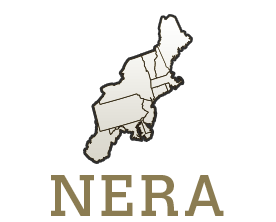
NE1838: Development of a Weed Emergence Model for the Northeastern United States
(Multistate Research Project)
Status: Inactive/Terminating
NE1838: Development of a Weed Emergence Model for the Northeastern United States
Duration: 10/01/2018 to 09/30/2023
Administrative Advisor(s):
NIFA Reps:
Non-Technical Summary
Statement of Issues and Justification
Need for Project
Unlike crops, which have been selected for uniform emergence, weed species have evolved variability in timing of their emergence; even seeds maturing on the same plant may germinate at different times. This “bet-hedging” strategy, with which a weed avoids putting all its “seed in one basket” of emergence timing, enables weeds to escape control measures that are applied at the “wrong” time. Post-emergence management carried out too early, i.e. before most problem weeds have emerged, will yield low returns for the effort, investment, and ecological cost of the management (herbicide off-target effects, soil compaction, etc.), as weed seeds that have yet to germinate are often unaffected. Providing seedling emergence information so that farmers can effectively time their weed management operations can increase efficacy of control, reduce labor costs, and minimize any negative environmental impacts (e.g. reduce the likelihood that repeat applications of an herbicide or cultivation may be required for late germinating/emerging weeds). There is, therefore, an urgent need for the development of time-specific weed management tools to help address the frequently asked, yet to be answered, question of when is the “right” time to control weeds?
Weed seedling emergence is a complex process regulated by a multitude of internal (e.g. species-specific parameters such as base temperature, base water potential) and environmental (e.g. soil temperature and moisture) factors. A range of modeling approaches, varying from simple empirical to advanced mechanistic models, have therefore been adopted to quantify the extent and time of emergence for a significant number of weeds. These can be used to produce weed management decision support tools, which enable farmers to determine the percent emergence of a specific weed species by a given date, taking into account the weather, management actions, and field conditions to that point. Populations of weeds respond differently in different regions to climate and habitat, requiring that emergence models be modified for a particular region. No weed management decision support tool exists for the Northeastern region of the United States, despite recent advances in our understanding of regional weed emergence patterns and developments in fine-scale weather prediction and soil moisture modeling. Data exist to create a weed forecasting product similar to those available for insect and disease threats to Northeastern agriculture, which would enable farmers to approach weed management with more precision and planning. In the past decade, decision support tools have been developed to help farmers manage weeds effectively in the Midwestern United States and Europe; these would serve as a road map for the Northeastern decision support tool. Recent advancements in climate and weather models and computational power have generated detailed weather data that are available to the general public free of charge. In the Northeast, daily weather data are now available on a 4 × 4 km grid across the region using the Applied Climate Information System (ACIS) Web Services (DeGaetano et al. 2014). These databases provide an unprecedented opportunity to estimate parameters directly relevant to seedling emergence such as growing degree day and hydrothermal time, from soil temperature and moisture data at very fine spatial resolution
The overarching goal of this project is to work collaboratively across the northeast region to optimize farmers’ ability to manage weeds in agricultural systems, in the face of challenges posed from a changing climate and increased prevalence of herbicide resistant weeds.
In this proposal, our goal is to develop and validate a user-friendly, online decision support tool for the real time prediction of weed emergence in the northeastern US. The decision support tool takes GPS location, soil type, tillage, crop data, and accesses weather history to provide percent emergence of the farmer’s problem weeds at that location.
Importance of the Work
Weed management is a priority issue for Northeastern farmers, particularly with the increasing prevalence of organic production, the rise of herbicide resistant weeds, and the recent increase in small farms and urban farming. With interest in local food increasing particularly in urban areas, developing methodologies for weed management in the Northeast with specific, regionally-focused data and tools could provide great benefits to local growers and consumers, while reducing negative impacts on the environment. Weed management remains the major challenge for organic farmers that impacts yields (Baker & Mohler 2014; Jerkins & Ory 2016). Yield losses to weed competition are an increasing problem for conventional farmers as well, as the incidence of herbicide resistant weeds continues to increase (Heap 2018). The failure to account for the temporal variability of emergence can result in mistimed application of control measures, with poor control levels necessitating repeated applications that are not only costly to the farmers, but also hazardous to our environment. Better timed and more effective use of herbicides and/or cultivation are likely to delay the development of herbicide resistance and increase yield.
