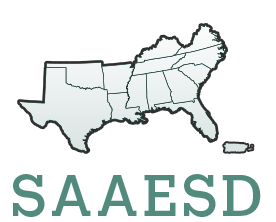
S1098: Autonomy for Agricultural Production, Processing, and Research to Advance Food Security through Sustainable and Climate-Smart Methods
(Multistate Research Project)
Status: Active
S1098: Autonomy for Agricultural Production, Processing, and Research to Advance Food Security through Sustainable and Climate-Smart Methods
Duration: 10/01/2024 to 09/30/2029
Administrative Advisor(s):
NIFA Reps:
Non-Technical Summary
Food security, food safety, and agricultural sustainability depend on the development of autonomous agricultural machine systems (i.e., agricultural autonomy) to overcome the lack of labor and optimization that currently occurs in agricultural research, production, and post-harvest processing. The goals of this project are as follows: (1) to develop autonomous systems for research, such as in phenomics for plants and animals; (2) to develop autonomous systems for agricultural production of plants and animals, such as in soil preparation, planting, weeding, disease management, insect management, harvesting, animal tracking, animal herding, machine navigation, and multi-machine coordination; and (3) to develop autonomous systems for agricultural processing such as in classification, sorting, and cutting of produce and meats. Target audiences will be (a) crop and animal breeders who will benefit from new tools that will enable them to be more efficient in their work and to have heretofore unavailable knowledge about how to make genetic improvements; (b) farmers and ranchers who will benefit from new tools that will enable them to be more efficient and sustainable in their operations; (c) post-harvest processors who will benefit from new tools that will enable them to be more efficient in their operations; (d) U.S. consumers who will continue to have abundant, safe, sustainably produced, and reasonably priced food products. Project activities will be focused on research on and development of these tools, which will provide new opportunities to researchers, producers, and processors within the next decade.
Statement of Issues and Justification
The need, as indicated by stakeholders
To meet the food demands of the world’s growing population – 8 billion today, projected to be 10 billion in roughly 30 years – U.S. agriculture needs autonomous field machines to optimize crop growth at a detailed level (i.e., precision agriculture) to keep farming sustainable with an ever-shrinking number of farm laborers. Some autonomy has been available on farms for over 20 years; e.g., automatic guidance on tractors. However, society has not yet accepted fully autonomous vehicles, and machinery manufacturers are slow to produce them, largely due to potential liability issues, lack of clear return on investment (Erickson and Lowenberg-DeBoer, 2022), etc. Technology gaps centered on sensing and decision-making currently prevent industry from having confidence in full autonomy for multiple functionality and safety reasons. The proposed multistate research project aims to address key sensing, artificial intelligence (AI), and robotics barriers to implementing intelligent autonomous agricultural machines and enabling precision agriculture in the face of the farm labor shortage. Our vision is that small and large farms of row-crops, specialty crops, and animal production will be able to transition from large human-driven equipment to full integration of compatible AI-based autonomous machines (agricultural autonomy, or A2) that will drastically increase the capacity of the human machine operator. The research project’s outcomes will pave the way toward the transition to teams of smaller, fully autonomous, machines, both on the ground and in the air. These machines could conduct most aspects of crop production, including scouting, planting, fertilization, pest (weeds, insects, diseases) control, and harvesting, at high spatial resolution, informed by autonomously acquired data and AI-based decision tools. We believe this transition will also benefit post-harvest processing and even agricultural research. The ultimate outcomes of the project will be (a) more prolific, profitable, and sustainable farming methods to meet world food and fiber demands; (b) safer and less labor-intensive processing systems based on agricultural-autonomy for grading, sorting, cutting, etc.; and (c) new autonomous research machines and systems that enable accurate field and greenhouse data to be collected with minimal human involvement and also enable the measurement of properties not previously measurable.
While A2 will likely ultimately eliminate the need for some machine operators, the aim is to keep farmers and farm workers in the loop and to assist them in enhancing farm profitability and sustainability. Moreover, farm managers’ expert knowledge should be captured and incorporated into machine learning to improve decision-making and operations. The average age of the U.S. farmer is approaching 60 years, so the time is critical to capture and maintain industry expertise before it vanishes. The farm tasks to be conducted autonomously will require large volumes of data (e.g., on insects in the field, weeds, diseases) collected by various platforms including ground-based and aerial robots and possibly stationary sensor networks. All these data must be analyzed and recommendations relayed to farm managers in an understandable and trustworthy way for strategic decisions. In later stages of development, the data must be analyzed for real-time tactical decisions by autonomous vehicles, which can be terrestrial and/or aerial, working together as a team to undertake the prescribed tasks in an efficient manner. All this sensing and analysis must be accomplished in rural and remote farm fields where broadband wireless infrastructure will be limited or non-existent for the foreseeable future.
The importance of the work, and what the consequences are if it is not done
While the American people make up just over 4% of the world’s population (U.S. Census Bureau, 2020), American row-crop farms produce about 33% of the world’s soybeans, 30% of corn, 15% of cotton, 8% of sorghum, and 8% of wheat (Our World in Data, 2022). The U.S. exports tremendous amounts of these commodities to feed and clothe the world, and the global population is expected to increase by roughly 25% in the next three decades (United Nations, 2022). Furthermore, rising living standards worldwide raise the demand for animal protein, which adds an additional requirement for grains as feed. On top of these worldwide demand pressures, expectations for environmental risk mitigation and sustainability are increasing, requiring that crop inputs be reduced. Moreover, a changing climate adds uncertainty to future yield capabilities, and high-quality farmland is being lost to urbanization and road construction. The farm labor shortage is an exacerbating factor. Aside from the aging of farmers, rising living standards are reducing the desirability of farm work among the world’s young; the average age of immigrant farmworkers in the U.S. rose by 7 years between 2006 and 2021 (USDA Economic Research Service, 2023). Moreover, immigration issues worldwide are reducing the flow of migrant farmworkers. A2 is the solution to the farm labor shortage and aging.
Autonomy is also an enabler of precision agriculture (PA), which holds great promise to help address the demand challenges. A recent study on potato production found that PA increased economic profitability by 21% and “social profit,” a term used as an overall measure of sustainability, by 26% (van Evert et al., 2017). PA optimizes inputs such as seed, fertilizer, irrigation, herbicide, and pesticide on individual zones in a farm field or even individual plants, thus increasing per-acre yield and using inputs more efficiently. The finer the scale, the more optimization is possible. Sensors, analytical tools, and electromechanical devices are used to make and implement these zone-specific optimization decisions. Mechanization has drastically improved the efficiency of individual farmworkers, but there is no room to further increase the size of tractors and harvesters, which have become huge, expensive, and heavy to the point of damaging crop performance by compacting the soil. Therefore, the principal remaining tool to solve the issues of labor and finer precision in PA is autonomy. PA can benefit from autonomy by having sensors on autonomous vehicles and platforms collecting farm data, computing devices determining whether to apply a specific input at a particular location, and electromechanical actuators converting the decisions into action. With the amazing advances that have been made in AI and robotics in the last few years, A2 now appears as a solution to optimizing production by increasing yield and reducing environmental risk at an extremely high level of precision, potentially even at the single-plant level, while counteracting the downward trend in available farm labor.
A long-term major collaborative effort is required to develop the multifaceted AI and capabilities needed to enable the U.S. to lead in A2.
The technical feasibility of the research
Compared to other autonomy efforts, the level of complexity in agriculture is very high. Farm fields require navigation over large areas with no established roads or signs, varying and challenging terrain, many types of obstacles, and rapidly changing surroundings like soil conditions, plant sizes, etc. The operating environment for autonomous agricultural machines will be particularly harsh, with wide variations in temperature, solar radiation, precipitation, humidity, and dust and dirt accumulation. The number and variety of objects and conditions that must be classified and quantified is large. For example, autonomous machines may be required to quantify the level of plant health; differentiate between weeds and crop plants and between various species of weeds; detect, identify, and quantify diseases and insects; and differentiate among soil conditions in terms of both fertility and texture. The ability of autonomous machines to communicate, particularly in a data-heavy environment as will be the case when imaging becomes commonplace, will be challenged by the lack of broadband connectivity in most rural and remote farm fields. Thus, edge computing will be a major component of A2. As opposed to on-road autonomous vehicles, for which the decisions are basically limited to speed and turning angle, the decisions required of autonomous agricultural vehicles will include navigation as well as numerous actions involving applying assorted farm inputs at various locations and in various amounts.
