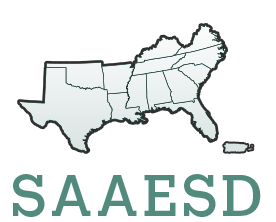
S1089: Advanced Understanding and Prediction of Pollutants in Critical Landscapes in Watersheds
(Multistate Research Project)
Status: Active
S1089: Advanced Understanding and Prediction of Pollutants in Critical Landscapes in Watersheds
Duration: 10/01/2020 to 09/30/2025
Administrative Advisor(s):
NIFA Reps:
Non-Technical Summary
Statement of Issues and Justification
Effective mitigation of nonpoint source (NPS) pollution is difficult because of the spatial and temporal variability of sources and pollutant fate and transport pathways. The cost associated with preventing and/or mitigating NPS pollution is ever increasing. Multiple barrier approaches are now in use by large water authorities to reduce and remove contaminants in upstream watersheds, reservoirs, and groundwater systems. Presently, conservation programs designed to install best management practices (BMPs) to mitigate NPS have not been targeted to those areas of the landscape that contribute to NPS pollution disproportionately. Large reductions in watershed-level nutrient loads could be achieved through coordinated placement of BMPs on high-contributing areas.
In general, selection and placement of BMPs is constrained by several factors, which include a myriad of pollutant transport pathways, heterogeneous landscape features, and variable landscape management. In addition, in agricultural settings, BMPs are often adopted at the farm scale, while desired water quality goals function best at a watershed scale. The objective of BMP implementation plans should be to achieve the maximum pollutant load reduction and minimize the costs. BMP experiments, when properly designed and implemented across a range of spatial scales, provide useful information about drivers controlling BMP design, placement, and maintenance. However, empirical information is intrinsically limited to the specific conditions of the field study and this often hinders generalization of the information gained. Mechanistic models, based on first principles, when properly tested with empirical data help to generalize our understanding of how BMPs may perform under a range of conditions, including extreme events that cannot be directly measured.
Therefore, the placement and optimization of BMPs for controlling NPS requires a suite of individual and coupled mechanistic models that effectively capture a range of factors and watershed processes. The goal of this multistate research project proposal is to explore effective solutions to predict BMP performance (individually and cumulatively) at the various spatial and temporal scales. Tools developed through this effort can be used to inform watershed management decisions and investments in the presence of pervasive and unavoidable uncertainty to achieve water quality while minimizing investment.
Related, Current and Previous Work
Sound watershed management planning is based on the quantitative estimates of the environmental impacts of mixed land-use management. Water quality problems associated with NPS pollution of surface waters has spurred the development and application of a wide variety of best management practices (BMPs) (Ritter and Shirmohamadi, 2000). However, before such practices are adopted, their effectiveness at various spatial and temporal scales must be evaluated through long-term monitoring and model applications (Borah and Bera, 2004; Ackerman et al., 2008; Bosch et al., 2013; Muñoz-Carpena et al., 2020) and in the context of regulatory applications like the Total Maximum Daily Load TMDL (Muñoz-Carpena et al., 2006). Existing models such as the Soil and Water Assessment Tool (SWAT), Hydrological Simulation Program FORTRAN (HSPF), Annualized Agricultural Nonpoint Source (AnnAGNPS), Areal Nonpoint Source Watershed Environment Response Simulation (ANSWERS-2000), Watershed Assessment Model (WAM), and Water Erosion Prediction Project (WEPP) have been used for developing BMP implementation plans. Sharpley et al., 2002 discussed other process-based nutrient transport models such as the Areal Nonpoint Source Watershed Environment Response Simulation—2000 (ANSWERS-2000); Guelph Model for Evaluating the Effects of Agricultural Management Systems on Erosion and Sedimentation (GAMES- 1985); Hydrologic Simulation Program - Fortran (HSPF- 1984); Agricultural Runoff Model (ARM- 1977); Erosion-Productivity Impact Calculator (EPIC- 1990); and the lumped parameter model Generalized Watershed Loading Functions (GWLF- 1987). However, as Ackerman et al. (2008) noted, selecting the appropriate model (tool) when developing a BMP implementation plan and/or predicting or assessing BMP performance should be based on selecting the most appropriate tool given the available input data and the desired application.
