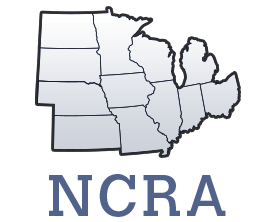
NC1026: Characterize Weed Population Dynamics for Improved Long-Term Weed Management Decision Making (NC202)
(Multistate Research Project)
Status: Inactive/Terminating
NC1026: Characterize Weed Population Dynamics for Improved Long-Term Weed Management Decision Making (NC202)
Duration: 10/01/2005 to 09/30/2011
Administrative Advisor(s):
NIFA Reps:
Non-Technical Summary
Statement of Issues and Justification
Weed science has lagged behind other pest management disciplines in the development of an IPM approach to weed management. One major impediment to developing truly integrated weed management systems is a lack of information on weed biology and ecology at both local and regional scales. Unless all weed management efforts are to be reactive, rather than proactive, we need to begin adressing the question, Why does a given weed species succeed under certain conditions and not others?
The NC-202 project is a well-established group of dedicated scientists from across the north-central region interested in understanding weed/crop interactions on a regional scale thereby facilitating a much broader ecological discussion focused on patterns and processes in the context of weed management decision making. Specifically, we are interested in developing integrated strategies that focus on long-term solutions to complex weed management problems while reducing our reliance on herbicides. Our strategy is to link weed biology/ecology information with economic cost-benefit analysis through bioeconomic weed management decision support systems.
A more unified framework is needed to incorporate risk in the development of weed management recommendations and decision support systems. Demography, or the study of population dynamics, is an excellent unifying structure for weed science. It provides a common language and tools for studying weeds. Most cropping systems of the Corn Belt are dominated by annual weeds, which all have the same basic life cycle: they germinate, grow, and produce seeds that then give rise to new plants or remain dormant in the soil. This permits the comparison of demographic rates within and between annual weed species at different times and locations. Unlike most previous studies of weed population dynamics, which looked at only one or two parameters at a time, the project proposed here will take a whole life cycle approach. This approach will help describe the relationship between different life stages and will allow us to develop a probabilistic understanding of weed populations in response to spatial, temporal and management variation.
Our current research proposal outlines a plan to enhance our understanding of weed population dynamics at both the local and regional scale. The project will focus on deliverables; providing a robust assessment of the future cost of weed escapes that will enable farmers to better balance the short- and long-term objectives of their weed control plans. By leveraging substantial research and development investments in the WeedSOFT decision support system, the NC-202 group will be able to deliver knowledge regarding weed demography to farmers within a familiar and widely distributed framework.
Related, Current and Previous Work
NC-202 research activities have advanced our understanding of local and regional variability in weed/crop competitiveness and the role of weed seedbanks in long-term decision making. To date, data collected on velvetleaf, giant foxtail, and common lambsquarters interference have described the extent of variation that exists among sites and years. Our weed seedbank data shows that seed depth and environmental factors such as moisture and temperature have a significant impact on weed seed dynamics. When the seed persistence data for velvetleaf, common lambsquarters, and giant foxtail are pooled over sites within seedbank burial periods, persistence rates for each burial period are the same across years for each of the species. The ability to forecast weed population dynamics under differing soil management scenarios has thus increased significantly. Our work has been instrumental in improving the weed-crop interference components of decision support tools such as WeedSOFT, a commercially available weed management decision support system available in the following states: Nebraska, Missouri, Kansas, Wisconsin, Illinois, Indiana, and Michigan. Members of NC-202 have met with the WeedSOFT group on numerous occasions to discuss opportunities and plans for integrating information. As of 2004, 620 new, updated, or complementary copies of WeedSOFT were in the hands of stakeholders across seven states in the Midwest. We also actively support the development of other weed management models, such as WeedCast and FoxPatch, which help growers time their weed management based on weed emergence patterns.
