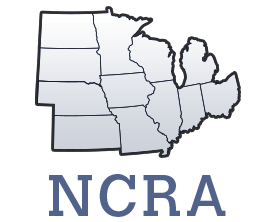
NC1212: Exploring the Plant Phenome in Controlled and Field Environments
(Multistate Research Project)
Status: Active
NC1212: Exploring the Plant Phenome in Controlled and Field Environments
Duration: 10/01/2021 to 09/30/2026
Administrative Advisor(s):
NIFA Reps:
Non-Technical Summary
Statement of Issues and Justification
To ensure the food, fiber and energy supply for a global population of 9.7 billion people by 2050, and increase biosecurity and social stability under projected climate change scenarios, we need to create crop plants that deliver more nutritional value and higher yields while requiring lower inputs (e.g. water and nutrient) and can resist local environmental challenges. To this end, plant scientists are developing and adopting cutting-edge advances in plant biology to establish novel traits in crop plants; however, greater knowledge of factors that influence crop growth and development is needed to improve plant breeding development pipelines.
During recent decades, there has been a tremendous increase in throughput and decrease in cost of genome sequencing (Shendure and Ji, 2008). As a result of advancements in next generation sequencing (NGS) technologies, many biological fields including plant breeding and genetics have been revolutionized. Plant breeders can use the high throughput data generated from NGS to genotype mapping populations for gene or quantitative trait loci (QTL) discovery as well as predict and select desired individuals based on their genome estimated breeding values (Varshney et al., 2014). Phenotyping, however, is a key requirement for successful implementation of molecular mapping and breeding strategies including linkage mapping, genome-wide association mapping (GWAS), marker assisted selection (MAS), and genomic selection. The level of throughput and data dimension obtained in estimation of phenotype has lagged behind that of genotype (White et al., 2012; Araus and Cairns, 2014). The challenge has shifted from understanding the genotype to understanding the phenotype – characteristics of a plant that are determined by the effects of genetic background, production environment, and management factors such as irrigation and fertilizer treatments.
Tackling the Phenotyping Bottleneck
More efficient plant breeding methodologies are needed to increase crop productivity to feed an ever-growing world population (Tester and Langridge, 2010). Most research programs are still relying on manual or semi-automated systems for collecting plant phenotype data resulting in high costs and low-throughput (White et al., 2012; Araus and Cairns, 2014). Low-throughput phenotyping is time-consuming and laborious, which often forces breeding programs to limit selection to yield evaluated in multi-environment, multi-year trials; even though the heritability of yield is among the lowest of commonly evaluated traits (Furbank and Tester, 2011).
Plant breeders and geneticists are interested in increasing the throughput of phenotyping at each stage of their breeding programs. Increased throughput is required because the typical breeding programs screen thousands of individuals at different stages of development. However, major changes have not been made in the phenotyping methodology over the years (Cooper et al., 2014). A relatively new approach to phenotyping, based on remote and proximal sensing, also known as High Throughput Phenotyping (HTP) or Phenomics in the Plant Science literature, may provide new tools for tackling the phenotyping bottleneck in plant breeding (Furbank and Tester, 2011; Araus and Cairns, 2014). HTP can increase the genetic gain by increasing selection intensity, phenotype repeatability, and trait heritability (Araus et al., 2018). Selection intensity is a function of the number of lines selected compared to the number of lines evaluated. With HTP, larger populations can be evaluated and more stringent selection criteria can be imposed. Responses to selection can be increased by minimizing the non-genetic variance through increasing trait repeatability and heritability (Bernardo, 2014). HTP also allows for increased replication and reduced between-measurement error by removing the human subjectivity in phenotyping. However, more work is needed to develop and optimize these systems.
High-Throughput Phenotyping Requires a Convergence of Technology in Agriculture
Phenomics is a key link between progress in plant genomics and novel trait development in agronomic crops because of its focus on assessing and quantifying plant traits at multiple scales. The phenotype of an organism refers to the observable morphological and physiological properties of the organism. Yet, plant phenotyping has been a bottleneck that limits efficient adoption of genomic tools to improve crop breeding. Until recently, the majority of plant phenotyping data have been manually collected and were low throughput, time-consuming, and labor intensive. Consequently, these traits were often limited to specific growth stages and did not reflect the dynamic response of crop plants to variations in environmental conditions. Recent advances in sensing technology, machine learning and computer vision technologies offer the opportunity of high throughput measurements of intricate morphological and biophysical traits of plants, providing us the capabilities to ascertain plant response to environmental variation both in controlled and field environments. These advancements empower plant biologists to acquire extensive information on key plant traits, therefore are of paramount importance in the quest to address current and emerging issues related to food security, link phenomics to underlying genes and gene networks, optimize yields, achieve resource use efficiencies (e.g., water, nutrient, and light), understand resistance to biotic and abiotic stresses, and develop biomass for bioenergy and other valuable traits in plants (Das Choudhury et al., 2020).
Remote sensing has been utilized to generate agricultural data for a number of years in a variety of ways. Remote sensing is defined as the field of deriving data from earth’s land and water surfaces using overhead images that are produced using reflected or emitted light from some region of the electromagnetic spectrum (Campbell and Wynne, 2011). Recent technological advancements in remote and proximal sensing have made it possible to extract massive volumes of morphological, physiological, and agronomic data from crops, but complexities in data processing, feature extraction, and data analytics make predictions of crop performance from remote sensing data a challenge. Multi-Sensor systems have been integrated on air-borne platforms for mobile mapping of agricultural fields (El-bahnasawy et al., 2018) and methods for spatial and temporal calibration have been developed (Habib et al., 2016; Habib et al., 2017; Ravi et al., 2018). Analytical tools for image and data processing, compression and integration have also been developed (Zhang et al., 2016; He et al., 2018; Ribera et al., 2018). Complete pipelines for measuring and predicting plant productivity and performance from multi-modal data, and linking all the way to changes in genotypes are being developed by different institutions and for diverse crops (Chen et al., 2017, 2018; Masjedi et al., 2020). High-throughput controlled environment phenotyping pipelines that include robotic, environment controlled, fully automated systems have been developed for greenhouses and growth chambers (Cotrozzi et al., 2020). Additional plant phenotyping pipelines for measuring and predicting plant productivity and performance from remotely sensed data are needed. These may include both ground-based and airborne sensor platforms and sensing apparatus for high-throughput phenotyping.
We propose the creation of a North Central regional plant phenotyping research project to promote advancements in phenotyping related research. This project will leverage established plant phenotyping facilities and capacities in research institutions in the North Central region and nurture research collaborations among scientists and engineers. Creation of this committee will coordinate multidisciplinary teams of scientists and engineers in the development of high-throughput phenotyping (HTP) facilities and systems in controlled and field environments. These systems will enable researchers to overcome the phenotyping bottleneck in plant science and crop improvement programs, thereby expanding our knowledge concerning the connection between genomics and predictive phenomics of key US crops at different sites across the region. This proposed project is completely aligned with USDA NIFA’s priorities for research to enhance food security and sustainable bioenergy through improved adaptation to climate variability and change.
The synergy of the regional HTP facilities across campuses will provide scientists and engineer unprecedented capability in conducting multi-site, hypothesis-based research. This science will characterize the impacts of biotic and abiotic environment, and production systems on the growth, productivity and resilience of various crops, hence providing greater accuracy in predicting crop performance under variable conditions. This committee will also catalyze and coordinate interdisciplinary research to facilitate the creation of novel knowledge of the impact of genome and environment interaction on plant phenotype, which eventually could result in increased productivity and sustainability of our agro-ecosystems. These efforts to develop complete pipelines for measuring and predicting plant productivity and performance from multi-modal data, and linking all the way to changes in genotypes of individual plants, is unprecedented, and is key for both mitigating climate change and sustainable agriculture intensification.