All this being said, the advancement of sensing devices, computational hardware and software, motors and mechatronics, IoT, wireless communications, etc., has recently enabled rapid development of autonomous systems, even for applications like agriculture.
The advantages for doing the work as a multistate effort
The multistate team is expected to be composed of investigators from institutions with a strong record of research and innovation in important elements of A2. Mississippi State University, University of Florida, Penn State University, Washington State University, University of California-Davis, etc., all have viable programs in autonomous systems for agriculture as well as strong faculty cohorts who are experts in agronomic, socioeconomic, and environmental aspects of production agriculture.
Different regions have different climates, crops, cropping systems, etc. Within regions a multistate research project can bring about more rapid progress due to collaboration and idea sharing on similar problems. Among regions, novel ideas from one application can spur innovation in other regions where novel techniques may be applied in different ways or on different crops.
What the likely impacts will be from successfully completing the work
Outcomes of this research will be advanced farming systems that enable highly optimized food production for food security and environmental sustainability. Socioeconomic studies will produce knowledge and recommendations about how A2 affects various farm scales. These outcomes will increase U.S. economic competitiveness by improving worker productivity.
Related, Current and Previous Work
Agricultural robotics has recently received considerable attention for crop production, especially in weeding and harvesting (Shamshiri et al., 2018; Yang et al., 2023). Over the past four decades, significant research has been conducted to develop harvesting robots as an alternative to methods requiring human action, with most of the focus on specialty crops (e.g., fruits and vegetables) (Bac et al., 2014; Barnes et al., 2021; Fue et al., 2020; Grift et al., 2008; Li et al., 2010; Li et al., 2011; Williams et al., 2019). Some research has also been done to consider swarms of small harvesting robots that could potentially replace large conventional harvesters (Gaus et al., 2017). Cotton harvesting provides an interesting example of improvements that could be made with the introduction of robotics. Aside from minimizing the need for scarce labor, using robots to harvest multiple times, instead of conventional cotton harvesters that harvest only once at the end of the season, could improve fiber quality and mitigate the need for chemical defoliation, and it could reduce yield losses caused by late-season extreme weather (Barnes et al., 2021). However, despite the expected advantages of robotics to address current and future issues (e.g., labor shortages, work inefficiency, and negative environmental impact), commercialization and adoption rates are low, because prototypes have commonly not been competitive with human labor in terms of cost, reliability, safety, and efficiency (Oliveira et al., 2021).
While it has been predicted that automation will move agricultural production to a highly efficient and productive, low-labor, sustainable industry (Grift et al., 2008), many agricultural robot prototypes are a long way from being competitive with human labor (Li et al., 2011). Required robotic improvements include task simplification, performance enhancement, clear evaluation metrics, and consideration of socioeconomic factors. Robot performance needs to be evaluated in real application scenarios with well-defined metrics, and some research is beginning to consider this fact. For example, a multi-arm kiwifruit harvester was shown to achieve a harvesting rate of 70.0%, but for commercial farms the drop rate of the fruit would have to be reduced through improved gripper performance (Williams et al., 2019). Significant improvement in harvest success rate and reduced cycle time have occurred in the last few years.
A robotic harvester can be considered as a collection of components (e.g., fruit detection, fruit localization, manipulation, gripper, servo control, mobile platform, material handling, etc.) whose individual efficiencies dictate the overall economics of harvesting. In robotic harvesting, not to mention navigation and weeding, image-based object detection is common in order to autonomously position the robot in relation to fruit for successful detachment based on measurements provided by the vision system. Recent advances in machine learning have paved the way for superior object detection algorithms based on deep learning (DL) techniques. Fruit detection and localization methods are at the core of automated yield estimation and robotic harvesting. The accuracy and efficiency of these methods can significantly impact the economic viability of robotic harvesting solutions. With the integration of DL models for navigation, detection, and sensing modules, and ongoing advancement of flexible, bio-inspired end-effectors, robotic harvesters will be able to match the accuracy and speed of human labor in the foreseeable future (Yang et al., 2023).
One team member has developed multiple UGV platforms for on-farm precision-agriculture applications. One, called weedbot, conducts real-time weed/crop object detection in field conditions. These platforms collect hyperspectral, RGB, and video data simultaneously.
Other multistate research projects are conducting related research. S1069 is focused on the use of UAS for various purposes like plant-height estimation, and W4009 is focused on sensors and automation for specialty crops. While there may be some overlap between these other projects and S10XX in terms of automation, the focus of S10XX is not on the applications of phenotyping or specialty-crops but on the tools being developed for autonomous navigation, multi-vehicle collaboration, multi-DOF arm movement, end-effectors, and so forth, which can be used for those purposes. Furthermore, S1090 is conducting research in the use of AI for crop status/growth monitoring, yield estimation/prediction, stress detection, and quality evaluation. In S10XX, the team intends to conduct research that includes AI in more sophisticated systems of autonomous machines. These machines will facilitate advances in the capabilities of research in, for example, crop growth monitoring. For instance, it may be beneficial in a plant phenotyping operation to not only gather remote-sensing data from an aerial robot (i.e., “drone” or “UAV”) flying at 400 ft above ground level, but also to gather proximal data from underneath the plant canopy and soil at the same time. One can imagine a coordinated approach between one or more UAVs and one or more terrestrial robots collecting the ground-level data. Such advances in autonomous systems are central to this multistate research project, in which the goal is focused on developing systems rather than collecting data. To put it another way, the focus of S10XX is on developing tools and not on using them over the long term. Finally, NCERA180 is focused on precision agriculture. Whereas autonomous systems are an enabler of precision agriculture, NCERA180 is focused on the use of precision tools, while S10XX will be focused on the development of tools. Also, the purpose of the tools developed in S10XX may include increased precision, but such tools will be autonomous, mitigating the need for scarce labor.
Objectives
-
Research and develop advanced autonomous systems for research, such as in phenomics for plants and animals.
Comments: This is not simply using drones and cameras or some other commercial off-the-shelf autonomous system to measure plant and animal characteristics. The focus here is on creating research tools including platforms and sensors to advance autonomous systems for measuring plant and animal characteristics. Sub-objectives: a.advanced autonomous systems for accurately measuring phenotypes and other activities to improve the efficiency and effectiveness of plant-based research.; b. advanced autonomous systems for accurately measuring phenotypes and other activities to improve the efficiency and effectiveness of animal-based research. -
Research and develop advanced autonomous systems for crop and animal production.
Comments: Sub-objectives: a. improved autonomous navigation in the field; b. coordination of multiple autonomous machines in the field; c. autonomous systems for real-time decision making and actuation, such as in management of weeds, diseases, insects, and nutrients; d. autonomous harvesting. -
Research and develop autonomous post-harvest processing systems.
Comments: Sub-objectives: a. autonomous classing and sorting systems for plant produce and animal products; b. autonomous systems for processing of plant produce and animal products such as cutting and trimming. -
Research the socioeconomic impacts of autonomy on various farm scales and make recommendations about potential business models.
Methods
The intent of this multistate project is to approach each objective in an integrated fashion in which multistate teams address each, with the expectation that the overall multistate team will share progress and new knowledge across subobjectives.
Objective 1: Research and develop advanced autonomous systems for research, such as in phenomics for plants and animals.
Objective 1 is focused on developing tools and techniques for researchers, including in phenomics, and it has two subobjectives that are naturally interdependent. For example, the spectral and spatial characteristics of image data have similar features regardless of whether the object under study is plant or animal, so new knowledge developed under one subobjective will often have application under the other.
Obj. 1a. Advanced autonomous systems for accurately measuring phenotypes and other activities to improve the efficiency and effectiveness of plant-based research.