One key component missing from current weed management decision support tools is to place weed management within the context of weed demography. How does temporal and spatial variation in weed demography affect the potential success of various weed management tactics? How will outcomes of present management decisions affect weed densities and management options in future years? How large are future costs associated with those weed escapes? What environmental factors are linked to the expansion of weed populations in some areas, but not others? To answer these questions, we will need to focus on weed life cycles, rather than individual stages, as the unit of study.
Population dynamics simulation models have been used previously to aid in weed management decision making (Gonazalez-Andujar and Fernandez-Quintanilla, 1991; Jordan, 1993; Davis et al., 2003). The proposed work will extend the use of such models from the local to the regional scale, and develop recommendation domains and risk estimates from these models. The current group of NC-202 researchers is well-positioned to implement such an approach, and to use the data to their full potential. A CRIS search for the phase weed demography returned 23 projects. NC-202 team members were PIs on five of these projects. Dr. Adam Davis, chair-elect of the NC-202 group, has been part of several studies using the whole life cycle approach to weed demography, coupled with matrix population modeling, to identify achilles heels in weed life cycles, and define recommendation domains for weed management based on model projections (CRIS accession number 0409136, Using demographic models to assess biological control of an invasive plant; CRIS accession number 0192621, Understanding weed dynamics in contrasting crop rotation systems: combining a pulse/chase field experiment with matrix models; Davis et al., 2004). Team member Dr. Edward Luschei has been active in using information on weed demography to develop measures of risk associated with weed management outcomes (CRIS accession number 0190493, An on-farm assessment of weed management decision making under uncertainty).
Our group will study variation in the demography of two model weeds, giant ragweed (Ambrosia trifida) and common sunflower (Helianthus annuus), across the North Central Region. We chose these weeds as model species for several reasons. First, giant ragweed and common sunflower follow gradients of decreasing abundance and weediness from south to north, and west to east, respectively, and are among the most competitive weeds in the central portions of their ranges. Second, both of these species have documented resistance to ALS herbicides (Zelaya and Owen, 2004). Finally, both species have relatively large seeds, permitting very accurate seedbank measurements as a solid foundation for our predictive models. Understanding what drives gradients of successful establishment and competition for these model species will help forecast range expansion for these and other weed species. Such an understanding will also provide essential data for predictions of the spread of herbicide resistant biotypes.
Our measurements of giant ragweed and common sunflower demographic rates will help explain their distribution across the landscape; but the question remains, why do these rates change? Soil heterogeneity has been proposed as one explanation for changes in the relative abundance of plants in terrestrial communities. Variability in soil chemical properties provides limited predictive power for understanding changes in weed abundance across the landscape (Dieleman et al. 2000). Plant-microbe relations may offer better explanations for variation in plant abundance. Feedback between soil microbes and old-field herbaceous plants has been shown to explain approximately 60% of the variation in species abundance in the field (Klironomos 2002). Invasive species showed neutral or positive associations with soil microbes when grown in soil where the same species or other species had previously been grown. In contrast, rare species had negative associations with soil microbes when grown in soil where the same species had been grown before, and neutral associations when grown in soil where different species had previously been grown. We propose to investigate underlying causes of variation in the demographic rates of common sunflower and giant ragweed using the approach pioneered by Klironomos (2002). We will add a new level of inquiry by 1) transferring the protocol to an agricultural system, and 2) examining the stability of plant-soil feedback relationships across the North Central Region.
