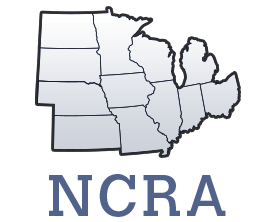
NC1005: Landscape Ecology of Whitetailed Deer in Agro-Forest Ecosystems: A Cooperative Approach to Support Management
(Multistate Research Project)
Status: Inactive/Terminating
NC1005: Landscape Ecology of Whitetailed Deer in Agro-Forest Ecosystems: A Cooperative Approach to Support Management
Duration: 10/01/2002 to 09/30/2008
Administrative Advisor(s):
NIFA Reps:
Non-Technical Summary
Statement of Issues and Justification
As a result of increases in the distribution and abundance of white-tailed deer (Odocoileus virginianus), stakeholders throughout the Midwest and Northeast Regions of the United States incur numerous positive (e.g., recreational viewing, hunting, and associated economic benefits) and negative effects (e.g., crop and forest depredation, vehicle collisions, and disease). Increased knowledge about white-tailed deer ecology and stakeholder values about deer is needed to match the level of acceptable impacts with capabilities of deer management. Specific needs include determining: (1) the landscape scale factors affecting the distribution and abundance of white-tailed deer throughout different types of landscapes; (2) stakeholder defined impacts desired from deer, and factors affecting the willingness of stakeholders to accept impacts from deer; and (3) changes necessary in land management, education, communication, and hunting regulations to enhance the effectiveness of wildlife management.
ISSUES AND JUSTIFICATION
Once rare in many regions of the United States (US), white-tailed deer (Odocoileus virginianus) now represent one of the greatest challenges facing communities and natural resource managers in the 21st century (Warren 1997). What was once a wildlife resource of interest only to hunters and state management agencies, deer now are of widespread interest to the general public.
As a result of events such as herbivory on commercial crops and ornamental vegetation, deer-vehicle collisions, and outbreaks of bovine tuberculosis and Lyme disease, stakeholders throughout the Midwest and Northeast Regions of the US are incurring an array of economic, aesthetic, psychological, and health-related impacts. Concurrently, societal values regarding wildlife have changed over the past few decades and managing wildlife for human benefit (e.g., hunting and viewing) has become increasingly controversial (Muth and Jamison 2000). White-tailed deer are considered a keystone herbivore in agricultural and forested ecosystems by some biologists and ecologists (e.g., Ostfield et al. 1996, Waller and Alverson 1997). White-tailed deer have been implicated in restricting forest regeneration (Tilghman 1989, Campa et al. 1993, Healy 1997), decreasing songbird abundance (DeCalesta 1994), and diminishing the aesthetic attributes of landscapes (Underwood and Porter 1991). Concurrently, deer provide positive economic, recreational, and aesthetic attributes such as recreation from hunting, viewing of wildlife, as well as a significant role in ecosystems functions (Conover 1997). Balancing the positive and negative impacts of deer on the environmental and social landscapes requires increased knowledge about stakeholders and the ecological relationships of deer and their environment.
A great deal has been learned about white-tailed deer in the last few decades (e.g., Halls 1984, Warren 1997). However, the ability to control deer numbers has not kept pace with the growth and expansion of deer populations in most areas (Warren 1997). Very little empirical data exist on deer population distributions and dynamics at large scales, nor have researchers adequately attempted to investigate the ways habitat and population management can affect deer populations at these scales across the Midwest and Northeast Regions of the US. For example, do deer in landscapes dominated by agricultural crops use woodlots as refugia during difficult winter periods (i.e., prolonged deep snow or ice) or as metapopulation activity centers and as a result influence crop production and forest characteristics? In more southern states, because of mild fall and winter conditions, are deer more widely distributed among many different vegetation types, such as residual cropland that provide food and thus have relatively less impact on the regeneration of natural vegetation types? How can deer populations be regulated at the landscape scale under changing human demographics (Brown et al. 2000)? And lastly, can deer population and habitat management programs be designed to affect the distribution and abundance of deer to meet management objectives?
Just as there are questions about how landscapes throughout the Midwest and Northeastern Regions influence deer distributions there are also many questions regarding how landscape characteristics influence deer population structure and demographic processes. For example, how does the distribution of vegetation types throughout the Midwest influence the relative abundance of antlerless deer (vs. antlered) in specific landscapes? What are the cause-specific mortality patterns between sexes and productivity patterns among age classes in various landscapes? These are examples of critical questions that wildlife managers need to address to meet ecological and social management objectives regarding deer impacts.
Wildlife managers are recognizing that management of white-tailed deer is also an extremely controversial societal issue (Swihart and DeNicola 1997). For example, some wildlife management is refocusing its purpose to one of managing impacts of wildlife on people (Decker et al. in review). Impacts are the beneficial and detrimental effects, defined and weighted by human values, of events or interactions involving: (a) individual organisms, populations, and communities, (b) wildlife management interventions, and (c) stakeholder-to-stakeholder interactions vis-`-vis wildlife. Impacts, therefore, are the subset of effects of wildlife-related interactions and events that are determined to be sufficiently important to warrant management attention; they can be considered the priority effects from a management perspective. Events or interactions can be of several types: inter- and intraspecific among wildlife, between wildlife and their habitat, between wildlife and people, between people and wildlife habitat, and among people where wildlife is the reason for the interaction.