Introduction
Research in plant breeding is critical to produce higher yielding crop varieties to maintain food security and to produce more resilient varieties because of climate change and related stresses. Considering more genetic variability in breeding increases the likelihood of improvement in plant traits, so it is important to phenotype more genotypes of a given plant than has been done in the past. Furthermore, agricultural labor, even in research, is increasingly difficult to find, so phenotyping approaches that minimize labor are increasingly important. Finally, new sensing technologies facilitate the attainment of new knowledge about plants and often cannot be applied effectively manually. In summary, plant breeders need autonomous systems that collect phenotypic data faster than humans, that are available when human labor is not, and that can appropriately apply advanced sensing technologies to gain new insights that are unattainable by other means. In addition to breeding research, agronomic plant-based research can also benefit greatly from autonomous systems. For example, autonomous systems for rapid collection of leaf or soil samples would be of great value.
Imaging and spectroscopic techniques play a pivotal role in understanding and characterizing plant phenotypes. These phenotypes encompass a wide range of traits, including anatomical, ontogenetical, physiological, and biochemical properties of plants. Characterizing these traits enables a more comprehensive view of plant performance, which should be possible by non-destructive and high-throughput means. Various spectroscopic techniques in terms of their modes of operation (ATR, DRIFT, and Raman) and wavelength ranges (Visible, near infrared, shortwave infrared, and mid infrared) have been used to obtain plant phenotypes, but not all techniques are being actively used for autonomous phenotyping due to challenges with integrating instrumentation and autonomous platforms.
Detailed activities/procedures
Plant phenotypes can include canopy reflectance, plant height, etc. However, this project will focus on more advanced phenotypes including Raman spectroscopy, and how to apply complex sensors to crop plants with an autonomous platform. Novel spectroscopy-based sensors for plant phenotyping will be developed which can be easily integrated with autonomous systems to be used under greenhouse or field conditions. Plant leaf sensors based on ATR and DRIFT spectroscopy in different wavelength regions (Visible, near infrared, shortwave infrared, and mid infrared) will be designed, fabricated, and tested on different crops to obtain and store spectra. Such sensing systems can serve as end-effectors of robotic manipulators which can be used for autonomous plant phenotyping.
Atefi et al. (2019 and 2020) recently developed “phenotyping robots” that were able to probe the leaves and stems of corn and sorghum plants in a greenhouse. Specialized sensors were designed and assembled onto the robotic manipulator to measure key plant traits including leaf temperature, chlorophyll content, spectral reflectance, and stem thickness. A vision system comprised of RGB and depth cameras was used to localize the plant leaf and stem and drive the robotic end effector for probing, grasping, and sensing. In this project, this robotic system will be further developed for the field phenotyping of corn, sorghum, and soybean. The robotic manipulator and the cameras will be mounted onto a Husky rover platform, and navigation strategies will be developed to allow the system to move through experimental plots autonomously. Compared to the greenhouse, field environments present additional challenges for the computer vision system. Naturally varying illumination, tightly packed plants, and complex background can cause failure in the rule-based image processing algorithms for target leaf/stem identification and require development of deep-learning based algorithms for this purpose. Microclimate sensors (solar radiation, air temperature, relative humidity, and vapor pressure deficit) will be mounted on robotic platform to complement the phenotyping data collection. When corn plants are tall in the late season, the robotic platform will be able to navigate under canopy and to measure under-canopy microclimate variation a new specialized sensor for leaf stomatal conductance will be designed based on commercially available handheld porometers to enable measurement of this key physiological trait autonomously in field conditions.
Unmanned ground vehicles have been widely used to collect phenotypic data in the field. A robotic system has been developed to collect side-view images of tomato plants for tomato disease phenotyping under field conditions. The system can efficiently maneuver through tomato crop rows, which typically have 36-inch row spacing. This dimension aligns with standard spacing for other vegetables like pumpkins and fresh-market cucurbits and brassicas. The system is equipped with a sensor mast capable of carrying cameras and GPS devices for georeferenced image data, and a customized stereo imaging system with strobe lighting was developed for high-resolution 3D reconstruction relatively unaffected by variable lighting under field conditions. With self-navigation functions enabled by GPS and local range sensors, the system allows autonomous operation in unstructured environments and mapping to within 3 cm accuracy.
Objective. 1b. Advanced autonomous systems for accurately measuring phenotypes and other activities to improve the efficiency and effectiveness of animal-based research.
Introduction
Research in animal phenotyping is critical to produce higher yielding and more resilient animals. Considering more genetic variability in breeding increases the likelihood of improvement in animal traits, so it is important to phenotype more genotypes of a given animal than has been done in the past. Furthermore, agricultural labor, even in research, is increasingly difficult to find, so phenotyping approaches that minimize labor are increasingly important. Finally, new sensing technologies facilitate the attainment of new knowledge about animals and often cannot be applied effectively manually. In summary, animal scientists need autonomous systems that collect phenotypic data faster than humans, that are available when human labor is not, and that can appropriately apply advanced sensing technologies to gain new insights that are unattainable by other means. In addition to breeding research, other animal-based research can also benefit greatly from autonomous systems. For example, autonomous systems such as UAVs for rapid collection of data on animal position, health, and behavior would be of great value.
Imaging techniques play a pivotal role in understanding and characterizing animal phenotypes. These phenotypes encompass a wide range of traits, including anatomical, ontogenetical, physiological, and biochemical properties of animals. Characterizing these traits enables a more comprehensive view of animal performance, which should be possible by non-destructive and high-throughput means. An example of phenotyping in animals is measuring their respiration rate with video data. Farm animals are typically housed in groups, and automated detection and tracking of individual animals is important. Wang et al. (2023) recently designed a video-measurement system that selects video clips of pigs at rest, uses an oriented object detector for each animal, selects a region of interest without manual intervention, and measures respiration rate by analyzing time-varying features extracted from the region of interest on individual animals. Measurements made with the system have shown good agreement with standard methods. Animal production is highly complex, with many species and genotypes of animals as well as many different production methods. Much research is needed in this area to elucidate important phenotypes across the range of animals produced and production methods used.
Detailed activities/procedures
Research in animal breeding is critical to produce higher yielding animals to maintain food security and to produce more resilient animals. Considering more new phenotypes increases the likelihood of improvement in animal traits, and new phenotyping approaches are critical. Imaging techniques play a pivotal role in understanding and characterizing animal phenotypes. These phenotypes encompass a wide range of traits including size, gait, temperature, etc. Characterizing these traits enables a more comprehensive view of animal performance, which should be possible by non-destructive and high-throughput means. This project will focus on how to apply appropriate imaging sensors with autonomous platforms.
Objective 2: Research and develop advanced autonomous systems for crop and animal production.
Autonomous systems for crop and animal production can be broken into multiple tasks focused on row crops, specialty crops, and animals. Key aspects in all these areas include autonomous navigation, coordination of multiple autonomous machines, autonomous systems for real-time decision making and actuation (such as in management of weeds, diseases, insects, and nutrients), and autonomous harvesting. Specific to animals are such activities as herding and vaccination.
Obj. 2a. Improved autonomous navigation in the field.
Introduction
High-fidelity autonomous navigation for row crops has been available for almost two decades. However, various aspects of autonomous agricultural navigation remain to be considered in-depth. For example, a great amount of research remains to be done in obstacle avoidance, including animals, negative obstacles, etc. Additionally, it is common in agriculture to need means of navigation besides GNSS-based guidance, such as row following.
Detailed activities/procedures
We will focus in two areas. First, we will consider avoidance of all types of obstacles of practical importance in on-farm scenarios. These have been categorized into positive obstacles, negative obstacles, moving obstacles, and difficult terrain (Reina et al., 2016). Second, we will consider key optical-perception issues in agricultural scenarios such as variable lighting, dust, etc.
According to Reina et al. (2016), positive obstacles in agricultural fields include objects like high-vegetated areas, trees, crops, metallic poles, buildings, and agricultural equipment. Negative obstacles in agricultural fields include ditches, sink holes, and other depressions. Moving obstacles in agricultural fields include vehicles, people, and animals. Difficult terrain in agricultural fields includes steep slopes, highly irregular terrain, etc. The team will work to further catalog obstacle types and how they vary according to farm type and region. We will then focus on how autonomous farm equipment can perceive and avoid all these types of obstacles.
Optical systems such as camera imaging and Lidar are negatively affected by dust, smoke, and fog, whereas radar systems tend to be immune to this form of particulate entrainment. However, radar also has problems with specularity effects and limited range resolution, which may make it difficult to extract object features (Reina et al., 2016). Radar and vision systems can be combined to improve performance, and this area of sensor fusion will be pursued as a means to alleviate problems with particulate entrainment.