Related, Current and Previous Work
Multiple institutions in the NC region have invested heavily in plant phenomics research capacity in recent years. As founding members of this committee, we are interested in contributing to this collaborative effort. In the following, we detail each founding institution’s respective strengths that will serve as the basis of cross-institution science.
At Purdue University, existing research teams are already contributing to the Ag-Alumni-Seed Controlled Environment Phenotyping Facility (AAPF) on campus and the Indiana Corn and Soybean Innovation Center (ICSC) for plant phenomics research at the Agronomy Farm. The AAPF is an HTP facility that enables high-throughput, non-destructive measurements on multiple traits of diverse crop species in a growth chamber that supports analyses of up to 256 plants simultaneously under precisely controlled environmental conditions. The ICSC supports field-based phenotyping research on a >1600 acre research farm near campus. The phenotyping gantry in ICSC provides the unique capability to collect multi-mode, shade-less crop images in the field. Plants can be imaged every ten (10) minutes using multiple imaging systems. Similar remote sensing instruments are used in each environment including color (RGB), hyperspectral (400 – 2500 nm), and thermal imaging systems. Light Detection and Ranging (LiDAR) systems are being used for 3-D plant detection and a new X-ray CT system for root imaging. Combining these imaging systems and environments with image analysis pipelines will make it possible to conduct autonomous measurements on various traits of a variety of crops.
The University of Nebraska-Lincoln has made significant investments in transformative HTP technologies at multiple scales. There are two controlled environment platforms and one field platform. The LemnaTec HTS is a growth chamber-sized system designed for imaging short or small plants (e.g., Arabidopsis and turf grass) or emerging seedlings of monocots and dicots (<12 cm in height). The system is equipped with 4 types of imaging cameras (visible, thermal infrared, near infrared, and fluorescence). The LemnaTec3D Scanalyzer is a fully automated and environmentally controlled system with 672 pot capacity located in a greenhouse, with automated watering and weighing stations (for water manipulation), and 4 chambers equipped with cameras capable of collecting images from the top and/or side views (for 3D reconstruction) in the visible, infrared, fluorescent and hyperspectral range of each plant up to maturity or 2.5 m height. The Spidercam© Field Phenomics Facility is located at Eastern Nebraska Research and Extension Center, MEAD (ENREC). This is a 1 acre field phenotyping site that features the automated cable-driven robotic system (Spidercam©, Austria) that carries multiple cameras (e.g., visible, near infra- red, hyperspectral, and multispectral) and sensors (e.g., micrometeorological, and LIDAR scanner), which can be accurately positioned over a plant or a plot for sensing and plant imaging. This is a high-resolution platform that can image plants/plots from germination to 6 m in height. This is the only Spidercam platform in the USA and the second in the world. The field site is also equipped with an advanced automated weather station, and a state-of-the-art subsurface drip irrigation system for precision water application at plot level (15*20 ft2). In addition, UNL and through the Center for Advanced Land Management and Information Technology (CALMIT) offers unique capabilities through its aircraft with Hyperspectral and Fluorescence capabilities, and tractor technologies.
Iowa State University established the ENVIRATRON, which is a phenomics platform that enables researchers to monitor the performance of plants throughout their lifespan when subjected to a variety of environmental conditions, including anticipated future environments. The ENVIRATRON permits researchers to incrementally alter critical variables to better simulate changing conditions that we face in the future. The ENVIRATRON enables control of variables including temperature, day length, light intensity, humidity, CO2 levels and water potential in the soil and will be able to simulate current climatic conditions in different areas of the world and future climatic scenarios. The ENVIRATRON consists of an array of plant growth chambers to create different environmental conditions. Unlike commercial plant phenomics systems, plants are not conveyed out of the growth chambers to monitor their growth performance; instead, a rover with a robotically controlled arm periodically visits each chamber to image and analyze the plants. In addition to more standard RGB, fluorescence, near IR and IR imaging, sensors on the rover provide hyperspectral and holographic imaging and Raman spectroscopy. The robot-assisted sensing approach enables precise location-specific data acquisition, resulting in improved sampling strategies and data quality.
The University of Minnesota has invested heavily in informatics and data management capabilities along with distributed low-cost sensing for phenotyping and envirotyping in recent years. As a founding member of this committee, UMN is interested in also contributing to this collaborative effort. These technologies are based in and maintained by the GEMS Informatics Center. The GEMS Informatics ecosystem consists of (1) an informatics platform that supports metadata creation and standardization, data cleaning, management, smart sharing, and analytics tools; (2) internet of things hardware and software technologies that connect scientific instrumentation in real-time to the GEMS core tools; and (3) GEMS API’s that include harmonized global gridded meteorological products, breeding tools for predicting GxExM performance, and pedigree cleaning, among others in the growing portfolio of GEMS API’s.
Other similar projects/committees
There are fifty projects in the CRIS system that are related to plant phenotyping directly or indirectly, but with very diverse research interests that are different from what we are proposing. While we are proposing a synergizing mechanism to facilitate research in sensor development and application, as well as data analysis and management platforms to facilitate large scale research collaboration, of the above mentioned projects, seventeen (17) are research on cropping system and crop genetic improvement for specific stress tolerances, eleven (11) are related to leveraging the development in automation or plant sensing technologies to facilitate improvement in breeding and management of specialty crops. Seven (7) of these projects focus on leveraging progress in data sciences/cyber systems in processing, management plant management data, four (4) of them are research on precision/smart-farm technologies, the rest are either about application of CRISP or automation technologies in crop breeding or management, or workshops as well as establishing research community. Two specific project, The BTT EAGER, Utilizing high throughput phenotyping approaches to advance plant breeding (1018322), and Integrated systems research and development in automation and sensors for sustainability of specialty crops (1017805), come with similar focus as what are proposed in this project. However, the former works only on improving corn breeding leveraging phenotyping technologies, while the later focuses more on application of automation and sensing technologies in specialty crop production.
There are four committees in the NIMSS (NC1210, NCERA 180, W3009, S1069) that specifically referred to the application of plant phenotyping technologies. However, NC1210 and S1069 focus on leveraging UAS to collect crop performance data, NCERA180 focuses more on precision agriculture rather than plant phenomics/phenotyping, and W3009 focuses on leveraging sensors and robotics exclusively on specialty crop-related studies. For this project, collecting remote sensing data and metadata in crop performance assessment and quantification is just one organic part of the overall goals. Development of proximal and remote-sensing platforms and novel sensor systems will also be emphasized in this project. High-throughput plant phenotyping systems development, application of high-throughput phenotyping systems in controlled-environments, development of data analysis methods and data management platforms are another aspect that differentiate the proposed project from the above committees. The outcomes from this project will benefit the work of researchers contributing to NC1210, NCERA180, W3009, and S1069.
Objectives
-
Improving crop production and performance to accommodate a growing global population and mitigate for climate change is one of the most important challenges for humanity in the 21st Century. Developing high-throughput phenotyping (HTP) strategies to evaluate crop plants for functional traits required to stabilize yield under variable environments and extreme weather events will be fundamentally important to a sustained increase in agricultural productivity. The NC plant phenotyping committee will catalyze and coordinate fundamental research to establish in-depth connections between the genomic background, phenotypic parameters, and the environmental and management contexts of key crops in the region. Although the project will support collaborative and multi-disciplinary research across many different plant species to develop HTP approaches for key functional traits, this committee will initiate pilot studies in maize to produce public data sets that support development of technologies and tools for data acquisition and processing, data analysis, and new methods in gene discovery that can be transferred to other species and systems as the research community grows.
