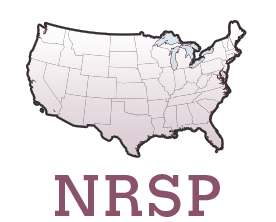
NRSP_Temp13: Artificial Intelligence for Agricultural Autonomy
(National Research Support Project Summary)
Status: Submitted As Final
NRSP_Temp13: Artificial Intelligence for Agricultural Autonomy
Duration: 10/01/2025 to 09/30/2030
Administrative Advisor(s):
NIFA Reps:
Non-Technical Summary
U.S. agriculture needs autonomous systems1 (a.k.a., agricultural autonomy, or AA) to mitigate the growing lack of labor2, to enhance safety and efficiency in farming, and to optimize crop management at a very precise level. Solutions to simple AA problems are emerging3,4, like GPS and inertial autonomous guidance, but only for elementary tasks like tillage on large, open, flat fields. In complex agricultural environments, great difficulty exists in autonomous perception for various tasks like detection, characterization, and avoidance of obstacles, including negative obstacles5 like holes and ditches. AA requires sensors to provide situational data, artificial intelligence (AI) to analyze the data and make decisions, and electromechanical systems to translate the decisions into physical action like navigation or precise movement of robotic arms and end-effectors (e.g., grippers). Whereas autonomous mobile robots have become commonplace for moving boxes and pallets in distribution warehouses, where environments are controlled and relatively simple to navigate, the complexity associated with numerous crops, cropping systems, field shapes and sizes, topography, etc., makes developing highly sophisticated AA extremely challenging. Our goal is to bridge existing gaps in AI that are slowing the development and adoption of AA. The objectives focus on building and expanding large, curated, open-source datasets for complex agricultural operations, building collaboration with computer scientists, and expanding information sharing regarding AI for AA. The target audience for this research includes public and private researchers working to develop AA. The activities to be undertaken will make benchmark data available to researchers nationwide and span a wide range of AA applications, enable development of AI algorithms and architectures specifically developed for AA, and accelerate advancement in AA through collaboration and sharing of data.
Statement of Issues and Justification
A key difficulty in bringing full autonomy to agriculture is the high level of complexity associated with numerous crops, cropping systems, field shapes and sizes, topography, etc. In many respects, this situation can be seen as similar to the difficulty autonomous car companies (Waymo, Cruise, etc.) have had in bringing their cars to market; originally expected to be widely marketed by 2020, the limited number currently on the road work well only in simple, open-road, clear-sky conditions, but they fail in highly complex and unusual roadway scenarios6 for which there is inadequate training data or when their sensors are hampered by weather conditions. It is clear that inadequate early investment was made to solve these challenging “edge-case” conditions in the few years that was anticipated. There remain countless fundamental agricultural tasks, particularly in specialty crops7,8,9,10,11,12, that need automation and full autonomy, but major gaps exist between the current state of autonomous systems and eventual autonomous solutions. Investment in bridging the key gaps will minimize the time required to produce practical on-farm solutions. In this project, we will focus on two key technology gaps, the bridging of which will allow for rapid development of real-time autonomous decision-making that enables precise navigation, harvesting, input applications, and collection of critical data such as yield estimates.
First, there are inadequate large open-source datasets representing the needed level of variability to train the AI algorithms and models that will drive the analytical aspect of robust and reliable autonomous solutions. We believe this situation can be rectified only through a National Research Support Project (NRSP) in which researchers collaborate (a) to build large well-curated datasets that span a wide range of applications and for which commercial providers do not have the financial incentive to approach, (b) to collect data with a high level of ground-truth validation, and (c) to determine standards for data collection and sharing so that researchers and corporations across the country can exploit the data to develop new autonomous solutions. As part of this work, a few example AI models will be trained with the broader datasets created, providing a baseline for other researchers and tech companies to further advance the technology.
Second, most AA researchers use AI algorithms and data inputs that are generic and largely application-independent; e.g., the YOLO (You Only Look Once)13 family of algorithms. We believe an NRSP is needed to formalize a national effort in which agricultural researchers work with computer scientists to develop AI algorithms and architectures fit for the purpose of AA. Three key areas of focus will be (a) AI architectures that account for the fusion of heterogeneous (a.k.a. multimodal) data14 including color images, videos, multispectral and hyperspectral images, 3D point clouds, attribute tables, temporal sequences, etc., on different geospatial and time scales (e.g., historical satellite and drone data, soil data, weather data, and yield maps, fused with real-time vision data), (b) feature engineering15 in which the data inputs for a specific application are analyzed for information content to maximize algorithm efficiency, and (c) data that are practically available to a grower or agronomic consultant without tremendous additional effort or expense.
We expect this NRSP to facilitate a broad array of research activities in AA to include various types of sensing, analytics, actuation, integrated systems, and related technologies.
Prerequisite Criteria
How is the NRSP consistent with the mission?
This NRSP focuses on the development of enabling technologies through support activities including standardized collection, assembly, storage and distribution of vast amounts of data for the development of AI in support of high-priority autonomous agricultural systems. The data includes images, video, Lidar and other types of data that facilitate development and training of AI for autonomous systems. This work is not primarily research but facilitates a broad array of research activities including AI model development and training.
How does the NRSP pertain to a national issue?
The need for autonomous systems in agriculture is a nationwide problem, and while there are great variations in topography, climate, crops, cropping systems, etc., many issues associated with AI for image-based perception and decision making in AA are common to agricultural production nationwide, such as the prevalence of negative obstacles, challenges posed by unknown obstacle rigidity (soft vs. hard), variations in lighting and weather, occlusion of fruit behind foliage, etc. The development of large open-source databases, along with the creation of data-collection standards, as well as AI algorithms and models specific to agricultural machine vision, will benefit researchers and technology companies in this field across the country.
The technological gaps to be addressed, which must be bridged to enable AA to have more than a cursory effect on agricultural production, are specific in nature and require an NRSP to advance the state of the art. If these gaps are not bridged, AA research will continue to see simply incremental progress in fundamental research, with few real-world improvements needed to address pressing challenges currently faced by farmers (e.g., high-labor use and production costs) and to maintain food security.
