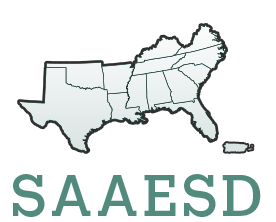
S1069: Research and Extension for Unmanned Aircraft Systems (UAS) Applications in U.S. Agriculture and Natural Resources
(Multistate Research Project)
Status: Active
S1069: Research and Extension for Unmanned Aircraft Systems (UAS) Applications in U.S. Agriculture and Natural Resources
Duration: 10/01/2021 to 09/30/2026
Administrative Advisor(s):
NIFA Reps:
Non-Technical Summary
Statement of Issues and Justification
Biotic and abiotic stresses are key limiting factors in crop and animal production as well as in managed ecosystems. Characterizing plant and animal behavior in response to changing environmental conditions is a critical pursuit for researchers across the country, necessary for breeding and genetic improvement as well as for improving management practices to maximize resilience and productivity. Remote sensing with unoccupied/unmanned aerial systems (UAS, also referred to as drones) has recently demonstrated the ability to characterize crops, animals, forests, estuaries and their various stresses at much higher throughput than previously possible (Shi et al., 2016; Resop et al., 2019; Chandel et al., 2021). This capability will, for example, enable breeders to make rapid and robust selection decisions and farm managers to quickly respond to stresses with appropriate mitigation tactics. UAS can even potentially be used to effectuate those mitigation tactics by, for example, spot-applying input materials such as herbicides. However, UAS use in agriculture and natural resources is still at an early stage, and substantial development is needed for each situation and to enable routine use.
While UAS data collection is becoming common in agronomic research, improvement is needed in the types of measurements, in the accuracy and repeatability of the measurements, and to enable positive impacts through knowledge and decision making. UAS has not yet taken hold in production agriculture, although many of the larger seed and agricultural companies have started to incorporate it in specific breeding and management tools. Early interest in agricultural UAS resulted in substantial and growing financial investment in UAS startups (over $700M by 2017), with over $200M invested in 2017 alone (Davis, 2017). Over a five-year period from 2012 to 2017, investments in agricultural technology including UAS increased by 80% annually (Walker et al., 2016). A wide range of UAS platforms and sensors is now available in the marketplace, but UAS have yet to directly enhance profitability in agriculture. A recent survey indicated that only 25% of agricultural service dealers who offer UAS services were seeing a positive return on investment (Erickson and Lowenberg-DeBoer, 2020). The value of UAS in production agriculture will remain low until research can clearly demonstrate reliable systems and workflows that provide return on investment. A major difficulty is that agricultural systems are extremely complex, with many potential stressors that can appear simultaneously, and their measurable symptoms are often indistinguishable. On the other hand, most published UAS research has focused on proving an application in single fields, single crops, and single physiological phenotypes or stresses; it has thus been far from having comprehensive appeal. Methods that have broad applicability across multiple fields, crops, times, and regions are essential to exploiting the benefits of UAS in production agriculture.
Another large gap is in education on UAS usage, both in classroom and extension environments. Such education is needed not only by those in farming enterprises but also by researchers and crop consultants who interact with them. In a recent survey of over 13,000 members (2,181 responding) of Certified Crop Advisors (2020), only 43% are comfortable using UAS for remote sensing. It is telling that 38% are not yet using aerial imagery, while 16% are using such imagery more than once per week. This large disparity could be alleviated with educational programming. When respondents were asked what type of education they would like more of, the top answers were prescription field management (63%), remote sensing (56%), use of on farm sensors (50%), and UAVs (33%).
Researchers at individual universities often lack sufficient crop diversity and geographic variability to adequately demonstrate broad UAS utility. Furthermore, their limited resources reinforce such a restricted focus. Therefore, a formal mechanism for communication and collaboration is necessary to facilitate the multi-institutional activities in UAS needed to adequately support the Land Grant missions of research, education and extension across the US. With a community built from a multistate approach, we expect to more rapidly advance UAS applications and practices and clearly demonstrate their benefits.