In 2014, Giri et al.evaluated the performance of ten best management practices (BMP) in an agricultural watershed in the Saginaw River basin using the SWAT model and four alternative BMP targeting methods. Lee et al (2012) applied an EPA decision-support system tool, System for Urban Stormwater Treatment and Analysis Integration (SUSTAIN), to evaluate alternative BMP implementation plans for stormwater quality management and flow abatement techniques in an urban watershed in Kansas City, MO. Chaubey et al., 2010 used SWAT to evaluate 171 different BMP combinations incorporating grazing and pasture management, riparian and buffer zones, and poultry litter applications. Easton et al. (2008) and Rao et al. (2009) indicated that identifying critical source areas of soil and phosphorus losses was and targeting BMPs in those areas was crucial for achieving water quality improvements Hoss et al., 2016 used the EPA – SUSTAIN model to run 22 years of runoff data from the Patuxent River (Maryland) through seven types of BMPs, and found that BMP effectiveness decreased more rapidly under larger storm events than assumed in the Chesapeake Bay Phase 5 watershed model. Pradhanang et al.(2013) applied the SWAT-WB model to study long term streamflow response to climate change in the New York City watersheds. Mukundan et al. (2013) and Pradhanang et al. (2013) applied a non-curve number model that accounted for saturation excess runoff mechanism to study runoff and sediment distribution under various climate scenarios.
While many models are capable of handling hydrologic and nutrient transport within mixed land use watersheds, some studies have focussed on single land use types. Gao et al. (, 2015) evaluated the performance of the stormwater system in Ma’anshan, China by applying SUSTAIN model for sizing and locating BMPs. Urban BMPs have been studied through monitoring and modeling the effectiveness of stormwater management practices (Im et al., 2013; Her et al., 2017; Seo et al., 2017). These studies often include large structural practices such as detention/retention ponds and constructed wetlands that primarily and smaller BMPs known as low impact development (LID). Mao et al., 2017 used the EPA-SUSTAIN model to assess the ecological benefits of aggregate LID practices for stormwater treatment in Foshan New City, China. When grouped with streams, riparian buffers as a linked network or system, these practices are known as green infrastructure. Green infrastructure (GI) has earned global recognition as the preferred control practice to alleviate the effects of urban runoff. .
Sunde et al., 2018 developed a method for estimating future imperviousness as a function of population growth, and used downscaled climate model projections and SWAT to characterize potential water regime changes in a mixed-use watershed in central Missouri, USA. Alamdari et al., 2017 developed a methodology using an auto-calibration tool to calibrate a previously developed Storm Water Management Model (SWMM) of Difficult Run in Fairfax, Virginia, and then used downscaled global climate models to develop projections of future changes in stream discharge and sediment, nitrogen and phosphorus loads. Tasdighi and Harmel, 2018 presented a probabilistic approach to assess the performance validity of the empirical Curve Number (CN) and physically based Green and Ampt (G&A) rainfall-runoff methods in the SWAT model. Specifically, they explored the effects of modeling uncertainties on characterization of the hydrologic budgets and streamflow regimes at different spatial scales and upstream land use conditions. Regardless of the significant impacts of agriculture on water quantity and quality, limited literature exists describing the compounded impacts of agriculture, rural-to-urban land use change and climate change on future bioenergy crop yields and watershed hydrology.
While integrated water quality models provide the opportunity to analyzing the combined performance of BMPs across a range of spatial and temporal scales, it is important to ensure that the individual BMPs are properly described using process-based models and avoid commonly used simplistic rules or (semi-) empirical approaches that largely increase the uncertainty associated with predictingBMP performance . For example, to evaluate effectiveness of widely used vegetative buffers, empirical exponential equations with length are used, where the coefficients are sometimes selected on the basis of soil, land use or other characteristics. While (semi-) empirical approaches are computationally efficient, their large prediction uncertainty can often negate the value of the intended BMP application (Fox and Sabbagh, 2009). Instead, mechanistic models like VFSMOD, for vegetated buffers (Muñoz-Carpena 1993a-b, 1999), can be used either directly (Muñoz-Carpena et al., 2004) or as the basis for development of simplified stand-alone solutions (Dosskey et al. 2011a,b; Tomer et al., 2009) or components of integrated models like SWAT (White and Arnold, 2009) and others. Importantly, the mechanistic nature of these approaches makes possible the formal assessment of BMP performance uncertainty (Shirmohammadi et al, 2006; Muñoz-Carpena et al., 2007). In addition, the application of objective frameworks for the testing and evaluation of mechanistic models used in assessing BMP performance is a critical requirement for a successful outcome (Ritter and Muñoz-Carpena, 2013; Harmel et al., 2014).