The aggregated acceptance of stakeholders or their desire for relief from impacts related to interactions with deer can be manifested in the concept of wildlife stakeholder acceptance capacity (WSAC) (Attachment A, Figure 1). WSAC represents the wildlife population level (or effects from the perceived population) acceptable to stakeholders; it is a function of the perceived benefits and costs associated with a wildlife population (Carpenter et al. 2000). WSAC can provide guidance for wildlife managers who are attempting to balance benefits and concerns of wildlife perceived by stakeholders. However, a serious shortcoming of WSAC considerations in deer management is a lack of detailed knowledge about factors influencing WSAC for different stakeholder groups. By examining several factors that influence WSAC and are influenced by WSAC, this study also will attempt to determine the complex causal relationships contributing to WSAC and acceptability of deer management actions.
White-tailed deer ecology, and the values associated with deer, have been investigated historically within individual states. However, the Midwest and Northeastern environmental and socioeconomic landscapes, and white-tailed deer abundance have changed dramatically in the past several decades. Given these changes, natural resources managers are now challenged with managing an abundance of deer in different areas than they once occurred for many, oftentimes, conflicting human values. For example, conventional methods to manage deer populations, such as hunting, have become infeasible in some areas due to changing human values and land use patterns (Muth and Jamison 2000, Chase et al. in review), and the extent of these areas where conventional methods are not acceptable is expected to increase in the future (Brown et al. 2000, Muth and Jamison 2000).
Research is needed that can contribute to a knowledge base that informs wildlife managers how to better match the level of impacts acceptable to stakeholders with current and future capabilities of deer management. Specific research needs include: (1) a better understanding of landscape scale factors affecting the distribution and abundance of white-tailed deer; (2) a better definition and understanding of stakeholders= willingness to accept impacts from deer; and (3) development of processes to affect necessary changes in land management, hunting regulations, education, and communication to enhance the effectiveness of deer management.
To properly address the research objectives, a multi-state multi-region project is required. A basic premise of this project is that deer population density, demographic vital rates, management, and stakeholder acceptance capacity will vary across a continuum of landscapes. In particular, we expect these ecological and management characteristics to vary with the relative proportion of potential deer habitat, particularly the ratio and types of forests and cropland. Many of these ecological and management characteristics will reflect the state-specific historic pressures to which human and deer populations have been exposed (e.g., deer hunting regulations). Therefore, many of the factors to be examined do not vary enough within one state or are too confounded within the state to allow for valid state-by-state comparisons. To achieve the necessary level of variability and replication of characteristics, large landscapes (i.e., from the Northeastern region of the United States through the Midwest region) are required. Additionally, given the size of the required study sites and costs associated with collecting data on these sites, no single state can afford the required replication (even if it existed).
The goal of this project is to improve the capabilities of state wildlife management agencies, local governments, and other stakeholders to make decisions about the management of white-tailed deer throughout their northern range in the United States. To achieve this goal, improved understanding is needed about the effects of landscape characteristics on the biological, environmental, and human dimensions of white-tailed deer management, and the components needed for effective outreach to stakeholders. Initiating a multidisciplinary collaboration among researchers that addresses these specific needs is essential to comprehensively assess and manage the white-tailed deer in diverse regions throughout the United States. The committee members in the proposed study are leaders in their respective fields of wildlife biology and human dimensions of wildlife management. Adaptive impact management (AIM), developed by several of the members, can serve as a framework for guiding the collection of relevant data and providing meaningful assistance to natural resource managers (Riley et al. in review). One aspect of this multidisciplinary, multi-state project is to modify research designs of many similar but geographically separated studies to develop new and comparable data sets. An opportunity to gain fresh, innovative insights, and to affect large-scale change in wildlife management will be created if this project is implemented.
Related, Current and Previous Work
A search of the Multistate Regional Project CRIS database identified only 1 project pertaining to white-tailed deerCNCT-185, the project from which this proposal was developed. A full text search of the CRIS database yielded 114 projects that pertained to white-tailed deer to some extent. None of the identified projects, however, involved quantifying variations in deer landscape use patterns, demographic characteristics, and social components of deer management across a gradient of landscape types (i.e., agriculture dominated to various agro-forest dominated landscapes; we define agro-forest landscapes as those composed of a mix of agriculture crop types and forest vegetation types). The projects that were described for individual states are addressing some of the similar habitat, population, or human dimension components as we propose to investigate (e.g., deer population demographics), however, none of the projects listed have addressed or integrated the results from the three components and none of the studies are linking results and analyses among regions in the US. All of the investigators that will be involved with this project (Attachment B) have an extensive amount of research experience with the ecological or social dimensions of deer management within their respective institutions.