Research is also needed to expand our understanding of climatic and management factors responsible for this variation. For example, in many situations plants are competing with weeds for water and N. To develop corrective management practices it is important to separate the combined effect of competition-induced yield losses due to water and N stress into the individual components. Smeltekop et al. (2002) used a 13C discrimination () approach to evaluate why intercropped medic (Medicago scetellata) reduced corn yield 32% at Beresford SD. Based on yield data, yield loss was attributed to interplant competition for water. However, results from analysis showed that 23.2% (1689 kg grain/ha) of the yield loss was attributed to competition between medic and corn for N. Based on this analysis it was hypothesized that N placement could be used as a tool to reduce interplant competition for N. The approach also provides a tool to separate losses due to stress into different components during intraspecific competition among C3 plants (wheat) (Clay et al. 2001) and C4 plants (corn) (Clay et al. 2005), adds value to competition studies, gives insight into designing management solutions to reduce yield loss, and reduces the number of treatments needed to evaluate certain aspects of competition. We will use seed production and 13C discrimination to quantitatively identify and separate yield differences due to competition for water and N across the North Central region of the US. Specifically, we want to 1) determine the impact of N and water on yield, N uptake, and of common sunflower and giant ragweed plants grown across the NC region, 2) modify, if necessary, the equations presently used in interpreting in single species models to account for interspecific competition, and 3) to compare the modified approach for assessing plant competition for N and water with traditional approaches for assessing interplant competition for resources.
Objectives
-
1. Quantify the demography of giant ragweed (Ambrosia trifida L.) and common sunflower (Helianthus annuus L.) across the North Central region and use this information to enhance weed management decision support systems.
-
1a. Compare the relative importance of spatial variation in demographic rates at different levels of scale to inter-annual variation within sites.
-
1b. Examine soil feedback mechanisms as they relate to variation in species demography.
-
1c. Partition nutrient and water stress in common sunflower and giant ragweed plants alone and in combination with crops.
-
2. Incorporate weed demographic information into a WeedSOFT module that quantitatively accounts for the economic losses of weed escapes and explore the significance of escapes with a risk-based approach.
Methods
Objective 1. Quantify the demography of giant ragweed (Ambrosia trifida L.) and common sunflower (Helianthus annuus L.) across the North Central region and use this information to enhance weed management decision support systems. Subobjective 1A) Compare the relative importance of spatial variation in demographic rates at different levels of scale to inter-annual variation within sites. It may not be feasible for everyone in our group to complete a detailed field study, especially given time and labor constraints. Therefore, we will give investigators the option of completing protocols that fulfill requirements for one of three datasets; a full, intermediate and reduced dataset (Table 1). Full data sets will comprise whole life cycle measurements of demographic rates for both giant ragweed and common sunflower in weed monocultures and in competition with soybean. We will focus on giant ragweed and common sunflower because they are early emerging weed species, have vastly different geographic ranges (sunflower common in western part of region whereas giant ragweed is common in eastern part of region), and are considered a major weed problem in the NC region. Intermediate data sets will consist of whole life cycle measurements of demographic rates for giant ragweed and common sunflower in monocultures. Reduced data sets will consist of an annual census of mature giant ragweed and common sunflower grown in monoculture. All locations will conduct experiments to fulfill requirements for the reduced set. However, investigators will be strongly encouraged to try the intermediate or full data sets. Two experimental locations (Illinois and Wisconsin) have already pledged to do the full protocol in all years of the study. Full data set (see Figure 1 for schematic of plot layout and data collection) The whole life cycle of giant ragweed and common sunflower will be assessed for three years beginning in September 2005. A randomized complete block will be used with 4-6 replications. This study will also incorporate a nested approach to analysis of variance with random factor levels as follows: rep within site, state within region, and year. The experiment will be split into two main areas comprising a crop and a non-crop component. All data will be obtained from 1 m x 2 m permanent quadrats randomly placed within each cropped and non-cropped area. As previously stated, we are interested in obtaining accurate assessments of weed seedbank and recruitment parameters. However, an accurate assessment of the seedbank will require regular disruption of the soil in the quadrats. Therefore, each 1 m x 2 m quadrat will be split into a left and right 1 m2 quadrat that will be randomly assigned as either below-ground (seedbank) or above-ground (recruitment/fecundity) sub-quadrats. This will allow us to obtain accurate estimates of recruitment and fecundity in an undisturbed environment. Separate quadrats will be used for each weed species. Common accessions of giant ragweed and common sunflower will be obtained from Illinois and Kansas, respectively, and used at all sites. Therefore, a second area immediately adjacent to the experimental plots will be identified and used as a weed seed increase area using these common accessions. Although we recognize the high degree of genetic variation in giant ragweed and common sunflower plants across the region (and its impact on demography), we will first focus on common accessions to avoid the confounding of genetics with environment. However, investigators will have the option of using local accessions along with the common accession if interested. Weed seed increase plots will be established during early spring of 2005. Seedbank persistence and recruitment information will be based on assessments from below-ground quadrats (1 m x 1 m). Six 20 cm by 20 cm by 5 cm deep trays will be placed in each below ground quadrat and buried flush with the soil surface. Seed trays will be constructed with 1 mm square mesh screen to allow for drainage. In September of 2005, soil from one tray within the quadrat will be exhumed and elutriated to determine background seed densities. At the same time, giant ragweed and common sunflower seed from the seed increase plot will be uniformly sown across the entire quadrat area at a density of 200 seeds/m-2. To reduce initial or artificial predation of newly added seed (sometimes known as the buffet effect), straw will be spread uniformly across the quadrat area. In March of 2006, a second tray will be exhumed and seeds recovered through elutriation. Recovered seeds will be tested for viability and data used to determine to seed bank decline over the winter period. In the spring of 2006, seedlings will be counted weekly in all trays. This information will be used to separate seedbank decline due to mortality and seedbank decline due to recruitment. Once seedlings are counted, they will be clipped at the soil surface to prevent seed return. A third tray will be removed and elutriated in September 2006 to determine seedbank decline during the spring/summer period. Immediately afterwards, seed from adjacent seed increase plots will again be uniformly applied to the below ground quadrat. Seeding rate will be determined by an allometric equation describing the relationship between plant biomass and seed production. Per plant seed production will be multiplied by the number of seedlings removed during the spring and summer to arrive at a final seeding rate. Variation in maternal environment during seed development can create a large amount of variability in seed quality (Kegode and Pearce, 1998). Therefore it is critical to the interpretation of our seedbank results that all seed lots be thoroughly characterized. This will entail a two-step procedure. First, the bulk seed rain (all seeds formed on the mother plant) will be analyzed for percent viability, percent insect damage, and percent unfilled seeds. Using an air column separator, the bulk seed will be cleaned to remove the majority of damaged and empty seeds, leaving a primarily viable fraction. Second, the cleaned seed will be subjected to a further characterization for percent viability and dormancy. Because we will start with a precise measure of the viability of the seeds entering the seedbank in the fall, we will be able to make a better determination of seed fate during the course of the experiment. The entire experimental cycle will repeat in 2006-7 and 2007-8. Seedling survival and fecundity information will be based on assessments from above-ground quadrats (1 m x 1 m). In September 2005, seed from the seed increase plots will be broadcast over each above-ground quadrat at a density of 200 seeds m-2. In spring of 2006, seedlings will be counted as they emerge and marked with plastic colored bands. The total number of germinated seeds will be divided by the seedbank density determined in the adjacent below-ground quadrat to estimate percent recruitment. In August 2006, a final census of reproductively mature adults will provide two types of useful data: 1) population size for calculation of population growth rate and direct comparison to the reduced data sets, and 2) seedling survival rate. Mature plants will be harvested at physiological maturity prior to seed dispersal. Potential seed production for each above ground quadrat will be determined with destructive counts of the immature seeds. When seeds in the neighboring seed increase plots are mature, around September 2006, they will be added to each above ground quadrat at the estimated level of seed return. The experimental cycle will repeat in 2006-2007 and 2007-2008. Within-crop plots will be initially established in non-cropped areas. At planting the following spring, seedbank trays are transplanted into plots, as well as soil from AG plots which had over wintered in the non-cropped areas. Following planting through weed seed production, the cropped area plots are treated exactly as shown in Figure 1. After seed production, the trays containing seed from the within-crop plots and the top 10 cm of soil from the AG plots will be moved to the non-cropped area (so that they remain buried over winter) and will be returned again the following year after planting. In other words, we are using the non-cropped area as a refuge so that the