Modeling seedling emergence has long been recognized as a practical approach for tackling the problem of timing in weed management programs (Forcella et al. 2000; Hardegree et al. 2003; Masin et al. 2005). However, after two decades of effort to develop weed emergence models, these models have not been widely adopted by growers or extension specialists and the decision to when best control weeds still involves guesswork to a large extent. We believe limitations due to the availability/accessibility of input data and ease-of-use have been the two major impediments to adopting emergence models. That is, 1) these models have not been presented in a format that can be accessed and used easily by the growers, and 2) the required input data, mainly weather data, have not been available or easily accessible at the proper temporal and spatial resolutions. However, thanks to recent technological and analytical advancements, these barriers no longer exist and we are in a unique position to deliver weed emergence decisions support tools to farmers in a simple and usable format. Further, as increasing variability in weather presents farmers with more extreme and unusual weather patterns, a functional and more site-specific weed emergence model will help farmers better time weed control measures and protect crop yields. Information on the temporal pattern of seedling emergence can also help improve the efficiency of site-specific weed management by guiding the timing of the ground and aerial mapping of weed infestations.
Technical Feasibility
Weed emergence models for a range of weed species already exist, including models developed in the Northeast and Midwest, but no decision support tool has been developed and validated for the Northeast. We have used the emergence models published in Myers et al. (2005) to construct a basic web-based decision support tool pilot (https://alexsinfarosa.github.io/weed-modelV2/), and have tested this tool against two years of emergence data collected in 2016 and 2017 in central New York State (DiTommaso et al. 2018). We have also tested the emergence equations from WeedCast against these data. The two sets of emergence models have bracketed the actual emergence data collected, with one overpredicting early emergence and the other underpredicting emergence. We believe that with the collection of additional data to provide a set of data covering three years from across the Northeast, we can work with these two sets of equations to modify them to fit the emergence patterns observed in our region. We can then use those modified models with our fine-scale weather data, soil moisture prediction tools and fine-scale soil maps to build a Northeast-specific weed management decision support tool. This tool will provide percentage emergence for common agricultural weeds in the Northeast region at a given time based on previous weather conditions and also include short-term weather forecasts to help farmers determine the optimal timing to manage weeds. Weed scientists from across the region are committed to collecting the data necessary to create an effective and easy-to-use decision support product; the proposal includes researchers from Maine, New Hampshire, New York, New Jersey, Delaware, Maryland, Virginia, and California. Dr. Mesgaren from California has expertise and experience in developing and using weed emergence models, and has partnered with this project to provide this critical knowledge to our project. Dr. Art DeGaetano and his team at the Northeast Regional Climate Center developed the pilot decision support tool, and have produced the other weather-based decision support products on the Network for Environment and Weather Applications (NEWA; http://newa.cornell.edu/) website, including apple moth, early and late potato blight, and many other agricultural pest and diseases. Their extensive experience working with existing vector models to develop decision support tools will be crucial to this project. This interdisciplinary group of weed scientists and climate modelers have the expertise and experience necessary to develop a regional weed emergence decision support tool for the Northeast.
Our multistate partners have experience in conducting research on seed germination and seedling emergence of weeds.
Cornell University weed ecologists have been at the forefront of seed burial and movement research (e.g. Mohler 1993; Mohler et al. 2006), and secured funds to initiate the first phases of this project through NE-IPM (“Establishing a Northeastern IPM Weeds Working Group”, a 2-year grant totaling $20,000). Through this project, a network of weed scientists from around the region was convened to: 1) plan the research needed to validate existing weed emergence models for the Northeast, 2) apply for funding to conduct the research and, 3) develop a weed management decision support tool. This group met in December 2017 and January 2018, and developed the project as set forth in this proposal. Dr. DiTommaso also secured funds for the 2016 and 2017 growing seasons to collect pilot data for this project and create the proof-of-concept tool based on the emergence models published in Myers et al. (2005), which can be found at: https://alexsinfarosa.github.io/weed-modelV2/.
This proof-of-concept model was based on data from a project in which another multistate partner, Dr. VanGessel, participated (Myers et al. 2004; Myers et al. 2005). Dr. VanGessel has also conducted research on weed emergence of winter annuals (VanGessel et al. 2015) and horseweed (Conyza canadensis) (Dauer et al. 2007).