Related, Current and Previous Work
Research and development for UAS in agriculture has focused primarily on remote sensing applications, the greatest progress being in crop-plant characterization, but research has also been conducted in livestock, forests, and freshwater and marine resources. Relative to alternative remote sensing options such as satellites, UAS have ability for markedly improved revisit intervals and better resolution, however they have limited ability to cover large areasacreages. However, the use of UAS is justified, even for larger missions, under certain conditions. The responsiveness of UAS remains one of its most stalwart advantages. Although newer satellite platforms offer near daily re-visit potential, cloud occlusion is still a complication to reliable collection of image data, particularly in humid regions. With older satellite systems such as Landsat, research shows the probability of having any one image free from cloud cover is approximately 80% (Roy and Ju, 2008). However, the need for consecutive images, as often occurs in agriculture for monitoring of crop development, reduced the probability for success to 60% in critical summer months. Thus for agronomic stresses of immediate importance, it is essential to have the increased flexibility that UAS can provide, operating when openings in the cloud deck permit and having multiple hours for collection compared to a single window of time when the satellite passes overhead. In fact, UAS can still collect data when clouds are overhead, though the quality of the data is typically reduced. Additionally, satellite resolution, although much improved than in decades past, does not even approach the spatial resolution available with UAS, particularly for thermal infrared imaging. One benefit of a tandem satellite-UAS approach to data collection is the synergy achieved through data fusion (Maimaitijiang et al., 2020). Matching UAS imagery to satellite imagery can enable validation of satellite data and scaling of UAS results, thus overcominge the potential hurdles imposed by the smaller areal coverage of UAS systems currently available to most agricultural researchers. Furthermore, UAS platforms can be customized for atmospheric, weather and air quality sensing/mapping in vertical and horizontal spatial dimensions as required for some of the very specific agricultural and natural resources user-cases. Such scientific data can’t be collected using high or low-orbiting satellites. Finally, for small plot and plant breeding experiments only UAS have the resolution and revisit time to allow new approaches to differentiate varieties and predict the highest yielding or other desirable traits (Adak et al. 2021).
In addition to strictly characterization of crop plants and other resources, research has also been conducted in spatiotemporally precise economic and environmental management of inputs and resources relative to UAS-based measurements (i.e., what can be done with the measurements being made). UAS currently consists of an aerial platform, positioning and communication devices, and imaging sensors, but other capabilities can be added. For instance, exciting research has begun to consider UAS as a management tool as well (e.g., spot-spraying weeds). All these efforts are discussed below, starting with remote sensing, in order from the most developed (UAS high-throughput plant phenotyping in the field) to the least (UAS for measurements in marine environments), and ending with a focus on applications not involving only remote sensing, such as aerial chemical applications. In each section, some fundamental aspects and literature in the field of study are discussed, with selected examples from current S1069 membership interest.
High throughput phenotyping and characterization
High-throughput phenotyping (HTP) is a data collection approach in which researchers use sensors on autonomous or semi-autonomous platforms to make phenotypic measurements in lieu of measurements by humans. Already a number of field HTP methods based on UAS remote sensing have been reported and used extensively in the research community for extracting traits like plant height (Pugh et al., 2018), plant population (Sankaran et al., 2015), lodging level (Chu et al., 2017), biomass level (Bendig et al., 2014), pest density (Stanton et al., 2017; Wiesner-Hanks et al., 2018), etc. Where the crop’s end-product is visible (e.g., wheat and sorghum grain and open cotton bolls), yield may be estimated before harvest from overhead images (Malambo et al., 2019; Yeom et al., 2018). Predicting yield before the crop's end-product is visible may prove more useful to growers in the long run, because it may enable them to adjust management tactics earlier in the season, so much research remains here. Furthermore, the prediction of crop quality traits is also being considered. Replacing manual measurements can be validated when there are high correlations with manual measurements. Most UAS-based HTP research studies fly small areas (< 2 ha) of replicated research plots, but once a method and algorithm have been developed, current UAS can conduct flight missions over a 20-ha field in about 20 min, covering thousands of plots of varying genotypes. Collecting this amount of data manually would be resource-prohibitive or even impossible. Furthermore, UAS technology allows data collection temporally during growth, which has never before been feasible.
An example of UAS-based HTP research involves ongoing breeding work at Texas A&M University involving measurement of plant height in Texas maize (Zea mays L. aka corn). While plant height is not indicative of maize crop yield in all growing regions, in Texas commercial varieties it is 40% to 60% correlated with yield (Farfan et al., 2013) and useful in indicating stressful conditions throughout the Southern U.S. in an assortment of crops. Plant height has historically been determined with a measuring stick at the end of the season before harvest, providing only a terminal growth measurement. Manual plant height measurement is labor intensive, but it can be automated with UAS images and Structure-from-Motion (SfM) calculations (Pugh et al., 2018). Among the first related studies, plant height in small plots was measured with moderate to high precision with imaging (Chapman et al., 2014) and light detection and ranging (LiDAR) (Anthony et al., 2014). In short order this capability was used to show UAS-based temporal growth curves, modeled from seedling to harvest, which discriminate genotypes and better predict yield (Anderson et al., 2019). Furthermore, with DNA markers it has even been demonstrated that different regions of the genome are responsible for early season growth, the manifestations of which are not detectable later in the season (Anderson et al., 2021). Moreover, the same types of plant structure imaging could be used to estimate temporal biomass accumulation (Temu et al., 2021), beyond plant height alone; such estimates are advantageous for both breeding and management decisions in field crops like forages.
