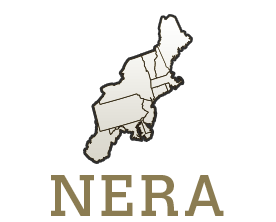
NE2204: A regional network of social, behavioral, and economic food systems research
(Multistate Research Project)
Status: Active
NE2204: A regional network of social, behavioral, and economic food systems research
Duration: 01/20/2023 to 09/30/2027
Administrative Advisor(s):
NIFA Reps:
Non-Technical Summary
Statement of Issues and Justification
The global food system will need to feed 10 billion people by 2050, but currently, more than 10% of American households are food insecure, and global food insecurity has increased by over 30% because of the COVID-19 pandemic (Coleman-Jensen et al., 2020; Baquedano et al., 2021) Rapid population growth, shrinking farmland, dwindling natural resources, erratic climate, and shifting market demand will push the global agricultural production system into a new paradigm (FAO, 2017). This development implies that the food system must become more productive in output, efficient in operation, resilient to climate change, and sustainable for future generations (Vaio et al., 2020).
Artificial intelligence (AI) holds promise in addressing the challenges of this new paradigm by advancing agricultural technologies, improving supply chain management, and generating new knowledge regarding the functioning, interactions, and consequences of the U.S. food system (Liu, 2020). Yet, most AI innovations in agriculture are limited to production data collection and analysis, with some post-farmgate applications targeting product traceability and quality monitoring (Kakani et al., 2020; Misra et al., 2020; Paul et al., 2021). This constraint indicates a clear need for new research that builds on AI and big data beyond the farmgate to answer research questions of national scope related to marketing (including supply chain logistics), food retailing, and consumer behavior.
Interdisciplinary approaches have been recommended for topics including health and agriculture (Waage et al., 2019), food security (Acevedo, 2011; Foran et al., 2014), rural economy and land use (Lowe and Phillipson, 2006), and agri-food science (Horton et al., 2017). The complexity of the social, economic, and environmental interactions in the food system requires problems to be addressed through an interdisciplinary lens (Duffy et al., 1997; Foran et al., 2014). A consequence of not taking a systems approach, and instead pursuing siloed research projects, is that it leads to an incomplete framing of the problem and the development of inadequate research priorities (Lowe and Phillipson, 2006).
Big data, such as the location and description of points of interest (POIs), including grocery stores, restaurants, health facilities and centers, and indoor and outdoor physical activity locations, provides an exciting opportunity to understand and measure the nuances of a ‘healthy’ neighborhood and access to related POIs (Gibson et al., 2014; Wilkins et al., 2019; Shannon et al., 2021). This potential of big data and related data science techniques extends the current work on ‘neighborhood and health’ as neighborhoods can now be measured with rich and detailed multidimensional data. This research will benefit a variety of stakeholders, including policymakers, consumers, and agribusinesses.
Related, Current and Previous Work
While interdisciplinary work on big data has been increasing because of improved and more affordable processing, storage, and transmission capabilities (National Academy, 2005), this work has progressed more slowly within the agricultural domain. Most discussions of agricultural data innovations involve production data collection and analysis, with some post-farmgate applications targeting product traceability and quality monitoring (Kamilaris et al., 2017; Klerkx et al., 2019). This limitation indicates a clear need for increased use of big data to answer questions related to marketing (including supply chain logistics), food retailing, and consumer choices.
Mobility big data and AI systems can play a critical role in advancing food production, processing, and consumption systems (Misra et al., 2020). Such data hold substantial potential for research into food systems because mobility profiles allow researchers to understand agent behavior, interactions, and incentives, a fundamental goal of social science research (Kakani et al., 2020). Mobility data also enables us to evaluate the impact of public policies and the implications for human health, providing new and potentially revolutionizing insights into the structure and conduct of the food system and its consequences for the social, economic, and health well-being of U.S. society. There is an expressed need for frontier research that builds on modern AI systems and big data beyond the farmgate to develop new insights into the agriculture and food system. Although technological developments play a critical role in advancing the efficiency and sustainability of the food system, a substantial advancement of knowledge is to be expected from research that expands the current research paradigm beyond the farm gate (Paul et al., 2021). Multi-domain mobility big data are a critical innovation that holds significant promise in this research area. Social science research enabled through AI systems and the mobility big data will help improve the efficiency and sustainability of the previously unimaginable food production, processing, and consumption system due to data and methods limitations, academic silos, and missing opportunities for transdisciplinary engagement and collaboration in the food systems sciences. The proposed research and network initiatives will help close this gap by providing an innovative platform for transdisciplinary research, collaboration, and engagement to foster food systems research and generate new knowledge that can inform policymakers and other stakeholders through new insights from modern AI systems and mobility big data.