cropped area weeds avoid getting dispersed by tillage or soil preparation equipment. Intermediate data set: The protocol for the intermediate data set is the same as for the full data set, except that demographic rates are only measured in non-crop areas. Reduced data set In September 2005, 200 seeds m-2 will be added to 1m2 quadrats in non-crop areas, with separate quadrats for each of the study species. In August of 2006, 2007 and 2008, the number of reproductively mature individuals in each quadrat will be counted, and seed rain density estimated. Plants will clipped immediately afterwards, and when seed from nearby seed increase plot is mature, seed will be broadcast over each quadrat at the measured rate of seed return. Investigators are encouraged to use three cohorts, beginning in 2005, 2006 and 2007. This will allow time to be treated as a random variable, allowing for a full random model, which permits straightforward variance decomposition, as % of SSTO. Data Analysis Demographic parameters will be estimated from the count data in a manner similar to other published weed demographic studies (Davis et al. 2003). Overwinter seedbank persistence: sw = Nseed(mar, t+1)/Nseed(sept, t) Recruitment: g = Nsdl/Nmar, where Nsdl is based on cumulative emergence Spring/summer seedbank persistence: ss = Nseed(sept)/(Nseed(mar)-Nsdl) Plant survival to reproductive maturity: ssdl = Nmplt/Nsdl Fecundity: f Full demographic model construction: Annual transition rule: Nt+1 = Nt*[(sw* ss) + (sw* g*ssdl*f)] Population growth rate: l = Nt+1/Nt We will attempt to parse out the spatial component of variance in l using variance decomposition and mixed-effects models as described by Rao (1997). Coordination between locations: The coordination of research efforts at different locations will take place at our annual meeting in July, and through email and conference calls. In July, 2005, Dr. E. C. Luschei will present preliminary results from an advance study located at the Wisconsin site, and will discuss potential pitfalls that he encountered. Common seed lots for giant ragweed and common sunflower will be distributed by the Illinois and Kansas locations, respectively, to other research sites. 1B) Examine soil feedback mechanisms as they relate to variation in species demography. At each study location, greenhouse bioassays will be performed using a randomized complete block design. The treatment design consists of 10 replications of a factorial of species (giant ragweed or common sunflower) by plant community (same species grown previously in the pot (SAME) versus another species grown previously in the pot (OTHER)). The experiment will be performed twice. Coordination of research efforts will be accomplished as described for Objective 1a. In the autumn, sufficient field soil will be collected to fill 80 pots, 30 cm in diameter and 30 cm deep. 40 pots will be used for the first run of the experiment and 40 for the second run. Giant ragweed will be planted in 20 pots and common sunflower planted in the remaining pots and transferred to a greenhouse. Giant ragweed seed will need to be preconditioned or vernalized by storing seeds in 4 °C moistened sand. Each species will be grown for 10 week period and removed from the soil at the end of this period (phase I). This procedure will be repeated using the exact same pots (phase II). During this time, soil for the second run of the experiment will be stored at 5 C. At the end of phase 2, ten of the pots previously planted to giant ragweed will be planted to giant ragweed and the other 10 will be planted to common sunflower (phase 3). These plants will grow for 10 weeks, after which they will be harvested and biomass recorded. A similar procedure will be performed for common sunflower with 10 of the pots previously planted to common sunflower to be planted to common sunflower, and the other 10 to be planted to common sunflower (Fig. 2). At the end of the first run, the second run will be started. For each experimental unit, approximately 27 cm3 of soil will be randomly sampled both before and after the plant growth assay. These samples will be immediately stored in a freezer at -20 °C. If the results of growth assays demonstrate that the treatments had an impact on the plant biomass accumulation, then samples will be processed to examine the composition and diversity of the microbial community as described in Klironomos (2002). The biomass measurements for the SAME and OTHER treatments for each species i in phase 3 will be used to calculate plant feedback (F) scores according to equation 1: Fi = biomassi (SAME) biomassi (OTHER) {1} Analysis of variance models containing terms for Rep, Species and Site will be used to detect differences in F for common sunflower and giant ragweed within and between experimental locations. The relationship of F to demographic rates and population growth rates of the two species within and between locations will be explored with Pearson correlations with Bonferroni-corrected P-values. We hypothesize that F is helping to regulate weed population dynamics in the North Central Region. Therefore, we expect that F will be positive and large at locations with high population growth rates and smaller (and possibly negative) at locations with lower population growth rates. 