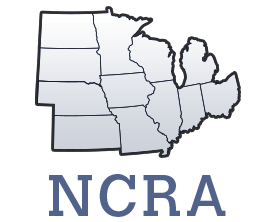
NC94: Impact of Climate and Soils on Crop Selection and Management
(Multistate Research Project)
Status: Inactive/Terminating
NC94: Impact of Climate and Soils on Crop Selection and Management
Duration: 10/01/1999 to 09/30/2004
Administrative Advisor(s):
NIFA Reps:
Non-Technical Summary
Statement of Issues and Justification
Producers need guidance on the relative benefits and probable impacts of soils and climate resources on crop production. This need for planning and decision aids exists in spite of the increase in data availability on the web and the accumulation of research knowledge-and may be labeled the information age paradox. Justification: A literature search of the AgriCola and CAB databases from the early 1970's to the present revealed that assessment of agricultural risks in the United States have generally dealt with economics. Most studies focused on the management of risk associated with price fluctuations, of both inputs and commodities, and the effects of government policy (Fleisher, 1990). Often agricultural economic problems were preceded by extreme weather events. The lack of research on the link between weather and agricultural economic impacts is a void that needs serious attention. United States government policy from World War II to the end of the Cold War was to stabilize food prices and agriculture through the use of price supports and production restrictions during times of food abundance. Beginning in 1995 a major shift occurred in agriculture policy. The Freedom to Farm Act removes planting restrictions and most price supports on the major agronomic crops grown in the North Central Region. Farmers no longer plant a proscribed number of acres of a particular crop to ensure price supports. At the same time they have lost the safety net that comes with price supports and government disaster payments. Is it by chance that these major changes came on the tails of a "benign" weather period from the fifties through the seventies when climate variability was small (Baker et al., 1993)? The loss of the "governmental safety net" exposes producers in the Midwest to greater climate risks. Further, the government programs encouraged production of some crops in areas of the Midwest where the soils and climate were marginally favorable for their growth. Therefore, we propose to define the climate risks of the major crops grown in the North Central Region in the context of the soils, production management practices, and economics of the region. Basic data exits on natural resources and includes measures of the mean and variation in soils and climate. The natural variations associated with climatic events (e.g. El Nino Southern Oscilation, ENSO) are now well documented as well. Generally, historical crop production levels are indicative of weather's impact on crop growth and development. The plants integrate the effects of weather relative to the soils at the observing site and in the immediate area. Recent simulation models estimate the growth and development of crops and insects in a local or field basis. There are economic and weather forecast models as well that add further information to address a complex situation. The producer must determine how to use the different sources of information to increase his profits and conserve his resources. Decisions on crop type, cultivar, planting time, tillage, fertilizer, pesticide, irrigation, harvest, storage, and sale of the crop must be made. This project will bring together the data from natural resources and the simulation models to provide guidance on the relative benefits and probable outcomes associated with agronomic decisions. Our proposal is linked to the crosscutting research priorities set by the North Central Regional Association (NCRA). In the Food Production Area it responds to the 1st Objective; to develop improved plant production systems which are environmentally sound and profitable. In the IPM Area it relates well to the 3rd Objective; refining and developing rapid and positive pest detection and identification techniques to enhance the capability to predict the occurrence and magnitude of pest populations/infestations. In preparation for this work it will be necessary to develop and maintain regional data bases on production systems and develop beneficial uses of these for applications such as modeling and decision support systems (this responds to the Food Production Priority, Objective 5). Because of the pervasive nature of weather, the research team was able to link more NCRA priorities to this work: Natural Resources (Identify ecosystem management principles and practices for utilization and protection of resources and management of rural landscapes); and Economic Development (Create new management decision-making models. Design optimal management systems for cropping systems etc.).