Chief among the goals of this project is creating data and databases that other AA researchers will readily use. Thus, leaders of this project intend to liaise with leaders of NRSP 10 (National Database Resources for Crop Genomics, Genetics and Breeding Research) concerning development of databases and standardized data collection that are findable, accessible, interoperable, and reusable (FAIR). Furthermore, leaders of this project will liaise with leaders of NRSP 11 (Building Collaborative Research Networks to Advance the Science of Soil Fertility: Fertilizer Recommendation Support Tool) concerning efforts to develop a community of practice to galvanize interest and participation around the subject of interest here, AA. Finally, this project will include the best minds and resources within the State Agricultural Experiment Stations, many of whom will be members of the new multistate research project, S1098 (Autonomy for Agricultural Production, Processing, and Research to Advance Food Security through Sustainable and Climate-Smart Methods).
Rationale
Priority Established by ESCOP/ESS
This proposal supports Grand Challenges 1 and 6 identified by the ESCOP Science and Technology Committee (STC) Science Roadmap for Food and Agriculture (2020) as stated below.
Grand Challenge 1: We must enhance the sustainability, competitiveness, and profitability of U.S. food and agricultural Systems.
Grand Challenge 6: We must heighten environmental stewardship through the development of sustainable management practices.
Our project enhances sustainability and heightens environmental stewardship by enabling the optimization of production inputs at the individual-plant or highly localized level under the constraint of minimum environmental risk. It enhances competitiveness and profitability by solving labor problems and enabling the precise application of production inputs while maximizing production, which must be balanced against environmental risk.
Relevance to Stakeholders
The leadership team of this NRSP project has been and will continue to be engaged with a large number of researchers and tech company members through various events including the “Technology Adoption Workshop” (organized by Co-PI Karkee and held on July 25, 2024 in Richland, WA) and the “AI in Agriculture Conference” series (organized by a group of members of the S1090 multistate project, AI in Agroecosystems: Big Data and Smart Technology-Driven Sustainable Production, including PI Thomasson and Co-PI Ampatzidis). These interactions have repeatedly indicated that academic and industry researchers working on autonomy solutions for agriculture have been hindered by the lack of standardized expansive datasets and optimized AI models for agricultural challenges. This project, with the objectives discussed above, will be highly relevant to the primary stakeholders – AA researchers at the state, federal, and corporate levels – enabling them to accelerate the advancement and adoption of AA solutions. At a minimum, this group is over 50 researchers across the nation who signed up to participate in the new multistate research project, S1098, and that number does not include federal and corporate researchers who will also have interest. This stakeholder group is considered primary because they will be the principal users of the open-source datasets and artificial intelligence (AI) algorithms produced in the project. These products will be generated as a collaboration among researchers at land grant universities who will be the main developers of the datasets, data collection standards, and AI algorithms to be used by this stakeholder group. Leaders of this NRSP will be purposeful in liaising with S1098 to garner their feedback on project activities and management plans.
Secondary stakeholders include farmers, agricultural workers, equipment manufacturers, technology companies, policy makers, government agencies, and consumers. This NRSP will create an advisory board that includes one row-crop farmer, one specialty crop farmer, one member from both a major machinery manufacturer and a small startup company engaged in AA product development, one policy-oriented member, and one member of S1098 who is not on the leadership team of this NRSP. This advisory board will meet with the leadership team of this NRSP twice annually to review progress of the project and to provide advice on future direction.
Furthermore, investigators will work closely with early adopter farmers to identify real-world problems that could benefit from an agricultural-autonomy solution, enabling the investigators to more carefully select on-farm scenarios for data collection. The investigators will also work with these growers to field test emerging technologies. Through these interactions, this group of secondary stakeholders will benefit from heightened awareness and understanding of these emerging technologies, and they will aid in the dissemination of the research results to the broader farming community. Each university receiving funds from this NRSP will identify at least two such farmers to work with.
The leadership team will continue to organize meetings and workshops over the project duration to share project outputs and demonstrate the tools developed in this work with researchers, tech company members, and farmers. These events will facilitate the utilization of project outputs and provide the team additional opportunities to learn from stakeholders to continuously improve project relevance based on evolving stakeholder needs. The team will also conduct before-and-after surveys at these events to quantitatively assess how widely project outputs are being and will be used by stakeholders. The advancement of autonomy solutions as well as increased interest from farmers to adopt these technologies will inform policy makers, who will also be a part of our outreach engagement during the project, to discuss and formulate policies that are practical and conducive to enabling broader adoption of autonomy solutions on future farms.
Implementation
Objectives
-
1. To develop large, comprehensive, open-source datasets that make benchmark data available to researchers nationwide and span a wide range of agricultural-autonomy applications.