Another example involves measurement of leaf area index (LAI) and other traits in multiple crops. Virginia Tech’s Tidewater Agricultural Research and Extension Center is conducting research to develop HTP methods for screening LAI and wilting from UAS images collected with a multispectral camera. LAI, the ratio of total one-side leaf area to ground area, is a proxy for plant biomass accumulation, radiation interception by leaves, and plant photosynthesis, growth, and yield (Breda, 2003; Chen and Black, 1992; Watson, 1947). Reduction of LAI in several crops including peanut (Arachis hypogaea L.), soybean [Glycine max (L.) Merr.], alfalfa (Medicago sativa L.), sorghum [Sorghum bicolor (L.) Moench], barley (Hordeum vulgare L.), and wheat (Triticum aestivum L.) (Breda, 2003; Fang et al., 2012; Ma et al., 1992; Nutter Jr and Littrell, 1996) has been related to reduction of biomass and yield. Direct measurement of LAI is slow and limited to a few plants sampled from an entire plot. Similarly, rating wilting for thousands of breeding plots is time consuming and subject to human error (Milberg et al., 2008). However, assessment of wilting is a critical component of breeding research programs. Visual rating based on morphological changes of leaves resulting from reduced turgor pressure is an important tool to quantify leaf wilting and drought tolerance in plants (Balota and Oakes, 2017; Engelbrecht et al., 2007; Hamidou et al., 2012; Luis et al., 2016; Zhou et al., 2020). UAS-based methods with low-cost cameras and vegetation indices can intensify phenotyping in breeding programs (Borra-Serrano et al., 2018). Advances in this area will allow for automated, repeatable, and high-resolution breeding solutions for future crops.
Small fruit, tree fruit, and nuts
The small fruit, tree fruit, and nut industries face significant challenges to elevate production while maintaining high-quality products. Aside from common production stresses like pests, pathogens, and environmental conditions, these industries are acutely susceptible to declining labor availability, international competition, and increasing consumer demand for improved quality and reductions in chemical inputs. Growers of these commodities must elevate production efficiency considerably to remain profitable and sustainable. Research and extension efforts are critical to developing and communicating new technologies for scouting and treatment of production pests.
Researchers at the University of Georgia (UGA) and Washington State University (WSU) are using UAS with ground-truth measurements to develop indices that quickly recognize disease presence and environmental stressors in several important crops. For example, Xylella fastidiosa (Xf) causes bacterial leaf scorch of blueberry and pecan as well as phony peach disease (PPD) in peaches. Though Xf currently infects pecans, peaches, and blueberries only in southern states, a changing climate may result in the spread of Xf-induced diseases to more northerly regions. UGA researchers are conducting a study to identify spectral indicators (e.g., NDVI, NDRE and RGB-reflectance) of the Xf pathogen in peaches, pecans and blueberries. The end goal of the Xf research is to determine the optimal timing and spectral indices for identifying infected peach trees -- and later pecans and blueberries -- with UAS so that control measures can be deployed. Another example of disease in specialty crops is Phytophthora cinnamomi (Pci), which causes root rot in blueberries and is prevalent in poorly drained areas. Infection symptoms include poor shoot growth, root necrosis and dieback, yellowing or reddening leaves, and in severe cases dead canes and branches and plant death. Prevention and early treatment are critical. Proper drainage can prevent disease outbreaks, but containment is also important. UGA researchers are attempting to determine whether root rot can be diagnosed with UAS images and then spot-sprayed to contain its spread. They surveyed an affected field with a UAS carrying a multispectral sensor, and NDVI and RGB-reflectance values were used to identify symptomatic areas. The goal is to develop a method to identify infected locations in an orchard and create a prescription map for a UAV to spot-spray those locations.
Similarly, the WSU team is exploring apple powdery mildew (PM) infestation detection and mapping using UAS-based high-resolution multispectral imaging combined with advanced machine-learning (Chandel et al., 2020). Attempts with RGB-image snapshots have been shown to have disease detection accuracy of 77% (with K-means clustering), whereas multispectral vegetation indices (VIs) could also differentiate healthy (Mean: 0.25–0.84) and infected (Mean: 0.01–0.25) leaves. Maps derived from these VIs could be useful to apple growers in directing resources (hand pruning labor or fungicide application) for precision management, so the team is further enhancing the data-analysis workflow and data-visualization tools. Besides disease mapping, UAS based imagery can also help in understanding crop water use such that tree-fruit, grape, and small fruit growers can implement timely irrigation management for optimal fruit quality and yield. To this end, the WSU team has explored the application of small UAS-based multispectral imagery to map crop water use (evapotranspiration or transpiration) of perennial crops grown in the U.S. Pacific Northwest. For example, they validated the crop water-use (i.e., transpiration) mapping approach with the modified energy balance model (Chandel et al., 2021) over three seasons with UAS images collected in cooperating commercial vineyards. They are also exploring the applicability of this approach in commercial apple orchards where fruit quality is strongly affected by in-season irrigation management and evaporative-cooling based heat stress management.
