm research groups will come together discuss and debate challenges in implementing OFPEs, receiving data from farm machinery, and working with participating farmers. The project’s outreach activities will be conducted under the purview of this group, with members collaborating in the formation and organization of common outreach methods and software. Meagan Diss of the University of Illinois will head this group.
The Characteristics Mapping Group will consist of researchers who develop and/or work with proximal and remote sensors, to collect field characteristics data. This group will also be in charge of bringing weather data into the system. The group will be lead by Dr. John Jones of the University of Illinois.
The Empirical Analysis Group will consist of those whose research involves applying statistical and machine-learning techniques to estimate yield response with OFPE, field characteristics, and weather data. Members of the group will support each other, and no doubt sometimes critique each other, in both applied yield response estimation, and in theoretical analytical research. Dr. Taro Mieno of the University of Nebraska will head this group.
Literature Cited
Ackello-Ogutu, C., Q. Paris, and W.A. Williams. 1985. “Testing a von Liebig Crop Response Function against Polynomial Specifications.” American Journal of Agricultural Economics 67 (4): 873–80. https://doi.org/10.2307/1241829.
Anderson, S.L., S.C. Murray, L. Malambo, C. Ratcliff, S. Popescu, D. Cope, A. Chang, J. Jung, and J.A. Thomasson. 2019. “Prediction of Maize Grain Yield before Maturity Using Improved Temporal Height Estimates of Unmanned Aerial Systems.” The Plant Phenome 2 (1). https://doi.org/10.2135/tppj2019.02.0004.
Anselin, L., R. Bongiovanni, and J. Lowenberg-DeBoer. 2004. “A Spatial Econometric Approach to the Economics of Site-Specific Nitrogen Management in Corn Production.” American Journal of Agricultural Economics 86 (3): 675–87. https://doi.org/10.1111/j.0002-9092.2004.00610.x.
Ashapure, A., J. Jung, J. Yeom, A. Chang, M. Maeda, A. Maeda, and J. Landivar. 2019. “A Novel Framework to Detect Conventional Tillage and No-Tillage Cropping System Effect on Cotton Growth and Development Using Multi-Temporal UAS Data.” ISPRS Journal of Photogrammetry and Remote Sensing 152 (June): 49–64. https://doi.org/10.1016/j.isprsjprs.2019.04.003.
Awika, H.O., R. Bedre, J. Yeom, T.G. Marconi, J. Enciso, K.K. Mandadi, J. Jung, and C.A. Avila. 2019. “Developing Growth-Associated Molecular Markers Via High-Throughput Phenotyping in Spinach.” The Plant Genome 12 (3). https://doi.org/10.3835/plantgenome2019.03.0027.
Bullock, D.G., and D.S. Bullock. 1994. “Quadratic and Quadratic-Plus-Plateau Models for Predicting Optimal Nitrogen Rate of Corn: A Comparison.” Agronomy Journal 86 (1): 191–95. https://doi.org/10.2134/agronj1994.00021962008600010033x.
Bullock, D.S., Matías L.R, D.G. Bullock, and G.A. Bollero. 2009. “The Value of Variable Rate Technology: An Information-Theoretic Approach.” American Journal of Agricultural Economics 91 (1): 209–23. https://doi.org/10.1111/j.1467-8276.2008.01157.x.
Cerrato, M. E., and A. M. Blackmer. 1990. “Comparison of Models for Describing; Corn Yield Response to Nitrogen Fertilizer.” Agronomy Journal 82 (1): 138–43. https://doi.org/10.2134/agronj1990.00021962008200010030x.
Chang, A., J. Jung, M.M. Maeda, and J. Landivar. 2017. “Crop Height Monitoring with Digital Imagery from Unmanned Aerial System (UAS).” Computers and Electronics in Agriculture 141 (September): 232–37. https://doi.org/10.1016/j.compag.2017.07.008.
Coble, K.H., A.K. Mishra, S. Ferrell, and T. Griffin. 2018. “Big Data in Agriculture: A Challenge for the Future.” Applied Economic Perspectives and Policy 40 (1): 79–96. https://doi.org/10.1093/aepp/ppx056.
Cook, S.E., M.L. Adams and R.J. Corner. 1999. “On-farm experimentation to determine site-specific responses to variable inputs.” Precision Agriculture: Proceedings of the 4th International Conference (Part A), edited by P. C. Robert, R. H. Rust and W. E. Larson (ASA, CSSA, SSSA, Madison, Wisconsin, USA), 611–621.