Comments: Pilot study in maize phenotyping This committee will initiate pilot studies in maize phenotyping in controlled and field environments to produce public data sets that support development of technologies, tools, and methods for (Objective 1) data acquisition and processing, (Objective 2) data analysis, and (Objective 3) new methods in gene discovery that can be transferred to other species and systems as the research community grows. A panel of diverse maize inbred and hybrid genotypes will be planted and phenotyped manually and by remote sensing in controlled and field production environments in multi-location trials. Other crops and research communities will be integrated as interest grows. Teams and sub-teams at each of the founding institutions as well as future collaborators will conduct research to create a NC phenotyping network that does not exist today. Collaborators at Purdue, UNL, and ISU will conduct joint research projects that link current and future phenotyping platforms to support research studies on crops that are important to the region beginning with pilot studies on corn. These collaborations will support development of new technologies and platforms for data acquisition and processing and integration of phenomic and genomic models for trait prediction. These teams and collaborators at the UM will use data from these studies to create and support a regional data sharing and modelling initiative that supports processing, storage, and management of phenotypic data as well as systems that encourage data sharing and respect intellectual property across plant phenotyping facilities and infrastructures. -
Novel technologies and platforms for data acquisition and processing will be developed for application in plant phenotyping research.
Comments: a. An array of remote sensing instruments and platforms will be developed and adapted to monitor plants in controlled and field environments. b. Systems and methods will be developed to integrate multiscale sensing for data acquisition and processing. c. New techniques to advance FAIR(ER) systems will be established to support processing, storage, and management of phenotypic data. d. Data access mechanisms will be developed that encourage data sharing and respect intellectual property across plant phenotyping facilities and infrastructures. -
We will develop fundamental methods in data analysis to integrate multi-modal and multi-temporal data for trait prediction.
Comments: a. New methods will be developed to integrate multidimensional data from multiple sources (e.g. genetics, management, and environment) across space and time. b. Mechanistic and empirical models will be developed to support trait prediction. c. High-performance systems and intelligent visualization will be developed. -
We will explore methods to ascertain the integration of phenomic and genomic models to predict traits.
Comments: a. Methods will be developed to predict phenotypes from multi-view image sequences captured by cameras in different modalities (e.g., visible light, fluorescent, hyperspectral, and infra-red) in diverse germplasm, environments and management systems. b. Genetic studies will be conducted to discover plant genes that contribute to agricultural productivity, sustainability, profitability and/or quality.
Methods
1. Novel technologies and platforms for data acquisition and processing will be developed for application in plant phenotyping research.
Remote sensing instruments to monitor plants in controlled and field environments
An array of remote sensing techniques will be developed and leveraged in plant monitoring in controlled and field production environments.
RGB imaging: These imaging techniques will be used to explore static or dynamic plant characteristics based on time-series measurement of plants and to quantify dynamic plant traits such as biomass accumulation, leaf expansion, stem elongation, and fruit development. These traits are impractical to measure with the traditional destructive sampling method, but can be reliably estimated from time-series images of the plants. Three dimensional (3D) sensing technologies will be heavily leveraged in measurement of the variation in plant morphology and structure.
Multispectral and narrow-band hyperspectral (HS) imaging, which includes imaging in the Visible/NearInfraRed (VNIR) and Short-wave infrared (SWIR) spectrum ranges These imaging techniques will generate spectral information that can be used to predict plant leaf traits related to nutrient concentration (such as nitrogen, phosphorus, and potassium), physiology (such as chlorophyll, water content, and photosynthesis parameters), and metabolites (such as sucrose). Our research activities will focus on the deployment of hyperspectral sensors in controlled and field environments to acquire high-quality hyperspectral data of plants, as well as development of new spectral processing and modeling approaches to relate hyperspectral data to the target traits.
Thermal infrared (IR) imagery: Thermal IR radiometry can be used to measure plant traits related to stomatal conductance and transpiration. Leaf stomata regulate the process of gas exchange between the plant and the atmosphere, and therefore play an important role in plant growth and water use. Thermal infrared sensing of leaf surface temperature is proved to be an indirect but effective approach to estimate leaf stomatal conductance, and shows the potential to rapidly differentiate genotypes for water-use related and drought stress related traits.
X-ray Computed Tomography (X-ray CT): X-ray CT will be used to characterize above and below-ground plant traits including Root System Architecture (RSA). X-ray CT brings the capability to non-destructively scan and quantify root systems growing in soil/soil-like growth media in 3D over multiple days. Recent progress in X-ray image processing has led to significant improvements in 3D RSA reconstruction and will be used to address fundamental questions in root biology such as (1) what genes control root branching in crops, (2) how roots respond to changes in environmental conditions and what genes triggers these responses, as well as (3) how roots respond to different soil physical properties and nutrient conditions.
Chlorophyll fluorescence imaging: These systems will be used to acquire information on key photosynthesis processes and biochemical pathways that are directly or indirectly related to photosynthesis (Kalaji et al, 2016; Chen et al., 2019). Knowledge established using these systems will facilitate the determination of the mechanisms(s) for desirable traits which would lead to strategies for enhanced stress tolerance and improved yield potential in a wide variety of crops in different climate zones.
Light Detection and Ranging (LiDAR): Discrete return LiDAR will provide information required for extracting plant structure including plant height, geometric structure, and canopy architecture.
Remote sensing platforms
Numerous sensor platforms have been developed for plant phenotyping in controlled and field production environments including manually operated carts (Bai et al., 2016; Jimenez-Berni et al., 2018), tractor- and sprayer-based vehicles (Andrade-Sanchez et al., 2014; Jiang et al., 2018), field robots (Underwood et al., 2017; Shafiekhani et al., 2017), UAS (Sankaran et al., 2015; Shi et al., 2017), fixed overhead gantry/cable-driven systems (Virlet et al., 2017; Kirchgessner et al., 2017), and stationary sensor networks. In this project, we will further develop and explore new applications of these platforms for various crop species and environmental conditions.
UAS-based platforms will be developed to incorporate more advanced sensors for field phenotyping. Advanced sensors are challenging for UAS because more sophisticated integration between the sensors and the UAS platform and more rigorous sensor calibration are needed for high quality data collection.
Ground-based robotic systems and platforms will be developed to support field phenotyping applications. Ground robotic systems can have a larger sensor payload than UAVs and images from the ground robotic systems are usually of high resolution. Research efforts will focus on innovations in phenotyping robotic systems including development of robotic platforms that can automatically and persistently navigate in crop fields, new contact and non-contact probing devices that can measure traits from leaves, stems, panicles, and other plant organs, and new coordination schemes between the ground robots and UAVs.
Sensor-network-based platforms will also be developed for phenotyping. There are two advantages of the sensor network approach compared to other platforms. First, soil sensors can be incorporated into the sensor node along with the plant sensors, relevant soil properties such as soil temperature, soil moisture, organic matter, and pH can be measured and recorded at higher spatial density. Second, sensors on the stationary sensor network can take measurements at very high temporal resolution and will enable new information being captured and analyzed (for example, event detection related to disease inception), as well as the new methods of data analysis (deep learning with videos).
Systems and methods to integrate multiscale sensing for data acquisition and processing
Multiscale sensing includes sensors that are placed in indoor facilities, static or mobile in the field, in the air deployed on unmanned aerial vehicles, or in outer space on satellites. Integrating across different scales and sensing platforms (with varying levels of noise in the data) presents challenges in data integration and management, and also demonstrates the need of research on systems and methods to maintain privacy and security of data. Traditional and new approaches in other fields such as computer science and the geospatial sciences will be evaluated and adapted to address these issues, including analyses of ontology creation and integration across the plant and computational sciences to support automated reasoning (e.g. see Haller et al., 2017; OGC, 2016; Singleton et al., 2016; Gooch and Chandrasekar, 2017), sensor data provenance approaches to specify the data quality from point of collection through to use (Fredericks and Botts, 2018), data harmonization approaches (Kugler et al., 2015; Alber et al., 2019), and mechanisms for decentralized secure and private data management and sharing (Truong et al., 2019).