Comments: In AA, cameras and/or various other sensors are used to generate data on plant canopies and surroundings, which are then processed with AI models to perceive and understand the environment and to make operational decisions. Then, ground (or potentially aerial) vehicles, and actuators such as robotic arms and end-effectors (or “hands”), are used to effectuate/implement the operational decisions including navigation through agricultural fields (including orchards) and perform specific field operations such as autonomous harvesting. A great deal of data collection has gone into building image databases that use AI for autonomous detection of individual weeds, but not as much has been done on other crop-plant stressors like diseases and insects, and very little has been done in collecting other types of data that inform AA, like images for pruning, flower thinning, crop identification, and images of trees, fruit, foliage, and crop rows at different growth stages. There is a need to build larger, nationwide, open databases from a variety of sensors, such as RGB, multiIn AA, cameras and/or various other sensors are used to generate data on plant canopies and surroundings, which are then processed with AI models to perceive and understand the environment and to make operational decisions. Then, ground (or potentially aerial) vehicles, and actuators such as robotic arms and end-effectors (or “hands”), are used to effectuate/implement the operational decisions including navigation through agricultural fields (including orchards) and perform specific field operations such as autonomous harvesting. A great deal of data collection has gone into building image databases that use AI for autonomous detection of individual weeds, but not as much has been done on other crop-plant stressors like diseases and insects, and very little has been done in collecting other types of data that inform AA, like images for pruning, flower thinning, crop identification, and images of trees, fruit, foliage, and crop rows at different growth stages. There is a need to build larger, nationwide, open databases from a variety of sensors, such as RGB, multispectral, thermal, RGB-D, and hyperspectral cameras, as well as 3D Lidar, etc. The implementation of this objective is divided into three tasks, with specific deliverables and success criteria given in the attached Table 1. Task 1.1: Crop Selection and Field Data Collection In building these databases, we will focus first on higher-value crops including tree and bush fruits, vegetables, nuts, and some row-crop fruits like cotton bolls. Farmers of higher-value crops will likely be the first to adopt autonomous technologies, not only because of their greater economic flexibility, but also because they tend to have higher labor needs. Over the long term, however, we expect to broaden the research to additional row crops and forages. We will consider the robotics issues that are common among the various crops, such as identification and classification of the fruit (particularly in circumstances of occlusion), the effects of stressors including weeds, diseases, insects, and abiotic stressors, as well as complexity of the farming environment for robust, robot navigation. Specifically, we will select several high-value crops from across the U.S. based on economic value. Some target crops include cotton, apples, citrus, and strawberries. Image/video data will be acquired for crop-plant stressors like diseases and insects, weeds, plant canopy at different growth stages, and branches and trunks during dormant season. For example, images and videos in apple orchards will be collected during key phenological/operational stages such as early flowering, full bloom, fruit set, early fruit growth, harvest and dormant seasons. In addition, images and videos will be collected from a wide range crop rows, headlands and inter-field paths/spaces from the selected crops. We will also consider various illumination conditions, image resolutions, and image backgrounds, such as bare soil and stubble. Task 1.2: Enhancing Data Variability, Quality and Access The data-collection framework will include a variety of sensors to capture data under various conditions. Field collected images will be manually annotated for various objects or regions of interest such as flower, fruit, foliage, branches, trunks, ground, grass, sky, dirt, and paths, among others. In addition, we will consider adding synthetically generated data and improving data quality and resolution using generative AI models. Our past research16 has shown promise for generating realistic apple orchard images using DALL.E. This type of technique will be expanded to generate synthetic datasets for all the crops involved with both the text-to-image and image-to-image capabilities of Generative AI models. Additionally, our recent work17,18 on the closed-loop real2sim paradigm has demonstrated the significance of generating synthetic datasets based on real-world sensor observations and knowledge constraints for data scarcity issues, especially 3D robotic perception. These activities are expected to broaden the variability and reliability of the dataset, helping generalize the AI models trained with these datasets. A wide range of ground truth data will be collected to ensure high levels of data validation. This data generation framework will support a wide range of agricultural tasks and create large, open-source datasets accessible to researchers nationwide. Such access will facilitate collaboration among researchers, which is essential to further expand these datasets and utilize them to train novel AI models and develop autonomous machines. We acknowledge that other open-source data sets are already available. For example, PlantVillage has a significant amount of plant-disease related data from various parts of the world. Such data on stress conditions are important because early pest, disease, nutrient, and water deficiency detection are important for autonomous decision-making to limit the spread and severity of occurrences and minimize crop damage. On the other hand, we also realize that much more data is needed for all types of stressors and also for many more crops, conditions, and sensors. The open-source datasets developed under this NRSP will include image data and other types of autonomous vehicle perception data such as Lidar, and they will include ground-truth data. These new data will be an exponential expansion of the open-source data currently available. It is also worth noting that the protocols for data collection under this NRSP are likely to be different than those used to produce current open-source databases, so while some currently available data may be useful for training new broadly applicable AI models, the current data are small compared to the overall need. Furthermore, the standardized data collected during the 5 years of this NRSP will be adequate for benchmarking and reliable AI solutions for specific AA applications, but new applications requiring additional data will continue to arise in the future. Our expectation is that this NRSP will enable multiple important AI-driven solutions and set the stage for increasingly rapid data collection and AI-driven solution development in the future. Task 1.3: Data collection and sharing standards This project addresses standards critical to agricultural data collection, management, and sharing. We note the new ISO Strategic Advisory Group on Smart Farming, which is a standardization effort that touches on optimization of farming operations and is thus in line with this NRSP. Standards efforts will focus on aspects of data collection (including sensor calibration and metadata documentation) that ensure the collection of data for perception in autonomous agricultural systems adheres to the FAIR (Findability, Accessibility, Interoperability, and Reuse) Guiding Principles for scientific data management and stewardship. Standards should define standard data formats and implement quality control measures to ensure data reliability. Standards development will be conducted in conjunction with ASABE (American Society of Agricultural and Biological Engineers) and/or other standards development bodies such as ANSI, ISO, and NIST. Our overarching strategy involves leveraging ASABE as a platform to collaborate with other standards organizations, including ISO, ANSI, and NIST. The project leadership team includes long-time ASABE members with significant roles in various technical committees within the Machine Systems (MS) and Information Technology, Sensors, and Control Systems (ITSC) communities. Their extensive experience with ASABE processes enables them to initiate standards, coordinate in-society and cross-society communications for co-development, gather feedback, revise standards, and oversee final submissions and ongoing maintenance of published standards. Additionally, the team includes key contributors such as Co-PI Jiang who serves as a representative to the National Institute of Standards and Technology's (NIST’s) U.S. AI Safety Institute. Roles like this provide a direct liaison between the project team, ASABE committees, and other standards organizations. Together, these collaborations aim to collectively develop and maintain standards for agricultural data collection and curation, thereby advancing the development and adoption of AI-driven solutions in AA. Standards development and maintenance will be a continuous activity, because numerous standards are needed and standards, once developed, must be maintained and improved over time. In addition to collaborations with ASABE and other standards organizations, we will involve key non-profit organizations such as AgGateway, which develops resources and standards to help companies access information and solve digital challenges in agriculture and related industries. This will allow proactive engagement with diverse stakeholders from both academia and industry in the standards-development process to ensure standards are meaningful and implementable for future adoption. We will also work with leaders of the S1090 multistate project to facilitate a workshop on developing standards for data collection and sharing at the annual AI in Agriculture Conference. -
2. To develop AI algorithms and architectures that account for the fusion of heterogeneous (a.k.a. multimodal) data on different geospatial and temporal scales, which take advantage of feature engineering to maximize algorithm efficiency and data that are practically available to growers and agronomic consultants.