Watershed scientists, planners, and water managers are increasingly using advanced technologies in water quality monitoring not only due to a need to document the rapid degradation of water quality but also to capture spatial and temporal variabilities. Traditional methods for water quality monitoring and modeling are heavily dependent on many stages including on-site data collection, laboratory analysis, and physical and computational models. However, traditional methods are not only costly, they also have spatio-temporal limitations. Because of the limitations of traditional methods, there is widespread interest in continuous on-line monitoring of water quality and hydrologic conditions using advanced sensor technologies across spatiotemporal resolutions. Physicochemical parameters of the water system include parameters such as flow, hydraulic head, temperature, pH, conductivity, turbidity, oxidation reduction potential (ORP), microorganisms, solutes concentration, etc., are easily adaptable to monitoring using online instrumentation and monitoring systems (Jorquera et al., 2010, Dufour et al., 2003; Vaughan et al., 2017; Seybold et al., 2019) . Real time monitoring sensors are widely used in water quality research.
Real-time water quality monitoring includes systems such as the MPC-Bouy system which is applied to the real time monitoring of algae in reservoirs and lakes; the system uses ultrasound for algae monitoring and treatment. The use of microsensors for in-field monitoring of water quality parameters is gaining interest due to their advantages over conventional sensors or methods (Birgand et al., 2007). These sensors can be low-cost (depending on the application), wireless, multi-sensor networks for measuring the physicochemical water parameters; empowering continuous monitoring. The system implements flow, temperature, conductivity and pH sensors from first principles. All the data from the sensors are processed and analyzed, and transmitted wirelessly to a notification node. Machine Learning algorithms can be established to detect possible pollution events.
Previous multistate project was focused on
- developing and evaluating models that can be used to predict the effectiveness of BMPs and BMP implementation at the farm, subwatershed and watershed scales,
- improving and assessing the ability of watershed management models to address emerging environmental issues, and
- developing methods to quantify modeling and monitoring uncertainty as affected by model representations of watershed processes and model input data.
Linking modeling and monitoring to improve BMP design and placement to address pressing water quality issues is critical to mitigate anthropogenic impacts. This project will build on previous iterations of this multi-state effort to advance both monitoring and modeling tools to improve the targeting of BMPs to mitigate NPS pollution of surface waters. A multistate effort is required because of the plethora of BMPs that must be investigated, and because of the site-specific nature of selected land uses and associated BMPs. Successfully completion of this project will reduce the uncertainty and costs associated with BMP implementation efforts and improve local, regional, and national water quality.
Objectives
-
Develop tools that utilize both monitoring and modeling to better inform targeted BMP implementation
-
Advance water quantity and quality models for mixed-use watersheds
-
Test advanced/new monitoring technologies to detect water quality issues, and
-
Conduct integrated assessment of uncertainty and sensitivity analysis of monitoring and modeling approaches both individually and in combination
Methods
The research methods specific to each objective are summarized in the following sections.
Objective 1: Develop tools that utilize both monitoring and modeling to better inform targeted BMP implementation.
Water quality improvements at the watershed-scale due to BMP implementations have been slow and often not as extensive as expected (Meals et al., 2010; Lee et al., 2012; Sharpley et al., 2012, 2013). The lack of progress in addressing water quantity and water quality issues could be due to the overly high expectation of long-term effectiveness of BMPs, the possible degradation of BMP effectiveness over time, and possibly BMPs themselves becoming sources of pollutants. While the need for assessing effectiveness of past and current BMPs have been widely acknowledged, implementation of novel BMPs that are targeted and precision-based is needed. Insights into the processes that determine pollutant fluxes, their fate and transport and the part that each BMP plays in pollutant reduction are needed. In addition to the process of pollutant transport and removal over time and space, it is essential to more fully understand the role that BMP targeting may have in NPS reduction, and at what scale that targeting may be most effective. In order to make this assessment, detailed information about forcing-functions, BMPs specific data, at various temporal and spatial scales are needed.
Monitoring and assessment activities should be considered as an integrated program, rather than monitoring standing alone as separate and distinct, for two reasons. One is that the ultimate value of monitoring is only realized when it is coupled with assessments in which raw data are converted to information that can form the basis of actions. The second reason is that assessment is a crucial step in the adaptive management process. Findings of the assessment process, whether they are statistically robust or are only general indications, should be the trigger to modify future monitoring activities as well as future watershed management activities. Having a strong data analysis and assessment program, with a wide range of tools and products, is vital to the continued success of the BMPs implementation. The products need to include those aimed at regulatory agencies as well as the interested public, decision-makers (including those who decide about future investments of public funds), and the science community.
Therefore the major task for the Objective 1 is to collect data at the BMP, field- and watershed-scale and various time scales as budget allows. Data will include water quantity, water quality (e.g. sediment, nutrients, and pathogens), pollutant removal kinetics, various climatic variables, etc. Data collection will also likely involve measuring fluxes at the soil-air, water-air, and soil-aquifer interfaces to better understand BMP performance. The targeted BMPs for this research include: agricultural and urban BMPs such as riparian buffer zones/filter strips, constructed wetlands, stream-side fencing, sediment detention practices, nutrient management, integrated pest management, denitrifying bioreactors, conservation tillage, infiltration practices, porous pavement, green roofs, grass waterways/vegetated swales, rain garden, soil carbon enhancement, etc.