Related, Current and Previous Work
This review focuses on the database required to perform the research, the predictive aspects related to crop production in the region, and the application of simulation models to prioritize management alternatives Data base for Natural Resources The NC-94 Committee developed a Natural Resource DataBase consisting of county level climate-soil-crop production for the North Central Region (Gage and Thomas, 1998; Hollinger, 1995). The previous project focused successfully on the preparation and quality assurance of this database. In addition to the proposed research, the databases on county crop production, soils ,and weather will be maintained and updated. Thus, in this proposed research program, we will focus on developing innovative uses of the Natural Resources DataBase and will interface these data with models and decision support systems. Natural variations in crop yield and the role of ENSO The Midwestern U.S. is a significant producer of agricultural products. Much of the product is exported to other lands. Thus, production becomes an important part of this countries economic balance. The year-to-year variation of corn production and weather throughout the Midwest was large during the last two decades following a relative "benign" period from the fifties through the seventies (Baker et al. 1993). Inter annual weather variation is related to sea surface temperature variation - El Nino /LaNina - (Rasmusson and Carpenter 1983, Ropelewski and Halpert 1985,and others). After accounting for technology, much of the per acre yield variation is connected to anomalous weather (Thompson 1988). Carlson et al. (1996), Mauget and Upchurch (1998), and others have shown significant relationships between ENSO activity in the Pacific and, weather's effect on corn and wheat production in the Midwest. Therefore, we propose to use the Midwest weather, soils, and crop production data assembled by the committee to determine the strength of these relationships with other Midwestern crops. In the previous NC-94 research project, preliminary consideration was given to the role of interdecadal variations in annual precipitation and temperature in the central plains of the U S (Hu et al. 1998). An analysis of interdecadal as well as interannual variations of surface air temperatures in the central plains region was undertaken for a portion of the region (Missouri Illinois, Iowa, Nebraska, and Kansas). We intend to expand this work to the remaining states in the North Central Region. Estimating crop development and production The application of computer hardware and software in agriculture has rapidly increased during the last five years. The introduction of precision farming and related technologies has increased demand for computer programs that process real-time data. Farmers and consultants are now requesting computer models and decision support systems to help them interpret data and make appropriate management decisions. Several models are moving from the research phase to on- farm decision support phase and are being evaluated for on-farm management applications In this project the application of computer models at a regional level is significant because of the existing collaboration between scientists in the region, as well as the regional data base which has been developed during the current regional project. Computer models can be applied in different modes. In a strategic mode prior to planting models provide information about appropriate crops and cultivars to plant, as well as predicting planting dates and other management strategies. In a tactical mode during the growing season models underpin management decisions related to irrigation, fertilizer and pesticide applications. Finally, in a forecast mode, during the growing season, models predict final yield and harvest date. The scale of agricultural model applications is a critical and important issue. Most computer models are point-based systems, in that they only simulate yield and other agronomic parameters for a particular location or site. This is due to the fact that input variables for these models are from point-based, weather stations and soil surveys. For precision farming applications the models are applied at a field scale and normally the models are run for locations in a particular field, depending on the availability of input data. Outputs are then interpolated between points using computer techniques such as kriging. Models can be scaled up from a field application to a farm and watershed application or regional application, such as a county level or crop reporting district. Accurate model inputs are critical for each level, especially weather and soil inputs as well as crop management information. In addition field measurements, including yield and yield components, are important to provide input to local evaluation of the models. An aspect which is ignored in many applications is the selection of the best model for that particular application. Some models, although developed for specific objectives or tasks have been applied out of the original context for which they were designed. A clear example is the use of models to study the impact of climate change. Thus, the evaluation of model performance for a particular application is very critical. In this project a major emphasis will be placed on the proper selection and evaluation of crop simulation models to ensure relevant and applicable models for the region. The performance of a single model is often provided in the literature. However, a comparison of the performance of different models is not common. This regional project is well positioned to play an active role in the performance evaluation of different models for the North Central region. One of the most time consuming factors in model comparisons is the adaptation of the data sets for different models. Most models use specific standards