1C) Partition nutrient and water stress in giant ragweed and common sunflower plants alone and in combination with crops. Giant ragweed and common sunflower will be grown at participating locations across the NC region. Plot size, planting density, common seed source, cultural practices, and number of replications will be the same as described in objective 1a. An addition N treatment will be imposed so that there is a low N treatment (no or limited N applied, as with a starter fertilizer 15 to 30 lb/A) and a non-limiting N treatment (20% above rate used for high yielding corn in the area). In addition to the above-mentioned demographic data, other environmental data will need to be collected at each site. Soil samples to a depth of 120 cm will be collected in 15 cm increments twice each year (at planting and at harvest) following the protocols described by Clay et al. (1997). Gravimetric soil water, Olsen-P, soil test K, and nitrate and ammonium will be determined from these samples using standard methods. Rainfall and growing degree day data will also be obtained at each site. At physiological maturity, seeds will be harvested from the plants, yield determined by weight and number, and average seed size calculated. A 10 to 15 g sample from each treatment will be sent to South Dakota and used for isotope mass spectrometery analysis to determine total C, total N, 15N, and carbon discrimination. Crop samples from areas surrounding the experimental site will also be requested to provide a reference dataset. Coordination of location efforts will be led by Dr. Sharon Clay, ND, and will be accomplished as described in Objective 1a. Objective 2. Incorporate weed demographic information into a WeedSOFT module that quantitatively accounts for the economic losses of weed escapes and explore the significance of escapes with a risk-based approach. We propose to accomplish this objective by: (a) obtaining the source code for the ADVISOR module of WeedSOFT. This step has been completed, including the drafting of appropriate legal access to the source code by the University of Wisconsin Madison on behalf of Dr Luschei and University of Nebraska Lincoln. (b) Recode the model into Mathematica, an object-oriented symbolic mathematics package for analysis and prototyping the new module. (c) Arrange for a large set of initial scenarios to run for a number of years, where each weed management decision is followed over time and the annualized net returns calculated. Each scenario necessarily contains an assumption about the demographic response of the weed population(s) to the selected management. WeedSOFT calculates seedling mortality from a large lookup table of products and weeds, but we will utilize reasonable assumptions, eventually from information created in objective 1, for the other components of the life-cycle. (d) Search over all combinations of management scenarios and rank them on annualized net return. (e) Compare the individual one year rankings with the multiyear ranking and calculate the difference in net return between the single and multiyear optimizations. We will examine the value of demographic information by running the scenarios with different demographic assumptions and supposing that one is correct. Any sub-optimality caused by the incorrect information represents the value of the information. This technique is referred to as the value of perfect information in the decision theory literature (Raiffa and Schlaifer 2000). The activity of investigating the value of demographic information will help us understand how much attention needs to be paid to the precision of our forecasts. We will also develop a stochastic dynamic weed population model using weed demographic information collected as part of Objective 1 and from previous NC-202 protocols. The model will be implemented as a WeedSOFT module, building on the existing static deterministic model. The approach will build upon the framework used by Pannell (1994) where a deterministic model for weed control in wheat was extended to include stochastic elements, and Swinton and King (1994) where a deterministic model for weed control in corn and soybean cropping systems was extended to include stochastic and dynamic elements. Like the existing WeedSOFT model, stochastic and dynamic elements will be implemented as a simulation module that identifies the potential impacts of management alternatives, and not as an optimization model that identifies an overall best strategy. The general stochastic framework includes variability in weed density, weed control efficacy, weed damage parameters, and weed free yields. Distributions of stochastic variables can be affected by management and environment. Following the framework of Swinton and King (1994), the distributions of stochastic variables will be estimated through both mechanistic linkages to underlying variables (e.g. linking herbicide efficacy to random weather events), and direct empirical estimation of unexplained random variation. Weed population dynamics will be included by modeling weed escapes and their potential effect on weed seedbanks and future weed densities. This is already implemented in WeedSOFT in a qualitative fashion, and will be extended to include stochastic quantitative estimates. These quantitative estimates will be used in conjunction with management information to estimate economic effects. The module will be used to evaluate economic risks associated with common weed management practices under varying environments.Measurement of Progress and Results
Outputs
- Obtain seedbank persistence/recruitment and seedling survival/fecundity information necessary to analyze and compare demographic rates within and between annual weed species at different times and locations.