Field crop production
Practical applications of UAS remote sensing are currently limited for growers and service providers (e.g., fertilizer applicators). While UAS technology has matured, on-farm methods are still largely application-specific, requiring translation and calibration between crops and climates (Ishimwe et al., 2014) as well as varying light conditions, soil conditions (Muller and Decamps, 2001), crop moisture levels (Carter, 1991), etc. This situation is exacerbated by differences in sensors and payloads (von Bueren et al., 2015). Research is needed to determine the effectiveness and specificity of stress-detection with UAS images as well as the economic viability of UAS remote sensing as a tool. Clear protocols for use of UAS and methods for management decisions based on UAS remote sensing are critical. Ideally these systems, protocols, and methods would be invariant to extraneous factors such as geography and season.
One example of a field-crop management decision that could benefit from UAS remote sensing involves disease in peanuts. In the Virginia-Carolina peanut region, leaf wilting is a symptom of drought stress but also of white mold soil-borne disease (caused by Sclerotium rolsfii [Sacc.]). Both stresses can reduce yield, but the distribution of wilting varies between the two. Relatively uniform wilting indicates drought stress from heat and lack of rainfall, while isolated patches of wilting are indicative of disease. Research efforts are currently underway to differentiate among the stressors that cause wilting and yield reduction in peanut fields in the region. The ability to identify the cause of wilting could be used to trigger remediative actions (e.g., irrigation in response to drought stress), as well as to avoid unnecessary inputs (e.g., irrigating the crop when disease is the cause of wilting, not lack of water). Researchers at Virginia Tech are using UAS remote sensing to determine crop water status and wilting distribution, similar to prior work in Texas involving remotely sensed canopy temperature for triggering irrigation (Evett et al., 1996). Used in this way, UAS can be a tool to ensure economical water use, a fact corroborated by similar research in Georgia (Rowland et al., 2012). This kind of research is critical, because determining economic benefit is critical to on-farm use of UAS.
Another disease-related example involves cotton root rot (CRR) disease (caused by Phymatotrichopsis omnivora). Uniformly applying flutriafol fungicide to CRR-infested fields has become common practice over the last decade on cotton farms with CRR prevalence. However, the disease affects only 10 to 75% of a field and is not homogeneously distributed in fields, but it does occur at the same field positions each year. Remotely sensed historical positions of CRR incidence can be used to precisely apply fungicide in subsequent years. Therefore, variable-rate technology (VRT) can be used to automatically adjust the fungicide application rate, thereby reducing cost by eliminating unnecessary application. Yang et al. (2014) developed prescription maps based on images collected with manned aircraft and successfully used VRT to apply fungicide during planting to control CRR, applying the full rate in known infested areas and zero in non-infested areas. More recently, a research team involving Texas A&M and USDA-ARS (Wang et al., 2020a) successfully developed methods to use UAS remote sensing to develop these prescription maps. In related work, Wang et al. (2020b) took advantage of the high resolution of UAS images to improve the method such that it is capable of applying fungicide at levels approaching that of a single plant. This research also led to the development of automated algorithms to produce the prescription maps directly from mosaics of UAS images (Wang et al., 2020c), and it clearly demonstrated economic and environmental benefits of UAS remote sensing.
Livestock
UAS remote sensing has potential in monitoring and management of cattle, sheep, horses, swine, and even poultry. Research must be done to create “field edge” solutions that can autonomously make data-driven decisions for livestock management. For pasture-based research, monitoring multiple fixed locations of interest (e.g., waterers, shelters, shade structures, feed bunks) is not practical, because the locations can be numerous, remote, and lack necessary electrical utilities for sensors. Nonetheless, the speed and altitude of UAS enable coverage of large areas (roughly 2.0 ha per image at 120 m above ground level, depending on camera and lens). UAS research in detecting, locating, and quantifying animals would enable this inefficient manual task to be automated and conducted expeditiously. Determining which animals are not located with the main group would indicate potential health issues with the missing animals or possibly pending parturition. Alternatively, the inability to detect particular animals in a field may suggest escaped livestock (requiring rapid response). Additionally, forage quality and quantity for grazing livestock can potentially be ascertained with UAS (Pullanagari et al. 2018; Temu et al. 2021). Interestingly, UAS is not relegated to outdoor operations, as confined livestock operations (poultry, swine, and dairy) can also benefit from it. Prior work at Mississippi State University (Parajuli et al., 2019) demonstrated the use of UAS in poultry houses to monitor the flock.