Objective 2: Advance water quantity and quality models for mixed-use watersheds.
With monitoring data from Objective 1 and previous studies we can use physically and statistically-based predictive modeling to improve the understanding of linkages between driving variables and water-quality outcomes. The driving variables can include land uses, land-use management (e.g., cropping systems, stormwater management systems, waste disposal systems), and climatic factors such as the probability distributions of air temperatures and precipitation amounts. The water quality outcomes can be probability distributions of concentrations or fluxes of water-quality variables in surface water bodies, streams, and reservoirs. The improvement in understanding and planning of watershed management strategies is accomplished through analysis and methods that use the observed weather, hydrology, land use, and water quality data to estimate the parameters of geospatially referenced modelling tools that statistically relate the inputs to relevant water-quality outputs. Novel theoretical approaches to address watershed issues associated with emerging contaminants will need to be better integrated with present models or developed anew. Interdisciplinary cooperation with researchers, watershed specialists, agricultural producers, local stakeholders, agency personnel, etc., will need to be incorporated during the development of novel watershed modeling approaches. The purpose of such models is to better predict water quality responses to the combination of changes in climate, land use, land-use management, and conservation practice implementation that anticipate to occur short or long-term in the future. The models need to be improved to be capable of prioritizing management action plans and investments to help ensure that the highest quality of water is available for use. As the result of achieving this objective, model evaluation, model development, and data collection responsibilities for the participating states/partners will be established. The results will be shared among stakeholders as research progresses. The application of objective frameworks for the testing and evaluation of BMP modeling tools like FITEVAL (Ritter and Muñoz-Carpena, 2013) and that of Harmel et al. (2014) will be a signature feature of this proposal. Therefore the major tasks of this objective are to develop, improve and evaluate process-based models and other approaches for planning and management of mixed land use watersheds.
Objective 3: Test advanced/new monitoring technologies to detect water quality issues
New and advanced technologies that enhance precision measurement both in time and space have now transformed the field of water quality research. High frequency water quality measurements obtained through in-situ sensors enable direct measurement at time and spatial scale commensurate with various physical, biological and socio-economical drivers. While automated data collection and traditional laboratory processing of discrete samples have yielded enormously informative sub-daily data, the transformation of stream and watershed science will occur primarily in response to increasing availability of automated in situ sensors and innovative non-invasive methods such as use of unmanned aircraft vehicles (UAVs). The major task for the objective 3 is to implement high-frequency sensors to collect water quality data at varied time and spatial scales. The UAVs equipped with multispectral sensors/infrared cameras or ordinary cameras will be also used to map fields and watersheds, including land uses, vegetation coverages, crop patterns and other data.
Objective 4: Conduct integrated assessment of uncertainty and sensitivity analysis of monitoring and modeling approaches both individually and in combination.
It is essential to know how modelers present information on model prediction uncertainty and its important controlling factors so that decision makers and stakeholders are better off than they were in the absence of knowledge of this uncertainty. Uncertainty estimates corresponding to measured data can contribute to improved BMPs design and implementation, model application, and informed decision-making. While the results of the first three project objectives (1, 2 and 3) will advance our knowledge about the potential impacts of BMP implementation at various spatial and temporal scales, there still is a large knowledge gap that needs to be addressed. Reckhow (2014) presents a decision analytic approach for using model prediction uncertainty that could serve as a mental framework. This approach is based on the expected value of sample information (EVSI), which can be calculated for a model prediction accompanied by uncertainty analysis. A decision analytic approach when the cost of each land use/land cover, BMPs/model is compared to the cost of the corresponding additional monitoring and/or modeling requirement could also be used in addressing model and monitoring uncertainties. There are many tools that are now used to address monitoring (Birgand et al., 2007) and model uncertainties (Shirmohammadi et al, 2006). In particular, global sensitivity and uncertainty analysis (GSUA) (Saltelli et al., 2004, 2008; Muñoz-Carpena et al., 2007) is a powerful modern framework to include uncertainty in management applications. Therefore the major task for this objective is to analyze uncertainties associated with the monitoring and predictive models at various temporal and spatial scales using GSUA and other state-of-the-art tools. GSUA accounts for all sources of uncertainty, such as model inputs, model structure, model parameters, and measured data. We will conduct uncertainty analyses on model inputs (data from monitoring, forcing data) and outputs to produce a prediction.