- Determine if soil feedback mechanisms exist and if soil feedback is contributing to variation in weed species demography.
- Determine the impact of nitrogen and water on variation in weed species demography.
- Incorporate weed demography information into a bioeconomic decision support system that can be used by producers and other agricultural professionals to evaluate economic risk of alternative weed management strategies.
Outcomes or Projected Impacts
- Develop a probabilistic understanding of weed populations in response to spatial, temporal and management variation. This will allow scientists to design integrated weed management strategies that address local and regional issues (economic, ecological, and social) while reducing our reliance on herbicides. We will also be better able to address more long-term issues such as weed invasiveness and biological control.
- Increased use of bioeconomic weed management decision support systems among farmers and other agricultural professionals. This will ultimately reduce economic risk and provide a framework that enhances learning and knowledge building between various user groups.
Milestones
(2006): Quantitative assessment of common sunflower and giant ragweed demography.(2007): Quantify and partition nitrogen and water stress and their effects on common sunflower and giant ragweed growth and fecundity.
(2009): Quantify and partition crop stress that occurs when grown with common sunflower and giant ragweed at different densities across the Midwest
(2009): Weed demography information incorporated into bioeconomic management model.
Projected Participation
View Appendix E: ParticipationOutreach Plan
We have been, and will continue to be, very visible to a wide range of scientific and end-user groups in the North Central Region and across the country. Our group sponsored a symposium at the Weed Science Society of America meeting and has actively presented results of our research to various user groups through the hard work of individuals within each state. Data have been presented at North Central Weed Science Society annual meetings each year by individual state representatives, some presenting state-specific data, other presenting a regional summary of data. We will also continue to actively write manuscripts for peer reviewed publications.
Our primary goal is to support the development of applied weed management decision support systems through innovative regional research that addresses long-term decision making. Members of NC202 have met with the WeedSOFT group on numerous occasions to discuss opportunities and plans for integrating information. As of 2004, 620 new, updated, or complementary copies of WeedSOFT were in the hands of crop consultants, agronomists, county agents, and producers across seven states in the Midwest. We expect this number to increase as we move forward to expand our research base as well as begin new initiatives that address issues important to growers.
Most notably, NC 202 has provided the framework for cooperative research among weed scientists, economists, and modelers. This type of multi-disciplinary interaction is critical for addressing complex questions such as the objectives of NC 202. This interaction has stimulated numerous research ideas and has broadened many of the scientists' views of their individual research efforts. This type of interaction would not have occurred without the support of NC 202.
The success of NC202 serves as a model for regional research efforts. The participating scientists developed research protocols that were conducted in multiple environments, leading to a database much more powerful than would have been possible working independently. The result is the generation of valuable biological and environmental data in a very efficient manner.
Organization/Governance
The project will be planned and executed by a Technical Committee composed of one representative from each state. However, each experiment station and federal agency may have more than one representative on the committee where the scope of the project involves more than one subject matter discipline. The administrative advisor and CSRS-USDA representatives are non-voting but retain veto power.