Corwin, D.L., and S.M. Lesch. 2003. “Application of Soil Electrical Conductivity to Precision Agriculture.” Agronomy Journal 95 (3): 455. https://doi.org/10.2134/agronj2003.0455.
Cite grant: number 2019-67023-29418 https://portal.nifa.usda.gov/lmd4/recent_awards?report_title=Recent%20Awards&from_site=NIFA&search_label=Awards%20Listing
“E-Fields On-Farm Research | Digital Ag.” Accessed 18 Nov. 2019. https://digitalag.osu.edu/efields.
Enciso, J., J. Jung, A. Chang and G. Cavazos. 2018. “Assessing Land Leveling Needs and Performance with Unmanned Aerial System.” Journal of Applied Remote Sensing 12 (01): 1–8. https://doi.org/10.1117/1.jrs.12.016001.
Fleming, K.L., D.G. Westphall, D.W. Wiens, L.E. Rothe, J.E. Cipra, and D.F. Heerman. 1998. Proceedings of 4th International Conference on Precision Agriculture.
Fleming, K.L., D.G. Westphall, D.W. Wiens, L.E. Rothe, J.E. Cipra, and D.F. Heerman. 1998. Proceedings of 4th International Conference on Precision Agriculture. 335-343
Griffin, T. 2018. “Collating and Analysing Small Data to Make Big Decisions — Can It Improve Farm Productivity and Profitability?” Grains Research and Development Corporation. 2018. https://grdc.com.au/resources-and-publications/grdc-update-papers/tab-content/grdc-update-papers/2018/02/collating-and-analysing-small-data-to-make-big-decisions.
Heady, E.O., and J. Pesek. 1954. “A Fertilizer Production Surface with Specification of Economic Optima for Corn Grown on Calcareous IDA Silt Loam.” Journal of Farm Economics 36 (3): 466–82. https://doi.org/10.2307/1233014.
Heiniger, R.W., R.G. Mcbride, and D.E. Clay. 2003. “Using Soil Electrical Conductivity to Improve Nutrient Management.” Agronomy Journal 95: 508–19.
Hoselton, G.S.W., M.A. Boerngen, and D.S. Bullock. In Preparation. “Farmers’ Awareness of and Concerns about Nutrient Loss.” Journal of Soil and Water Conservation.
Hoselton, G.S.W., M.A. Boerngen, and D.S. Bullock. In Preparation. “Illinois Corn Farmers’ Adoption of Best Management Practices in Response to Nutrient Loss Concerns.” Journal of Environmental Quality.
Hurley, T.M., X. Rao, and P.G. Pardey. 2014. “Re-Examining the Reported Rates of Return to Food and Agricultural Research and Development.” American Journal of Agricultural Economics 96 (5): 1492–1504. https://doi.org/10.1093/ajae/aau047.
IEPA, IDOA, and University of Illinois Extension. 2015. Illinois Nutrient Loss Reduction Strategy. n.d. Https://Www2.Illinois.Gov/Epa/Documents/Iepa/Water-Quality/Watershed-Management/Nlrs/Nlrs-Final-Revised-083115.Pdf.
“Iowa Soybean Association.” 2016. Iasoybeans.Com. Accessed 18 Nov. 2019. https://www.iasoybeans.com/programs/isa-research/what-we-do/.
Ip, R.H.L., L. Ang, K.P. Seng, J.C. Broster, and J.E. Pratley. 2018. “Big Data and Machine Learning for Crop Protection.” Computers and Electronics in Agriculture 151 (August): 376–83. https://doi.org/10.1016/j.compag.2018.06.008.
Jaynes, D. B., J. M. Novak, T. B. Moorman, and C. A. Cambardella. 1994. “Estimating Herbicide Partition Coefficients from Electromagnetic Induction Measurements.” Journal of Environment Quality 24 (1): 36–41.
Jaynes, D. B., J. M. Novak, T. B. Moorman, and C. A. Cambardella. 1995. “Estimating Herbicide Partition Coefficients from Electromagnetic Induction Measurements.” Journal of Environment Quality 24 (1): 36–41. https://doi.org/10.2134/jeq1995.00472425002400010005x.