With 18% of total agricultural commodity receipts, the largest agricultural industry is cattle production (USDA ERS). Researchers at the University of Kentucky are using UAS to monitor the health of beef cattle. As part of this research, the physiological and behavioral response of cattle to UAS is being evaluated to determine altitude and approach angle recommendations for producers. Work performed to date suggests that UAS monitoring of pasture-raised cattle at or above 10 m AGL will not induce significant changes in cattle behavior or physiology. Moreover, 3-D rendering of life-sized cattle figures in pasture with a UAS has demonstrated the potential for making volume measurements (Pampolini, 2020), from which weight can be estimated in the field. As cattle are bought and sold on a weight basis, this capability would be tremendously beneficial, enabling market decisions without having to work the animals through a handling facility.
Natural Resource Management
Previous UAS studies related to natural resources have identified key knowledge gaps, calling for research on soil erosion at fine-spatial and medium-temporal scales (Ciampalini et al., 2012) and inexpensive and rapid analysis approaches that quickly process a large number of samples for tracer-based soil erosion studies (Guzmán et al., 2013). Soil erosion is a primary conservation concern due to impacts on soil health, land productivity, and surface water quality. Despite increases in conservation practices, erosion remains a threat to agricultural sustainability (Montgomery, 2007). Quantitative studies on erosion are necessary to provide a basis for effective conservation and land management strategies (Guzmán et al., 2013). UAS remote sensing is capable of providing realistic and geometrically accurate 3D representations of landscapes. These 3D models can be created with image-based photogrammetric techniques (Structure from Motion, SfM) or from UAS-based LiDAR. The SfM process results in a visually appealing product that can be viewed from different angles, and with ground control data the 3D models can be geometrically accurate and thus used for more exacting applications. Researchers at Virginia Tech have used UAS remote sensing to map riverscapes and found that drone-based laser scanning (DLS) provided higher-resolution data that were better than those collected from manned aircraft (aerial laser scanning, ALS) at providing details of the channel profile as well as detecting small vegetation on the floodplain (Resop et al., 2019). This team is now finalizing research comparing UAS-based SfM with DLS for mapping riverscape characteristics, and initial findings suggest that both provide similarly dense point clouds, but SfM imagery has greater difficulty sensing through thick vegetation (Prior et al., 2020).
Mississippi State University has used UAS remote sensing to map flooding. The researchers developed a mobile device application that can be used to visualize flooding on a landscape via augmented reality (Prince Czarnecki et al., 2020). The first version used ALS, but recent tests have verified that SfM products from UAS data produce similar results. SfM surfaces generally have a higher spatial resolution, can be generated for a fraction of the cost of ALS, and have similar resolutions to DLS (Prior et al., 2020). The Virginia Tech group is utilizing DLS and SfM to map floodplains, with a particular focus on estimating vegetated roughness of floodplains for use in 2D hydrodynamic modeling (Aquilina, 2020; Prior et al., 2020). These efforts seek to change the way professionals assess managed lands, implement conservation on the landscape, and predict increased flood hazards due to climate change. Advances in UAS-based natural resource mapping will be a significant tool for precision conservation.
Aquaculture
Aquaculture is the fastest growing protein industry in the world (FAO, 2018), and an existing $17 billion seafood-market shortfall is driving development of new, larger, nearshore marine aquaculture systems to meet U.S. demand (NOAA, 2018). Understanding water quality and quantity is critical for aquaculture, and UAS remote sensing can potentially provide low-cost, rapid monitoring in coastal (mariculture) and inland aquaculture operations. UAS have the potential to add tremendous value in mariculture environments for water quality observation, as these environments are difficult to access and require monitoring at large scales. Most mariculture operations are located within 3 miles of the coast, and conditions within the water column in these nearshore production sites can become unfavorable due to chaotic near-shore processes and limited control over system boundaries, which also present concerns for food safety. The opening and closing of production areas like oyster reefs over food safety concerns are dependent on water quality, specifically fecal coliform levels in the water. Temporary harvest closures on the East Coast due to water quality concerns can cause over 25% total revenue loss to the industry (Evans et al., 2016). Inland aquaculture operations tend to be on smaller scales but can be even more susceptible to water quality issues, a key factor in determining the quality of products and the economics of production. In both environments, UAS can offer high-resolution remote sensing of water spectral properties for estimating water quality.