Despite its potential to generate new knowledge in the food domain, the use of AI systems in the social sciences is in its infancy, which is primarily due to data constraints and limited research collaboration between computer science and the social sciences (Athey, 2019; Storm et al., 2020). Recent studies point toward the benefits of big data and AI for socioeconomic analysis (Einav and Levin, 2014; Varian, 2014; Bajari et al., 2015; Grimmer, 2015; Monroe et al., 2015; Athey and Imbens, 2016) and business management (Raj et al., 2015; Shekhar et al., 2017; Coble et al., 2018; Kamilaris and Prenafeta-Boldú, 2018). Although the rising use of AI techniques in the social sciences is encouraging, little systematic work has been done to advance our knowledge of the functioning and conduct of the U.S. food system.
Objectives
-
Create an interdisciplinary network of researchers that use mobility big data to address questions related to the food system, agriculture, health, and the environment.
-
Assess the impact of economic and social shocks, such as COVID-19, competitor market entry, and public policies, on consumer, producer, and supplier behavior.
-
Analyze the degree to which the community food landscape and neighborhood characteristics interact to analyze a) consumer behavior regarding traditional and non-traditional retailers and other points of interest and b) firm behavior regarding entry, exit location choice, and competition.
Methods
This research will capitalize on a unique dataset of 200 million cell phone users. To assess the usability and quality of the mobility big data, we initiated procedures to access critical databases for this research project. This cell phone location data will come from Veraset (2021), one of the leading location data companies in the United States. Veraset provides a real-time feed of accurate mobility data for more than 200 million mobile devices. The average device reports its location 150-200 times a day, mainly during the daytime, with an accuracy of 25 cm evaluated using multiple sensors, relying primarily on GPS information, Beacons, and WiFi signals for cross-validation. Every location signal is examined for quality and origin and then flagged to validate point of interest (POI) visits. All device data are fully anonymized, and no personal information can be inferred from the database.
A core team of researchers in agricultural economics, geography, supply chain logistics, and computer science has already begun working with this data. While computer scientists will primarily complete data cleaning and manipulation, this project intends to coordinate research collaborations using cleaned and refined data. Specifically, this multistate hatch will engage students, professionals, universities, and the public and private sectors in food systems research. While we provide examples of research questions and analytical techniques for each objective based on the interest of the current research team, it is expected that this will expand as the number of participants grows.
Objective 1:
The primary goal of this multistate Hatch project is the facilitation of networking amongst potential collaborators, including both academics and practitioners interested in food systems research. Potential disciplines include business economics, local and regional food systems, geography, supply chain management, computer science, systems science, food retail marketing, nutrition, health, and food policy. The mobility data provides location-based movement information, which researchers can combine with other food and agricultural datasets, including NETS, Nielsen, and USDA's food atlas. Following FAIR standards, we will share code and data through a public webpage and GitHub. These shareable data products will promote the integration of visualization and analytic methods to support discovering and analyzing trends and policy-relevant findings concerning food and agriculture.
A key focus of this collaborative network is to address relevant, stakeholder-driven research questions across the entire food system. Priorities can be developed through outreach to policymakers, Extension staff, producers, and food and agricultural organizations. For instance, interest has been expressed in using this mobile data to assess whether a sub-state marketing effort led to increased traffic at direct-marketing establishments.
To create and sustain interest in the network, we will establish an annual symposium and virtual seminar series through UConn’s Zwick Center for Food and Resource Policy. The emphasis will be on presenting policy-relevant research findings that appeal to various audiences. To engage students in this interdisciplinary project, a part of the symposium will be dedicated to undergraduate and graduate student networking and professional development.
Objective 2:
The COVID-19 pandemic is expected to have long-lasting effects on agricultural supply chains and food access, local agricultural systems, and consumer choices and health. The longitudinal nature of our data will also allow us to assess the impacts of COVID-19 on various parts of the food system as we will have information on consumer movement in 2019 (pre-COVID), 2020-2022 (during COVID), and 2023-2025 (post-COVID). This type of modeling has been applied to leisure activities (Brey, 2020), understanding social distancing activities (Brzezinski et al., 2020; Tian et al., 2021), and healthcare utilization (Cantor et al., 2021). However, despite the mentioned technical advances and growing use of phone mobility data, there is a lack of systematic, comprehensive, and fine-grained consumer choice research related to consumer food retailer choice and supply logistics.
Frontier research methods (Freyaldenhoven et al., 2019; Borusyak and Jaravel, 2020; Clarke and Schythe 2020) will be used to measure the impact of economic and social shocks on consumer choice. A count data regression model (e.g., Poisson PML) can be used to analyze how visits to various points of interest change due to a shock, such as COVID-19 or the entrance or exit of new retailers. Combined with machine learning, this will also allow for assessing complementary behavior and the interplay with sociodemographic characteristics.