Obj. 2b. Coordination of multiple autonomous machines in the field.
Introduction
For decades, agricultural machinery has been growing in size to maximize the efficiency of the individual farm worker. With the move to autonomous systems, the size of the machine is less important from an operator-efficiency standpoint, and there are some benefits to smaller machines in terms of lowering soil compaction, maintaining productivity when a portion of the system fails, etc. Thus, there is a need to consider how teams of smaller machines can achieve similar throughputs to single large machines. This means that efficient navigation of teams must be studied.
Detailed activities/procedures
We will focus in three areas. We will consider teams of terrestrial vehicles, teams of aerial vehicles, and teams of terrestrial and aerial vehicles combined. In each case, considerations will include how teams can be controlled optimally to maximize efficiency, effectiveness, and robustness in light of challenging communications environments, potential difficulty with GPS, difficult weather conditions, difficult terrain, the potential for unforeseen obstacles, and likely nighttime operations.
An infinite number of potential scenarios involving numbers and types of autonomous vehicles can be considered, but the work herein will focus on real-world agricultural operations in which teams of autonomous machines can add significant value over current methods. An example of a team of terrestrial vehicles involves a group of, say 5, small ground-based spot-spray capable vehicles that could work together to precisely spray a field as needed. In this example, the value added would include the ability to reduce the spray footprint of an individual vehicle to an area of, for instance, 1.0 m^2 instead of a much larger swath, minimizing the likelihood of application at undesired locations and reducing the overall cost of the material applied. An example of a team of aerial vehicles involves a group of 2 UAVs collaboratively collecting remote-sensing data with synchronized image acquisition in order to achieve stereoscopic image pairs in real time and thus to create highly accurate plant-height maps. An example of a team of terrestrial and aerial vehicles combined involves 1 small ground-based spot-spray capable vehicle and 1 UAV providing aerial surveillance, identifying objects of interest on the ground, and directing the ground-based vehicle to (e.g.) inspect and spray precise locations in a field.
Obj. 2c. Autonomous systems for real-time decision making and actuation, such as in management of weeds, diseases, insects, and nutrients.
Introduction
Integration of autonomous systems for real-time decision-making and actuation for plant stress management is pivotal to enhance productivity, efficiency, and sustainability in modern agricultural systems. Current stress-management methods require extensive labor and material investment but are commonly imprecise, leading to suboptimal resource use and negative environmental impact. It is important to develop large-scale and comprehensive databases of various stress conditions as well as AI-driven models in order to develop intelligent systems capable of precision decision-making and actuation. In animal production, many studies have been focusing on the detection of animal health and welfare conditions. There is thus also a great need to build larger databases of behaviors and phenotypic data of animals with diseases and welfare issues, and to develop AI-based methods for early detection of those problems.
Detailed activities/procedures
Initial activity will involve developing comprehensive image databases for identifying weeds, diseases, insects, nutrient deficiencies and other stresses in a variety of crops. Project participants will conduct fieldwork to capture high-resolution images of stresses in their local regions. We will catalog images according to crop species, growth stages, and stress markers, lighting conditions, image resolutions, and image backgrounds, ensuring a wide representation of the conditions encountered in agricultural practices. We expect to collect images using digital RGB, multi-/hyper-spectral, and thermal cameras as well as LiDAR and other devices, carried by either aerial or ground vehicles, depending on equipment available to the participants. Images will be captured under various lighting conditions, ranging from the low light of dawn/dusk to the bright midday sun, and against a multitude of backgrounds such as different soil types, stubble, and other natural surfaces. Detailed imaging plans will be made at the beginning stage of this project to balance these essential categories in the datasets.
To bolster the robustness of autonomous agricultural systems, we will also generate synthetic images using both conventional data augmentation methods and AI-driven generative models. These images will be designed to fill gaps in the database, particularly for rare or hard-to-capture conditions. We will implement geometric transformations (rotation, flipping, cropping, and scaling), color augmentations (adjusting the brightness, contrast, saturation, and hue), and perspective transformations (skewing or distorting). We will also develop and optimize generative models such as Generative Adversarial Networks (GANs) and Variational Autoencoders (VAEs) to create highly realistic images that augment the image datasets from real-world scenarios.
The collected and synthetic images will be used to train AI-driven models for stress identification. Images must first be processed (e.g., for geometric and radiometric correction) and manually annotated for model training. We will experiment with various advanced AI model architectures, such as Convolutional Neural Networks (CNN) and Vision Transformers (ViT), together with object detection or semantic segmentation features for different tasks in this objective. Additionally, we may explore newer architectures or develop custom models tailored to the specific challenges of agricultural imagery. The developed models will undergo rigorous validation and refinement processes to ensure they meet the accuracy requirements of real-world application. We will explore a variety of options to ensure that our systems are not only accurate but also computationally efficient for real-time decision-making.
Obj. 2d. Autonomous harvesting.
Introduction
The harvesting of certain crops is already fully mechanized, so automating this type of harvesting will generally involve navigation, speed control, and cleaning control. While there may yet be some work remaining to be done in these areas, the focus here will mainly be on specialty crops. These crops typically include fruits, vegetables, and nuts.
Autonomous harvesting of fruit crops has been heavily focused on apples, citrus, peaches, kiwifruit, etc. Progress has been made during the past several years, but several items need to be considered, including target fruit detection and localization in field settings with varying illumination, robotic arm control and manipulation, end-effector control and manipulation, and co-robot (i.e., human-robot) coordination for tasks like “pick-and-catch”.
Computer vision systems have been investigated for accurate fruit detection under various conditions based on deep-learning (DL) based approaches. State of the art DL architectures like YOLO can help researchers achieve high detection accuracies when training datasets are sufficient. Recently, YOLOv9 was released with an updated architecture in which oriented bounding boxes can be implemented for enhanced detection. Once target fruits are detected at the pixel-level, localization plays a vital role for bridging detection with actuation, particularly when 2D coordinates need to be mapped to 3D coordinates. Cameras that can assess depth or distance are currently designed for industry purposes such as vehicle/pedestrian detection, not for agricultural purposes. Consequently, both stereo and Time-of-Flight (ToF) cameras (or RGB-depth cameras) suffer from obtaining accurate localization results (for example, the optimal range of a depth camera does not work well in agricultural settings). Experiments have been conducted on some indoor trails, but field testing is greatly needed to solve real-world agricultural problems.
Robotic arm control and manipulation has also been researched for fruit harvesting. Depending on their actuation and/or structural features, the types of manipulation can be categorized into serial, parallel, and coordinate manipulations, among which the serial manipulators are widely adopted for agricultural harvesting tasks. Serial manipulators are flexible with sufficient working space, which can be controlled to reach the target fruit from various angles by driving the connected joints. This type of manipulator normally uses the open-loop kinematic chain mechanism. While a 6-degree-of-freedom (DoF) robotic arm is common, the arm can be further simplified (e.g., 4-DoF or 5-DoF) for different agricultural tasks, or it can be further complicated (e.g., 7-DoF) to avoid additional layers of obstacles or reach to the target from a certain angle. Many commercial robotic arm serial manipulators can be found in 6/7-DoF, such as Universal Robotics and Kinova series. Different from serial manipulators, parallel manipulators are connected and controlled by two or more kinematic chains or branches to accomplish various tasks. They often have less working space and higher rigidity compared to their serial counterparts, and they avoid accumulated errors from connected joints that can occur with serial manipulators. For example, robotic parallel manipulators were manufactured and used by Abundant Robotics for fresh apple harvesting in orchards. Finally, Cartesian coordinate manipulators are commonly adopted in agricultural tasks due to their control simplicity and high positioning accuracy. A good example from industry is Fresh Fruit Robotics (FFRobotics Inc.), who utilized 12 Cartesian manipulators on a prototype for fresh apple harvesting in orchards.