New techniques to support processing, storage, and management of phenotypic data
Data storage, processing, and managing systems are the backbone of phenotyping. These multiple data types at different spatiotemporal scales often lack the necessary metadata that make them findable, accessible, interoperable, and reusable. Furthermore, data need to be managed ethically, respecting informal and formal intellectual property, and connected to reproducible scientific workflows. These principles apply to farmer, scientific, and agribusiness generated data sources. Identifying and deploying new techniques to advance such FAIR(ER) systems is a critical research objective (Wilkinson et al., 2016), including, but not limited to, advances in ontology standardization, spatiotemporal data harmonization, and genomics x environment x management integration (Allan et al., 2017). Other challenges in data platform development that will be addressed include efforts to study: (1) the linkage of secure cloud computing environments with decentralized architectures data collection, storage, and processing systems (see Lavassani et al. 2018), (2) semi- and fully-automated phenotyping systems management (Debauche et al. 2017), (3) selective storage and archival procedures and systems to manage mid- and long-term data (see National Agricultural Library, Ag Data Commons; Beagrie 2006), and (4) developing modularized data transfer standards and systems that tightly couple metadata and data (e.g. GEMS 2020; OpenTEAM 2020; OATS 2020; Selby et al. 2019).
Data access mechanisms that encourage data sharing and respect intellectual property across plant phenotyping facilities and infrastructures
Phenomics at scale requires access to interoperable data across centers and sectors. Today, much of the phenomics data exist in the private sector or in inaccessible forms in research labs. Creating a robust phenomics data sharing ecosystem requires standardizing workflows and protocols in a way that also respects intellectual property. This objective will focus on developing the expertise, scale, workflows, and standardized approaches that are deemed broadly acceptable to both private industry and public research labs. This will unlock the scientific and economic promise of cross-center and cross-organization crop phenomics. In particular, we will advance existing state-of-the-art approaches to secure data sharing and storage architectures (Allan et al., 2017). These include decentralized and networked approaches to shared computing resources across different federated hubs (Celesti et al. 2016) and furthering secure, multi-party computation approaches in ways tailored for the plant sciences (Cramer et al. 2015). Work in such spaces would require additional advances in computing infrastructure to support decentralized model training (e.g. horizontal and vertical federated learning; Yang et al. 2019), as well as many other domains.
2. We will develop fundamental methods in data analysis to integrate multi-modal and multi-temporal data for trait prediction.
New methods to integrate multidimensional data from multiple sources across space and time
The multi-modal data generated in the project will be from multiple sources and dimensions including the genomic, weather, soil, and phenotyping data from various indoor and field based sensors across geographical areas and seasons. Determination of how plants respond to the environment has become possible due to advances in high-throughput imaging technologies coupled with advances in deep learning and image analysis (Singh, 2016; Pound, 2017; Namin, 2017). Current models that are built on the legacy of linear responses have good predictive ability (Heslot, 2012) but become limited when trying to predict phenotype and genotype into new parameter spaces, e.g., new genotype combinations and new, previously untested (or changing) environments (Burgueño, 2012). In this project, we will develop predictive models that integrate the latest machine learning (ML) and artificial intelligence (AI) techniques and push the frontier of data science. Such new models will enable us to capture and understand the effects of gene-by-environment (and by-management) interactions on phenotype that are needed to provide predictions for breeding cultivars for future and changing climates (National Academies of Sciences, 2018).
Time series modeling for phenotypic trait prediction can also provide new opportunities for plant phenotyping by analyzing an image sequence as a discrete time series. The proposed research will consider modeling of a phenotypic time series to (1) predict phenotypes for missing imaging days due to mechanical breakdown of the system or for a time in the future based on analyzing past measurements; (2) predict a composite phenotype from its one or more constituents and (3) bridge the phenotype-genotype gap to contribute in the study of improved crop breeding and understanding the genetic regulation of temporal variation of phenotypes.
Integrating Mechanistic and Empirical Models to Support Trait Prediction
While empirical methods have been used to predict and model complex plant phenotypes under dynamic environmental conditions, modeling crop performance for complex traits is a challenge given the impacts of genotype by environment interactions (Cooper et al. 2002). We will explore methods that integrate (1) simplified crop growth models to predict how relatively fixed traits (e.g. leaf canopy architecture, relative flowering time) play different and non-linear roles in overall plant performance under different environmental conditions or agronomic management regimes, (2) evolutionary models that simplify the use of simplified crop growth models (CGMs) to predict how various traits will effect field yield in different environments (Millet et al., 2019; Technow et al., 2015), (3) evolutionary algorithms to simplify and reduce the dimensionality of environmental data to a core subset of traits that impact plant growth. Well-developed crop growth models as well as high-throughput phenotyping approaches have been developed in recent years (Demarez et al., 2008; Casa et al., 2010; Parent et al., 2019; Jiang et al., 2019); however, strategies that accommodate crop growth models as part of high-throughput phenotyping pipelines have not been thoroughly explored. Efforts will be made to develop phenotyping analysis workflows and scenario analysis tools for execution of crop models for experimental plots based on observed and remotely sensed phenotypic data.
Development and utilization of high-performance systems and intelligent visualization
Compared to many existing machine-learning approaches, the main challenges with our target research are two folds. First, our design needs to holistically consider highly complex data to enable interactive exploration that links multiple views of various different data representations. Second, various machine-learning techniques are characterized by different data access and communication patterns (Dryden et al., 2019), making it extremely difficult to design a high-performance system in support of these techniques in a unified fashion. We will develop high-performance system support that will directly account for partitioning and distribution schemes, stores, and computation models for various data. We will study visualization and interaction techniques to appeal to users’ familiarity with them and facilitate comparison studies exploiting the strength of different machine learning techniques.
3. We will explore methods to explore the integration of phenomic and genomic models to predict traits.
Methods to predict phenotypes in diverse germplasm, environments and management systems
Predicting how different genotypes will perform across diverse environments is a key challenge in plant genetics and plant breeding. Once a line has been genotyped it is generally possible to predict trait values for that line in a given environment if both genetic and trait data is already available for a large number of related lines in that same environment. However, if predictions are being made in a previously untested environment, or the line is not closely related to those lines which have been tested in the environment, the accuracy of prediction drops rapidly.
A number of approaches to predicting phenotype across environments have been explored in the past. The challenge is that there are many environmental factors and few observed environments. One approach to integrating both the impact of environmental factors and the genotype by environmental factor interactions directly within a genomic prediction model (Jarquín et al., 2014; Jarquín et al., 2017) requires more data than is presently available. We will seek to address this challenge by both working collaboratively across the multi-state hatch project to grow large trials of common genotypes across many controlled and field production environments under diverse management practices. The proposing institutions have experience working as members of the Genomes to Fields consortium and access to field sites that represent diverse environments and management regimes as part of their research and extension infrastructure.
Once a modeling approach is found to be effective in a single context or for a single species, an area for future research would be the use of domain adaptation to transfer AI models from one crop species or one environment to another. Domain Adaptation is a machine learning setting where data from a source domain is used to predict a target domain, under the assumption that the source and target domains have different distributions (a.k.a., domain shift) but share some similar patterns. In unsupervised domain adaptation, the labels of the source domain data are available while the labels of the target domain data are not available. The task is to learn a model for the target data using the labeled source data and the unlabeled target data.