Objective 3:
Prior research demonstrates a clear relationship between neighborhood socioeconomic characteristics and location outcomes (Lytle and Sokol, 2017), with healthier or more diverse food stores located in higher-income neighborhoods (Zenk et al., 2005; Zhang et al., 2015). This disparate level of food access has long been a concern of policymakers targeting both the supply and demand side. Researchers can use mobility big data to dig deeper into social justice questions and assess whether neighborhood-level racial/ethnic segregation and poverty are associated with various individual-level choices, including food shopping behavior, restaurant patronage, and healthcare utilization. This DEI framework will be incorporated into each of the following sub-objectives.
Objective 3a: Consumer Behavior
Researchers can answer whether people living in segregated neighborhoods (tract or block groups) can visit retailers and other points of interest within their communities. Through mobile data, we can identify the neighborhood demographics of all patrons and travel distance to their chosen outlets. Researchers can identify the effect of distance on consumer choices and assess moderation and interaction effects. AI systems allow for identifying visitors to retailers/restaurants, complementary behavior, and general patterns. At the same time, computer scientists and other data science researchers may design and execute the machine learning algorithms, and all partners can develop research questions around access, choice, and health.
To understand consumer behavior concerning retailers, theory traditionally suggests that consumers will choose the combination of establishments in multi-purpose trips that maximize total utility (Marianov et al., 2018). By applying machine learning to mobility big data we can take advantage of complex models of consumer product preferences that assess demand for different types of stores and specific stores within each category simultaneously (Donnelly et al., 2021). Through nested factorization, this approach overcomes the data problem that on any given day, the probability of going to a particular store or restaurant is relatively low while also accounting for the correlation between demand for different establishments. Machine learning is well-suited to this type of analysis, and we can explore potential heterogeneous impacts by separating nearby businesses by industry.
For food away from home, an extensive literature links the choice to visit a restaurant, the amount spent, and consumer characteristics (e.g., Anderson and Matsa, 2011; Eckert and Vojnociv, 2017; Athey et al., 2018). Equity concerns in restaurant location choice, including whether neighborhood-level racial/ethnic segregation and poverty are associated with consumer restaurant choice, can be assessed. Depending on the researcher's interests, this analysis can be extended to other points of interest, including healthcare utilization and visits to open spaces and exercise facilities.
AI systems and mobile big data allow researchers to answer questions about agricultural direct marketing not previously possible due to data constraints. Most studies of agricultural direct marketing operations have relied on either survey data or case studies, which are primarily localized and rely on sample sizes of less than 500 respondents, calling into question their external validity and the ability for causal inference (see, e.g., Govindasamy et al., 1998; Eastwood, 2001; Andreatta and Wickliffe, 2002; Hunt, 2007; Velasquez et al., 2005; Baker et al., 2009; Alonso and O’Neill, 2011; Ruelas et al., 2011; Dodds et al., 2014; Gumirakiza, 2014; Jilcott Pitts et al., 2014). This is a concerning gap as the prevalence of local foods has proliferated in the past decade (Martinez, 2010; USDA, 2013; Tropp, 2014), and local food access provides benefits to consumers, producers, and the local community (see, e.g., Loureiro and Hine, 2002; Feenstra et al., 2003; Govindasamy et al., 2003; Otto and Varner, 2005; Boyle et al., 2008; Carpio et al., 2008; Darby et al., 2008; Henneberry et al., 2008; McCormack, 2010; George et al., 2011; Young et al., 2011; Fang et al., 2013; Richards, 2014; Hughes and Massa, 2015; Fedorowicz et al., 2020; Hadavas, 2020). Of particular concern to policymakers, stakeholders, and Extension personnel striving to support smaller farming operations, the number of direct-marketing operations has continued to grow over the last two decades without noticeable changes in sales, suggesting a saturation of the market (Low et al., 2015; Helmer, 2019). We can identify the neighborhood demographics of all patrons and travel distance to their chosen establishments and combine this with other datasets on locational characteristics. This allows us to assess the degree to which retail store availability and neighborhood characteristics interact to predict the procurement of products through agricultural direct marketing establishments such as farmers’ markets or farm stands, which has implications for producers and policymakers. Following other work on retail store choices (Fox et al., 2004; Singh et al., 2006; Dong and Stewart, 2012) we can use a two-stage utility-maximizing decision-making process to analyze both establishment choice and time spent. Researchers can assess this consumer behavior regarding distinct marketing arrangements on a national level, including attributes that impact consumer market choice and potential spillover effects.