Jin, Y., and W.E. Huffman. 2015. “Measuring Public Agricultural Research and Extension and Estimating Their Impacts on Agricultural Productivity: New Insights from U.S. Evidence.” Agricultural Economics 47 (1): 15–31. https://doi.org/10.1111/agec.12206.
Jones, R.W., S.J. Rathke, D.A. Laird, and J.F. McClelland. 2013. “Real-Time Sensing of Soil Nitrate Concentration in the Parts per Million Range While the Soil Is in Motion.” Applied Spectroscopy 67 (9): 1106–10. https://doi.org/10.1366/13-07064.
Jung, J., M. Maeda, A. Chang, J. Landivar, J. Yeom, and J. McGinty. 2018. “Unmanned Aerial System Assisted Framework for the Selection of High Yielding Cotton Genotypes.” Computers and Electronics in Agriculture 152 (September): 74–81. https://doi.org/10.1016/j.compag.2018.06.051.
Kindred, D.R., R. Sylvester-Bradley, A.E. Milne, B. Marchant, D. Hatley, S.L. Kendall, S. Clarke, K. Storer, and P.M. Berry. 2017. “Spatial Variation in Nitrogen Requirements of Cereals, and Their Interpretation.” Advances in Animal Biosciences8 (2): 303–7. https://doi.org/10.1017/S2040470017001327.
Kitchen, N.R., K.A. Sudduth, and S.T. Drummond. 1996. “Mapping of Sand Deposition from 1993 Midwest Floods with Electromagnetic Induction Measurements.” J. Soil Water Conserv. 51 (4): 336–40.
Kitchen, N.R., K.A. Sudduth, and S.T. Drummond. 1996. “Mapping of Sand Deposition from 1996 Midwest Floods with Electromagnetic Induction Measurements.” J. Soil Water Conserv. 51 (4): 336–40.
Kitchen, N.R., S.T. Drummond, E.D. Lund, K.A. Sudduth, and G.W. Buchleiter. 2003. “Soil Electrical Conductivity and Topography Related to Yield for Three Contrasting Soil–Crop Systems.” Agronomy Journal 95 (3): 483–95. https://doi.org/10.2134/agronj2003.0483.
Kweon, G. 2012. “Toward the Ultimate Soil Survey: Sensing Multiple Soil and Landscape Properties in One Pass.” Agronomy Journal 104 (6): 1547–57. https://doi.org/10.2134/agronj2012.0156.
Lund E.D., C.S. Christy, and P.E. Drummond. 2000. “Using Yield and Soil Electrical Conductivity (EC) Maps to Derive Crop Production Performance Information.” Retrieved on January 5, 2004 from Https://Pdfs.Semanticscholar.Org/3dd6/F8241781d07ca40593ec193c16d61b05c6a5.Pdf?_ga=2.41031266.1997047317.1574390094-134168074.1574390094.
Makowski, D., and D. Wallach. 2002. “It Pays to Base Parameter Estimation on a Realistic Description of Model Errors.” Agronomie 22 (2): 179–89. https://doi.org/10.1051/agro:2002002.
Marenya, P.P., and C.B. Barrett. 2009. “State-Conditional Fertilizer Yield Response on Western Kenyan Farms.” American Journal of Agricultural Economics 91 (4): 991–1006. https://doi.org/10.1111/j.1467-8276.2009.01313.x.
Marks, B., and M.A. Boerngen. 2019. “A Farming Community’s Perspective on Nutrient Loss Reduction.” Agricultural & Environmental Letters 4 (1): 0. https://doi.org/10.2134/ael2019.02.0004.
McBride, R.A., S.C. Shrive, and A.M. Gordon. 1990. “Estimating Forest Soil Quality from Terrain Measurements of Apparent Electrical Conductivity.” Soil Science Society of America Journal 54 (1): 290–93. https://doi.org/10.2136/sssaj1990.03615995005400010047x.
Mishra, S., D. Mishra, and G.H. Santra. 2016. “Applications of Machine Learning Techniques in Agricultural Crop Production: A Review Paper.” Indian Journal of Science and Technology 9 (38). https://doi.org/10.17485/ijst/2016/v9i38/95032.