Genetic studies to discover plant genes that contribute to agricultural productivity, sustainability, profitability and/or quality
Just as limitations on the availability of large scale datasets of common lines in diverse environments have limited the development of tools to predict phenotype across environments, the same limitations on datasets have also constrained researcher’s ability to identify genes that contribute to phenotypic plasticity. We will address this constraint by conducting large-scale trials of crop varieties under diverse environmental conditions to quantify plant responses to the environment. We will identify genes contributing to variation in plant responses using conventional GWAS and transcript level based analysis (TWAS) (Lin et al., 2017; Kremling et al., 2019). These approaches have been shown to complement each other by identifying distinct subsets of genes which each contribute to controlling phenotypic variation for any given trait analyzed using both methods. Specific phenotyping strategies will include:
4D plant phenotyping analysis: While not well-explored, image sequence analysis can address many complex unsolved computer vision tasks in phenotyping. We will explore the genetic regulation of the 3D phenotypes as a function of time.
Plant stress classification and analysis of its temporal propagation: Machine learning techniques will be developed to identify signals from hyperspectral imagery for detecting and quantifying abiotic and biotic stresses, and by using suitable supervised and unsupervised classification techniques will classify the stress in different levels, e.g., mild, moderate and extreme.
Event-based phenotypes: Machine learning techniques will be used to detect the timing of important events in the life cycle of a plant and use advanced tracking methods to automatically track the newly emerged organ throughout the plant’s life cycle (Samal et al. 2020).
Ecophysiological and biophysical traits: Hyperspectral imaging will be used to predict variation in important plant biophysical and ecophysiological traits (Mazis et al., 2020).
Efforts to model plant responses to the environment will initially utilize Bayesian Finlay-Wilkinson regression (Lian and de los Campos, 2016) to partition variation in traits across environments into trait means, linear plasticity, and nonlinear plasticity (Kusmec et al., 2017; Miao et al., 2020; Wang et al., 2020).
Measurement of Progress and Results
Outputs
- Achieving the stated objectives of this project will push the frontiers of predictive modeling, artificial intelligence, and analytics thereby seizing opportunities presented by the growing availability of data to improve the resilience and efficiency of food and agricultural production.
- New and existing high throughput phenotyping tools and technologies will be developed to provide the essential capabilities for assessing morphological and physiological responses of crops to changing environmental conditions.
- New techniques to advance such FAIR(ER) systems will be established to support processing, storage, and management of phenotypic data.
- Image analysis and data modeling strategies to quantify plant growth and development, morphological and physiological characteristics, and chemical composition.
- Improved models to predict the performance of diverse germplasm in untested environments.
- The integrated high-throughput phenotyping facilities will empower efficient plant biology assessment and gene discovery by leveraging non-invasive imaging, machine vision, spectroscopy driven by automated robotics and automated data pipeline based on high-performance computing.
- Identification of genes controlling target traits that plant breeders can target for introgression into elite lines to develop new cultivars which will improve farmer profitability and sustainability.
- Contribute to the development of next-generation workforce.
- Increase the sustainability and productivity of agroecoystems and improve our capability to mitigate for climate change.
- Deliver science-based information in an effective and efficient way to ensure sustainability and profitability of the land.
Outcomes or Projected Impacts
- Creation of this committee will coordinate multidisciplinary teams of scientists and engineers in the development of high-throughput phenotyping (HTP) facilities and systems in controlled and field environments. These systems will enable researchers to overcome the phenotyping bottleneck in plant science and crop improvement programs, thereby expanding our knowledge concerning the connection between genomics and predictive phenomics of key US crops at different sites across the region. This falls under USDA priority to increase production and food quality and other desirable traits and alleviate stresses, and reduce environmental impacts from climate change and extreme weather events (USDA Blueprint 2020).
- New remote sensing data acquisition and processing capacities will provide regional scientists and engineers un-precedent capability in surveilling and conducting hypothesis-based research that lead to better understanding on the impact of differences in weather, environmental conditions, and production systems on the growth and productivity of various crops, hence providing greater accuracy in predicting crop performance under variable conditions, this is key for mitigating biotic and abiotic stresses.
- Public datasets will enable further development and improvement of predictive models both within the multi-state hatch project and by outside groups unable to support large scale multi-environment field trials.
- Advanced modelling strategies will provide biologists unprecedented capabilities in assessing the response of a wide variety of crops (e.g. corn, sorghum, soybean, wheat) to abiotic and/or biotic stresses, such as nutrient deficiency, drought stress, salt and heavy metal stress, high/low temperature, herbicide injury, or disease/insect infection, across the region.
- Accurate predictive models will accelerate plant breeding, enable exploring the possibility space for plant traits and agronomic practices to identify new optimal combinations of plant properties and management practices to optimize farmer profitability and sustainability. This research effort will facilitate the creation of knowledge of the impact of genome and environment interaction on plant phenotype that will result in the deployment of crops of superior varieties and species. This will lead to the delivery of nutritious and safe food.
- Facilitate rural prosperity and economic development, and thus social stability.
- Develop technology and tools that aid in strengthening stewardship of the land
Milestones
(2023):Develop remote sensing instruments and platforms; Integrate multi-dimensional data from multiple sources.(2024):Develop new techniques to support processing, storage, and management of phenotypic data; Predict plant phenotype in diverse germplasm, environments and management systems
(2025):Encourage data sharing and respect intellectual property across plant phenotyping facilities
(2023):Integrate multiscale sensing for data acquisition and processing; Develop mechanistic and empirical models
(2025):Develop high-performance systems and intelligent visualization Discover plant genes that contributes to agricultural productivity, sustainability, profitability and/or quality
Projected Participation
View Appendix E: ParticipationOutreach Plan
In terms of technology outreach, we aim to deliver science-based information in an effective and efficient way to ensure sustainability and profitability of the land. We will pursue three key outreach activities during this project including (1) publish the results of our work in scientific journals, (2) present the results of this work at national and international conferences (e.g. North American Plant Phenotyping Network), (3) report this work to local crop producers, representatives of local and national crop commodity organizations, and private sector partners interested in developing and commercializing new crop varieties, (4) incorporate research outcomes and tools into classroom learning, and (5) offer virtual and in person training workshops.
Organization/Governance
We propose that the NCR be organized as a collaboration among the founding members as well as researchers at future participating institutions under the direction of a jointly-appointed Executive Committee and Technical Committee.
The Executive Committee will consist of a Chair, Vice-Chair, and Secretary with each officer serving terms of 1-year with the Chair succeeded by the Vice-Chair and the Vice-Chair succeeded by the Secretary at the beginning of each year. Candidates for Secretary will be nominated by the membership of the NCR and selected by majority vote at the annual meeting. The Executive Committee will oversee the administrative functions of the project, plan the annual meeting, and provide reviews and reports as required. The Chair will convene quarterly meetings of the Executive and Technical Committees. The Chair will also convene, on an annual basis, a research summit meeting with the entire team, and will prepare the annual report.
The Technical Committee will provide oversight to the research activities of the program. The Technical Committee will consist of a Director and Associate Director for each of the key research thrusts: (1) data acquisition and processing, (2) fundamental methods in data analyses, and (3) phenotype modeling and gene discovery. The Director and Associate Director will serve 1-year terms with the Director succeeded by the Associate Director at the beginning of each year. Candidates for Associate Directors will be nominated from the membership and be selected by majority vote at the annual meeting. Each thrust has two co-leads to increase communication and synergy. The Technical Committee will meet quarterly with participating members and be responsible for drafting yearly reports on their progress and outputs.
The project will be initiated with leadership from Chair Mitch Tuinstra, Vice-Chair Tala Awada, and Secretary Patrick Schnable. The Directors and Associate Directors for the Technical Committee will include Yufeng Ge and Bryan Runck for data acquisition and processing, Yang Yang and Jennifer Clark for fundamental methods in data analysis, and James Schnable and Diane Wang for phenotype modeling and gene discovery.