Dr. Thierry Besancon has ongoing research focused on environmental parameters that govern germination/emergence of volunteer cranberry (Vaccinium macrocarpon seeds and Carolina redroot (Lachnanthes caroliana, two weeds that are challenging for New Jersey cranberry growers, either because of genetic pollution of the cranberry beds or because of direct competition with the cranberry vines. Dr. Besancon provides New Jersey growers with weekly recommendations on efficient weed management strategies for various specialty crops, which would greatly benefit from data generated by an emergence model for some of the most troublesome weed species. With data from the model, he could provide growers with timely information on mechanical or chemical weed control strategies, improve the efficiency of these strategies by controlling weeds at the most sensitive stage, and reduce reliance on herbicides.
Dr. Jacob Barney has worked on seed germination and biology of weeds impacting cropland and non-cropland ecosystems. In particular, he has extensive experience evaluating the intraspecific variation of hydro-thermal dynamics of weeds. He also has experience evaluating the role of propagule pressure, seed size, and density on weed emergence (Barney et al. 2015).
Dr. Mohsen Mesgaran is a modeler with extensive experience in developing both physiological and statistical models for seed germination and seedling emergence of weeds. Evaluating eight hydrotime time models on four weed species, he found that the most common model in the literature, which assumes a normal distribution of base water potential among seed sub-populations, provides the poorest prediction of germination dynamics (Mesgaran et al. 2013). He therefore developed a new hydrothermal model that not only gives better fits to germination data but also provides useful insights about the adaptive strategies evolved by weed to respond to unpredictable environments (Mesgaran et al. 2017). In collaboration with Dr. Andrea Onofri (Perugia, Italy), he also contributed to development of new statistical methods (e.g. “cure” model; Onofri et al., 2011) to overcome statistical issues arising from the use of traditional methods (e.g. ANOVA) for the analysis of germination data as well as provided guidelines on the proper experimental design for germination/emergence studies (Onofri et al. 2014). He also has experience in developing software for data analytics including the ExDet tool (https://www.climond.org/ExDet.aspx) that enables the comparison of multidimensional climate envelopes across space and time (Mesgaran et al. 2014).
Multistate Advantages
Weather patterns and soil types are highly variable across the Northeast, making the collection of data from across the region critical for developing and refining the hydrothermal weed emergence model. Additionally, Cordeau et al. (2017) found that populations of weed species had different emergence patterns in different Northeastern states; whether that difference is due to genetic variability within the species or plasticity in emergence patterns depending on climatic conditions is not yet known. Thus, it is critical to replicate the same basic weed emergence experiment at all sites across the region. To create optimal weed management solutions that link weed emergence to weather data, several years of data from around the region are required to capture both spatial and temporal variability. Participating researchers will include Eric Gallandt (Maine), Richard Smith (New Hampshire), Mark VanGessel (Delaware), Antonio DiTommaso and Matt Ryan (New York), Thierry Besancon (New Jersey), Bill Phillips (Maryland), Jacob Barney (Virginia), and Mohsen Mesgaran (California). Having thorough coverage of the region will ensure that the model will be useful to farmers throughout the Northeast.
Likely Impact
Optimizing the ability of farmers to manage weeds in agricultural systems, despite the challenges of a changing climate and occurrence of herbicide resistant weeds, is likely to improve farm profitability and reduce environmental impacts of inefficient weed management programs. The weed emergence decision support tool produced by this multistate effort will provide farmers with real-time information on weed emergence in specific fields, enabling them to more effectively target weed management actions and hence increase the efficiency of control measures. The support tool we propose will focus on summer annual species, and will benefit both conventional and organic farmers. In conventional farming, where herbicides are the main means of weed control, our predictive tool has great potential to reduce herbicide use by avoiding applications that are too early or too late to provide any effective weed control. The tool can therefore reduce both costs and negative environmental impacts of multiple treatments and delay development of herbicide resistance. Organic farmers can use the tool to reduce the number of cultivations thus reducing impacts to soil health from disturbance and compaction. For all users, the ability to quantify emergence patterns for common weeds will bring a level of predictability to weed management similar to models used for other crop pests such as powdery mildew, late blight, and apple scab. Although the use of unmanned aerial vehicles (UAV) for mapping weeds has received soaring popularity in recent years, the picture of weed distribution they provide largely depends on the timing of snapshots taken from the field; if captured at the wrong time, the picture may not accurately represent when a critical weed emergence level has been attained. Our tool can reduce the costs (e.g. avoid flights that are too early) and contribute to the success of this growing technology by guiding flight operation timing based on the predicted level of seedling emergence. The proposed tool can also serve as a “time management” system for field operations where growers (particularly new farmers) can better plan their weed management practices based on short-term weather forecasts through the emergence information provided about a potential weed problem.