Morris, T.F., T. Scott Murrell, D.B. Beegle, J.J. Camberato, R.B. Ferguson, J. Grove, Q. Ketterings, et al. 2018. “Strengths and Limitations of Nitrogen Rate Recommendations for Corn and Opportunities for Improvement.” Agronomy Journal 110 (1): 1. https://doi.org/10.2134/agronj2017.02.0112.
“Nebraska On-Farm Research Network.” 2015. CropWatch, Accessed 18 Nov. 2019. https://cropwatch.unl.edu/on-farm-research.
Paris, Q. 1992. “The von Liebig Hypothesis.” American Journal of Agricultural Economics 74 (4): 1019. https://doi.org/10.2307/1243200.
Paris, Q., and K. Knapp. 1989. “Estimation of von Liebig Response Functions.” American Journal of Agricultural Economics71 (1): 178. https://doi.org/10.2307/1241786.
Piepho, H.P, C. Richter, J. Spilke, K. Hartung, A. Kunick, and H. Thöle. 2011. “Statistical Aspects of On-Farm Experimentation.” Crop and Pasture Science 62 (9): 721–35. https://doi.org/10.1071/CP11175.
Proximal Soil Sensing. 2010. “Proximal Soil Sensing | Raphael A. Viscarra Rossel | Springer.” https://www.springer.com/gp/book/9789048188581.
Pugh, N.A., D.W. Horne, S.C. Murray, G. Carvalho, L. Malambo, J. Jung, A. Chang, et al. 2018. “Temporal Estimates of Crop Growth in Sorghum and Maize Breeding Enabled by Unmanned Aerial Systems.” The Plant Phenome 1 (1): 0. https://doi.org/10.2135/tppj2017.08.0006.
Pugh, N.A., X. Han, S.D. Collins, J.A. Thomasson, D. Cope, A. Chang, J. Jung, et al. 2018. “Estimation of Plant Health in a Sorghum Field Infected with Anthracnose Using a Fixed-Wing Unmanned Aerial System.” Journal of Crop Improvement 32 (6): 861–77. https://doi.org/10.1080/15427528.2018.1535462.
Reimer, A., J.E. Doll, B. Basso, S.T. Marquart-Pyatt, G.P. Robertson, D. Stuart, and J. Zhao. 2016. “Moving toward Sustainable Farming Systems: Insights from Private and Public Sector Dialogues on Nitrogen Management.” Journal of Soil and Water Conservation 72 (1): 5A-9A. https://doi.org/10.2489/jswc.72.1.5a.
Robert, P.C., R.H. Rust, W.E. Larson, S. E. Cook, M. L. Adams, and R. J. Corner. 1999. “On-Farm Experimentation to Determine Site-Specific Responses to Variable Inputs.” ACSESS Publications. https://doi.org/10.2134/1999.precisionagproc4.c60.
Rodriguez, D.G.P., D.S. Bullock, and M.A. Boerngen. 2019. “The Origins, Implications, and Consequences of Yield-Based Nitrogen Fertilizer Management.” Agronomy Journal 111 (2): 725. https://doi.org/10.2134/agronj2018.07.0479.
Ruffo, M.L., G.A. Bollero, D.S. Bullock, and D.G. Bullock. 2006. “Site-Specific Production Functions for Variable Rate Corn Nitrogen Fertilization.” Precision Agriculture 7 (5): 327–42. https://doi.org/10.1007/s11119-006-9016-7.
Rund, Q. 2000. “Enhanced Farm Research Analyst: Tools for on-Farm Crop Production Research.” In ESRI Conference. Accessed November 18, 2019. Available at http://citeseerx.ist.psu.edu/viewdoc/download?doi=10.1.1.138.3728&rep=rep1&type=pdf
Sadi S.G., Q. Paris and W.A. Williams. 1987. “A von Liebig Model for Water and Nitrogen Crop Response.” Western Journal of Agricultural Economics 12 (2): 182–92.
Saikai, Y., and P.D. Mitchell. 2019. “Bayesian Optimization for Precision Agriculture.” Presented at the 2019 Agricultural and Applied Economics Association Annual Meeting, Atlanta, GA. https://doi.org/10.22004/ag.econ.291207
Saikai, Y., P.D. Mitchell, and J. Colquhoun. 2018. “Multi-Armed Bandit for Experimental Plot Selection.” Presented at the 2018 Agricultural and Applied Economics Association Annual Meeting, Washington, D.C. https://doi.org/10.22004/ag.econ.274353
Saikai, Y., V. Patel, S.P. Conley, and P.D. Mitchell. 2019. “Adaptive Experimental Design Using Bayesian Optimization to Improve the Cost Efficiency of Small-Plot Field Trials.” Under Review at PLOS ONE.