The team will incorporate the following principles for governance to: 1) Provide adequate administrative and scientific infrastructure to enable this complex multidisciplinary project; 2) Support collaborations; 3) Develop short-term, medium-term, and long-term deliverables; and 4) Establish an inclusive environment that fosters flexibility and responsiveness. The governance of this project depends on clear plans for communication. As outlined above, there are established schedules for interaction and clear lines of responsibility both top-down and across the research areas.
Literature Cited
Alber, M., Tepole, A. B., Cannon, W. R., De, S., Dura-Bernal, S., Garikipati, K., Kuhl, E. 2019. Integrating machine learning and multiscale modeling—perspectives, challenges, and opportunities in the biological, biomedical, and behavioral sciences. NPJ digital medicine, 2(1), pp. 1-11.
Allan, G., Erdmann, J., Gustafson, A., Joglekar, A., Milligan, M., Onsongo, G., Zhou, P.,2017. G.E.M.S.: An Innovative Agroinformatics Data Discovery and Analysis Platform.
Aminikhanghahi, S. and Cook, D.J., 2017. A survey of methods for time series change point detection. Knowledge and information systems, 51(2), pp.339-367.
Andrade-Sanchez, P., Gore, M.A., Heun, J.T., Thorp, K.R., Carmo-Silva, A.E., French, A.N., Salvucci, M.E. and White, J.W., 2014. Development and evaluation of a field-based high-throughput phenotyping platform. Functional Plant Biology, 41(1), pp.68-79.
Angelopoulou, T., Tziolas, N., Balafoutis, A., Zalidis, G. and Bochtis, D., 2019. Remote sensing techniques for soil organic carbon estimation: A review. Remote Sensing, 11(6), p.676.
Bai, G., Ge, Y., Hussain, W., Baenziger, P.S. and Graef, G., 2016. A multi-sensor system for high throughput field phenotyping in soybean and wheat breeding. Computers and Electronics in Agriculture, 128, pp.181-192.
Bai, G., Ge, Y., Scoby, D., Leavitt, B., Stoerger, V., Kirchgessner, N., Irmak, S., Graef, G., Schnable, J. and Awada, T., 2019. NU-Spidercam: A large-scale, cable-driven, integrated sensing and robotic system for advanced phenotyping, remote sensing, and agronomic research. Computers and Electronics in Agriculture, 160, pp.71-81.
Beagrie, N., 2006. Digital Curation for Science, Digital Libraries, and Individuals. The International Journal of Digital Curation. 1(1), pp. 3-16
Bühlmann, P. and Van De Geer, S., 2011. Statistics for high-dimensional data: methods, theory and applications. Springer Science & Business Media.
Burgueno, J., Campos, G., Weigel, K., and Crossa, J. 2012. Genomic prediction of breeding values when modeling genotype x environment interaction using pedigree and dense molecular markers. Crop Sciences, 52(2), pp. 707-719
Casa, R., Beret, F., Buis, S., Lopez-Lozano, R., Pascucci, S., Palombo, A., 2010. Estimation of maize canopy properties from remote sensing by inversion of 1-D and 4-D models. Precision Agriculture, 11(4), pp. 319-334.
Celesti, A., Fazio, M., Giacobbe, M., Puliafito, A., & Villari, M., 2016. Characterizing cloud federation in IoT. In 2016 30th International Conference on Advanced Information Networking and Applications Workshops (WAINA), pp. 93-98.
Chen, Y., Ribera, J. and Delp, E.J., 2018, April. Estimating plant centers using a deep binary classifier. In 2018 IEEE Southwest Symposium on Image Analysis and Interpretation (SSIAI)( pp. 105-108). IEEE.
Chen, Y., Ribera, J., Boomsma, C. and Delp, E.J., 2017, September. Plant leaf segmentation for estimating phenotypic traits. In 2017 IEEE International Conference on Image Processing (ICIP) (pp. 3884-3888). IEEE.
Chen, Y.E., Su, Y.Q., Zhang, C.M., Ma, J., Mao, H.T., Yang, Z.H., Yuan, M., Zhang, Z.W., Yuan, S. and Zhang, H.Y., 2018. Comparison of photosynthetic characteristics and antioxidant systems in different wheat strains. Journal of Plant Growth Regulation, 37(2), pp.347-359.
Chen, Y.E., Wu, N., Zhang, Z.W., Yuan, M. and Yuan, S., 2019. Perspective of monitoring heavy metals by moss visible chlorophyll fluorescence parameters. Frontiers in plant science, 10, p.35.
Clark, R.T., Famoso, A.N., Zhao, K., Shaff, J.E., Craft, E.J., Bustamante, C.D., McCouch, S.R., Aneshansley, D.J. and Kochian, L.V., 2013. High‐throughput two‐dimensional root system phenotyping platform facilitates genetic analysis of root growth and development. Plant, cell & environment, 36(2), pp.454-466.
Cooper, M., Chapman, S.C., Podlich, D.W. and Hammer, G.L., 2002. The GP problem: quantifying gene-to-phenotype relationships. In silico biology, 2(2), pp.151-164.
Cotrozzi, L., Peron, R., Tuinstra, M.R., Mickelbart, M.V. and Couture, J.J., 2020. Spectral phenotyping of physiological and anatomical leaf traits related with maize water status. Plant Physiology, 184(3), pp.1363-1377.
Cramer, R., Damgård, I. B., & Nielsen, J. B. 2015. Secure multiparty computation. Cambridge University Press.
Choudhury, S.D., Maturu, S., Samal, A., Stoerger, V., and Awada, T. 2020. Leveraging Image Analysis to Compute 3D Plant Phenotypes based on Voxel-Grid Plant Reconstruction. Frontiers in Plant Science, in press.
Debauche, O., Mahmoudi, S., Manneback, P., Massinon, M., Tadrist, N., Lebeau, F., & Mahmoudi, S. A., 2017. Cloud architecture for digital phenotyping and automation. In 2017 3rd International Conference of Cloud Computing Technologies and Applications (CloudTech), pp. 1-9.
Demarez, V., Duthoit, S., Beret,m F., Weiss, M., and Dedieu, G., 2008. Estimation of leaf area and clumping indexes of crops with hemispherical photographs. Agricultural and Forest Meteorology, 148, pp. 644 - 655.
Downie, H., Holden, N., Otten, W., Spiers, A.J., Valentine, T.A. and Dupuy, L.X., 2012. Transparent soil for imaging the rhizosphere. PLoS One, 7(9), p.e44276.
Dryden, N., Maruyama, N., Moon, T., Benson, T., Snir, M. and Van Essen, B., 2019, November. Channel and filter parallelism for large-scale CNN training. In Proceedings of the International Conference for High Performance Computing, Networking, Storage and Analysis (pp. 1-20).
Elbahnasawy, M., Shamseldin, T., Ravi, R., Zhou, T., Lin, Y.J., Masjedi, A., Flatt, E., Crawford, M. and Habib, A., 2018, July. Multi-sensor integration onboard a UAV-based mobile mapping system for agricultural management. In IGARSS 2018-2018 IEEE International Geoscience and Remote Sensing Symposium (pp. 3412-3415). IEEE.
Fan, J. and Li, R., 2001. Variable selection via nonconcave penalized likelihood and its oracle properties. Journal of the American statistical Association, 96(456), pp.1348-1360.
Fredericks, J., & Botts, M. 2018. Promoting the capture of sensor data provenance: a role-based approach to enable data quality assessment, sensor management and interoperability. Open Geospatial Data, Software and Standards, 3(1), 3.
Gamon, J.A., Somers, B., Malenovský, Z., Middleton, E.M., Rascher, U. and Schaepman, M.E., 2019. Assessing vegetation function with imaging spectroscopy. Surveys in Geophysics, 40(3), pp.489-513.