Related, Current and Previous Work
Many studies have been published on weed seedling emergence rates and patterns. The most practical models for farmer use are based on hydrothermal models; these models map seedling emergence against soil moisture and growing degree days. There are a range of such hydrothermal models available for a range of agricultural weeds (Forcella 1993, 1998; Oryokot et al. 1997; Roman et al. 2000; Leblanc et al. 2004; Masin et al. 2005, 2014; Werle et al. 2014). These models use temperature and precipitation information, and sometime basic soil type and time of plowing, to predict what percentage of specific weed seedlings will have emerged in a given location at a given point in time during the growing season. In general, these regionally specific models have had good predictive ability in their target regions (Grundy 2003; LeBlanc et al. 2004; Martinson et al. 2007; Masin et al. 2012, 2014; Royo-Esnal et al. 2010; Clay et al. 2014; Werle et al. 2014). Some of these works focus on a single species, while a few address a range of agriculturally significant weeds. The Midwestern US decision support tool for weed seedling emergence, WeedCast, uses emergence models based on Midwestern weed emergence data, information on the previous year’s crop, tillage, crop residue, basic soil type, water capacity, and temperature to provide seedling emergence potential, emergence timing, and seedling growth (Forcella 1998). While WeedCast has correlated well with data from the north-central United States, its outputs have not matched as well with data collected in New York State or Delaware (DiTommaso & VanGessel, unpublished). Since the WeedCast decision tool was introduced some two decades ago, its user-friendly interface does not take advantage of current weather products available online; farmers are required to upload individual Excel files for each metric needed, and supply their own soil data. A similar set of emergence models have been developed based on data collected in New Jersey and Delaware (Myers et al. 2005), but no decision support tool was built using these models. In addition, the aforementioned weather mapping advances allow the general public access to fine-scale weather data (e.g. Northeast Regional Climate Center http://www.nrcc.cornell.edu/). Given the existence of a range of weather-based prediction tools on the Network for Environment and Weather Applications website (http://newa.cornell.edu/), development of a weather-based prediction tool for weed emergence by putting existing models and weather tools together and validating them against data collected in the Northeast is a logical next step for better informed farming practices in weed management.
Objectives
-
Link Northeastern weed emergence timing data to existing weed emergence models and modern weather prediction models to create an online tool for farmers that will help them plan their weed management for optimal weed control.
Comments: This tool will include three weeds that are problematic across the region: common lambsquarters (Chenopodium album), redroot pigweed (Amaranthus retroflexus) and large crabgrass (Digitaria sanguinalis). Common ragweed (Ambrosia artemisiifolia) will also be included in the northern portion of the Northeast and morningglory species (Ipomoea spp.) in the southern portion of the region. Individual participating states may also include one additional species of particular interest to their state. -
Collect weed emergence data across the region to validate and refine the existing weed emergence models to fit Northeastern data, and refine the decision support tool through testing by select farmers and extension staff.
Methods
The overarching goal of the project is to work collaboratively across the Northeast to optimize the ability of farmers to manage weeds in agricultural systems, despite the challenges of a changing climate and increasing prevalence of herbicide resistant weeds. Recent advances in weed ecological research and technology in general have opened the door to more targeted weed management decision support tools, and developing such tools will bring weed management in the Northeast into the 21st century.
Objective 1. The emergence model equations published in Myers et al. (2005) were used to create a preliminary emergence prediction tool by fitting existing weed emergence data to precipitation, temperature and soil data for two research farm locations central in New York State. This prediction tool uses the soil temperature model of Bittelli et al. (2015) as presented in their book [Soil Physics with Python, 1st Edition Oxford University Press]. The model is linked to gridded daily temperature and precipitation data via the Applied Climate Information System (DeGaetano et al. 2014). The temperature grid is based on the methods of DeGaetano & Belcher (2007) and the precipitation grid uses the procedure outlined in DeGaetano & Wilks (2009). The model also requires daily evapotranspiration for which we use DeGaetano et al. (1994) to compute pan evaporation, which is then adjusted to bare soil evaporation using Allen et al. (2006). The forecast data are extracted from the National Digital Forecast Database (NDFD) (Glahn & Ruth 2003). The resulting pilot model is available at https://alexsinfarosa.github.io/weed-modelV2/.