Outreach: The results will be communicated to stakeholders and decision-makers in a form usable to these varied audiences, including workshops, webinars, and factsheets. Team members will share with state and local stakeholders research findings especially targeted BMPs for the regions where each member will carry out studies
Measurement of Progress and Results
Outputs
- Objective 1: Comments: ● Data will emerge to better understand BMP performance ● Assessment of tools used for monitoring and modeling of BMPs ● Conference presentations and journal publications on the BMP performance assessed by monitoring and modeling tools
- Objective 2: Comments: ● Cooperation between researchers, local stakeholders, agencies, and agricultural producers ● Data will emerge from water quantity and quality models for mixed-use watersheds ● Improved models for mixed-use watersheds ● Conference presentations and journal publications on water quantity and quality models for mixed-use watersheds; also share results with local stakeholders
- Objective 3 Comments: ● Data from advanced/new monitoring technologies to detect water quality issues ● Conference presentations and journal publications on detection of water quality issues from advanced/new monitoring techniques
- Objective 4 Comments: ● Increased knowledge in decision makers and stakeholders on uncertainty of models ● Assessment of uncertainty and sensitivity analysis of monitoring and modelling approaches ● Conference presentations and journal publications on uncertainty and sensitivity analysis of monitoring and modelling approaches
Outcomes or Projected Impacts
- Objective 1: Improved targeting tools
- Objective 2: Mixed-watershed modeling improvements
- Objective 3: Increased integration and utilization of new water quality sensors
- Objective 4: Better address and communicate uncertainty related to monitoring and modeling An important outcome of the regional project will be increased knowledge concerning the appropriateness of various BMPs for mixed land use watersheds. Another important outcome of the project will be timely and more accurate decision making with high frequency water quality data from the in situ sensors and survey maps and spectral plume maps from the UAVs. The overall outcome of the project will be a healthy and productive watershed with targeted BMP implementation in mixed land use watersheds. The members of the project will collaboratively work on publishing joint research articles on BMP performance and monitoring and modeling methods at the watershed and subwatershed scales. Annual meetings will be hosted to update all the members with their project progress. The ultimate goal of these applications is to ensure that techniques used are based on the best science available and precision BMPs and their implementation are economically and socially feasible.
Milestones
(2021):Regional project meeting to agree upon priority BMPs, emerging environmental issues, advanced technologies, and uncertainty analysis goals for research and identify research teams to address each objective and/or sub-objectives. (1st quarter of 1st year of the project)(2021):Conduct literature reviews on identified priority targeted BMPs for the mixed land-use watersheds, application of high frequency sensors, UAVs and emerging environmental and uncertainty issues for submodel/methods development and testing. (2nd quarter of 1st year of project)
(2021):Identify short and long-term sources of precision BMP performance and hydrologic and water quality data (grab samples and high frequency data) that can be used for BMP assessment and submodel/monitoring development and uncertainty analysis. (3rd quarter of 1st year report)
(2022):Develop collaborative proposals to fund priority BMP, submodel/monitoring development, and uncertainty analysis research. (Year two and thereafter)
(2022):Develop improved protocols and methods for incorporating uncertainty analysis into watershed and subwatershed scale models. (Year two and thereafter)
(2022):Incorporate and validate developed BMP submodels and uncertainty analysis methods into watershed and subwatershed scale models currently being used for watershed management planning. (Year two and thereafter)
(0):Annual project meeting to discuss project progress, additional research needs, new collaborations, and plans for the coming year. (Annually).
(0):Conference with published proceedings and journal articles detailing the results and findings of the project
Projected Participation
View Appendix E: ParticipationOutreach Plan
The ultimate beneficiaries will be land users, home owners, watershed planners, municipal stormwater managers and other stakeholders who will be impacted by the BMPs program. All stakeholders will benefit from potential water quality improvements and landowners and taxpayers will benefit from the development efficient BMPs implementation plans that are more economically feasible. The immediate and direct beneficiaries of the project will be Federal, State and local agencies personnel and consultants. The results of this project will be communicated through the project web page, factsheets, publications of members, annual meetings and reports, and the concluding project conference on Advanced Understanding and Prediction of Pollutants in Critical Landscapes in Watersheds.