Gooch, R. and Chandrasekar, V., 2017. Integration of real-time weather radar data and Internet of Things with cloud-hosted real-time data services for the geosciences (CHORDS). IEEE International Geoscience and Remote Sensing Symposium (IGARSS), Fort Worth, TX, pp. 4519-4521.
Goodfellow, I., Bengio, Y., Courville, A. and Bengio, Y., 2016. Deep learning (Vol. 1, No. 2). Cambridge: MIT press.
Grapov, D., Fahrmann, J., Wanichthanarak, K. and Khoomrung, S., 2018. Rise of deep learning for genomic, proteomic, and metabolomic data integration in precision medicine. Omics: a journal of integrative biology, 22(10), pp.630-636.
Gururani, M.A., Venkatesh, J. and Tran, L.S.P., 2015. Regulation of photosynthesis during abiotic stress-induced photoinhibition. Molecular plant, 8(9), pp.1304-1320.
Habib, A., Han, Y., Xiong, W., He, F., Zhang, Z. and Crawford, M., 2016. Automated ortho-rectification of UAV-based hyperspectral data over an agricultural field using frame RGB imagery. Remote Sensing, 8(10), p.796.
Habib, A., Xiong, W., He, F., Yang, H.L. and Crawford, M., 2016. Improving orthorectification of UAV-based push-broom scanner imagery using derived orthophotos from frame cameras. IEEE Journal of Selected Topics in Applied Earth Observations and Remote Sensing, 10(1), pp.262-276.
Haller, A., Janowicz, K., Cox, S. J. D., Lefrançois, M., Taylor, K., Le Phuoc, D., … Stadler, C. (2018). The modular SSN ontology: A joint W3C and OGC standard specifying the semantics of sensors, observations, sampling, and actuation. Semantic Web, 10(1), 9–32. https://doi.org/10.3233/SW-180320
He, F., Zhou, T., Xiong, W., Hasheminnasab, S.M. and Habib, A., 2018. Automated aerial triangulation for UAV-based mapping. Remote Sensing, 10(12), p.1952.
Helslot, N., Yang, H., Sorrells, M.E., and Jannink, J., 2012. Genomic selection in plant breeding: A comparison of models. Crop Sciences, 52(1), pp. 146-160
Hochreiter, S. and Schmidhuber, J., 1997. Long short-term memory. Neural computation, 9(8), pp.1735-1780.
Iyer-Pascuzzi, A.S., Symonova, O., Mileyko, Y., Hao, Y., Belcher, H., Harer, J., Weitz, J.S. and Benfey, P.N., 2010. Imaging and analysis platform for automatic phenotyping and trait ranking of plant root systems. Plant physiology, 152(3), pp.1148-1157.
Jarquín, D., Crossa, J., Lacaze, X., Du Cheyron, P., Daucourt, J., Lorgeou, J., Piraux, F., Guerreiro, L., Pérez, P., Calus, M. and Burgueño, J., 2014. A reaction norm model for genomic selection using high-dimensional genomic and environmental data. Theoretical and applied genetics, 127(3), pp.595-607.
Jarquín, D., Lemes da Silva, C., Gaynor, R.C., Poland, J., Fritz, A., Howard, R., Battenfield, S. and Crossa, J., 2017. Increasing genomic‐enabled prediction accuracy by modeling genotype× environment interactions in Kansas wheat. The plant genome, 10(2), pp.1-15.
Jeudy, C., Adrian, M., Baussard, C., Bernard, C., Bernaud, E., Bourion, V., Busset, H., Cabrera-Bosquet, L., Cointault, F., Han, S. and Lamboeuf, M., 2016. RhizoTubes as a new tool for high throughput imaging of plant root development and architecture: test, comparison with pot grown plants and validation. Plant methods, 12(1), p.31.
Jiang, Y., Li, C., Robertson, J.S., Sun, S., Xu, R. and Paterson, A.H., 2018. GPhenoVision: A ground mobile system with multi-modal imaging for field-based high throughput phenotyping of cotton. Scientific reports, 8(1), pp.1-15.
Jiang, L., Sun, L, Ye, M., Wang, J., Wang, Y., Bogard, M., Lacaze, X., Fournier, A., Beauchene, K., Gouache, D., 2019. Functional mapping of N deficiency-induced response in wheat yield-component traits by implementing high-throughput phenotyping, Plant Journal, 97, pp. 1105-1119
Jimenez-Berni, J.A., Deery, D.M., Rozas-Larraondo, P., Condon, A.T.G., Rebetzke, G.J., James, R.A., Bovill, W.D., Furbank, R.T. and Sirault, X.R., 2018. High throughput determination of plant height, ground cover, and above-ground biomass in wheat with LiDAR. Frontiers in plant science, 9, p.237.
Kalaji, H.M., Jajoo, A., Oukarroum, A., Brestic, M., Zivcak, M., Samborska, I.A., Cetner, M.D., Łukasik, I., Goltsev, V. and Ladle, R.J., 2016. Chlorophyll a fluorescence as a tool to monitor physiological status of plants under abiotic stress conditions. Acta physiologiae plantarum, 38(4), p.102.
Kirchgessner, N., Liebisch, F., Yu, K., Pfeifer, J., Friedli, M., Hund, A. and Walter, A., 2017. The ETH field phenotyping platform FIP: a cable-suspended multi-sensor system. Functional Plant Biology, 44(1), pp.154-168.
Kremling, K.A., Diepenbrock, C.H., Gore, M.A., Buckler, E.S. and Bandillo, N.B., 2019. Transcriptome-wide association supplements genome-wide association in Zea mays. G3: Genes, Genomes, Genetics, 9(9), pp.3023-3033.
Kugler, T. A., Van Riper, D. C., Manson, S. M., Haynes II, D. A., Donato, J., & Stinebaugh, K. 2015. Terra Populus: Workflows for integrating and harmonizing geospatial population and environmental data. Journal of Map & Geography Libraries, 11(2), pp. 180-206.
Kusmec, A., Srinivasan, S., Nettleton, D. and Schnable, P.S., 2017. Distinct genetic architectures for phenotype means and plasticities in Zea mays. Nature plants, 3(9), pp.715-723.
Lavassani, M., Forsström, S., Jennehag, U., & Zhang, T. 2018. Combining fog computing with sensor mote machine learning for industrial IoT. Sensors, 18(5), 1532.
Le Marié, C., Kirchgessner, N., Marschall, D., Walter, A. and Hund, A., 2014. Rhizoslides: paper-based growth system for non-destructive, high throughput phenotyping of root development by means of image analysis. Plant methods, 10(1), p.13.
Lian, L. and de los Campos, G., 2016. FW: An R Package for Finlay–Wilkinson Regression that Incorporates Genomic/Pedigree Information and Covariance Structures Between Environments. G3: Genes, Genomes, Genetics, 6(3), pp.589-597.
Lin, H.Y., Liu, Q., Li, X., Yang, J., Liu, S., Huang, Y., Scanlon, M.J., Nettleton, D. and Schnable, P.S., 2017. Substantial contribution of genetic variation in the expression of transcription factors to phenotypic variation revealed by eRD-GWAS. Genome biology, 18(1), pp.1-14.
Mathieu, L., Lobet, G., Tocquin, P. and Périlleux, C., 2015. “Rhizoponics”: a novel hydroponic rhizotron for root system analyses on mature Arabidopsis thaliana plants. Plant Methods, 11(1), p.3.
Mazis, A., Choudhury, S.D., Morgan, P.B., Stoerger, V., Hiller, J., Ge, Y. and Awada, T., 2020. Application of high-throughput plant phenotyping for assessing biophysical traits and drought response in two oak species under controlled environment. Forest Ecology and Management, 465, p.118101.