Comments: As mentioned previously, AI is used to analyze available data for making decisions (e.g., which fruit to pick, when to stop if there is an obstacle, etc.) and implementing those decisions (e.g., moving optimally to grab and pick fruit, navigating around an obstacle, etc.) in autonomous agricultural systems. It is important in this work to build a formal collaboration with a group of interested computer scientists to develop efficient AI algorithms and architectures that fit the practically available data types. The implementation of this objective is divided into three tasks, with specific deliverables and success criteria given in the attached Table 2. Task 2.1: Models for autonomous navigation in agricultural fields: A great deal of research has gone into autonomous driving systems and autonomous carts developed to minimize load-carrying by workers. The current major gap associated with autonomous navigation technology involves algorithms for differentiating obstacle types (e.g., soft weeds that can be driven over from hard rocks or trees that cannot), detecting negative obstacles (e.g., ditches), and negotiating common types of turns such as at headlands. While the basic capability for autonomous driving has been largely completed, obstacle detection and avoidance, and driving for more complicated tasks like complex navigation, leader-follower scenarios, and collection, sorting, and stacking of harvested products such as bales and apple bins, still require significant research and development of robust algorithms. We will consider object detection and avoidance in complex environments and challenging driving scenarios like leader-follower, bale collection, and fruit/vegetable picking. Specifically, algorithms involving deep learning will be developed to integrate multi-modal sensing in order to utilize various types of data including from LiDAR scanners, hyperspectral imaging systems, and color cameras to recreate 3D environments and determine safe and efficient paths for autonomous vehicles to follow in agricultural fields like apple orchards and strawberry fields. Spatial as well as temporal information (e., g., overlaying of dormant and growing season information) will also be utilized to determine soft vs hard obstacles and detect negative obstacles. Task 2.2: Models for automating labor-intensive agricultural operations: In addition to vehicle navigation, numerous other tasks, particularly in specialty crops that are labor-intensive, need to be automated such as harvesting, thinning, pruning, and precision spraying, etc. Automating these tasks requires the development of appropriate perception and control algorithms. Thus, algorithms will be developed to provide robust identification of plant components like fruits, early detection and classification of stressors (e.g., pests, diseases), decision-making involving characterizing plant canopies and relating that information to nutrient and water needs, etc. These models need to provide perception information in real time or near real time to fulfill the time constraint of specific tasks. Subsequently, control models, especially learning-based controllers (e.g., via imitation learning) will be developed to take the perception information and convert it to decisions that actuators can act on accordingly. Task 2.3: Partnership with computer scientists to develop robust AI models: To successfully complete the AI modeling activities described in Tasks 2.1 and 2.2 and build a broader community of researchers in AA, we will develop a close partnership with experts from academia and industry working in AI, machine learning and robotics. Each institution involved in this proposal has strong expertise in these fields, and we will reach out to one or two experts at each institution to work with us as collaborators and co-supervisors of our students and scholars. In addition, NSF and USDA have funded five AI Institutes for agriculture over the last four years. These institutes are making progress in agriculture-inspired AI models with broader applicability. A large number of AI scientists are involved in these institutes. Co-PI Karkee is a thrust co-lead in one of the institutes led by Washington State University (AgAID.org). AI researchers working with AgAID and other AI institutes, who are already aware of the agricultural environment, will be invited to build collaborative partnerships with our team so that we can learn from their ongoing efforts and then advance AI models to address the unique challenges in AA. Our team has also been working technology companies like John Deere, CNH, Hylio AgroDrones, Microsoft, IBM, etc. Thus, we intend to partner with AI developers there as well.
Projected Outcomes
- By making benchmark data widely available, the project will enable the replication and validation of research findings across various agricultural contexts, ultimately leading to more robust and reliable AI-driven solutions. Comments: The proposed project is expected to yield significant outcomes and impacts that will advance AA. First, the development of large, comprehensive, open-source datasets will be a game-changer for researchers nationwide.
- The establishment of standardized data-collection methods will further ensure consistency and accuracy in the research process, facilitating the comparison of results across different studies and regions. Comments: This standardization will also promote the adoption of best practices among researchers and practitioners, leading to common protocols that can be applied broadly in AA.
- The goal is to create a team of computer scientists and AI experts who will commit a significant portion of their time to working in AA pursuits, specifically focusing on developing AI algorithms tailored to AA applications. Comments: Leaders of and participants in this NRSP will identify a cadre of computer scientists with an interest in AA without directly allocating funds from this NRSP; rather, we will seek externally funded collaborations and recruit them to membership in S1090 and/or S1098. Some of this work has already begun; e.g., Dr. Shahram Rahimi, formerly Department Head of Computer Science and Engineering at Mississippi State University (now at University of Alabama), has been collaborating with Dr. Thomasson on AA for over 4 years. Recruitment of these AI specialists will involve inclusion in funded research proposals, which should lead to long-term collaborations. This collaboration with computer scientists is expected to generate new, innovative AI technologies that will directly impact agriculture. By focusing on the fusion of heterogeneous (a.k.a. multimodal) data (e.g., images, videos, point data, tables) across different geospatial and temporal scales, and by maximizing algorithm efficiency through advanced feature engineering, the project will produce AI systems that are both powerful and practical for growers and agronomic consultants. These advancements will not only enhance the precision and effectiveness of autonomous systems but also make them more accessible to a wider range of users, from small-scale farmers to large agricultural enterprises.