While the pilot lacks the ability to alter soil characteristics and soil moisture cutoff mechanisms, it serves as a proof-of-concept for the proposed tool. We have tested the resulting tool against data collected by DiTommaso’s research group in two extreme precipitation years (2016, 2017) in New York State (DiTommaso et al. 2018), and incorporated soil moisture cutoffs from WeedCast (Forcella et al. 1998). At the end of Year 1, data from the Multistate project will be used to test both the emergence equations and assumptions made in the decision support tool and those on which WeedCast was based. We will use the equations for each species with the closest fit and modify them as needed to fit the emergence patterns observed across the Northeast. Data from Year 2 will be used to validate the resulting tool and refine its fit across the region. In addition, a select subset of cooperating farmers and extension educators from each participating state will test a draft of the online decision support tool in year 2. Their qualitative feedback will be used to refine the online interface. In Year 3, data from research and farmer input will be used to further validate and refine the model, and a fully functional version will be installed on the NEWA site (http://newa.cornell.edu/.
Objective 2. All regional collaborators will establish research plots to validate/refine the emergence model. This proposal focuses on warm season annual weeds, which are difficult to control and are projected to become more problematic with climate change. Data will be collected from earliest available crop planting date until the regional date of soybean canopy closure. Dates will vary by site, as the study area extends from Virginia to Maine and annual weather patterns influence planting date. Each site will include two treatments, one with initial tillage in a field with a history of tillage and one with no tillage in a field with a history of no-till agriculture. Eight 1 m2 plots for each treatment will be established, for a total of sixteen plots per site. Either 0.25 or 0.5 m2 subplots will be sampled per plot, depending on the density of weed seedlings. Data will be collected weekly; all emerged weeds of the selected species of interest will be identified and counted, and all emerged plants removed without disrupting the soil (clipped or pinched). Each participating institution will plant a mixture of three species of common interest across the region, selected by the collaborators: common lambsquarters (Chenopodium album), redroot pigweed (Amaranthus retroflexus) and large crabgrass (Digitaria sanguinalis). In addition, southern states will also incorporate morningglories (Ipomoea spp.) into the weed mix, while northern states will plant common ragweed (Ambrosia artemisiifolia). These species were selected by requesting the top five most problematic weeds from the collaborating weed scientists, and selecting the species that appeared most frequently in their lists. All species except large crabgrass are among the Weed Science Society of America’s (WSSA) top ten most troublesome or common weeds, and three species (common ragweed, common lambsquarters, and redroot pigweed) currently have herbicide resistant biotypes in the participating states. Finally, each location will add an additional species critical for their state that is not part of the larger study; these species will be added to the decision support tool for that state.
As the lead institution, Cornell will establish all weed species included in any trial, with the exception of weeds such as Palmer amaranth (Amaranthus palmeri) that are not yet found in locations where the trials will be established. We will conduct site visits to ensure that comparable data are collected across sites. Cornell will repeat the experiment at three sites with a range of soil types, ensuring data coverage for different soil conditions. In Year 2, we will solicit feedback on a draft decision support tool from a select group of cooperating farmers and extension educators to assess whether the model is providing accurate information across the region. This feedback will inform research and model modifications in Year 3.
The cooperating researchers from across the Northeast will meet annually to share data and experiences, refine the study design as necessary, and plan for the upcoming year.
Projected Participation
Cornell University
School of Integrated Plant Science, Soil and Crop Sciences
PI: Antonio DiTommaso – Professor
Co-PI: Matthew Ryan –Assistant Professor
PM: Caroline Marschner –Invasive Species Specialist
This Cornell group will take the lead on this proposal. The team will work at three sites that cover three categories of soil texture, and will establish all the weed species that are used in other states unless they do not yet occur in New York State (e.g. Palmer amaranth). The experiment will be managed by Dr. DiTommaso’s lab, with technical and field support from Dr. Ryan’s staff.
Department of Earth and Atmospheric Sciences
Art Degaetano – Professor
Alex Sinfarosa – Climate Application Programmer
Rick Moore – Research Support Specialist
Keith Eggleston – Research Support Specialist
The team from the Department of Earth and Atmospheric Sciences will be responsible for the modeling and application development portion of the project. Working with NY-IPM research funds, they have already developed a pilot model for weed emergence that incorporates soil temperature, one year of weed emergence data, and a recently developed weather model that uses a four- kilometer grid. They will expand their weather modeling applications to include forecast data, integrate any necessary soil models to accurately predict seedling emergence, and use the weed data collected by participants to test and refine the existing draft model.