This Multi-state proposal includes participants with both research and extension appointments. Both Dr. Gold, of URI, and Dr. Benham of Virginia Tech have extension appointments and oversee active water quality extension programs that use a Logic Model framework with measurable outcomes that are regularly assessed. In addition their programs engage stakeholders in watershed management and best management practices. Stakeholders groups include Conservation Districts, local government, NRCS and watershed organizations. Drs. Benham, Gold and Jaber have a strong network of water quality extension colleagues that coordinated activities closely over the dozen years through programs such as, National Integrated Water Quality Program. Project members will collaborate with USDA-ARS to organize a nationally broadcasted webinar in the last year of the project to summarize the findings of the project and provide stakeholders with resources to increase adoptability of BMPs. Increase in knowledge and adoption willingness will be measured by a retrospective pre- post survey. At annual project meetings, time will be devoted to interactions with extension professionals to create a two-exchange of information. The project participants will share insights and advances and the extension professionals provide feedback and suggestions on how to improve outputs that are useful and accessible to stakeholders.
Organization/Governance
The project’s membership will elect a project chair annually in December. The project chair will be responsible for organizing the annual project meeting and submitting the project’s annual report. The proposal/technical committee consisting of researchers and extension specialists from various land grant institutions (shown below) will serve as the project’s executive committee and will assist the project chair in developing the agenda for the annual meeting and in tracking progress towards achieving the project objectives. The chair elected in the final year of the project will be responsible for submitting the final report. The project’s past chairs and executive committee will assist the current chair with compiling the final report. Administrative guidance will be provided by an assigned Administrative Advisor and a CSREES Representative. The detailed list of the project participants could be found in the project website hosted by the NIMSS.
Member |
Title |
Affiliation |
Expertise |
Objective that fits closely to the expertise |
Soni M Pradhanang |
Associate Professor |
University of Rhode Island |
Hydrology, water Quality, Modeling |
Obj.1,2,3,and 4 |
David J Sample |
Associate Professor/Extension Specialist |
Virginia Tech |
Hydrology & water quality, BMPs, lifecycle costs |
Obj. 1, 2, and 3 |
Aleksey Sheshukov |
Associate Professor/Extension Specialist |
Kansas State University |
Hydrology, Water quality, Modeling |
Obj. 1, 2 |
Fouad Jaber |
Professor/Extension Specialist |
Texas A&M AgriLife |
Urban hydrology, Stream geomorphology |
Obj. 1 and 2 |
Zhuping Sheng |
Professor |
Texas A&M AgriLife |
Hydrogeology, water resources engineering & modeling |
Obj. 1, 2, and 4 |
Saurav Kumar |
Assistant Professor |
Texas A&M AgriLife |
Hydrologic system dynamics |
Obj. 1, 2, 3 and 4 |
Latif Kalin |
|
|
|
|
Rafael Munoz-Carpena |
Professor |
University of Florida |
Hydrological modeling, model evaluation and uncertainty |
Obj. 2, 4 |
Arthur Gold |
Professor |
University of Rhode Island |
Ecohydrology, Monitoring |
Obj 1, 3 |
Kelly Addy |
Research Associate IV |
University of Rhode Island |
Ecohydrology, Monitoring |
Obj 1, 3 |
Brian Benham |
Professor/Extension Specialist |
Virginia Tech |
Hydrological modeling, watershed management planning |
Obj. 2, 4 |
Literature Cited
Ackerman, D. and Stein, E.D., 2008. Evaluating the effectiveness of best management practices using dynamic modeling. Journal of Environmental Engineering, 134(8), pp.628-639.
Alamdari, N., Sample, D.J., Steinberg, P., Ross, A.C. and Easton, Z.M., 2017. Assessing the effects of climate change on water quantity and quality in an urban watershed using a calibrated stormwater model. Water, 9(7), p.464.
Birgand, F., Skaggs, R.W., Chescheir, G.M. and Gilliam, J.W., 2007. Nitrogen removal in streams of agricultural catchments—a literature review. Critical Reviews in Environmental Science and Technology, 37(5), pp.381-487.
Borah, D.K. and Bera, M., 2004. Watershed-scale hydrologic and nonpoint-source pollution models: Review of applications. Transactions of the ASAE, 47(3), p.789.
Bosch, N.S., Allan, J.D., Selegean, J.P. and Scavia, D., 2013. Scenario-testing of agricultural best management practices in Lake Erie watersheds. Journal of Great Lakes Research, 39(3), pp.429-436.
Chaubey, I., Chiang, L., Gitau, M.W. and Mohamed, S., 2010. Effectiveness of best management practices in improving water quality in a pasture-dominated watershed. Journal of soil and water conservation, 65(6), pp.424-437.
Dosskey, M.G., M.J. Helmers and D.E. Eisenhauer. 2011. A design aid for sizing filter strips using buffer area ratio. Journal of Soil and Water Conservation 66(1):29-39. doi: 10.2489/jswc.66.1.2
Dosskey, M.G., Z. Qiu, M.J. Helmers and D.E. Eisenhauer. 2011. Improved indexes for targeting placement of buffers of Hortonian runoff. . Journal of Soil and Water Conservation 66 (6):362-372. doi:10.2489/jswc.66.6.362
Dufour A., Snozzi M., Koster W., Bartram J.,, Ronchi E., and Fewtrell L, 2003. Assessing Microbial Safety of Drinking Water: Improving Approaches and Methods. London, U.K.: IWA Publishing, 2003.