- Another key outcome of the project will be the expansion of nationwide information sharing among U.S. researchers. Comments: By fostering a collaborative environment, the project will facilitate the exchange of ideas, data, and methodologies, leading to more coordinated research efforts in AI-enabled AA. This increased communication will help identify critical research and education issues that need to be addressed and accelerate innovation in the field. The project will also inspire the organization of symposia, workshops, and other events that bring together experts from different disciplines to discuss the latest developments and challenges in AA.
- The publication of joint extension bulletins, research articles, and review articles will further disseminate the knowledge generated by this project, contributing to the global body of research in agricultural AI. Comments: These publications will not only highlight the project's findings but also set the stage for future research initiatives by identifying gaps in the current understanding and proposing new directions for exploration.
- By addressing the critical need for labor-saving technologies, improving the accuracy and reliability of AI systems, and fostering a collaborative research community, the project will contribute to the long-term sustainability (economic, environmental and social) and competitiveness of U.S. agriculture. Comments: Ultimately, the expected impacts of this project will be far-reaching. This NRSP will also serve as a model for how multidisciplinary research and nationwide coordination can drive innovation and solve some of the agricultural sector's most pressing challenges. As mentioned previously, the impact of the work in terms of stakeholder use of (or willingness to use) the outputs of this project will be assessed with surveys of researchers and farmers who attend our meetings and technology demonstrations.
Management, Budget and Business Plan
Six land-grant universities (LGUs) spanning all four regions of the State Agricultural Experiment Stations (SAES) will lead this NRSP: Mississippi State University (MSU) and University of Florida in the Southern Region, University of California-Davis and Washington State University in the Western Region, Cornell University in the Northeast Region, and Kansas State University in the North Central Region. MSU will serve as the lead institution in terms of receiving funding centrally and will subcontract to the other five LGUs. Individual Co-PIs from each of these LGUs will constitute the leadership team, which will meet monthly to establish priorities and plans and to review project status and progress. Each of these LGUs will reach out to other LGUs in their respective regions, including 1890 and 1994 LGUs, and coordinate activities that support this NRSP. The participants in the S1098 multistate project will serve as the primary, but not only, source of additional participants in this NRSP. Additional participants from the federal and corporate research communities will be sought out as appropriate.
This leadership team is already active individually in seeking funds for AA research and has had significant success. For example, Dr. Thomasson has garnered $1.5M from Mississippi’s Hearin Foundation, a private foundation to support economic development in the state, to establish the Agricultural Autonomy Institute at MSU. This is an ongoing initiative – currently in year 2 of a three-year funding cycle – that supports the overall development of MSU’s AA research program. This NRSP will provide an opportunity to leverage those funds specifically for the development of critical databases and AI algorithms to support AA research. Furthermore, this leadership recently worked together to build a pre-proposal to the National Science Foundation for an Engineering Research Center called AgTUATOR. That pre-proposal was not selected for full proposal submission, but it will be improved and submitted to future funding opportunities. Such proposals have the potential to provide large funding streams to focus on AA research. Again, this NRSP will provide a framework for leveraging those funds for a large-scale nationwide effort. Moreover, the leadership team has successfully collaborated in the past and have ongoing collaborations in research, educational, and professional activities that provide the foundation for and ensure seamless and effective collaboration in the proposed NRSP. For example, Drs. Karkee and Vougioukas recently co-authored two published review articles on harvesting and canopy management in tree fruit crops.
As previously mentioned, this NRSP will have an advisory board that will include a row-crop farmer, a specialty crop farmer, a member from both a major machinery manufacturer and a small startup company engaged in AA product development, a policy-oriented member, and a member of S1098 who is not on the leadership team of this NRSP. This structure will serve as a step towards bringing in research organizations and industry to address issues and advise the leadership team regarding additional funding. Furthermore, the leadership team has been and will continue to be engaged in organizing and attending conferences and workshops that either focus on or touch on AA, such as the AI in Agriculture conference series sponsored by the S1090 multistate research group. This type of activity brings together key players from academia, industry, and federal agencies to gain and share knowledge about the work supported by this NRSP.
The regional experiment stations have been and continue to be supportive of the overall AA research effort. For example, the Mississippi Agricultural and Forestry Experiment Station (MAFES) has provided offices, lab space, and an experimental farm field that are dedicated for use by MSU’s Agricultural Autonomy Institute. MAFES has also supplied seed funding for the research of individual investigators focused on AA such as Drs. Xin Zhang and Hussein Gharakhani. Finally, MAFES and University of Florida's Institute of Food and Agricultural Sciences (IFAS) as well as Texas A&M AgriLife Research and Alabama Agricultural Experiment Station have provided sponsorship and backing funds for the AI in Agriculture conferences over the past three years.
Over the five-year funding period of this NRSP, the leadership team intends to submit proposals to USDA-NIFA, NSF, other federal and state agencies, as well as corporations like John Deere, Microsoft, IBM, and Nvidia, in order to expand the nationwide research effort. USDA-ARS often has funds for non-assistance cooperative agreements as well as scientists engaged in similar research, so efforts to work with and seek support from USDA-ARS are also foreseen.