Objective 3b: Firm Behavior
A highly innovative aspect of this research is the ability to define market size. Researchers make arbitrary assumptions by defining markets based on ZIP codes and county borders due to data constraints (e.g., Basker, 2005; Allcott et al., 2019; Chenarides and Jaenicke, 2019). However, these assumptions can mask potential interactions between stores/locations and who is going there. Similarly, studies of competitive behavior (e.g., Bresnahan and Reiss, 1991) have concentrated on rural and isolated counties to allow for identification. Mobile data allows us to calculate travel distance to distinct types of establishments and identify the home neighborhoods of patrons. AI systems and machine learning allows us to not only define markets for each retail store and assess competition effects but also link to questions concerning equity and access to SNAP and other food assistance resources.
Defining boundaries also allows us to study the competitive behavior of firms. Any market structure study begins with carefully defining market boundaries (Bresnahan and Reiss, 1991; Berry, 1992; Mazzeo, 2002), often using proxies such as isolated counties or highway exits. We can build on this literature by using data-driven analytical methods to construct market boundaries based on consumer choices. As we observe the dwelling of each visitor to the point of interest, we can use that information to estimate the spatial and temporal boundaries of each consumer market using a spatial boundary construction algorithm. We can then assess the impact of a new entrant on not just firm behavior but also consumer choice.
Measurement of Progress and Results
Outputs
- Annual in-person research symposia.
- Monthly virtual presentations and networking opportunities.
- Trained students in multidisciplinary methods.
- Geospatial cyberinfrastructure system that will pave new directions for the research community in food production, processing, and consumption systems through our novel AI applications.
- A user-friendly database combining research results will be made available to the public through a dedicated web interface and GitHub that integrates visualization and statistical methods.
Outcomes or Projected Impacts
- Developing a sustainable network of collaborators on big data and food systems, resulting in increased consumer and producer welfare. Metrics will include the number of collaborators, attendance at seminars and symposia, and the number of research publications and presentations.
- Increase in stakeholder-driven research in food systems. Metrics will include the number of projects that address a research question identified by stakeholders, the number of publications with a non-academic co-author, and the number of extension reports, policy briefs, and stakeholder presentations.
- Improved understanding of consumer and producer spatial interactions in the food system. Will be assessed through surveys of participants on the perceived significance of research findings and citation counts of publications.
Milestones
(2024):The first research symposia will be held in the Spring of 2024Projected Participation
View Appendix E: ParticipationOutreach Plan
Research through this project will lead to new knowledge regarding the structure and functioning of food production, processing, and consumption systems based on newly collected data and rigorous statistical assessment enabled through mobility big data and innovative AI systems. This research will improve our understanding of the relationship between public policies, food production, retailing, and consumer choices. The project will have a dedicated community-facing webpage on the University of Connecticut system. There will be separate sections for research findings and conclusions, policy-specific reports and stakeholder presentations, student engagement, and the research network. An example can be found at https://cannabis.cahnr.uconn.edu/.
Through partner Extension offices and producer groups, stakeholder input will be solicited in developing research questions and disseminating results. Producers and other food systems stakeholders will be actively recruited to participate in the research symposia, and listening sessions will be held to understand the research needs of these communities.
The project team will explore ways to actively disseminate research and network findings of national scope through the media. General interest articles will be published in Nature or Choices. Extension and outreach reports will be published through UConn’s Zwick Center for Food and Resource Policy. Collaborations with the USDA will be used to inform the legal and executive branches about research findings. All non-proprietary data and research methods will be made available to the food systems community through web access to datasets and codes via the dedicated project webpage and GitHub. All datasets and the procedures for processing and analyzing the data have the potential to be particularly useful for future research. The research will also be presented at academic conferences and industry workshops (AAEA, ASSA, NBER, ACM UbiComp, WWW) and published in well-recognized academic journals, such as Land Economics, the American Journal of Agricultural Economics, IEEE Transactions on Mobile Computing, and IEEE Transaction on Knowledge and Data Engineering.
Organization/Governance
All members of this multi-state HATCH project are eligible for office. The organization is as follows:
Officers: The chairperson is elected by the voting members to a one-year term and may be re-elected for additional terms of office. In consultation with the administrative advisor, the chairperson notifies the committee members of the time and place of meetings, prepares the agenda, and presides at the committee and executive committee meetings. They are responsible for preparing the annual report of the project. Following the chairperson’s term, the project secretary will become the chairperson.
Secretary: The secretary records the minutes and performs other duties assigned by the committee or the administrative advisor. They are elected by the voting members to a one-year term and will become the committee's chair. A new secretary for the new committee will be elected for a one-year term as secretary and then will become chair in the following year.