with respect to file formats and naming conventions for weather files, soil files, crop management, as well as output files. Unfortunately they are usually different for each model. In the 1980s the International Benchmark Sites Network for Agrotechnology Transfer (IBSNAT) Project attempted to define a standard format and file structure for crop simulation models. This effort has continued with the International Consortium for Agricultural Systems Applications (ICASA). Hunt and Boote (1998) define a set of file standards which have been implemented in the Decision Support System for Agrotechnology Transfer (DSSAT) (Jones et al., 1998). DSSAT v3 includes more than 15 different crop simulation models, which use the same input files and generate identical output files for model applications (Tsuji et al., 1994). The Global Change of Terrestrial Ecosystems (GCTE) group initiated an effort to compare different crop models. To facilitate this comparison, GCTE decided to use the ICASA file format as their standard for model comparison. Working groups organized by commodity. The most advanced group is the wheat group, because of the economic importance of this crop on a world scale. Several meetings of crop modelers and experimental data collectors have been held to define input data sets for model comparison and to actually conduct these comparisons (Porter et al., 1993; Semenov et al., 1996; Jamieson et al., 1998). A second group is the rice group, which held a model comparison workshop in 1997 in Bogor, Indonesia (Manton et al., 1997). No groups currently exist for soybean and maize, two of the most important crops in the North Central region. A unique approach to model comparisons was used for potato models during a workshop held in the Netherlands in 1990 (Kabat et al., 1995). Potato data sets collected in experiments conducted during the 1980s were provided to potato modelers. To maintain maximum objectivity the modelers provided model outputs to organizers, who then conducted the comparisons between models. Several model evaluations have been conducted using experimental data, including locations in the North Central region (Moulin and Beckie, 1993; Haskett et al., 1995; Kiniry et al., 1997;Boote et al., 1997; Sexton et al., 1998). However, most of these evaluations are based on field plots and point data, rather than regional data. Models have been used in Canada (Dumanski et al., 1996), Australia (Muchow and Bellamy, ed., 1991), the United States (Vanderlip et al., 1995), and the Netherlands (van Keulen and Wolf ed., 1986). Dumanski et al. (1996) also used crop insurance data to evaluate wheat production risks in Canada. However, such an approach has not been utilized in the United States to determine production risks. Modeling approaches have been utilized to evaluate the effects of climate variability and change on specific crops. However, these studies have tended to be focused on small areas and looked only at mean yield changes and not production risks due to climate variability as confounded by economics. A compilation of the variables for potential use in monitoring/modeling the biosphere would not fit in this limited space. Even if we compiled an extensive list, it is not possible or desirable, to measure the state of the atmosphere, soils, and crops continuously in space and time. The reality in that historical in situ measurements are limited to specific observation times, locations, and time periods. The NWS operates a Cooperative Observer Network that was established under the Organic Act of 1890. Currently there are about 12,000 Coop stations in the nation. Observers at these Coop stations measure maximum and minimum air temperature and the accumulated precipitation and snowfall for 24 hour periods. In the 1980-s state local and regional mesonets began to appear which measure more variables to which crops respond-relative humidity, wind speed and direction, soil temperature, and solar irradiance in addition to temperature and precipitation (e.g. Hubbard, et al., 1983). A recent National Research Council report on modernization of the Coop Network recommends that these mesonet stations could reasonably supplement or augment the current Coop Network (NAS, 1998). Decisions on the appropriate size of the Coop Network loom ahead. The NAS report recommends that a determination be made of the minimum number of stations that would meet all anticipated needs of major long term users, including Agriculture. Obviously, the USDA requires reliable and qualitative information on the weather variables and an adequate density of sites to meet their needs. In the past, weather data were summarized for a week, month or growing season and departures from normal used to assess the recent effects of weather. Today, computer-based biological simulation models are used to synthesize weather's effects in light of different management practices and land-use systems on agricultural production and also to assess the impact of these practices on the environment (Engel, et al, 1997). These mondels require maximum and minimum air temperature, precipitation, and solar irradiance as weather inputs. The integration of data from differing networks may be a cost effective alternative to operating completely separate networks. A case study by Ashraf et al. (1997) indicates that a mixture of full and partial weather stations could maintain the spatial accuracy while reducing overall costs The Root mean square error and explained variance (Hubbard, 1994) are two measures of the performance of networks at specified station densities and configurations. Predicting pest problems Annual crop production losses caused by insects, diseases, and weeds have been estimated at about 30% of potential production and has steadily increased despite exponential increases in pesticide use. Agricultural sustainability ultimately depends on controlling these losses without