Members of the technical committee of this project will meet annually. In addition to addressing routine business matters, the general purpose and motivating exercise will be to critique the adequacy and effectiveness of established research procedures, to discuss and debate the meaning and interpretation of data, suggest avenues to follow for best objective achievement, and to discuss the regional implication that the research will have. This process is viewed as critical and contributory to the regional research concept.
The officers shall consist of a chairperson, vice chairperson, and secretary. The vice chairperson and secretary will be elected annually, and the vice chairperson shall become chairperson, in consultation with the administrative advisor. The chairperson presides over the Technical Committee and Executive Committee and is responsible for preparing or supervising the preparation of the annual report of the regional project. The Chairperson is also responsible for site selection and coordination of the annual meeting and other meetings held at regional and national discipline meetings. The secretary records and distributes the minutes and performs other duties assigned by the Technical Committee.
Subcommittees will also be organized around each objective. A sub-committee chairperson will be appointed by the Technical Committee chair and will provide oversight responsibilities for activities related to the designated objective. The sub-committee chair will also coordinate meetings outside of the annual meeting, if necessary.
Literature Cited
Clay, D.E., R.E. Engel, D.S. Long, and Z. Liu. 2001. Using C13 discrimination to characterize N and water responses in spring wheat. Soil Sci. Soc. Am J. 65:1823-1828.
Clay, D.E., S.A. Clay, D. Lyon, and J. Blumenthal. 2005. 13C discrimination in corn grain can be used to separate and quantify yield losses due to water and nitrogen stress. Weed Sci. (in press).
Davis, A.S., P.M. Dixon and M. Liebman. 2003. Cropping system effects on giant foxtail demography: II. Retrospective perturbation analysis. Weed Sci. 51:930-939.
Davis, A. S., P. M. Dixon and M. Liebman. 2004. Using matrix models to determine cropping system effects on annual weed demography. Ecol. Appl. 14: 655-668.
Dieleman, J.A., D.A. Mortensen, D.D. Buhler, C.A. Cambardella, and T.B. Moorman. 2000. Identifying association among site properties and weed species abundance. I. Multivariate analysis. Weed Sci. 48: 567-575.
Gonzalez-Andujar, J. L. and C. Fernandez-Quintanilla. 1991. Modelling the population dynamics of Avena sterilis under dry-land cereal cropping systems. J. Appl. Ecol. 28: 16-27.
Jordan, N. 1993. Simulation analysis of weed population dynamics in ridge-tilled fields. Weed Sci. 41: 468-474.
Kegode, G. O. and R. B. Pearce. 1998. Influence of environment during maternal plant growth on dormancy of shattercane (Sorghum bicolor) and giant foxtail (Setaria faberi) seed. Weed Sci.
Klironomos, J.N. 2002. Feedback with soil biota contributes to plant rarity and invasiveness in plant communities. Nature 417:67-70.
Neeser, C., J.A. Dille, G. Krishnan, D.A. Mortensen, J.T. Rawlinson, A.R. Martin and L.B. Bills. 2004. WeedSOFT®: a weed management decision support system. Weed Science 52:115-122.
Pannell, D.J. 1994. The value of information in herbicide decision making for weed control in Australian wheat crops. Journal of Agricultural and Resource Economics 19(2):366-381.
Raiffa, H. and R. Schlaifer. 2000. Applied Statistical Decision Theory. John Wiley and Sons, New York, NY. 356pp.
Rao, P. 1997. Variance components estimation: mixed models, methodologies, and applications. New York, NY: Chapman and Hall/CRC. 204 pp.
Smeltekop, H., D.E. Clay, and S.A. Clay 2002. The impact of intercropping annual Sava snail medic on corn production. Agron. J. 94:917-924.
Swinton, S.M., and R.P. King. 1994. The value of pest information in a dynamic setting: The case of weed control. American Journal of Agricultural Economics 76(1):36-46.
Zelaya, I.A. and M.D.K. Owen. 2004. Evolved resistance to acetolactate synthase-inhibiting herbicides in common sunflower, giant ragweed, and shattercane in Iowa. Weed Sci. 52:538-548.