New York State IPM program
Bryan Brown –Integrated Weed Management Specialist
Dan Olmstead – Coordinator of the Network for Environment and Weather Applications (NEWA)
NYS-IPM has funded the pilot research that led to the current proof-of-concept model now being tested. Dr. Bryan Brown will provide advice and data to the project. Dan Olmstead’s NEWA program will be hosting the preliminary and final model applications on their website, which currently hosts a suite of similar models for insect pests and pathogens such as apple maggot, downy mildew, and late blight.
University of Maine
Eric Gallandt –School of Food and Agriculture, Professor of Weed Ecology
Dr. Gallandt will be establishing the basic project design, incorporating common ragweed as the northern regional weed of interest.
Virginia Tech
Jacob Barney – Dept. of Plant Pathology, Physiology, and Weed Science, Associate Professor
Dr. Barney will be establishing the basic project design, incorporating the morningglories and adding Johnsongrass (Sorghum halepense). Johnsongrass is present through most of the southeast and mid-Atlantic regions and into New York, and is a serious agronomic weed in Virginia.
University of New Hampshire
Richard Smith – College of Life Sciences and Agriculture, Associate Professor
Dr. Smith will be establishing the basic project design, incorporating common ragweed as the northern regional weed of interest.
Rutgers University Experiment Station
Thierry Besancon – Assistant Extension Specialist in Weed Science
Dr. Besancon will be establishing the basic project design, incorporating common ragweed and adding hairy galinsoga (Galinsoga quadriradiata), a very common and difficult-to-control weed species in NJ vegetable cropping systems.
University of Delaware
Mark VanGessel – College of Agriculture & Natural Resources, Professor and Extension Specialist
Dr. VanGessel will be establishing the basic project design, incorporating both morningglories and common ragweed as both are an issue in his state. He will also add Palmer amaranth (Amaranthus palmeri), a serious problem weed especially in southern states. Most of our collaborators will be unable to include this weed, but Dr. VanGessel’s study site already has Palmer amaranth.
University of Maryland
Bill Phillips – Plant Science & Landscape Architecture, Assistant Clinical Professor
Dr. Phillips will be establishing the basic project design, incorporating both morningglories and common ragweed as both are an issue in his state.
University of California, Davis
Mohsen B Mesgaran – Assistant Professor, Department of Plant Sciences
Dr. Mesgaran will bring weed biology and weed emergence modeling expertise, and help with the experimental design.
Measurement of Progress and Results
Outputs
- Three years of emergence data from seven states on five high-impact summer annual species and a few additional species of importance to individual participating states.
- Both a draft and final model of weed emergence based on temperature and precipitation data. Comments: This tool will incorporate interactions of temperature and precipitation with soil type and integrate both past and short-term forecasted weather conditions.
- A review paper of the current state of weed emergence science. Comments: We will produce a review paper focused on our current knowledge of how weather conditions (temperature and moisture) affect weed emergence levels and timing.
Outcomes or Projected Impacts
- Increased effective weed control for farmers, ideally coupled with a reduction in weed management activities, particularly herbicide use. We anticipate that farmers and extension educators will use the tool to time their weed management activities for optimal weed control within their available window of treatment. In the final year, we will conduct a survey of farmers that have used this tool to assess actual impact, with success defined as a reduction in herbicide applications or cultivations, and/or reported assistance with farm operation planning or reduced problems due to weed populations.
- Better communication of scientifically sound weed management advice to farmers We also hope that this tool will assist extension educators to more effectively communicate scientifically sound weed management advice to their farmers; we will conduct a survey of extension educators that have employed the tool to assess its use for extension activities, with success defined as a reported assistance in delivering specifically targeted weed advice to extension recipients.
Milestones
(2019):Finalize field research plans(2019):Season 1 data collection
(2020):Season 1 data incorporated into draft model; model uploaded to website
(2020):Finalize year 2 field research plans
(2020):Recruit farmers and extension personnel to test draft model
(2020):Complete season 2 data collection
(2021):Incorporate season 2 data and stakeholder input; upload modified model
(2021):Finalize year 3 field research plans
(2021):Outreach to increase model use
(2021):Complete season 3 data collection
(2021):Review paper of the current state of weed emergence science submitted.