Detailed activities/procedures
We will consider the robotics issues that are common among various specialty crops. These issues include identification and classification of the fruit, particularly in circumstances of occlusion of the fruit. They also include guiding end-effectors to the fruit, and appropriate design of end-effectors for the various types of fruit. While most of the specialty crops are harvested mechanically, there is still a long way to go to fully mechanize all specialty crops to a level that is acceptable for the fresh-fruit market. There are mechanical harvesters for berries, apples, grapes, etc., but those machines either damage the fruit or the varieties with which they can work are limited. Therefore, two different directions are envisioned: 1) integrate autonomy and robotics into current mechanical harvesters to minimize the required labor, reduce the damage, increase harvesting speed, and even add other processes such as in-field sorting; or 2) develop custom robotic systems to harvest specialty crops. In some cases, the current mechanical harvesters cannot be improved and a custom robot needs to be developed. Consequently, work is needed in object detection, localization, pose estimation, end-effector development, robotic arm configuration and manipulation, control algorithms.
Detection involves utilizing appropriate image sensors, developing custom networks, utilizing machine vision techniques beside object detection networks, and controlling ambient illumination. Localization involves utilizing appropriate depth sensors, developing custom object detection algorithms (and/or adopting current techniques and modifying them),and controlling the ambient illumination. Developing an appropriate end-effector involves research on the mechanization of various picking methods (i.e., pulling, vibrating, twisting, etc.). Developing an appropriate robotic arm involves considering the minimum number of degrees of freedom (DOF) to be cost-effective, efficient, and agile. Adding more DOF will make the control algorithm complicated. However, in some cases more DOF is needed to avoid foliage and branches to reach a fruit or to manipulate a fruit before picking in order to put it in the right orientation relative to the end-effector. Developing control algorithms involves considering all inputs and outputs of the above-mentioned systems. Control algorithms receive inputs, process data, and send output to related systems. Developing a good control algorithm can have a major impact on a robot’s overall performance in terms of both the number of picked fruits among those available the cycle time required to pick one fruit and move the arm (and robot) to the next one.
Objective 3: Research and develop autonomous post-harvest processing systems.
Obj. 3a. Autonomous classing and sorting systems for plant produce and animal products.
Introduction
Both harvesting and post-harvest processing of various crops involve classing and sorting plant produce. Classing is commonly based on shape, size, and color (Kondo, 2010), but other factors can be important. Furthermore, classing and sorting are important in processing animal products such as meat, fish, and eggs. The focus here, for both plant and animal produce, will be on differentiating produce from extraneous matter, and classification based on various means of perceiving grade and quality.
Detailed activities/procedures
We will consider automated systems for both classing and sorting of plant and animal produce. The most important issue associated with this task is perception, which can be conducted by optical or other means. In the case of optical means, which are most common, various forms of cameras are commonly used. These can involve multispectral, hyperspectral, thermal, and depth based on IR scanning or stereo. Illumination for camera systems is also important, and constructed-Illumination systems have proven useful in differentiating traits in meats (Cai et al., 2024) and other produce. Other optical systems involve fluorescence cameras and the measurement of specular reflectance and light polarization. Non-optical systems include those for perception of chemical constituents, but these tend to be more difficult to automate in the field. Thus, the focus in this work will be on optical systems and will consider issues like differentiating produce from extraneous matter, measuring size and shape, and measuring color and other optical characteristics. In addition to these sensing activities, we will consider integration of sensor outputs and processing activities such as mechanical sorting.
Obj. 3b. Autonomous systems for processing plant produce and animal products such as cutting and trimming.
Introduction
Many types of crop and animal products require some type of cutting and trimming, which is commonly done manually. These tasks also often require precise positioning of the item prior to cutting. The focus here will be on autonomous systems for physical sorting, physical positioning of objects for processing activities such as cutting, defect detection, and the acts of processing themselves, such as cutting, where robotic arms and end-effectors are critical.
Detailed activities/procedures
We will consider positioning of objects for precise processing as well as the processing task itself. Processing tasks can include cutting, trimming, etc., and they can involve a large variety of end-effectors such as metal knives, oscillating cutters, and water-jet cutters. Sensing technologies may include 3D imaging, possibly Lidar, and acoustic monitoring. In each case, the focus will be on real-world applications in which autonomous systems can bring significant added value. Examples of such applications include catfish processing, for which robotic perception is need to replace human labor, which is largely unavailable to meet the needs of industry and is inherently dangerous and can lead to contamination and waste of fish meat. Robotic perception is needed to detect and localize the head, body, fins, tail, and image background with high inference speed. Also, precise and rapid positioning of cutting devices in conjunction with the robotic perception is required. Another example involves the classification and sorting of sweetpotatoes, which is commonly done in two stages, primarily at harvest and secondarily at the processing facility. At both locations, robotic perception is needed to replace human labor, which is largely unavailable. Robotic perception is needed to classify sweetpotatoes into USDA No. 1, USDA No.2, Jumbo, Canner, and Cull categories, which vary in value by an order of magnitude. Also, precise and rapid positioning of deflecting devices in conjunction with the robotic perception is needed for physical separation of the categories.
Objective 4: Research on Socioeconomic Effects of A2 on Various Farm Scales
Obj. 4a. Understanding the effects of A2 on various farm scales.
Introduction
Emerging A2 technologies are commonly designed to fit large-scale farms, while small farms often cannot access them to enjoy the benefits. Therefore, there has been growing concern that A2 will increase the competitiveness of large farms and drive smaller farms out of the market. The research goal under this objective is to provide scientifically based answers to this concern and recommend avenues through which farms at various scales can benefit. We will utilize various research techniques, including empirical models, budget simulations, and survey studies, to estimate A2 technologies’ potential negative impacts on small farms from various angles of historical trends, what-if scenarios, and stakeholders’ subjective opinions.
Detailed activities/procedures
First, we first propose to look at whether there is a connection between A2 adoption and farm size changes throughout history. If A2 technologies indeed favor large farms, there could be a “get big or get out” pressure (Carlson, 2021) on small farms, and thus we may expect an increase in farm size caused by A2. However, most existing A2 technologies are not fully commercialized and lack sufficient historical data, while the only exception is perhaps the autonomous guidance system, which has been commercially available in large farming equipment for nearly two decades. Therefore, in this project we will consider first the historical data of agricultural auto-guide adoptions and farm size changes to conduct an empirical test of their relationship.
The auto-guide adoption data will be collected from existing survey studies of agriculture technology adoptions. Examples are the Purdue University Precision Agriculture Dealership Survey (Erickson and Lowenberg-DeBoer, 2020) and the USDA Agricultural Resource Management Survey (ARMS). Published journal articles and reports which contain auto-guide adoption information will also be reviewed as supplementary data. The farm size history data at the county level will be primarily obtained from the USDA National Agricultural Statistics Service (NASS) and the Census of Agriculture from various years.
The correlation between auto-guide adoptions and farm size changes will be empirically tested by an econometric model. The model specification is built upon the literature of determinants of farm sizes (Akimowicz et al., 2013; Bartolini and Viaggi, 2013; DeBoer‐Lowenberg and Boehlje, 1986, which captures the fact that farm size changes are driven by complex socioeconomic and environmental factors such as farmland prices, legislation, government policy, geography, demography, and so on. The effect of auto-guide adoption on farm size changes is estimated while those other farm size impacting factors are controlled. Furthermore, to overcome the common endogeneity problem of making causal inference using observational data and econometric regression models, the classical instrumental variable (IV) approach will be utilized (Ebbes et al., 2016.).
The expected results will reveal whether regions or sectors with higher auto-guide adoption rates experienced faster farm size increases (i.e., farm consolidation). It can be regarded as historical fact-based evidence of A2 technologies negative impact on small farms. However, the potential pitfall of the results is the spurious relationship between auto-guide adoption and farm sizes. There could be some (unobserved) confounding factors that caused both auto-guide adoption and farm size changes. It could also be the reverse causality that it was the increase of farm sizes that pushed higher auto-guide adoption rates. We will use research techniques in other sub-objectives to complement these results.
The second approach to consider the potential impacts of A2 will involve the financial status simulation of large and small farms assuming the A2 is adopted. In the literature, the partial budgeting method is widely used to evaluate financial status changes caused by A2 adoption. But it has a major limitation of only considering cost and revenue changes directly by the adoption of A2 and assuming everything else is held constant (Lowenberg-DeBoer et al., 2020). In practice that is rarely the case, as farm management is highly likely to be adjusted as well after adopting new A2 technologies. In the simulation of this project, we will utilize a more comprehensive whole-farm profitability simulation following the methods of Knight and Malcolm (Knight and Malcolm, 2009) and Lowenberg-DeBoer (Lowenberg-DeBoer et al., 2019.).