the ever increasing use of chemical pesticides. Integrated pest management (IPM) tenets hold that chemical control of pests should only be used after natura1 controls have been exhausted, but too often IPM has meant, apply more chemical with little regard to the damage done to beneficial insects or the environment. Pesticides have contaminated ground water at locations across the nation. In addition, insect and disease resistance to these chemicals has increased exponentially at ever faster rates, and threatens production of several crops. A thorough understanding of the influence of climate and weather on crop and pest development is essential. Insect and disease pests are controlled by weather conditions which are often cyclic. Thus pests are only a problem in some years so little control is necessary in others. However prophylactic chemicals are applied regardless. The development and use of crop and pest calendars which describe the typical times of crop and insect phenological development will, as effected by weather, ultimately help reduce pesticide use and any associated adverse effects on the environment and humans through better timing of pesticide application. Crop development throughout the season is correlated with the accumulation of heat units during times when air temperatures exceed the threshold temperature for crop growth. Heat units, usually called growing degree days (GDD), have been used for many years in the canning industry to predict the proper harvest time for immature crops (Katz, 1952). It is a simple concept that has been applied in different forms to many different crops. It has even been applied to soybean, a photoperiodic crop whose development is somewhat dependent on day length (Major et al., 1975). The application of GDD to insects is more difficult because air temperature is usually not the controlling variable when the insect is in the soil. Soil temperature and water content may be as important as air temperature, and relative humidity may also play a role. However, despite these difficulties air temperature may still be a good proxy variable and GDD's have been used to successfully predict insect emergence in ND (Glogoza and Weiss, 1997) and corn borer in stars in Minnesota (Baker et. al., 1984). Baker et. al., (1982) developed a crop calendar for corn and soybean development in southern Minnesota using National Agricultural Statistics Service (NASS) survey data. Their figures show the earliest, average, and latest dates that these crops reached each development stage. They also plotted GDD's for corn on the figures, but no correlations were made. No similar calendars for pests were found in the literature. The NC-94 Committee has developed a county level climate-soil-crop data base for the North Central Region (Gage and Davis, 1998;Hollinger, 1995). Daily climatic data within this data base can be used to calculate standard growing degree day (GDD) indices for major crops and pests in the region. These values can be related to crop and pest phenological stages and displayed on GIS systems.
Objectives
-
Update and maintain the NC-94 regional databases on soils, crop production and weather
-
Develop predictive relationships for crop yield in the North Central region as a function of ENSO, SST, and frequencies associated with natural variability.
-
Viewing the optimum crop(s) selection as a dynamic decision on the basis of variability in natural resource patterns in the region.
-
Methods
Objective 1.0 Update and maintain the NC-94 regional databases on soils, crop production and weather. This task will build upon the county-level crops, soils and climate databases developed in the previous project. The crop production and county weather data will initially be extended from1992 to 1998. Then additional years of data will be added as soon as they are available. In addition we will assemble weather data from mesonets for the project. Most crop and other agricultural simulation models use daily weather data from mesonets as input. These variables encompass maximum and minimum air temperature, precipitation and solar radiation (Hunt, 1994; Ritchie et al., 1990; Hunt and Boote, 1998). Models that simulate a detailed energy balance, especially those that use the Penman-Monteith equation, might also require either dew point, relative humidity or vapor pressure deficit as well as wind speed. Models that predict disease occurrence and infestation normally also require weather information that relates to either dew point or relative humidity. For models that predict insect movement and distribution, atmospheric conditions such as wind speed and wind direction are critical. Although most models use daily weather data as input, they might actually have smaller internal time steps to facilitate accurate simulation of growth and development. This is workable at mesonet sites where hourly measurements of temperature, solar radiation, humidity, wind speed and precipitation are taken. At other sites, the models would be forced to use some type of interpolation or other curve-fitting technique to calculate hourly temperature and solar radiation data. For most modeling applications the limiting factor has been the availability of detailed weather information. Normally only daily maximum and minimum temperature and precipitation data are available. Using a different format or method to calculate weather data can have a significant impact on performance of simple weather calculators as well as simulation models (Nonhebel 1994a, 1994b; Watson, 1995). With the expansion of automated weather station networks there is an opportunity to expand the number of input variables for many models, as well as the time scale by moving from daily to hourly inputs. Mesonet and Coop Network data are archived at the High Plains Climate Center and the Midwest Climate Center. For the most part, these data are collected by the researchers