Applications for UAS include mariculture site selection and production monitoring. For example, growth modeling can be coupled with UAS-derived estimates of chlorophyll-a and total suspended solids concentrations from multispectral imagery to determine future production sites (Palmer et al., 2020). Multispectral imagery can also be used to estimate dissolved oxygen (DO) and turbidity in aquaculture production areas (Wang et al., 2020d), two parameters critical for aquatic organism health. Researchers at North Carolina State University are using multispectral imagery from UAS to measure water quality parameters related to production and performance, including chlorophyll, turbidity, and DO concentrations. Through these efforts, they plan to build and improve on existing models by generating remotely sensed multispectral data in nearshore shellfish production environments and collecting ground truth data by manual grab sampling for model development and evaluation. They also plan to develop more advanced UAS capable of in situ water sampling (e.g., Koparan et al., 2018) to measure water quality parameters like fecal coliform bacteria concentrations, which present serious food safety concerns but cannot be sensed remotely. These efforts will advance data collection for inland and coastal aquaculture to enable data-driven management decisions that ensure food safety, higher yields, and sustainable growth.
Agricultural UAS uses beyond remote sensing
Numerous applications in agriculture and natural resources could benefit from the unique capabilities of UAS beyond remote sensing. Traditionally, manned aircraft have been used for spraying and dusting applications in various U.S. crops. Irregularly shaped fields and the presence of power lines, communication towers, and various types of buildings present risks for damage to equipment and loss of life, not to mention the expense of the operation. UAS can potentially spray and dust fields and even spot-spray or spot-spread crop protection materials when sensors provide precise locations. UAS are likely to operate at much lower cost and present less risk than current solutions. Integrating application technology into UAS requires research in application efficacy, choice of nozzles, optimal nozzle placement to harness rotor downwash, and data-driven real-time actuation. Various crops with different row spacing and foliage densities create challenges and hence demand additional research in this area (Woldt et al., 2018).
In 2015 the FAA approved mid-sized UAS (e.g., Yamaha RMAX® with a max takeoff weight of 94 kg) for commercial agricultural operations. A team from the University of California-Davis tested the potential of using this UAS for chemical applications in rolling vineyards of wine grapes to determine its safety and efficacy relative to ground sprayers and manned helicopters (Giles, 2019). UAS also have potential uses for horticultural production loss management. For example, in cherry orchards, seasonal summer rain can damage sweet cherries for fresh markets by producing cracks on the skin or near the stem. In the Pacific Northwest, growers currently use traditional helicopters that fly over cherry crops after a rainfall to remove rainwater from cherry fruit surface and canopies, a costly and dangerous process. As an alternative, a research team at Washington State University tested a Yamaha RMAX with 3.1-m rotor diameter for effective rainwater removal. At its max payload, a mid-sized UAS was able to effectively remove 88 to 96% of rainwater operating at 2.7 m/s and 6.1 m above ground level (Zhou et al., 2016). Ideally, growers can use such technology for a range of operations in production management. Similar to the cherry-drying concept, a UAS could potentially be used for frost control in vineyards, cherry, and apple orchards.
Objectives
-
To research and develop UAS-based systems and methods for precise economic and environmental management of inputs in production of crops, livestock, freshwater aquaculture as well as mariculture, and forests and other natural resources.
-
To research and develop UAS-based remote sensing systems and methods for field phenotyping and characterization in crops, livestock, freshwater aquaculture as well as mariculture, and forests and other ecosystems.
-
To develop an understanding of stakeholder needs and produce classroom and extension education and training resources for UAS-based monitoring and management technologies for agriculture and natural resources.
Methods
Objective 1. A key aspect of the research on Objective 1 will be a focus on the economic viability and environmental effects of UAS integrated methods on-farm inputs and resources management. Furthermore, several questions on the suitability and scale of UAS-based sensing and management technologies need to be answered for adaptation in production of crops, livestock, freshwater aquaculture as well as mariculture, and forests and other ecosystems. Research on use protocols (i.e., best practices) will be conducted through synthesis of learnings from ongoing and new collaborative research projects among the multi-state members. These best practices will also be researched for potential customization for specific use cases. For example, a range of sensors, available or in concept, being integrated with UAS, need calibration and metadata collection protocols and data analysis templates for practical use in biotic and abiotic stress assessment. We will develop teams with common interests on specific use cases (e.g., crop stress quantification) to investigate and develop these protocols. Algorithms and models (application-specific) developed will predict stresses with UAS based sensing data from applicable sensors. Protocols, algorithms and models developed to evaluate stresses, etc., will be validated in multiple environments and various geographical areas for their applicability. The intent will be to produce broadly based results confirming that specific UAS practices have positive economic and/or environmental benefits in important common applications. Members will also undertake efforts to understand and model UAS platform attributes (e.g., number of rotors, their configuration, all-up weight changes and associated downwash and airflow swirl variations) and spray applicator (e.g., nozzle) configurations for effective input (e.g., foliar nutrients or plant growth regulator) applications with minimal to no material drift or losses. Pertinent results will be disseminated through peer-reviewed publications and presentations at professional national and international meetings. Efforts will be made to expand the membership of S1069 to include adequate representation from agricultural economists. Efforts will also be made to engage and transfer knowledge with other multistate projects having common interests. While research teams consisting of subsets of participants tend to form organically, teams will be encouraged to incorporate appropriate disciplinary breadth; e.g., economics, engineering, modelling, legal, agronomics, horticulture, pest management, information technology, etc. Where appropriate, efforts will be made to engage with UAS hardware and software manufacturers and designers/engineers.