Detailed cost information will be collected on specific A2 technologies (e.g., autonomous tractor), including the ownership and operational costs. Note, that if the A2 technology is not commercialized yet (which is common in this study), the prototype will be used as an approximation. Case study farms of different sizes will be used in the simulation. The labor, materials, and other management cost information will be collected through the participating farm owners. The whole-farm profitability will be simulated with and without adopting A2 technology. A key component in the simulation is the farm management impacts from implementing the new A2 technology, such as labor savings and productivity increases. Those impacts will be obtained from either on-farm trials with collaborating farmers, or from the prototype testing data from the manufacturers.
An interactive web-based tool will be designed to run the simulations, where different costs, prices, markets, and farm parameters are allowed to enter the simulation engine. The simulation results will predict the financial status impacts of the existing (or emerging) A2 technologies if they were adopted by farms of different sizes and provides quantified evidence of whether the current A2 technologies result in more profit gain to large farms. Different scenarios can also be tested to find out when the A2 technologies will be economically feasible for small farms, such as lower purchase prices, machine size reduction, equipment cost sharing by multiple farms, and so forth.
It should be noted that the simulation study results are based on artificial data and can be sensitive to the simulation’s settings. Also, the real-world scenarios will be more complex than the simplified simulation models. Therefore, results from this study can only be used for illustration purposes. However, by carefully setting the simulation parameters and conducting adequate sensitivity analyses, the simulation results can be regarded as a reasonably close approximation to the actual farms’ financial situations. In addition, the web-based simulation tool created from this study will also allow individual farmers to enter their own scenario parameters to generate customized financial simulation results for their reference.
Third, we will conduct focus groups and interviews to gather opinions and perspectives from farmers and industry members about the A2 technologies and their impacts relative to social and economic factors. Our goal is to bring together the farming community with industry members to discuss new technologies in development. To give farmers from different communities the ability to express their needs, challenges, and the benefits they see from the inclusion of A2 technologies into their operations. This will allow industry members to collaborate with small farmers to come up with viable solutions that are both socially impactful and economically feasible. Meetings will be held in-person whenever possible, but virtual options may also be used. With the increase in broadband capabilities in the state of Mississippi this is option is more readily available. This capability also makes it possible for greater usage of precision agriculture and for A2 technologies to be adopted. Similar to the methods described in sub-objective 1.3 the interviews and focus groups will be recorded. The students involved in the project will then transcribed those conversations for qualitative analysis. Through the qualitative data analytics, trends associated with the key beliefs and attitudes along with sentiment will be identified relative to all of the different stakeholders. This will help inform the research team of the prevailing impacts from both the industry and the small commercial farm perspectives.
Obj. 4b. Making recommendations for A2 relative to various farm scales.
Introduction
Based on the analyses conducted under objective 4a, we will suggest ways in which A2 can make small farm operations more competitive. We will also make recommendations relative to business models that enable small farm operations to enjoy the benefits of A2.
Detailed activities/procedures
A qualitative summary/collection of opinions from both industry members and small farmers will be generated, which is expected to provide a better understanding of the issues and concerns experience by both groups of stakeholders. From this knowledge gained, it is expected that proposed recommendations can be formed for addressing those concerns to the benefit of all stakeholders involved. By gaining a better understanding of the social and economic impacts of A2 technologies, a plan for adoption can be developed. The results from this research will inform the future directions of A2 and its adoption by both industry and farms of all sizes.
Finally, we will make a quantitative summary and analysis of the survey data collected in the previous objectives to identify the key factors that affect the A2 adoption decisions by small farms. Because there are rarely any actual A2 adoptions yet, the dependent variable in the analysis will be the intention to adopt A2 technologies. The intention data will be collected from the surveyed farmers by questions like “will you adopt A2 if you have more access to free A2 consultant service”. The quantitative relationship between small farms’ A2 survey responses (challenges, attitudes, and other farm characteristics) and the intention to adopt A2 survey will be estimated through a logistic regression model.
The model specification and independent variable selection will follow the large body of literature on farmers’ adoption of general precision agriculture technologies (Adrian et al., 2005; Li et al., 2020; Paustian and Theuvsen, 2017; Pierpaoli et al., 2013; Tey and Brindal, 2012), where the major barriers to adoption include technical obstacles (lack of skill and capability required, access to consultant services), economic incentives (high costs, unknown benefits), perception and attitudinal characteristics (awareness, understanding, risk), and farm characteristics (farm demography, farm size, crop types, years of experience, and so on). In particular, Pierpaoli et al. (Pierpaoli et al., 2013) provide a comprehensive structure of driving factors’ effects on agricultural technology adoption intention. Based on the survey data of this project, we will create a similar structure to describe and quantify the effects of various factors impacting small farmers’ intentions for A2 adoption.
The expected modeling results will provide a quantified understanding of the factors that impact small farmers’ intention to adopt A2, including the statistical significance and the magnitudes of the impacts. That will help manufactures, researchers, dealers, and farmers to have a better vision of the obstacles in the current A2 progress specific to small commercial farms, and also serve as fundamental facts for making recommendations for improving A2 development and adoption described in Objective 3. However, caution should always be taken that the A2 adoption survey in this project is based on the stated preference rather than the revealed preference (Wardman, 1988), and thus the results may include some inaccuracies and should not be overinterpreted.
Measurement of Progress and Results
Outputs
- Obj. 1a: New and advanced autonomous systems will be developed for accurately measuring plant phenotypes. Comments: These will involve the measurement of common phenotypes in new crops, the measurement of new phenotypes, more accurate measurement of phenotypes, and more efficient measurement of phenotypes.
- Obj. 1b: New and advanced animal phenotypes like temperature, etc., will be developed and associated sensors mounted on autonomous platforms for research and monitoring of animal health, distribution, etc.
- Obj. 2a: Sensors and associated algorithms for avoidance of all positive types of obstacles of practical importance in on-farm scenarios, including negative obstacles, will be developed.
- Obj. 2b: Methods for team coordination of terrestrial vehicles, aerial vehicles, and groups of terrestrial and aerial vehicles will be developed.
- Obj. 2c: Image databases for weeds, insects, and plant stress conditions under various lighting conditions, image resolutions, and image backgrounds, such as bare soil and stubble, as well as synthetically generated images, will be developed.
- Obj. 2d: AI-based imaging systems and end-effectors for specialty crops that can identify and classify fruit, particularly when it is occluded, and also sort and even pick the fruit, will be developed.
- Obj. 3a: Imaging systems for classing and sorting of plant produce, animals, and meats will be developed that will differentiate produce from extraneous matter, measure size and shape, and measure color and other optical characteristics.
- Obj. 3b: Sensing and actuation systems for positioning of objects for precise processing as well as the processing task itself (cutting, trimming, etc.) will be developed.
- Obj. 4a: An understanding of the socioeconomic effects of A2 on various farm scales will be developed.
- Obj. 4b: Recommendations about business models for A2 that will benefit small farm operations will be developed.
Outcomes or Projected Impacts
- Obj. 1a: The outcomes include new, more accurate, and more efficient measurement of phenotypes and other plant-based research data for a wide variety of crop plants that will enable more rapid and efficient advances in crop breeding and agronomic research programs leading to improved food security in an unpredictable future. The results of research under this subobjective will be tracked, and the level of information dissemination and uptake in the research community will be monitored. Metrics include publications, presentations, patents, and adoption studies.
- Obj. 1b: Automated animal health detection will enhance animal management on the range and in CAFOs. The results of research under this subobjective will be tracked, and the level of information dissemination and uptake in the research community will be monitored. Metrics include publications, presentations, patents, and adoption studies.
- Obj. 2a: Navigation in all types of on-farm scenarios, including over and around negative obstacles, will be available. The results of research under this subobjective will be tracked, and the level of information dissemination and uptake in the research and industrial communities will be monitored. Metrics include publications, presentations, patents, and adoption studies.