Projected Participation
View Appendix E: ParticipationOutreach Plan
The project will produce a tool for use by farmers, extension personnel, crop consultants, and the general public; the web-based weed emergence tool will be housed on the Network for Environment and Weather Applications (NEWA) website (http://newa.cornell.edu/). An integral part of the project is wide participation for the preliminary testing of the model in year 2, and outreach to make stakeholders aware of the final product in year 3. Multistate partners will actively extend their knowledge of the tool and encourage participation and use among stakeholders at a wide range of venues, from grower meetings to extension and scientific conferences. Some of these venues include:
Weed Science Society of America annual meeting
Northeastern Plant, Pest and Soils annual conference
Tri-Society of America annual meeting
New York Cooperative Extension Agricultural In-Service
Delaware Cooperative Extension In-Service
Cornell University Musgrave Research Farm Field Day
Delaware University’s Weed Science Field Day
Empire Vegetable Expo
New York’s Certified Crop Advisor meeting
What’s Cropping Up
Extension Insider (a weekly internal New York extension publication)
Field day / twilight tours / field walks
County extension meetings
Organization/Governance
The multistate research group will have an elected Chair, Chair-elect, and Secretary; we will elect them for the duration of our funded cycle. Administrative guidance will be provided by an assigned Administrative Advisor and a NIFA Representative.
Literature Cited
Allen RG, LS Pereira, D Raes and M Smith (2009) Crop evapotranspiration – Guidelines for computing crop water requirements – FAO Irrigation and drainage paper 56. FAO, Rome 300 (9), D05109
Baker BP, CL Mohler (2014) Weed management by upstate New York organic farmers: Strategies, techniques and research priorities. Ren Agric Food Syst 30:418-427
Barney J, DR Tekiela, MN Barrios-Garcia, RD Dimarco, RA Hufbauer, P Leipzig-Scott, MA Nunez, A Pauchard, P Pysek, M Vitkova, BD Maxwell (2015) Global Invader Impact Network (GIIN): toward standardized evaluation of the ecological impacts of invasive plants. Ecol Evol 5: 2878-2889
Bittelli M, GS Campbell, F Tomei (2015) Soil Physics with Python. Oxford University Press, NY, NY
Clay S, A Davis, J Dille, Lindquist A, HM Ramirez, C Sprague, G Reicks, F Forcella (2014) Common sunflower seedling emergence across the U.S. Midwest. Weed Sci 62:63-70
Cordeau S, RG Smith, ER Gallandt, B Brown, P Salon, A DiTommaso, MR Ryan (2017) How do weeds differ in their response to timing of tillage? A study of 61 species across the Northeastern United States. Ann Appl Biol 171:340-352
Dauer JT, BA Scott, MJ VanGessel, DA Mortensen (2007): Effects of emergence periodicity on growth and fecundity of horseweed. Northeastern Weed Science Society 61:6
DeGaetano AT, KL Eccleston, WW Knapp (1994) Daily Evapotranspiration and Soil Moisture Estimates for the Northeastern United States, NRCC Research Publication RR 94-1. 11 pp DeGaetano AT and BN Belcher (2007) Spatial interpolation of daily maximum and minimum air temperature based on meteorological model analyses and independent observations. J Appl Meteorol Climatol 46:1981–1992
DeGaetano AT, DS Wilks (2009) Radar-guided interpolation of climatological precipitation data. Inter J Climatol 29:185-196
DeGaetano AT, W Noon, KL Eggleston (2014) Efficient access to climate products in support of climate services using the Applied Climate Information System (ACIS) Web Services. Bul Amer Meteorol Soc 96:173–180.
DiTommaso A, CA Marschner, SH Morris, S Cordeau (2018). Weed seedling emergence in two extreme years in Central New York State. Abstracts Annual Meeting of the Weed Science Society of America. January 29-February 1, Arlington, VA. No. 87.