proposing this study. This project offers a unique opportunity for researchers to strengthen the applied research on operation, maintenance, and quality control of mesonet data. The county-scale weather, soils and crop production data are stored digitally by researchers from the regional project. Additional data on the rate of crop development will be assembled. The National Agricultural Statistics Service (NASS) in each state publishes weekly estimates of the number of acres(percentages) of major crops that have reached specific stages. For example, data include dates for planting, emergence, jointing, boot, heading, flowering, maturity, and harvest stages for wheat and similar data for other crops. A tabulation of these phenology data for many years willbe obtained from NASS offices in each state. Illinois will lead the team working on Objective 1.0. Participating states are Kansas, Michigan Minnesota, Nebraska, North Dakota and South Dakota. Objective 2.0 Develop predictive relationships for crop yield in the North Central region as a function of ENSO, SST, and frequencies associated with natural variability. Task 2.1 Determining the relationships between ENSO activity in the Pacific and, weather and crop production in the midwest. Carlson et al (1996), Mauget and Upchurch (1998), and others have shown significant relationships between ENSO activity in the Pacific and corn and weather in the Midwest. Therefore, we propose to assemble Midwest weather and additional crop production data and to determine the strength of these relationships for other Midwestern crops. Iowa will lead the team working on Task 2.1 with participation from Illinois, Michigan, North Dakota, Nebraska, and South Dakota. Illinois and Iowa have been working cooperatively to assemble the necessary weather and crop data. Additionally, predictive techniques have been studied so that predictive relationships can be expected fairly early in the life of the project. We will partition the yield data by El Nino, LaNina, and neutral years and study the correlation between ENSO activity in the Pacific and production of corn, soybean, wheat, sorghum, sunflower, etc. in the North Central States. Upper air data have been assembled and computer programs written to analyze the geographical variations associated with ENSO. This type of analysis (Kalnay et al., 1996) will ascertain those synoptic weather patterns associated with El Nino conditions so we can better understand the connection to crop response. Task 2.2 Determine the predictability associated with decadal or longer time scale precipitation and temperature variations in the NC region. The weather data will be analyzed to find any temporal variations of the maximum and minimum temperatures and precipitation over a long time period (1895 to 1995). A power spectral analysis will be run and the results subject to a filter to suppresses signals of periods shorter than 9 years. The technique of wavelet transforms will be applied. Differences in frequencies associated with precipitation will be noted and any tendencies for decadal or interdecadal variations will be recorded. A spatial analysis will result in regional maps from which predominant regional patterns will be observed. Differences across the region will be noted and the results used to provide qualitative models for predicting the next 'dry', 'wet', 'hot', or 'cold' spells. Nebraska will lead the team effort on Task 2.2 with participation from the states of Iowa, Michigan, and Minnesota. Objective 3.0 Viewing optimum crop(s) selection as a dynamic decision on the basis of variability in natural resource patterns in the region. Task 3.1 Comparison of crop models. One of the first tasks is to identify those models recognized for their ability to simulate maize and soybean growth and development. The literature on a number of models is sufficient to warrant their inclusion in the study. The first model is the Erosion Prediction Impact Calculator (EPIC)(Jones et al., 1991; Williams et al. 1989). EPIC was recently renamed to Environmental Policy Integrated Climate (EPIC). EPIC includes options to calculate yield for soybean, maize, wheat, and other crops. The second set of models include the grain legume model CROPGRO (Boote et al., 1998) and the grain cereal model CERES (Ritchie et al., 1998), which have been integrated in DSSAT (Jones et al., 1998; Tsuji et al., 1994). Other models will require further evaluation prior to a decision on inclusion. The modeling team will evaluate the models relative to input requirements, degree of empiricism, and previous performance. The models to be considered are those developed by Sinclair et al. (Sinclair and Muchow, 1995; Muchow and Sinclair, 1995)and by Acock et al. (Haskett et al., 1995; Reddy et al., 1995). The weather information available for the region will be converted into file formats compatible with the individual models. Similarly soil information will be obtained and converted into file formats that can be read by the individual models. In some cases detailed soil information might be difficult to obtain, as several models require soil input data that define a two-dimensional soil profile. The third set of data relates to crop management information, including cultivar planting date, row and plant spacing, irrigation and fertilizer applications, and other management information needed by the models. The models will be run for subregions selected for their unique input conditions with respect to the soil and climate. Specific years will be selected, which represent the climatic extremes to be expected m the region, such as dry and hot years, versus wet and cool years. The models will be compared with available crop development, yield and yield component data, available from the Agricultural Statistics Service and other organizations. Statistical analyses will be conducted to determine significant differences between