Subcommittees: The Project will have an executive committee designated to conduct the committee's business between meetings and perform other duties. It consists of the Project chairperson, secretary, and two other committee members. These two members are elected by the committee's voting members to one-year terms and may be reelected for additional terms of office. The chairperson names other subcommittees as needed for specific assignments such as developing procedures, planning conferences, and preparing publications.
Literature Cited
National Academy. 2005. Facilitating interdisciplinary research. url: https://www.nap.edu/catalog/11153/facilitating-interdisciplinary-research.
Acevedo, M. F. 2011. Interdisciplinary progress in food production, food security and environment research. Environmental Conservation 38 (2), pp. 151–171.
Agatz, N., Campbell, A., Fleischmann, M., and Savelsbergh, M. 2011. Time slot management in attended home delivery. Transportation Science 45 (3), pp. 435–449.
Akkas, A., Gaur, V., and Simchi-Levi, D. 2019. Drivers of product expiration in consumer-packaged goods retailing. Management Science 65 (5), pp. 2179–2195.
Allcott, H., Diamond, R., Dubé, J.-P., Handbury, J., Rahkovsky, I., and Schnell, M. 2019. Food deserts and the causes of nutritional inequality. The Quarterly Journal of Economics 134 (4), pp. 1793–1844.
Alonso, A. D. and O’Neill, M. A. 2011. A comparative study of farmers’ markets visitors’ needs and wants: the case of Alabama. International Journal of Consumer Studies 35 (3), pp. 290–299.
Anderson, M. L. and Matsa, D. A. 2011. Are restaurants really supersizing America? American Economic Journal: Applied Economics 3 (1), pp. 152–188.
Andreatta, S. and Wickliffe, W. 2002. Managing farmer and consumer expectations: a study of a North Carolina farmers market. Human Organization 61 (2), pp. 167–176.
Athey, S. 2019. The impact of machine learning on economics. The Economics of Artificial Intelligence. University of Chicago Press, pp. 507–552.
Athey, S., Blei, D., Donnelly, R., Ruiz, F., and Schmidt, T. 2018. Estimating heterogeneous consumer preferences for restaurants and travel time using mobile location data. AEA Papers and Proceedings 108, pp. 64–67.
Athey, S. and Imbens, G. W. 2016. Machine learning methods for estimating heterogeneous causal effects. Stat 1050 (5), pp. 1–26.
Bajari, P., Nekipelov, D., Ryan, S. P., and Yang, M. 2015. Machine learning methods for demand estimation. American Economic Review 105 (5), pp. 481–85.
Baker, D., Hamshaw, K., and Kolodinsky, J. 2009. Who shops at the market? Using consumer surveys to grow farmers’ markets: findings from a regional market in northwestern Vermont. Journal of Extension 47 (6), pp. 1–9.
Baquedano, F., Zereyesus, Y. A., Christensen, C., and Valdes, C. 2021. Covid-19 working paper: international food security assessment, 2020-2030: covid-19 update and impacts of food insecurity.
Basker, E. 2005. Job creation or destruction? Labor market effects of Walmart expansion. Review of Economics and Statistics 87 (1), pp. 174–183.
Belavina, E. 2021. Grocery store density and food waste. Manufacturing & Service Operations Management 23 (1), pp. 1–18.
Berry, S. T. 1992. Estimation of a model of entry in the airline industry. Econometrica: Journal of the Econometric Society, pp. 889–917.
Borusyak, K. and Jaravel, X. 2020. Revisiting event study designs. Working Paper. url: https://scholar.harvard.edu/files/borusyak/files/borusyak_jaravel_event_studies.pdf.
Boyle, M., MPH, S. S.-F., and DrPH, S. E. S. 2008. Environmental strategies and policies to support healthy eating and physical activity in low-income communities. Journal of Hunger & Environmental Nutrition 1 (2), pp. 3–25.
Bresnahan, T. F. and Reiss, P. C. 1991. Entry and competition in concentrated markets. Journal of Political Economy 99 (5), pp. 977–1009.
Carpio, C. E., Wohlgenant, M. K., and Boonsaeng, T. 2008. The demand for agritourism in the United States. Journal of Agricultural and Resource Economics 33 (2), pp. 254–269.
Chenarides, L. and Jaenicke, E. C. 2019. Documenting the link between poor food access and less healthy product assortment across the US. Applied Economic Perspectives and Policy 41 (3), pp. 434–474.
Clarke, D. and Schythe, K. 2020. Implementing the panel event study. Discussion Paper Series 13524. url: https://papers.ssrn.com/abstract=3660271.