- Obj. 2b: Groups of terrestrial and aerial vehicles will be able to autonomously perform farm tasks in newly efficient ways, often with smaller vehicles. The results of research under this subobjective will be tracked, and the level of information dissemination and uptake in the research and industrial communities will be monitored. Metrics include publications, presentations, patents, and adoption studies.
- Obj. 2c: The multistate research team and external stakeholders will have access to massive image databases for weeds, insects, and plant stress conditions under various lighting conditions, image resolutions, and image backgrounds, such as bare soil and stubble, as well as synthetically generated images. They can use the data to generate AI algorithms for detection. The results of research under this subobjective will be tracked, and the level of information dissemination and uptake in the research and industrial communities will be monitored. Metrics include publications, presentations, patents, and adoption studies.
- Obj. 2d: The capability of autonomous systems to identify, classify, sort, and pick fruit in the field or an industrial plant will have been significantly advanced. The results of research under this subobjective will be tracked, and the level of information dissemination and uptake in the research and industrial communities will be monitored. Metrics include publications, presentations, patents, and adoption studies.
- Obj. 3a: The ability of autonomous systems to classify and sort plant produce, animals, and meats from extraneous matter and measure their size, shape, color, and other optical characteristics will have been significantly advanced. The results of research under this subobjective will be tracked, and the level of information dissemination and uptake in the research and industrial communities will be monitored. Metrics include publications, presentations, patents, and adoption studies.
- Obj. 3b: The capability of processing systems to sense and actuate devices for positioning and precise processing (cutting, trimming, etc.) of fruit, vegetable, and meat objects will have been significantly advanced. The results of research under this subobjective will be tracked, and the level of information dissemination and uptake in the research and industrial communities will be monitored. Metrics include publications, presentations, patents, and adoption studies.
- Obj. 4a: Politicians and industry stakeholders will better understand how A2 affects various farm scales. The results of research under this subobjective will be tracked, and the level of information dissemination and uptake in the research and policy communities will be monitored. Metrics include publications, presentations, patents, and adoption studies.
- Obj. 4b: Politicians and industry stakeholders will have a set of recommendations regarding A2 that can be considered. The results of research under this subobjective will be tracked, and the level of information dissemination and uptake in the research and industrial communities will be monitored. Metrics include publications, presentations, patents, and adoption studies.
Milestones
(2025):Obj. 1a, 1b, 2a, 2b, 2c, 2d, 3a, 3b, 4a, and 4b: In year 1, research teams and specific collaborative research projects will be formed for each objective.(2027):Obj. 1a: In year 3, new, more accurate, and more efficient method for plant phenotype measurement will have been developed. Obj. 1b: animal health detection methods will have been developed for the range and CAFOs. Obj. 2a: Autonomous navigation for a few specific types of on-farm scenarios will have been developed. Obj. 2b: In years 3-5, the research team will perform design and testing of autonomous teams in various scenarios. Obj. 2c: In years 3-5, the research team and external stakeholders will have completed preliminary image databases for weeds, insects, and plant stress conditions under various lighting conditions, image resolutions, and image backgrounds, as well as synthetically generated images. They can use the data to generate AI algorithms for detection. Obj. 2d: By year 3, the research team will have developed AI-based imaging systems and end-effectors for specialty crops that can identify and classify fruit, particularly when it is occluded, and also sort and even pick the fruit. Obj. 3a: By the end of year 3, the team will have developed the ability of autonomous systems to classify and sort plant produce, animals, and meats from extraneous matter and measure their size, shape, color, and other optical characteristics. Obj. 3b: The team will have developed the capability of processing systems to sense and actuate devices for positioning and precise processing (cutting, trimming, etc.) of fruit, vegetable, and meat objects. Obj. 4a: The team will have developed mechanisms by which they can assess opinions and attitudes about A2 at different farm scales.
(2029):Obj. 1a: At the end of the five-year project, development of autonomous systems with high accuracy in measuring important phenotypes (e.g., yield and quality) of important agricultural crops will have been completed. Obj. 1b: Automated animal health detection to enhance animal management on the range and in CAFOs will have been developed. Obj. 2a: Autonomous navigation for multiple on-farm scenarios will have been developed, tested, and refined. Obj. 2b: Design and testing of autonomous machinery teams for various scenarios will be complete. Obj. 2c: Massive image databases for weeds, insects, and plant stress conditions under various lighting conditions, image resolutions, and image backgrounds, as well as synthetically generated images will have been completed. Obj. 2d: The research team will have refined and tested AI-based imaging systems and end-effectors for specialty crops that can identify and classify fruit, particularly when it is occluded, and also sort and even pick the fruit. Obj. 3a: The team will have refined and tested the ability of autonomous systems to classify and sort plant produce, animals, and meats from extraneous matter and measure their size, shape, color. Obj. 3b: The team will have refined and tested the capability of processing systems to sense and actuate devices for positioning and precise processing (cutting, trimming, etc.) of fruit, vegetable, and meat objects. Obj. 4a: The team will have completed its analyses of survey and other data to determine the effects of A2 on various farm scales. Obj. 4b: The team will have made recommendations for considerations by politicians and industry stakeholders relative to A2 and its effects on various farm scales.
Projected Participation
View Appendix E: ParticipationOutreach Plan
Regarding outreach and extension, the team members plan to work with county agents and extension specialists, consultants, producers, and allied industries, regarding the critical needs for autonomy on the farm. The team will also work with extension faculty to develop training materials on the use of autonomous systems in farm operations. Training county agents will have a significant impact on technology transfer as they are the primary source of information to assist farmers for crop production issues related to advances in technology. For example, Mississippi State University’s Extension Service has an extensive network of county agriculture Extension Agents (CEAs) throughout the State of Mississippi in all 82 counties. Due to their important role in supporting our growers, they have to be updated on new issues and options. Therefore, we propose to develop training sessions that will be provided at the four research and extension (R&E) centers in the state: North Mississippi R&E Center at Verona, Delta R&E Center at Stoneville, Central Mississippi R&E Centre in Raymond, and Coastal R&E Center in Biloxi. Extension Specialists from each region will train and involve numerous CEAs in the design, evaluation, and dissemination of autonomy-based tools. Examples of extension and outreach activities/venues include: (i) development of digital communication and education materials; (ii) podcast series to present the developed AI-based technologies, designed to reach a broader audience; (iii) webinars that will focus on new developments; (iv) in-service training to teach extension agents (in-person and via the eXtension network to train agents across the U.S.); (v) field days; (vi) technology show and expo; (vii) extension publications, infographics, and fact sheets.
Organization/Governance
The Technical Committee of this multistate project will consist of project leaders from the contributing states, the administrative advisor, and CSREES representatives. Voting membership includes all persons with contributing projects.
This multistate research project will have three officer positions, Chair, Vice Chair, and Secretary. The officers will be elected from the voting membership at the first authorized committee meeting after the project has been approved. The Chair, Vice-Chair, and Secretary will serve two years if so desired by the membership. At the end of each term, the Vice Chair will become Chair, the Secretary will become Vice Chair, and a new Secretary will be elected. The three officers will make up the project’s Executive Committee responsible for project supervision, outreach plan implementation, activity coordination (e.g., annual meeting arrangements and annual reports), and project renewal proposal development.
Due to the diversity of the membership and applications, working groups may be formed for each target crop/operation/objective, and the coordinators of the working groups will be selected by the project Chair. The coordinators will be responsible for communication within the working group, developing a timeline for the targeted crop/operation, and coordinating activities among the four project objectives (as applied to the particular targeted crop/operation).
Administrative guidance will be provided by an assigned Administrative Advisor and a NIFA Representative.
Literature Cited
Adrian, A.M., Norwood, S.H., Mask, P.L., 2005. Producers’ perceptions and attitudes toward precision agriculture technologies. Computers and electronics in agriculture 48, 256-271.
Akimowicz, M., Magrini, M.B., Ridier, A., Bergez, J.E., Requier‐Desjardins, D., 2013. What influences farm size growth? An illustration in Southwestern France. Applied Economic Perspectives and Policy 35, 242-269.
Atefi, A., Y. Ge, S. Pitla, and J. Schnable. 2020. Robotic detection and grasp of maize and sorghum: stem measurement with contact. Robotics 163(1): 104854; doi.org/10.1016/j.compag.2019.104854.