Miao, C., Xu, Y., Liu, S., Schnable, P.S. and Schnable, J.C., 2020. Increased power and accuracy of causal locus identification in time series genome-wide association in sorghum. Plant Physiology, 183(4), pp.1898-1909.
Millet, E.J., Kruijer, W., Coupel-Ledru, A., Prado, S.A., Cabrera-Bosquet, L., Lacube, S., Charcosset, A., Welcker, C., van Eeuwijk, F. and Tardieu, F., 2019. Genomic prediction of maize yield across European environmental conditions. Nature genetics, 51(6), pp.952-956.
Mooney, S.J., Pridmore, T.P., Helliwell, J. and Bennett, M.J., 2012. Developing X-ray computed tomography to non-invasively image 3-D root systems architecture in soil. Plant and soil, 352(1-2), pp.1-22.
Namin, S. T., Esmaeilzadeh, M., Najafi, M., Brown, T. B., and Borevitz, J. O., 2018. Deep phenotyping: deep learning for temporal phenotype/genotype classification. Plant Methods, 14, 66, https://doi.org/10.1186/s13007-018-0333-4
Nguyen, H., Cressie, N. and Braverman, A., 2012. Spatial statistical data fusion for remote sensing applications. Journal of the American Statistical Association, 107(499), pp.1004-1018.
Pandey, P., Ge, Y., Stoerger, V. and Schnable, J.C., 2017. High throughput in vivo analysis of plant leaf chemical properties using hyperspectral imaging. Frontiers in plant science, 8, p.1348.
Parent B, Millet EJ, Tardieu F. 2019. The use of thermal time in plant studies has a sound theoretical basis provided that confounding effects are avoided. Journal of Experimental Botany, 70(9), pp. 2359-2370
Pound, M., Atkinson, J. A., Townsend, A. J., Wilson, M. H., Griffiths, M., Jakson, A.S., Bulat, A., Tzimiropoulos, G., Wells, D. M., Murchie, E.H., 2017. Deep machine learning provides state-of-the-art performance in image-based plant phenotyping. GigaScience, 6(10), pp. 1-10.
Ravi, R., Hasheminasab, S.M., Zhou, T., Masjedi, A., Quijano, K., Flatt, J.E., Crawford, M. and Habib, A., 2019, May. UAV-based multi-sensor multi-platform integration for high throughput phenotyping. In Autonomous Air and Ground Sensing Systems for Agricultural Optimization and Phenotyping IV (Vol. 11008, p. 110080E). International Society for Optics and Photonics.
Ravi, R., Lin, Y.J., Elbahnasawy, M., Shamseldin, T. and Habib, A., 2018. Simultaneous system calibration of a multi-lidar multicamera mobile mapping platform. IEEE Journal of selected topics in applied earth observations and remote sensing, 11(5), pp.1694-1714.
Rellán-Álvarez, R., Lobet, G., Lindner, H., Pradier, P.L., Sebastian, J., Yee, M.C., Geng, Y., Trontin, C., LaRue, T., Schrager-Lavelle, A. and Haney, C.H., 2015. GLO-Roots: an imaging platform enabling multidimensional characterization of soil-grown root systems. Elife, 4, p.e07597.
Richard, C.A., Hickey, L.T., Fletcher, S., Jennings, R., Chenu, K. and Christopher, J.T., 2015. High-throughput phenotyping of seminal root traits in wheat. Plant Methods, 11(1), pp.1-11.
Samal, A., Choudhury, S.D. and Awada, T., 2020. Image-Based Plant Phenotyping: Opportunities and Challenges. Intelligent Image Analysis for Plant Phenotyping, pp.3-24.
Sankaran, S., Khot, L.R., Espinoza, C.Z., Jarolmasjed, S., Sathuvalli, V.R., Vandemark, G.J., Miklas, P.N., Carter, A.H., Pumphrey, M.O., Knowles, N.R. and Pavek, M.J., 2015. Low-altitude, high-resolution aerial imaging systems for row and field crop phenotyping: A review. European Journal of Agronomy, 70, pp.112-123.
Selby, P., Abbeloos, R., Backlund, J. E., Basterrechea Salido, M., Bauchet, G., Benites-Alfaro, O. E., & Vasques Costa, B. 2019. BrAPI—an application programming interface for plant breeding applications. Bioinformatics, 35(20), pp. 4147-4155.
Shafiekhani, A., Kadam, S., Fritschi, F.B. and DeSouza, G.N., 2017. Vinobot and vinoculer: Two robotic platforms for high-throughput field phenotyping. Sensors, 17(1), p.214.
Shi, Y., Thomasson, J.A., Murray, S.C., Pugh, N.A., Rooney, W.L., Shafian, S., Rajan, N., Rouze, G., Morgan, C.L., Neely, H.L. and Rana, A., 2016. Unmanned aerial vehicles for high-throughput phenotyping and agronomic research. PloS one, 11(7), p.e0159781.
Singh, A., Ganapathysubramanian, B., Singh, A.K., and Sarkar, S. 2016. Machine learning for high-throughput stress phenotyping in plants. Trends in Plant Science, 2(2), pp. 110-124
Singleton, A. D., Spielman, S., & Brunsdon, C. 2016. Establishing a framework for Open Geographic Information science. International Journal of Geographical Information Science, 30(8), pp. 507-1521.
Technow, F., Messina, C.D., Totir, L.R. and Cooper, M., 2015. Integrating crop growth models with whole genome prediction through approximate Bayesian computation. PloS one, 10(6), p.e0130855.
Truong, H. T. T., Almeida, M., Karame, G., & Soriente, C. 2019. Towards secure and decentralized sharing of IoT data. In 2019 IEEE International Conference on Blockchain (Blockchain), pp. 176-183.
Underwood, J., Wendel, A., Schofield, B., McMurray, L. and Kimber, R., 2017. Efficient in‐field plant phenomics for row‐crops with an autonomous ground vehicle. Journal of Field Robotics, 34(6), pp.1061-1083.
USDA, 2020. Science Blueprint: A Roadmap for USDA Science From 2020-2025. https://www.usda.gov/sites/default/files/documents/usda-science-blueprint.pdf
Virlet, N., Sabermanesh, K., Sadeghi-Tehran, P. and Hawkesford, M.J., 2017. Field Scanalyzer: An automated robotic field phenotyping platform for detailed crop monitoring. Functional Plant Biology, 44(1), pp.143-153.
Wang, R., Qiu, Y., Zhou, Y., Liang, Z. and Schnable, J.C., 2020. A High-Throughput Phenotyping Pipeline for Image Processing and Functional Growth Curve Analysis. Plant Phenomics, 2020.
Wilkinson, M. D., Dumontier, M., Aalbersberg, I. J., Appleton, G., Axton, M., Baak, A., and Bouwman, J. 2016. The FAIR Guiding Principles for scientific data management and stewardship. Scientific data, 3(1), pp. 1-9.
Yang, Q., Liu, Y., Chen, T., & Tong, Y. 2019. Federated machine learning: Concept and applications. ACM Transactions on Intelligent Systems and Technology (TIST), 10(2), pp.1-19.
Yendrek, C.R., Tomaz, T., Montes, C.M., Cao, Y., Morse, A.M., Brown, P.J., McIntyre, L.M., Leakey, A.D. and Ainsworth, E.A., 2017. High-throughput phenotyping of maize leaf physiological and biochemical traits using hyperspectral reflectance. Plant physiology, 173(1), pp.614-626.
Zhang, Z., Pasolli, E., Yang, H.L. and Crawford, M.M., 2016. Multimetric active learning for classification of remote sensing data. IEEE Geoscience and Remote Sensing Letters, 13(7), pp.1007-1011.