Easton, Z.M., Walter, M.T. and Steenhuis, T.S., 2008. Combined monitoring and modeling indicate the most effective agricultural best management practices. Journal of environmental quality, 37(5), pp.1798-1809.
Fox, G.A. and G.J. Sabbagh. 2009. Comment on Major Factors Influencing the Efficacy of Vegetated Buffers on Sediment Trapping: A Review and Analysis. Journal of Environmental Quality. 38(1):1-3. DOI: 10.2134/jeq2009.0001le.
Gao, J., Wang, R., Huang, J. and Liu, M., 2015. Application of BMP to urban runoff control using SUSTAIN model: Case study in an industrial area. Ecological Modelling, 318, pp.177-183.
Giri, S., Nejadhashemi, A.P., Woznicki, S. and Zhang, Z., 2014. Analysis of best management practice effectiveness and spatiotemporal variability based on different targeting strategies. Hydrological Processes, 28(3), pp.431-445.
Harmel, R.D., P.K. Smith, K.W. Migliaccio, I. Chaubey, K.R. Douglas-Mankin, B. Benham, S. Shukla, R. Muñoz-Carpena, B.J. Robson. 2014. Evaluating, interpreting, and communicating hydrologic/water quality model performance considering intended use: recommendations and review of best practices. Env. Model. & Software 57:40–51. doi:10.1016/ j.envsoft.2014.02.013
Her, Y., Jeong, J., Arnold, J., Gosselink, L., Glick, R. and Jaber, F., 2017. A new framework for modeling decentralized low impact developments using Soil and Water Assessment Tool. Environmental modelling & software, 96, pp.305-322.
Hoss, F., Fischbach, J. and Molina-Perez, E., 2016. Effectiveness of best management practices for stormwater treatment as a function of runoff volume. Journal of Water Resources Planning and Management, 142(11), p.05016009.
Im, S., Brannan, K.M. and Mostaghimi, S., 2003. Simulating hydrologic and water quality impacts in an urbanizing watershed 1. JAWRA Journal of the American Water Resources Association, 39(6), pp.1465-1479.
Jokela, B., 1999. Phosphorus index for Vermont: Background, rationale, and questions. In Annual meeting of SERA-17 Minimizing P Loss from Agriculture. Quebec City, Canada.
Jorquera J.C., Orozco J., Baldi A., 2010. ISFET based microsensors for environmental monitoring, Sensors 2010, 10, 61-83; doi:10.3390/s100100061
Lee, J.G., Selvakumar, A., Alvi, K., Riverson, J., Zhen, J.X., Shoemaker, L. and Lai, F.H., 2012. A watershed-scale design optimization model for stormwater best management practices. Environmental Modelling & Software, 37, pp.6-18.
Lee, M., Park, G., Park, M., Park, J., Lee, J. and Kim, S., 2010. Evaluation of non-point source pollution reduction by applying Best Management Practices using a SWAT model and QuickBird high resolution satellite imagery. Journal of Environmental Sciences, 22(6), pp.826-833.
Mao, X., Jia, H. and Shaw, L.Y., 2017. Assessing the ecological benefits of aggregate LID-BMPs through modelling. Ecological modelling, 353, pp.139-149.
Meals, D.W., Cassell, E.A., Hughell, D., Wood, L., Jokela, W.E. and Parsons, R., 2008. Dynamic spatially explicit mass-balance modeling for targeted watershed phosphorus management: II. Model application. Agriculture, ecosystems & environment, 127(3-4), pp.223-233.
Mukundan, R., Pradhanang, S.M., Schneiderman, E.M., Pierson, D.C., Anandhi, A., Zion, M.S., Matonse, A.H., Lounsbury, D.G. and Steenhuis, T.S., 2013. Suspended sediment source areas and future climate impact on soil erosion and sediment yield in a New York City water supply watershed, USA. Geomorphology, 183, pp.110-119.
Muñoz-Carpena, R. and J.E. Parsons. 2004. A Design Procedure for Vegetative Filter Strips Using VFSMOD-W. Trans. of ASAE 47(5):1933-1941.
Muñoz-Carpena, R., C.T. Miller, and J.E. Parsons. 1993a. A Quadratic Petrov-Galerkin Solution for Kinematic Wave Overland Flow. Water Resources Research 29(8):2615-2627.