Forcella F (1993) Seedling emergence model for velvetleaf. Agron J 85:929-933
Forcella F (1998) Real-time assessment of seed dormancy and seedling growth for weed management. Seed Sci Res 8:201-209
Forcella F, RL Benech Arnold, R Sanchez, CM Ghersa (2000) Modeling seedling emergence. Field Crops Res 67:123-139
Glahn HR, DP Ruth (2003) The new digital forecast database of the National Weather Service. Bull Amer Meteorol Soc 84:195-201
Grundy AC (2003) Predicting weed emergence: a review of approaches and future challenges. Weed Res 43:1-11
Hargegree SP, GN Flerchinger, SS Van Vactor (2003) Hydrothermal germination response and the development of probabilistic germination profiles. Ecol Model 167:305-322
Heap I (2018) The International Survey of Herbicide Resistant Weeds. Online. Internet. Accessed: July 25, 2018. Available www.weedscience.org
Jerkins D, Ory J (2016) National Organic Research Agenda. Organic Farming Research Foundation: Santa Cruz CA 126 p
Leblanc ML, DC Cloutier, KA Stewart, C Hamel (2004) Calibration and validation of a common lambsquarters (Chenopodium album) seedling emergence model. Weed Sci 52:61-66
Masin R, MC Zuin, DW Archer, G Zanin (2005) WeedTurf: a predictive model to aid control of annual summers weeds in turf. Weed Sci 53:193-201
Masin R, D Loddo, S Benvenuti, S Otto, G Zanin (2012) Modeling weed emergence in Italian maize fields. Weed Sci 60:254-259
Masin R, D Loddo, V Gasparini, S Otto, G Zanin (2014) Evaluation of weed emergence model AlertInf for maize in soybean. Weed Sci 62:360-369
Martinson K, B Durgan, F Forcella, J Wiersma, K Spokas, D Archer (2007) An emergence model for wild oat (Avena fatua). Weed Sci 55:584-591
Mesgaran MB, HR Mashhadi, H Alizadeh, JR Hunt, KR Young, RD Cousens (2013) Importance of distribution function selection for hydrothermal time models of seed germination. Weed Res 53:89-101
Mesgaran, MB, B Webber, RD Cousens (2014) Here be dragons: a tool for quantifying novelty due to covariate range and correlation change when projecting species distribution models. Divers Distrib 20:1147–1159
Mesgaran MB, A Onofri, HR Mashhadi, RD Cousens (2017) Water availability shifts the optimal temperatures for seed germination: a modelling approach. Ecol Model 351:87–95
Mohler CL (1993) A model of the effects of tillage on emergence of weed seedlings. Ecol Appl 3:53-73
Mohler CL, JC Frisch, CE McCulloch (2006) Vertical movement of weed seed surrogates by tillage implements and natural processes. Soil Till Res 86:110-122
Myers MW, WS Curran, MJ VanGessel, DD Calvin, DA Mortensen, BA Majek, HD Karsten, GW Roth (2004) Predicting weed emergence for eight annual species in the Northeastern United States. Weed Sci 52:913-919
Myers MW, WS Curran, MJ VanGessel, BA Majek, DD Calvin, DA Mortensen, HD Karsten, GW Roth (2005) Effect of soil disturbance on annual weed emergence in the northeastern United States. Weed Technol 19:274-282
Onofri A, MB Mesgaran, F Tei and RD Cousens (2011) The cure model: an improved way to describe seed germination? Weed Res 51:516-524
Onofri A, MB Mesgaran, P Neve and RD Cousens (2014) Experimental design and parameter estimation for threshold models in seed germination. Weed Res 54:425-435
Oryokot JOE, LA Hunt, SD Murphy, CJ Swanton (1997) Simulation of pigweed (Amaranthus spp.) seedling emergence in different tillage systems. Weed Sci 45:684-690
Roman ES, SD Murphy, CJ Swanton (2000) Simulation of Chenopodium album seedling emergence. Weed Sci 48:217-224
Royo-Esnal A, J Torra, JA Conesa, F Forcella, J Recasens (2010) Modeling the emergence of three arable bedstraw (Galium) species. Weed Sci 58:10-15
Spokas K, F Forcella (2009) Software Tools for weed seed germination modeling. Weed Sci 57:216-227
Teasdale JR, SB Mirsky (2015) Tillage and planting date effects on weed dormancy, emergence, and early growth in organic corn. Weed Sci 63:477-490
VanGessel M, Z Zhang, T Ilvento, B Scott, Q Johnson (2015) Winter annual weed communities as a result of fall or spring weed control. Weed Sci Soc Amer 68:75
Werle R, LD Sandell, DD Buhler, RG Hartzler, JL Lindquist (2014) Predicting emergence of 23 summer annual weed species. Weed Sci 62:267-279
Wortman SE, AS Davis, BJ Schutte, JL Lindquist et al (2012) Local conditions, not regional gradients, drive demographic variation of giant ragweed (Ambrosia trifida) and common sunflower (Helianthus annuus) across northern US maize belt. Weed Sci 60:440-450