The general organizational structure of the NRSP is shown in Figure 1. The investigators who will lead this NRSP are as follows:
- Alex Thomasson, Agricultural and Biological Engineering, Mississippi State University
- Yiannis Ampatzidis and Wonsuk (“Daniel”) Lee, Agricultural and Biological Engineering, University of Florida
- Manoj Karkee, Biological Systems Engineering, Washington State University
- Stavros Vougioukas, Biological and Agricultural Engineering, University of California-Davis
- Yu Jiang, Integrative Plant Sciences and Cornell AgriTech, Cornell University
- Terry Griffin, Agricultural Economics, Kansas State University
The NRSP will be managed as follows. We expect the project to begin in November of 2025. MSU will either hire a part-time program manager or identify a full-time program manager and delineate a portion of that person’s time for this project. The key tasks this person will take on will be focused on communication and coordination among the leaders and participants; administrative paperwork related to funding, hiring, travel, etc.; information sharing, project management regarding completion of tasks according to project goals and priorities; and timely reporting. Most of the remaining funds will be used to support the salaries and expenses (travel, conference registration, publication charges, supplies and materials) of graduate students or postdocs whose sole focus will be the work of this NRSP. Recruiting for the graduate students and postdocs will begin as soon as a funding decision has been made so that they may start in January 2026. Because of the nature of the project, some of these students/postdocs will be in computer science, but others will be in agricultural/biosystems engineering or another agricultural field like agricultural economics, noting that Dr. Terry Griffin, the Co-PI from KSU, is an agricultural economist. In the first quarter of calendar-year 2026, we will hold a kickoff meeting at one of the funded LGUs in the project. Around this time, we will initiate – or begin contributing to existing – public image databases and code libraries for weeds, diseases, insects, and other plant stressors, as well as new AI-based methods for early detection of stressors and decision-making. By way of the graduate students and postdocs, we will initiate studies on topics like the following: (1) automation of the identification and classification of pests and diseases, targeting early detection, by utilizing advanced remote and proximal sensing technologies (aerial and ground) and novel AI techniques; (2) characterization of plant canopies and correlation of that information to nutrient needs; (3) object detection and avoidance in complex environments; (4) challenging driving scenarios like leader-follower and bale collection; (5) yield forecasting; and (6) secondary fruit inspection, sorting, and stacking. Early in the project, we will begin identifying other funding sources that could be leveraged for the development of national-scope projects focused on areas of common interest in AI-enabled AA. Figure 2 is a Gantt Chart of the general schedule of the NRSP.
With respect to the budget, MSU will receive all funds as the lead institution and distribute portions to the other institutions through subcontracts, in accord with the overall budget. Individual institutional budgets include the following:
- MSU $98,000 per year
- 50% Program Manager plus fringe per year
- 1 graduate student plus tuition and fringe per year
- $5,000 travel per year
- $2,219 supplies and other per year (average)
- UCD $88,000 per year
- 1 month faculty salary plus fringe per year (Vougioukas)
- 50% postdoc plus fringe per year
- $3,000 travel per year
- $13,000 supplies and other per year (average)
- UF $158,000 per year
- 1 month faculty salary plus fringe per year (Lee)
- 1 month faculty salary plus fringe per year (Ampatzidis)
- 1 month research engineer salary plus fringe
- 2 graduate students plus tuition and fringe per year
- $4,800 travel per year (average)
- $2,286 supplies and other per year (average)
- WSU $75,000 per year
- 2% faculty salary plus fringe per year (Karkee)
- 1 graduate student plus tuition and fringe per year
- $5,000 travel per year
- $10,000 supplies and other pear year
- CU $37,000 per year
- 2% faculty salary plus fringe per year (Jiang)
- 2 summer students plus tuition and fringe per year
- $2,000 travel per year
- $5,000 supplies and other per year
- KSU $44,000 per year
- 1.5 months faculty salary plus fringe per year (Griffin)
- 1 undergraduate student plus fringe per year
- $2,000 travel per year
- $3,000 supplies and other per year
The team has other research funds that relate to AA in general, but not specifically to the objectives in this project. As mentioned previously, the team wrote a grant pre=proposal to NSF for an Engineering Research Center based on AA, but again, it was not selected for submission of a full proposal Had it ultimately been awarded, those funds would not have specifically addressed the objectives in this project. The funds requested for this NRSP are for the specifics of developing databases and algorithms, which are essential to AA but challenging to support with other funding.
Integration
As part of this project, we intend to vastly expand the level of information sharing regarding AI-enabled AA that is occurring among researchers across the U.S. This project will add tremendous value by building concrete research connections among team members dealing with common issues as well as by enabling large-scale model creation for various critical AA needs through the development of large image, spectral signature, and 3D Lidar databases (free public domain). A good deal of effort and expense has already gone into developing image databases of important weed species. One result of these efforts is the recent release of “see and spray” technologies by John Deere, in which a large spray tractor equipped with a camera at each spray nozzle is used with AI to actuate nozzles only when weeds are detected, often saving large volumes of applied herbicide. While this is now a marketed technology, it is still in its infancy, and it is unlikely to work in areas where weed types are different from the training population. Furthermore, while some technology exists for insects, diseases, and nutrient management (e.g., the plantVillage dataset, which includes images of healthy and diseased plants), there is a concern that these conditions are not properly verified in the field. Other critical applications are in harvesting and post-harvest processing. In these cases, an object (e.g., strawberry) commonly must be identified, classified according to maturity status19 and quality, sorted, processed by slicing or some other means, or grasped and plucked from a plant. Nationally relevant images and other databases are an important step in the development of robust solutions. The participants in this project have already acquired many images of different crops and fields that can be shared at the beginning of this project.
Regarding expanding the level of AI-enabled AA information sharing among researchers, this project will establish – or contribute to existing – common databases and code libraries. The project will also leverage other funding sources by facilitating the development of national-scope projects focused on areas of common interest in AI-enabled AA.
Regarding dataset and codebase curation, we acknowledge the challenges of maintaining a single, centralized storage solution to curate all datasets indefinitely. Thus, the project will adopt a tiered strategy that integrates centralized and distributed cloud resources for managing organized datasets and developed algorithms (or AI system codebases). The first tier will be a dedicated website to serve as the project's central resource hub, maintained by the team. This homepage will provide links and concise descriptions of all curated datasets and codebases. The second tier is for individual datasets and codebases. The project will leverage existing democratized AI-supporting platforms. Specifically, guidelines will be provided for utilizing Hugging Face software for large imaging datasets and GitHub for codebases. These platforms will facilitate long-term curation of agricultural datasets and ensure their accessibility to the broader AI community for continued investigation. The third and final tier is the local backup solution. The project team will develop protocols for leveraging institutional data infrastructures to create local backup copies and for maintaining version control of data. These collective efforts aim to establish a centralized resource directory complemented by a distributed, sustainable data curation framework, allowing the implementation of FAIR guidelines for datasets and AI models to be collected and developed in this project and beyond.