Muñoz-Carpena, R., G. Vellidis, A. Shirmohammadi and W.W. Wallender. 2006. Evaluation of Modeling Tools for TMDL Development and Implementation[392KB]. Trans. of ASABE 49(4):961-965.
Muñoz-Carpena, R., J.E. Parsons, and J.W. Gilliam. 1993b. Numerical Approach to the Overland Flow Process in Vegetative Filter Strips. Trans. of ASAE 36(3):761-770.
Muñoz-Carpena, R., J.E. Parsons, and J.W. Gilliam. 1999. Modeling hydrology and sediment transport in vegetative filter strips. Journal of Hydrology 214(1-4):111-129.
Muñoz-Carpena, R., Z. Zajac and Yi-Ming Kuo. 2007. Global Sensitivity and uncertainty Analyses of the Water Quality Model VFSMOD-W. Trans. of ASABE 50(5):1719-1732.
Pradhanang, S.M., Mukundan, R., Schneiderman, E.M., Zion, M.S., Anandhi, A., Pierson, D.C., Frei, A., Easton, Z.M., Fuka, D. and Steenhuis, T.S., 2013. Streamflow responses to climate change: Analysis of hydrologic indicators in a New York City water supply watershed. JAWRA Journal of the American Water Resources Association, 49(6), pp.1308-1326.
Rao, N.S., Easton, Z.M., Schneiderman, E.M., Zion, M.S., Lee, D.R. and Steenhuis, T.S., 2009. Modeling watershed-scale effectiveness of agricultural best management practices to reduce phosphorus loading. Journal of Environmental Management, 90(3), pp.1385-1395.
Reckhow, K.H. 2014. Assessment of the Value of New Information to Inform Water Quality Management Decisions. http://kreckhow.blogspot.com/2014/05/assessment-of-value-of-new-information.html .
Ritter, A. and R. Muñoz-Carpena. 2013. Performance evaluation of hydrological models: statistical significance for reducing subjectivity in goodness-of-fit assessments. J. of Hydrology 480(1):33-45. doi:10.1016/j.jhydrol.2012.12.004
Ritter, W.F. and Shirmohammadi, A., 2000. Agricultural nonpoint source pollution: watershed management and hydrology. CRC Press.
Seybold, E., Gold, A.J., Inamdar, S.P., Adair, C., Bowden, W.B., Vaughan, M.C., Pradhanang, S.M., Addy, K., Shanley, J.B., Vermilyea, A. and Levia, D.F., 2019. Influence of land use and hydrologic variability on seasonal dissolved organic carbon and nitrate export: insights from a multi-year regional analysis for the northeastern USA. Biogeochemistry, 146(1), pp.31-49.
Seo, M., Jaber, F., Srinivasan, R. and Jeong, J., 2017. Evaluating the impact of low impact development (LID) practices on water quantity and quality under different development designs using SWAT. Water, 9(3), p.193.
Shirmohammadi, A., I. Chaubey, R.D. Harmel, D.D. Bosch, R. Muñoz-Carpena, C. Dharmasri, A. Sexton, M. Arabi, M.L. Wolfe, J. Frankenberger, C. Graff and T.M. Sohrabi. 2006. Uncertainty in TMDL Models. Trans. of ASABE 49(4):1033-1049.
Sunde, M.G., He, H.S., Hubbart, J.A. and Urban, M.A., 2018. An integrated modeling approach for estimating hydrologic responses to future urbanization and climate changes in a mixed-use midwestern watershed. Journal of environmental management, 220, pp.149-162.
Tasdighi, A., Arabi, M. and Harmel, D., 2018. A probabilistic appraisal of rainfall-runoff modeling approaches within SWAT in mixed land use watersheds. Journal of hydrology, 564, pp.476-489.
Tomer, M.D., M.G. Dosskey, M.R. Burkart, D.E. James, M.J. Helmers and D.E. Eisenhauer. 2009. Methods to prioritize placement of riparian buffers for improved water quality. Agroforest Syst. 75(1):17–25. DOI 10.1007/s10457-008-9134-5.
Vaughan, M.C., Bowden, W.B., Shanley, J.B., Vermilyea, A., Sleeper, R., Gold, A.J., Pradhanang, S.M., Inamdar, S.P., Levia, D.F., Andres, A.S. and Birgand, F., 2017. High‐frequency dissolved organic carbon and nitrate measurements reveal differences in storm hysteresis and loading in relation to land cover and seasonality. Water Resources Research, 53(7), pp.5345-5363.
White, M.J. and J.G. Arnold. 2009. Development of a simplistic vegetative filter strip model for sediment and nutrient retention at the field scale. Hydrological Processes. DOI: 10.1002/hyp.7291.