the performance of the crop simulation models as compared to observed data. Statistical indicators will include the r2, rmse, D-index, systematic and unsystematic mean square error. The models with best overall performance based on these indicators will be selected for each crop and a more detailed performance analysis will be conducted for all the counties and years for which measured data are available. We will identify and document any lack of performance by the models and collaborate with model developers to improve performance if needed. After this careful evaluation, we will apply the models in the other modeling tasks associated with this project. Georgia will lead the team working on Task 3.1 and team members will include Illinois Kansas Michigan, and Nebraska. Task 3.2 Determine the performance of simulation models relative to the source of weather data input (Coop Network or Mesonet) and the spatial density of stations to develop recommendations on future needs for in situ weather measurements in agriculture. Mesonet and Coop Network data are archived at the High Plains Climate Center and the Midwest Climate Center. These data are available to researchers for use in this study DSSAT v3 is a crop simulation system that will be used to simulate historical crop production. Pairs of Mesonet and Coop stations will be selected and the crop production simulated under 'best management' scenarios on a county basis for the time period common to each station. The model outputs forthe two cases (Mesonet and Coop) will be compared in each case to the historical county yieldusing as performance measures the r2, rmse (systematic and random), and the D-index. These station pairs will be selected so as to represent an east-west and a north-south transect in the region. Statistical t-tests will be performed to determine if there are any significant differences in the model outputs from the Mesonet and Coop station simulations. The spatial variability of model performance relative to density of input data will be studied We will run the simulation models for a group of Mesonet stations and calculate the RMSE between model outputs at any two sites (e.g. yield). The RMSE will then be plotted against separation distance to determine to what extent the errors increase with distance of separation. This will be repeated for the Coop stations and combinations of Mesonet and Coop stations. Significant findings in regard to the station density required to maintain reasonable spatial accuracy as determined from these simulations will be reported in light of the future needs for weather data in agriculture. Nebraska will lead the team working on Task 3.2 with participation from Georgia, Illinois, Michigan, and Kansas. Task 3.3 Define comparative performance probabilities for the major crops grown in the region based on the variations in climate, soils, and economics. This task will utilize the crops, soils and climate data bases developed in the previous NC-94project. The goal will be to define homogeneous areas of soil, climate and crop yield variations. We will then utilize the best models identified in task 3.1 to simulate the response of different crop varieties or hybrids in these homogeneous areas. Results will be analyzed to determine which areas are best suited for a specific crop variety or hybrid. In this context the variety or hybrid characteristics to be evaluated are the time it takes the crop to go from planting to maturity and the yield. Other management decisions that will be evaluated relate to crop rotations in an area, planting date management, and harvest decisions. Performance of the crops over time will be compared and probabilities derived for the expected crop yields. Economic models will be used to evaluate how the optimum growing regions may change with different economic conditions. We are expanding the project to include agricultural economists from the region. Kansas will lead the team for Task 3.3 with team members from Georgia, Iowa, Illinois, Michigan, Minnesota, Nebraska, and South Dakota. Task 3.4 Identify major annual features associated with the patterns of crop development and pest development in the region and simulate pest development and infestation in the NC region. Air temperature data in the North Central Region county level climate-soil-crop data base will be used to calculate GDD's for all major crops and pests in the region. These data will be correlated with available data from The National Agricultural Statistics Service (NASS) to create a climatology of crop and pest development across the region. A range of freezing temperature probabilities, i.e., temperatures of 36, 32, 28,... 16 F, for spring and fall will be calculated toprovide levels of risk associated with typical planting and harvesting activities in the region. NASS data for uniform time periods from across the region will be summarized to provide the range of dates during which each crop reached specific growth stages and the probabilities of reaching specific stages by a given calendar date. There are no pest data available from NASS, but some may be tabulated by Universities or State Agriculture Departments which would enhance the project. Patterns of crop developmental stage and pest developmental stage will be overlain each year to identify those situations when potential damage from a pest is high. A GIS application (or published maps) will display crop development progress week by week across the entire North Central Region for any growing season in the record as well as probabilities for each crop. Such information will be invaluable for planning at all levels of agriculture production processing, and marketing. Michigan will lead the team working on task 3.4 with team members from Illinois Iowa Minnesota, Nebraska, and North Dakota.Measurement of Progress and Results
Outputs
Outcomes or Projected Impacts
- See attached "Expected Outcomes"