Atefi, A., Y. Ge, S. Pitla, and J. Schnable. 2019. In vivo human-like robotic phenotyping of leaf traits in maize and sorghum in greenhouse. Computers and Electronics in Agriculture 9(3):58; doi.org/10.3390/robotics9030058.
Bac, C. W., van Henten, E. J., Hemming, J., & Edan, Y. (2014). Harvesting Robots for High-value Crops: State-of-the-art Review and Challenges Ahead. In Journal of Field Robotics (Vol. 31, Issue 6, pp. 888–911). John Wiley and Sons Inc. https://doi.org/10.1002/rob.21525.
Barnes, E., Morgan, G., Hake, K., Devine, J., Kurtz, R., Ibendahl, G., Sharda, A., Rains, G., Snider, J., Maja, J.M., Thomasson, J.A., Lu, Y., Gharakhani, H., Griffin, J., Kimura, E., Hardin, R., Raper, T., Young, S., Fue, K., Pelletier, M., Wanjura, J., Holt, G., 2021. Opportunities for Robotic Systems and Automation in Cotton Production. AgriEngineering 3, 339-362.
Bartolini, F., Viaggi, D., 2013. The common agricultural policy and the determinants of changes in EU farm size. Land use policy 31, 126-135.
Cai, J., Y. Lu, E. Olaniyi, S. Wang, C. Dahlgren, D. Devost-Burnett, and T. Dinh. 2024. Beef marbling assessment by structured-illumination reflectance imaging with deep learning. J. Food Eng. 369(1):111936.
Carlson, M. "Earl Butz-US politician brought down by racist remark." U.S. News. https://www.theguardian.com/world/2008/feb/04/usa.obituaries (accessed July 11, 2021).
DeBoer‐Lowenberg, J., Boehlje, M., 1986. The impact of farmland price changes on farm size and financial structure. American Journal of Agricultural Economics 68, 838-848.
Ebbes, P., Papies, D., van Heerde, H.J., 2016. Dealing with endogeneity: A nontechnical guide for marketing researchers. Handbook of market research, 1-37.
Erickson B., and J. Lowenberg-DeBoer, "2020 Precision Agriculture Dealership Survey," Purdue University, August 2020, issue 2020. [Online]. Available: https://ag.purdue.edu/digital-ag-resources/croplife-purdue-university-precision-agriculture-dealership-survey-2020-report-and-archive/.
Erickson, B., and J. Lowenberg-DeBoer. 2022. Precision Agriculture Dealership Survey. Departments of Agronomy and Agricultural Economics. West Lafayette, IN: Purdue University.
Fue, K., Porter, W., Barnes, E., & Rains, G. (2020). An Extensive Review of Mobile Agricultural Robotics for Field Operations: Focus on Cotton Harvesting. AgriEngineering, 2(1), 150–174. https://doi.org/10.3390/agriengineering2010010.
Gaus, C.-C., Urso, L.-M., Minßen, T.-F., & de Witte, T. (2017). Economics of mechanical weeding by a swarm of small field robots (p. 4). https://doi.org/10.22004/ag.econ.262169.
Grift, T., Zhang, Q., Kondo, N., & Ting, K. C. (2008). A review of automation and robotics for the bio-industry. In Journal of Biomechatronics Engineering (Vol. 1, Issue 1). www.tibm.org.tw.
Knight B., and B. Malcolm, "A whole-farm investment analysis of some precision agriculture technologies," Australian Farm Business Management Journal, vol. 6, no. 1, pp. 41-54, 2009.
Kondo, N. Automation on fruit and vegetable grading system and food traceability. 2010. Trends Food Sci. Technol. 21(1):145-152; doi:10.1016/j.tifs.2009.09.002.
Li, W., Clark, B., Taylor, J.A., Kendall, H., Jones, G., Li, Z., Jin, S., Zhao, C., Yang, G., Shuai, C., 2020. A hybrid modelling approach to understanding adoption of precision agriculture technologies in Chinese cropping systems. Computers and Electronics in Agriculture 172, 105305.
Li, B., Vigneault, C., & Wang, N. (2010). Research development of fruit and vegetable harvesting robots in China. In Stewart Postharvest Review (Vol. 6, Issue 3, pp. 1–8). https://doi.org/10.2212/spr.2010.3.12.
Li, P., Lee, S. H., & Hsu, H. Y. (2011). Review on fruit harvesting method for potential use of automatic fruit harvesting systems. Procedia Engineering, 23, 351–366. https://doi.org/10.1016/j.proeng.2011.11.2514.
Lowenberg-DeBoer, J., Behrendt, K., Godwin, R., Frankin, K., 2019. The impact of swarm robotics on arable farm size and structure in the UK, 93rd Annual Conference of the Agricultural Economics Society, University of Warkwick, England.
Lowenberg-DeBoer, J., Huang, I.Y., Grigoriadis, V., Blackmore, S., 2020. Economics of robots and automation in field crop production. Precision Agriculture 21, 278-299.
Oliveira, L. F. P., Moreira, A. P., & Silva, M. F. (2021). Advances in agriculture robotics: A state-of-the-art review and challenges ahead. Robotics, 10(2), 52.
Our World in Data. 2022. https://ourworldindata.org/agricultural-production#explore-data-on-agricultural-production, accessed on 29 JUN 2024.
Paustian, M., Theuvsen, L., 2017. Adoption of precision agriculture technologies by German crop farmers. Precision agriculture 18, 701-716.
Pierpaoli, E., Carli, G., Pignatti, E., Canavari, M., 2013. Drivers of precision agriculture technologies adoption: a literature review. Procedia Technology 8, 61-69.
Reina, G., A. Milella, R. Rouveure, M. Nielsen, R. Worst, and M. R. Blas. 2016. Ambient awareness for agricultural robotic vehicles. Biosyst. Eng, 146(1):114-132; doi.org/10.1016/j.biosystemseng.2015.12.010.
Shamshiri, R., Weltzien, C., A. Hameed, I., J. Yule, I., E. Grift, T., K. Balasundram, S., Pitonakova, L., Ahmad, D., & Chowdhary, G. (2018). Research and development in agricultural robotics: A perspective of digital farming. International Journal of Agricultural and Biological Engineering, 11(4), 1–11. https://doi.org/10.25165/j.ijabe.20181104.4278.
Tey, Y.S., Brindal, M., 2012. Factors influencing the adoption of precision agricultural technologies: a review for policy implications. Precision agriculture 13, 713-730.
United Nations. 2022. https://population.un.org/wpp/Graphs/DemographicProfile, accessed on 29 JUN 2024.
USDA Economic Research Service. 2023. https://www.ers.usda.gov/topics/farm-economy/farm-labor, accessed on 29 JUN 2024.
U.S. Census Bureau. 2020. https://www.census.gov/topics/population.html, accessed on 29 JUN 2024.
van Evert , F. K., D. Gaitán-Cremaschi, S. Fountas, and C. Kempenaar. 2017. Can Precision Agriculture Increase the Profitability and Sustainability of the Production of Potatoes and Olives? Sustainability 9(10):1863; doi.org/10.3390/su9101863.
Wang, M., X Li, M. L. V. Larsen, D. Liu, J. L. Rault, and T. Norton. 2023. A computer vision-based approach for respiration rate monitoring of group housed pigs. Computers and Electronics in Agriculture 210(1):107899; doi.org/10.1016/j.compag.2023.107899.
Wardman, M., 1988. A comparison of revealed preference and stated preference models of travel behaviour. Journal of transport economics and policy, 71-91.
Williams, H. A. M., Jones, M. H., Nejati, M., Seabright, M. J., Bell, J., Penhall, N. D., Barnett, J. J., Duke, M. D., Scarfe, A. J., Ahn, H. S., Lim, J. Y., & MacDonald, B. A. (2019). Robotic kiwifruit harvesting using machine vision, convolutional neural networks, and robotic arms. Biosystems Engineering, 181, 140–156. https://doi.org/10.1016/j.biosystemseng.2019.03.007.
Yang, Q., Du, X., Wang, Z., Meng, Z., Ma, Z., Zhang, Q., 2023. A review of core agricultural robot technologies for crop productions. Computers and Electronics in Agriculture 206.