The project will hold an annual meeting at one of the key universities in the project and invite researchers and industry representatives who are interested in AA to join and discuss collaboration. We envision that collaboration on data-collection standardization and dataset creation will proceed in two phases. In the first phase, the project team (PI and Co-PIs) will compile summaries of data acquisition practices they have used or are currently using for data acquisition for a wide range of crops. These summaries will include essential requirements and lessons learned. Using insights from data acquisition platforms, sensing modalities, and cropping systems, the team will then prepare a comprehensive white paper that systematically documents effective practices of data acquisition for various agricultural tasks. This document will serve as a foundation for developing standards to guide the team's ongoing and future research efforts involving data collection and management. In the second phase, the team will actively promote and disseminate the white paper through organized events such as conferences and workshops. They will also engage with relevant entities, including other multistate projects (e.g., S1090, S1098, and W4009) and ASABE’s Machine Systems (MS) and Information Technology, Sensors, and Control Systems (ITSC) communities. Furthermore, all team members on this project collaborate across disciplines, and attempts will be made to disseminate results through other technical societies such as ASA/SSSA/CSSA, AEAA, ASA/CSSA/SSSA, AHS, WSSA, ESA, and AAEA.
These efforts will introduce the proposed standards and train stakeholders on their application across a diverse range of crops, sensors, and platforms, thereby fostering standardized data acquisition and sharing practices. This approach aims to deliver a more systematic framework, enabling researchers from other land-grant universities (LGUs) to participate in and contribute to the project's data standardization efforts. The project’s lead PI will support a part-time program manager to ensure coordination, information sharing, and task completion regarding project goals and priorities.
Part of the solution to expanded information sharing is the development of a new multistate research project (S1098) that was established in October 2024. The expectation is that multistate funds will enable many interested parties across the nation to attend this multistate project meeting, and a preliminary poll of interested faculty members had over 50 responses, from universities in every region of the continental U.S. The support of this NRSP project for a program manager will also contribute to consistent planning, reporting, and follow-through for S1098. This proposed NRSP touches on the work of at least three multistate research projects, which deal with broad, open-ended research problems: S1090, S1098, and W4009 Integrated Systems Research and Development in Automation and Sensors for Sustainability of Specialty Crops
We will engage the broader group of stakeholders by including members on the project advisory board. We will engage with extension to ensure two-way communication with farmers, particularly considering farms of various scales. One vehicle for this is a USDA-NIFA funded project that Dr. Thomasson is currently involved in: The Social and Economic Impacts of Agricultural Autonomy (A2) on Small Farms: Status and Recommendations. In this project, surveys and focus groups are being conducted in conjunction with MSU’s Extension Service to inform growers about autonomous systems and to gain feedback from them. We will also engage with Extension regarding what data is most readily available to growers who would use AI-driven autonomous systems.
Finally, from an academic perspective, we intend to use the available assistantship funds to hire graduate students who may be in the disciplines of the funded Co-PIs or in computer science. It is important for students in agricultural/biosystems engineering and other agriculture-related fields to learn about AI, and it is equally important for computer science students to learn about applications of AI in agriculture.
Outreach, Communications and Assessment
The outreach, communications, and assessment plan for this project is designed to effectively communicate the program’s goals, accomplishments, and outcomes to a wide range of audiences. While the primary beneficiaries of the project will be other scientists working in AA and AI, careful consideration has been given to other potential end users of the information, including farmers, agronomic consultants, government agencies at the local, state, and federal levels, as well as the general public. Engaging these diverse stakeholders will ensure that the project’s results are not only disseminated widely but also utilized in ways that maximize negative impacts.
Intended Audience(s) and Stakeholder Engagement: The primary audience for this project includes researchers and scientists who are directly involved in AA, AI development, and related fields. The results will be disseminated through academic publications, conference presentations, and online platforms such as open-access journals and data repositories to ensure that these professionals can easily access and apply the project's findings. In addition to the scientific community, the project will engage stakeholders such as farmers and agronomic consultants, who will benefit from the practical applications of the research. Government agencies will also be engaged to help translate the project’s findings into policy recommendations and funding priorities. To ensure that the needs and perspectives of these stakeholders are incorporated into the project, the research team will involve them in various stages of the project, including the definition of research goals, data collection, and evaluation of results. Stakeholders will be invited to participate in focus groups, advisory panels, and team meetings where they can provide input and feedback on the project’s direction and outcomes.
Methodology for Measuring Accomplishments and Impacts: The project’s accomplishments and impacts will be assessed with a combination of quantitative and qualitative methods. Key performance indicators (KPIs) will be established to track progress toward specific objectives, such as the development and dissemination of open-source datasets, the number of AI algorithms developed and tested, and the extent of nationwide collaboration among researchers. Surveys will be distributed to participating scientists, stakeholders, and end users to gather feedback on the project’s outputs' effectiveness and applicability to real-world scenarios. The impact of the project on the scientific community will be measured through citation analysis, tracking how frequently the project’s publications are referenced in other research. Additionally, the project will employ professional evaluators to assess the quality and relevance of the research outputs, as well as the overall effectiveness of the communication and outreach efforts. Furthermore, the project includes an aspect of agricultural economics, to assess the economic benefits of AA.
Communication Pieces and Strategies: To effectively communicate the project’s activities, accomplishments, and impacts, a series of communication pieces will be developed. These will include detailed reports, policy briefs, infographics, newsletters, extension articles, and case studies that highlight the key outcomes of the project. These materials will be tailored to different audiences, ensuring that the information is accessible and relevant to each group. For example, policy briefs and case studies will be designed for government agencies and policymakers, while infographics, newsletters, and extension articles will be more suitable for the general public and agricultural producers. The communication pieces will be distributed through a variety of channels, including presentations at stakeholder meetings, seminars/webinars, field days, technology demonstrations, conferences, and workshops. The project will also leverage digital platforms such as websites, social media, and email newsletters to reach a broader audience. Additionally, the research team will work closely with the SAES/ARD directors, stakeholders, and their organizations, as well as funding sources and congressional delegations, to ensure that the project’s results are shared with the appropriate decision-makers and influencers.