Coble, K. H., Mishra, A. K., Ferrell, S., and Griffin, T. 2018. Big data in agriculture: a challenge for the future. Applied Economic Perspectives and Policy 40 (1), pp. 79–96.
Coleman-Jensen, A., Rabbitt, M. P., Gregory, C. A., and Singh, A. 2020. Household food security in the United States in 2019. url: http://www.ers.usda.gov/publications/pub-details/?pubid=99281.
Darby, K., Batte, M. T., Ernst, S., and Roe, B. 2008. Decomposing local: a conjoint analysis of locally produced foods. American Journal of Agricultural Economics 90 (2), pp. 476–486.
Dodds, R., Holmes, M., Arunsopha, V., Chin, N., Le, T., Maung, S., and Shum, M. 2014. Consumer choice and farmers’ markets. Journal of Agricultural and Environmental Ethics 27 (3), pp. 397–416.
Donnelly, R., Ruiz, F.J., Blei, D. and Athey, S. 2021. Counterfactual inference for consumer choice across many product categories. Quantitative Marketing and Economics 19, 369–407. https://doi.org/10.1007/s11129-021-09241-2
Eastwood, D. B. 2001. Consumers’ willingness to travel to farmers’ markets. Journal of Food Products Marketing 6 (3), pp. 31–44.
Eckert, J. and Vojnovic, I. 2017. Fast food landscapes: exploring restaurant choice and travel behavior for residents living in lower eastside detroit neighborhoods. Applied Geography 89, pp. 41–51.
Einav, L. and Levin, J. 2014. Economics in the age of big data. Science 346 (6210).
Fang, M., Buttenheim, A. M., Havassy, J., and Gollust, S. E. 2013. “It’s not an ‘if you build it they will come’ type of scenario”: stakeholder perspectives on farmers’ markets as a policy solution to food access in low-income neighborhoods. Journal of Hunger & Environmental Nutrition 8 (1), pp. 39–60.
FAO. 2017. The future of food and agriculture. url: http://www.fao.org/family-farming/detail/en/c/854650/.
Fedorowicz, M., Schilling, J., Bramhall, E., Bieretz, B., Su, Y., and Brown, S. 2020. Leveraging the built environment for health equity. url: https://www.urban.org/research/publication/leveragingbuilt-environment-health-equity.
Feenstra, G. W., Lewis, C. C., Hinrichs, C. C., Gillespie, G. W., and Hilchey, D. 2003. Entrepreneurial outcomes and enterprise size in US retail farmers’ markets. American Journal of Alternative Agriculture 18 (1), pp. 46–55.
Foran, T., Butler, J. R. A., Williams, L. J., Wanjura, W. J., Hall, A., Carter, L., and Carberry, P. S. 2014. Taking complexity in food systems seriously: an interdisciplinary analysis. World Development 61, pp. 85–101.
Freyaldenhoven, S., Hansen, C., and Shapiro, J. M. 2019. Pre-event trends in the panel event-study design. American Economic Review 109 (9), pp. 3307–38.
George, D. R., Kraschnewski, J. L., and Rovniak, L. S. 2011. Public health potential of farmers’ markets on medical center campuses: A case study from Penn State Silton S. Hershey Medical Center. American Journal of Public Health 101 (12), pp. 2226–2232.
Gibson, B. A., Ghosh, D., Morano, J. P., and Altice, F. L. 2014. Accessibility and utilization patterns of a mobile medical clinic among vulnerable populations. Health & Place 28, pp. 153–166.
Govindasamy, R., Italia, J., Zurbriggen, M., and Hossain, F. 2003. Producer satisfaction with returns from farmers’ market related activity. American Journal of Alternative Agriculture 18 (2), pp. 80–86.
Govindasamy, R., Zurbriggen, M., Italia, J., Adelaja, A. O., Nitzsche, P., and VanVranken, R. 1998. Farmers markets: consumer trends, preferences, and characteristics. Tech. rep. url: https://ageconsearch.umn.edu/record/36722/.
Grimmer, J. 2015. We are all social scientists now: how big data, machine learning, and causal inference work together. PS: Political Science & Politics 48 (1), pp. 80–83.
Gumirakiza, D., Curtis, K., and Bosworth, R. 2014. Who attends farmers’ markets and why? Understanding consumers and their motivations. International Food and Agribusiness Management Review 17, pp. 65– 82.
Hadavas, C. 2020. “We’re in a save-our-farm-from-collapsing mode”. url: https://slate.com/humaninterest/2020/04/csa-farmers-markets-coronavirus-demand-rise.html.
Helmer, J. 2019. Why are so many farmers markets failing? Because the market is saturated. url: https: //www.npr.org/sections/thesalt/2019/03/17/700715793/why-are-so-many-farmers-marketsfailing-because-the-market-is-saturated.