Shine, P., M.D. Murphy, J. Upton, and T. Scully. 2018. “Machine-Learning Algorithms for Predicting on-Farm Direct Water and Electricity Consumption on Pasture Based Dairy Farms.” Computers and Electronics in Agriculture 150 (July): 74–87. https://doi.org/10.1016/j.compag.2018.03.023.
Stanford, G. 1966. “Nitrogen requirements of crops for maximum yield.” W.H. McVickar et al., editors, Agricultural anhydrous ammonia technology and use. Agricultural Ammonia Institute, Memphis, TN, ASA and SSSA, Madison, WI. p. 237-257.
Stanford, G. 1973. “Rationale for Optimum Nitrogen Fertilization in Corn Production1.” Journal of Environment Quality 2 (2): 159. https://doi.org/10.2134/jeq1973.00472425000200020001x.
Stauber, M.S., O.R. Burt, and F. Linse. 1975. “An Economic Evaluation of Nitrogen Fertilization of Grasses When Carry-over Is Significant.” American Journal of Agricultural Economics 57 (3): 463–71. https://doi.org/10.2307/1238409.
Sudduth, K.A., D.B. Myers, N.R. Kitchen, and S.T. Drummond. 2013. “Modeling Soil Electrical Conductivity–Depth Relationships with Data from Proximal and Penetrating ECa Sensors.” Geoderma 199 (May): 12–21. https://doi.org/10.1016/j.geoderma.2012.10.006.
Sun, L., Y. Yang, J. Hu, D. Porter, T. Marek, and C. Hillyer. 2017. “Reinforcement Learning Control for Water-Efficient Agricultural Irrigation.” 2017 IEEE International Symposium on Parallel and Distributed Processing with Applications and 2017 IEEE International Conference on Ubiquitous Computing and Communications (ISPA/IUCC), December. https://doi.org/10.1109/ispa/iucc.2017.00203.
Tembo, G., B.W. Brorsen, F.M. Epplin, and E. Tostão. 2008. “Crop Input Response Functions with Stochastic Plateaus.” American Journal of Agricultural Economics 90 (2): 424–34. https://doi.org/10.1111/j.1467-8276.2007.01123.x.
Trevisan, R.G., D.S. Bullock, and N.F. Martin. n.d. “Site-Specific Treatment Responses in On-Farm Precision Experimentation.” Preprints 2019, 2019020007.
Tumusiime, E., B. Wade, J. Mosali, J. Johnson, J. Locke, and J.T. Biermacher. 2011. “Determining Optimal Levels of Nitrogen Fertilizer Using Random Parameter Models.” Journal of Agricultural and Applied Economics 43 (4): 541–52. https://doi.org/10.1017/s1074070800000067.
Viscarra, R.A., V.I. Adamchuk, K.A. Sudduth, N.J. McKenzie, and C. Lobsey. 2011. “Proximal Soil Sensing: An Effective Approach for Soil Measurements in Space and Time.” Advances in Agronomy, iii. https://doi.org/10.1016/b978-0-12-386473-4.00010-5.
Waugh, D.L, R.B. Cate, L. Nelson, and International Soil Fertility Evaluation And Improvement Program. 1973. Discontinuous Models for Rapid Correlation, Interpretation, and Utilization of Soil Analysis and Fertilizer Response Data. Raleigh, N.C.: International Soil Fertility Evaluation And Improvement Program.
Williams, BG, and D. Hoey. 1987. “The Use of Electromagnetic Induction to Detect the Spatial Variability of the Salt and Clay Contents of Soils.” Soil Research 25 (1): 21–27. https://doi.org/10.1071/SR9870021.
“WSU Farmers Network.” Accessed 18 Nov. 2019. https://armersnetwork.wsu.edu/
Yeom, J., J. Jung, A. Chang, M. Maeda, and J. Landivar. 2018. “Automated Open Cotton Boll Detection for Yield Estimation Using Unmanned Aircraft Vehicle (UAV) Data.” Remote Sensing 10 (12): 1895. https://doi.org/10.3390/rs10121895.