Distribution of Results: The results of this research support project will be shared through several mechanisms. Annual meetings of stakeholders will serve as a key platform for presenting findings and discussing their implications. The research team will also provide material to the Budget and Advocacy Committee of NASULGC Board on Agriculture Assembly and other relevant SAES/ARD organization committees. Furthermore, the project will assist CSRESS in preparing documents that highlight the impacts of the research, ensuring that the results are communicated effectively to a broader audience. By implementing this comprehensive outreach, communications, and assessment plan, the project will not only achieve its research objectives but also ensure that its findings are widely disseminated and utilized, leading to tangible benefits for the agricultural sector and beyond.
Projected Participation
View Appendix E: ParticipationLiterature Cited
- Grift, T., Zhang, Q., Kondo, N., & Ting, K. C. (2008). A review of automation and robotics for the bio-industry. J. Biomechatronics Eng. 1(1):37-54.
- USDA Economic Research Service. 2023. https://www.ers.usda.gov/topics/farm-economy/farm-labor,accessed on 29 JUN 2024
- Shamshiri, R., Weltzien, C., A. Hameed, I., J. Yule, I., E. Grift, T., K. Balasundram, S., Pitonakova, L., Ahmad, D., & Chowdhary, G. (2018). Research and development in agricultural robotics: A perspective of digital farming. Int. J. Agric. Biol. Eng., 11(4), 1–11. https://doi.org/10.25165/j.ijabe.20181104.4278.
- Yang, Q., Du, X., Wang, Z., Meng, Z., Ma, Z., Zhang, Q., 2023. A review of core agricultural robot technologies for crop production. Comput. Electron. Agric. 206(1):107701.
- Reina, G., A. Milella, R. Rouveure, M. Nielsen, R. Worst, and M. R. Blas. 2016. Ambient awareness for agricultural robotic vehicles. Biosyst. Eng., 146(1):114-132; doi.org/10.1016/j.biosystemseng.2015.12.010
- Zhao, X., Y. Fang, H. Min, X. Wu, W. Wang, and R. Teixeira. Potential sources of sensor data anomalies for autonomous vehicles: An overview from road vehicle safety perspective. Expert Systems with Applications 236(1):121358. https://doi.org/10.1016/j.eswa.2023.121358.
- Bac, C. W., van Henten, E. J., Hemming, J., & Edan, Y. (2014). Harvesting Robots for High-value Crops: State-of-the-art Review and Challenges Ahead. J. Field Robotics 31(6):888–911. Inc. https://doi.org/10.1002/rob.21525.
- Barnes, E., Morgan, G., Hake, K., Devine, J., Kurtz, R., Ibendahl, G., Sharda, A., Rains, G., Snider, J., Maja, J.M., Thomasson, J.A., Lu, Y., Gharakhani, H., Griffin, J., Kimura, E., Hardin, R., Raper, T., Young, S., Fue, K., Pelletier, M., Wanjura, J., Holt, G., 2021. Opportunities for Robotic Systems and Automation in Cotton Production. AgriEngineering 3, 339-362.
- Fue, K., Porter, W., Barnes, E., & Rains, G. (2020). An Extensive Review of Mobile Agricultural Robotics for Field Operations: Focus on Cotton Harvesting. AgriEngineering, 2(1), 150–174. https://doi.org/10.3390/agriengineering2010010.
- Li, B., Vigneault, C., & Wang, N. (2010). Research development of fruit and vegetable harvesting robots in China. In Stewart Postharvest Review (Vol. 6, Issue 3, pp. 1–8). https://doi.org/10.2212/spr.2010.3.12.
- Li, P., Lee, S. H., & Hsu, H. Y. (2011). Review on fruit harvesting method for potential use of automatic fruit harvesting systems. Procedia Engineering, 23, 351–366. https://doi.org/10.1016/j.proeng.2011.11.2514.
- Williams, H. A. M., Jones, M. H., Nejati, M., Seabright, M. J., Bell, J., Penhall, N. D., Barnett, J. J., Duke, M. D., Scarfe, A. J., Ahn, H. S., Lim, J. Y., & MacDonald, B. A. (2019). Robotic kiwifruit harvesting using machine vision, convolutional neural networks, and robotic arms. Biosyst. Eng.. 181(1):140-156. https://doi.org/10.1016/j.biosystemseng.2019.03.007.
- Diwan, T., G. Anirudh, and J. V. Tembhurne. Object detection using YOLO: Challenges, architectural successors, datasets and applications. Multimedia Tools and Applications 82(6):9243-9275. https://doi.org/10.1007/s11042-022-13644-y.
- Steyaert, S., M., Pizurica, and D. Nagaraj. Multimodal data fusion for cancer biomarker discovery with deep learning. Nature Machine Intell. 5(1):351–362. https://doi.org/10.1038/s42256-023-00633-5.
- Mumuni, A., and F. Mumuni. Automated data processing and feature engineering for deep learning and big data applications: a survey. J. Information and Intelligence 1(1):2949-7159. https://doi.org/10.1016/j.jiixd.2024.01.002.
- Sapkota, R., Meng, Z., & Karkee, M. (2024). Synthetic meets authentic: Leveraging llm generated datasets for yolo11 and yolov10-based apple detection through machine vision sensors. Smart Agricultural Technology, 9, 100614.
- Qiu, T., Du, R., Spine, N., Cheng, L., & Jiang, Y. (2025). Joint 3D Point Cloud Segmentation using Real-Sim Loop: From Panels to Trees and Branches. 2025 International Conference on Robotics and Automation (ICRA), Accepted.
- Qiu, T., Zoubi, A., Spine, N., Cheng, L., & Jiang, Y. (2024). (Real2Sim)−1: 3D Branch Point Cloud Completion for Robotic Pruning in Apple Orchards. 2024 IEEE/RSJ International Conference on Intelligent Robots and Systems (IROS), 23-30. http://doi.org/10.1109/IROS58592.2024.10803058
- Kondo, N. Automation on fruit and vegetable grading system and food traceability. 2010. Trends Food Sci. Technol. 21(1):145-152; doi:10.1016/j.tifs.2009.09.002.