Henneberry, S. R., Taylor, M. J., Whitacre, B. E., Agustini, H. N., Mutondo, J. E., and Roberts, W. 2008. The economic impacts of direct produce marketing: a case study of Oklahoma’s famers’ markets. Tech. rep. url: https://ageconsearch.umn.edu/record/6785/files/sp08he08.pdf.
Horton, P., Banwart, S. A., Brockington, D., Brown, G. W., Bruce, R., Cameron, D., Holdsworth, M., Lenny Koh, S. C., Ton, J., and Jackson, P. 2017. An agenda for integrated system-wide interdisciplinary agri-food research. Food Security 9 (2), pp. 195–210.
Hu, M., Xu, X., Xue, W., and Yang, Y. 2021. Demand pooling in omnichannel operations. Management Science.
Hughes, D. and Isengildina-Massa, O. 2015. The economic impact of farmers’ markets and a state level locally grown campaign. Food Policy 54.
Hunt, A. R. 2007. Consumer interactions and influences on farmers’ market vendors. Renewable Agriculture and Food Systems 22 (1), pp. 54–66.
Jilcott Pitts, S. B., Gustafson, A., Wu, Q., Mayo, M. L., Ward, R. K., McGuirt, J. T., Rafferty, A. P., Lancaster, M. F., Evenson, K. R., Keyserling, T. C., and Ammerman, A. S. 2014. Farmers’ market use is associated with fruit and vegetable consumption in diverse southern rural communities. Nutrition Journal 13, p. 1.
Kakani, V., Nguyen, V. H., Kumar, B. P., Kim, H., and Pasupuleti, V. R. 2020. A critical review on computer vision and artificial intelligence in food industry. Journal of Agriculture and Food Research 2, p. 100033. url: https://www.sciencedirect.com/science/article/pii/S2666154320300144.
Kamilaris, A., Kartakoullis, A., and Prenafeta-Boldú, F. X. 2017. A review on the practice of big data analysis in agriculture. Computers and Electronics in Agriculture 143, pp. 23–37. url: https://www. sciencedirect.com/science/article/pii/S0168169917301230.
Kamilaris, A. and Prenafeta-Boldú, F. X. 2018. Deep learning in agriculture: a survey. Computers and Electronics in Agriculture 147, pp. 70–90.
Klerkx, L., Jakku, E., and Labarthe, P. 2019. A review of social science on digital agriculture, smart farming and agriculture 4.0: new contributions and a future research agenda. NJAS-Wageningen Journal of Life Sciences 90. Publisher: Elsevier, p. 100315.
Low, S. A., Adalja, A., Beaulieu, E., Key, N., Martinez, S., Melton, A., Perez, A., Ralston, K., Stewart, H., Suttles, S., Vogel, S., and Jablonski, B. B. R. 2015. Trends in U.S. local and regional food systems: A report to congress. url: http://www.ers.usda.gov/publications/pub-details/?pubid=42807.
Lim, M. K., Mak, H.-Y., and Shen, Z.-J. M. 2017. Agility and proximity considerations in supply chain design. Management Science 63 (4), pp. 1026–1041.
Liu, S. Y. 2020. Artificial intelligence (AI) in agriculture. IT Professional 22 (3), pp. 14–15.
Loureiro, M. and Hine, S. 2002. Discovering niche markets: a comparison of consumer willingness to pay for local (Colorado grown), organic, and GMO-free products. Journal of Agricultural & Applied Economics 34, pp. 477–487.
Lowe, P. and Phillipson, J. 2006. Reflexive interdisciplinary research: the making of a research programme on the rural economy and land use. Journal of Agricultural Economics 57 (2), pp. 165–184.
Lytle, L. A. and Sokol, R. L. 2017. Measures of the food environment: a systematic review of the field, 2007–2015. Health & place 44, pp. 18–34.
Marianov, V., Eiselt, H. A., and Lüer-Villagra, A. 2018. Effects of multipurpose shopping trips on retail store location in a duopoly. European Journal of Operational Research 269 (2), pp. 782–792.
Martinez, S., H, M. S., Pra, M. D., Pollack, S., Ralston, K., Smith, T., Vogel, S., Clark, S., Lohr, L., Low, S. A., and Newman, C. 2010. Local food systems: concepts, impacts, and issues. Economic Research Report No. 97. United States Department of Agriculture. url: https://www.ers.usda.gov/webdocs/ publications/46393/7054_err97_1_.pdf?v=9070.8.
Mazzeo, M. J. 2002. Product choice and oligopoly market structure. RAND Journal of Economics, pp. 221– 242.