Objective 2. UAS-based field phenotyping and characterization within the scope of this project fall into three primary approaches: a) the automation of measuring existing phenotypic traits; b) the development of novel and previously infeasible measurements; and c) the development of new “random” phenotypes (previously unknown to be relevant or useful) as markers for germplasm characterization. Automation of measuring existing traits by UAS (e.g., height or lodging [Chu et al. 2018]) has been explained previously, but substantial protocol and tool development is still needed to improve cost and time efficiency, accuracy, routineness, and the ability to easily and repeatedly scale across crops, investigators and environments. The trade-off between measurement accuracy and the number of measurements is a major consideration in UAS-based phenotyping, and it was recently shown by computer simulation that increasing the volume of low-quality data can produce better decisions than more high-quality data in certain circumstances (Lane et al. 2021). Furthermore, it has been shown that, in some cases, UAS data is superior to ground truth data (Pugh et al. 2018). An example of the development of new, previously infeasible, measurements with UAS involves temporal measurements that scale over time throughout growth, allowing tracking of the development of different varieties and the identification of relationships with terminal traits of economic interest (e.g., yield). Such temporal measures can be modeled to generate additional and more powerful selection criteria (Anderson et al. 2019) as well as new fundamental understandings of plants (Anderson et al. 2020). The development of “random” phenotypes as markers for germplasm characterization is challenging to scientific dogma but has proven useful in the same way that genetic markers are used in genomic selection to characterize and predict the best varieties in breeding. Generally termed “phenomic selection” (Rincent et al. 2018, Lane et al. 2020), the collection of “random” features of crop plants en masse, enables relationships between genotypes to be characterized, and these can be useful in breeding and genetics models to predict yield and the best yielding varieties in much the same way as genomic selection has been using genomics tools. Furthermore, these random phenotypes might help to discover important new fundamental biology, such as growth timepoints, spectral features or physical characteristics that predict yield or stress. It is relevant that all three approaches can use the same experimental UAS conditions and data collection but require different downstream analyses. Members of this group will thus develop new methods, calibration protocols, as well as documented work flows and data repositories pertinent to phenotyping and characterization of crops, livestock, forests, etc. Similar to objective 1, results will be disseminated through peer-reviewed publications and presentations at professional national and international meetings. Efforts will be made to engage and transfer knowledge with other multistate projects having common interests. While research teams consisting of subsets of participants tend to form organically, teams will be encouraged to incorporate appropriate disciplinary breadth; e.g., economics, engineering, modelling, legal, agronomics, horticulture, pest management, information technology, etc. Where appropriate, efforts will be made to engage with UAS hardware and software manufacturers and designers/engineers.
Objective 3. We will use online tools (e.g., Qualtrics) as well as in-person surveys during extension/outreach events conducted by members to better understand stakeholder (academic, industry, growers) needs related to UAS technology. High-quality questionnaires are critical to meaningful feedback, so members will work with university extension faculty and sociologists to develop these questionnaires. The goal is to identify additional research and education needs in the domain areas. Members will continue to build teams organically and develop customizable extension education material (e.g., editable Powerpoint files), technology-use and case-specific fact-sheets, and videos. Such material will be published on the S1069 website and through participating member’s regional groups (e.g., Pacific Northwest Extension publishing). Efforts will also be made to develop UAS-technology course material and teaching cohorts from within this group. For example, a course on “UAS in Agriculture and Natural Resource Management” will be developed with modules on fundamentals of UAS technology, sensing principles and sensor types, sensor integration and calibration methods, basics of data analytics, application-driven hands-on training modules, current Federal Aviation Administration (FAA) rules (Part 107), local regulations and safety aspects. Efforts will be made to expand the membership of S1069 to include adequate representation from agricultural extension/education professionals. Where appropriate, efforts will be made to engage with UAS hardware and software manufacturers and designers/engineers.
Measurement of Progress and Results
Outputs
- New software and/or computer code for analyses of UAS data for agriculture and natural resources Comments: Efforts will be made to coordinate code development, validation and usage across institutions. The number of released new software packages/code and publications using new software / code are both measures of progress in this area, the latter needing greater resources to measure.