McCormack, L. A., Laska, M. N., Larson, N. I., and Story, M. 2010. Review of the nutritional implications of farmers’ markets and community gardens: a call for evaluation and research efforts. Journal of the American Dietetic Association 110 (3), pp. 399–408.
Misra, N. N., Dixit, Y., Al-Mallahi, A., Bhullar, M. S., Upadhyay, R., and Martynenko, A. 2020. loT, big data and artificial intelligence in agriculture and food industry. IEEE Internet of Things Journal, pp. 1–1.
Monroe, B. L., Pan, J., Roberts, M. E., Sen, M., and Sinclair, B. 2015. No! Formal theory, causal inference, and big data are not contradictory trends in political science. Political Science & Politics 48 (1), pp. 71– 74.
Otto, D. and Varner, T. 2005. Consumers, vendors, and the economic importance of Iowa farmers’ markets: an economic impact survey analysis. url: https://core.ac.uk/download/pdf/38940586.pdf.
Paul, A., Gaur, S., and Ahamed, M. 2021. Artificial intelligence: importance in food chain industry. SSRN Scholarly Paper ID 3855252. Rochester, NY: Social Science Research Network. url: https://papers.ssrn.com/abstract=3855252.
Raj, M. P., Swaminarayan, P. R., Saini, J. R., and Parmar, D. K. 2015. Applications of pattern recognition algorithms in agriculture: a review. International Journal of Advanced Networking and Applications 6 (5), p. 2495.
Richards, A. 2014. Wyoming farmers markets have a big impact on the economy. url: https://kgab.com/wyoming-farmers-markets-have-a-big-impact-on-the-economy/.
Ruelas, V., Iverson, E., Kiekel, P., and Peters, A. 2011. The role of farmers’ markets in two low income, urban communities. Journal of Community Health 37 (3), pp. 554–562.
Shannon, J., Reese, A., Ghosh, D., Widener, M., and Block, D. 2021. More than mapping: improving methods for studying the geographies of food access. American Journal of Public Health 111 (8), pp. 1418-1422.
Shekhar, S., Schnable, P., LeBauer, D., Baylis, K., and VanderWaal, K. 2017. Agriculture big data (AGBD) challenges and opportunities from farm to table: a midwest big data hub community whitepaper. White paper for the US National Institute of Food and Agriculture.
Shen, Z.-J. M., Coullard, C., and Daskin, M. S. 2003. A joint location-inventory model. Transportation Science 37 (1), pp. 40–55.
Storm, H., Baylis, K., and Heckelei, T. 2020. Machine learning in agricultural and applied economics. European Review of Agricultural Economics 47 (3), pp. 849–892.
Tropp, D. 2014. Why local food matters: the rising importance of locally-grown food in the U.S. food system. url: https://ageconsearch.umn.edu/record/160752.
Vaio, A., Boccia, F., Landriani, L., and Palladino, R. 2020. Artificial intelligence in the agri-food system: rethinking sustainable business models in the covid-19 scenario. Sustainability 12 (12), p. 4851.
Varian, H. R. 2014. Big data: new tricks for econometrics. Journal of Economic Perspectives 28 (2), pp. 3–28.
Velasquez, C., Eastman, C., and Masiunas, J. 2005. An assessment of Illinois farmers’ market patrons’ perceptions of locally-grown vegetables. Journal of Vegetable Science 11 (1), pp. 17–26.
Waage, J., Cornelsen, L., Dangour, A. D., Green, R., Häsler, B., Hull, E., Johnston, D., Kadiyala, S., Lock, K., Shankar, B., Smith, R. D., and Walls, H. L. 2019. Integrating agriculture and health research for development: LCIRAH as an interdisciplinary programme to address a global challenge. Global Challenges (Hoboken, Nj) 3 (4), p. 1700104.
Wilkins, E., Morris, M., Radley, D., and Griffiths, C. 2019. Methods of measuring associations between the retail food environment and weight status: importance of classifications and metrics. SSM - Population Health 8, p. 100404.
Young, C., Karpyn, A., Uy, N., Wich, K., and Glyn, J. 2011. Farmers’ markets in low income communities: impact of community environment, food programs and public policy. Community Development 42 (2), pp. 208–220.
Zenk, S. N., Schulz, A. J., Israel, B. A., James, S. A., Bao, S., and Wilson, M. L. 2005. Neighborhood racial composition, neighborhood poverty, and the spatial accessibility of supermarkets in metropolitan detroit. American Journal of Public Health 95 (4), pp. 660–667.
Zhang, M. and Ghosh, D. 2015. Spatial supermarket redlining and neighborhood vulnerability: A case study of Hartford, Connecticut. Transactions in GIS 20 (1), pp. 79–100.