- New hardware/software systems and protocols (best practices) for consistently efficient and effective use of UAS for agriculture and natural resources. Comments: Efforts will be made to coordinate protocols/best practices development, joint hardware/software system prototyping and usage across institutions and use cases . In addition to those at the multi-state annual meeting, the number of released new hardware/software systems and protocols can be measured through presentations and publications.
- New applications for efficient and effective use of UAS for agriculture and natural resources. Comments: Sharing of research/education efforts by members will occur during multi-state annual meetings. This activity will stimulate inquiry regarding explored UAS technology (platform, sensors) and associated data analysis protocols to new unexplored applications.
- Framework for consistent data and metadata formats to be adopted for data sharing to enable collaborative research Comments: Efforts will be made to coordinate data usage across institutions to the extent possible for these activities. Multiple individuals and efforts have worked towards developing consistent data and metadata formats on UAS already but this is a huge undertaking for the larger community and this project can only provide guidelines for its members.
- Scientific publications validating the utility of UAS and specific protocols for particular applications. Comments: Efforts will be made to encourage coordinated development of multi-state research and& extension projects, implementation, and co-authorship of peer-reviewed publications. These can be measured as counts and citations, although additional resources might be needed for adequate tracking.
- Teaching modules and training materials for extension education/outreach on UAS in agriculture and natural resources. Comments: Efforts will be made to coordinate training material development and usage across institutions. These will be reported and shared at our multi-state annual meeting and implemented by our members. These are less likely to be published or communicated than research accomplishments so additional effort will be needed to encourage members to report on this activity.
Outcomes or Projected Impacts
- Usage of the outputs above by the greater community of UAS users in agriculture will be an important outcome and realize the largest impacts of the project - as highlighted below. Usage can be tracked by proxy through downloads, workshops held, or social media. Ultimately citations will be among the most reliable measures, but citations can lag by years and recognizing future authors do not always appropriately attribute software, teaching resources or ideas developed by a project like this. Citations of scientific publications associated with this project will be tracked. Efforts will be made to collect and track meaningful metrics of all outputs.
- Lowering the UAS technology adoption barriers to entry for UAS in agriculture and natural resources. Efforts will be made to collect and track meaningful metrics.
- Accelerated use of UAS in research in agriculture and natural resources, resulting in faster progress in the improvement of plants and animals. Efforts will be made to collect and track meaningful metrics.
- Accelerated use of UAS in the practical management of agriculture and natural resources, resulting in more efficient, profitable, and sustainable management. Efforts will be made to collect and track meaningful metrics.
- Improved decision making by researchers, improved profitability and sustainability for growers, catalyzing additional new software, hardware and technologies. Efforts will be made to collect and track meaningful metrics.
Milestones
(2022):Two or more multistate research teams focused on common (use-case inspired), specific, fundable research topics.(2022):Two or more multistate extension teams focused on common, specific, training material topics.
(2022):Completed needs assessment for research based on survey of researchers and practitioners in the field of UAS in agriculture and natural resources.
(2022):Completed needs assessment for formal classroom instruction and extension/outreach training materials based on survey of extension personnel and practitioners in the field of UAS in agriculture and natural resources.
(2023):At least one funded project for collaborative development of extension/outreach training materials from corporate, state, and/or federal sources.
(2023):At least one funded project for collaborative research from corporate, state, and/or federal sources.
(2023):Functional and accessible website for dissemination of project results.
(2024):At least one set of extension/outreach training material to meet the needs elucidated from the needs assessment conducted in earlier years.
(2024):At least three submitted multi-university research proposals to address needs elucidated from the assessment conducted in earlier years.
(2024):At least one customizable course curriculum (up to 3 credits) and pertinent education material/modules development to meet the needs elucidated from the assessment conducted in earlier years.
Projected Participation
View Appendix E: ParticipationOutreach Plan
Objective-specific results will be disseminated via project web page, CRIS reports, peer-reviewed and extension publications. An annual meeting has been and will continue to be held in conjunction with the group. A large proportion of outreach will occur naturally between and through these members, which the S1069 group catalyzes, but some may not be formally recognized as an outcome of S1069. This leads to an output and an outcome gap the group will need to accept. However it is telling that the group continues to grow in numbers of institutions and members, indicating that this form of outreach is a catalyst.
Organization/Governance
The project governance structure will consist of a Chair, Vice Chair, and Secretary, each with a one-year term. These three officers will make up the project Executive Committee. At the end of each term, the Vice Chair will become Chair, the Secretary will become Vice Chair, and a new Secretary will be elected. The end of term will coincide roughly with the annual meeting, such that elections and transitions can be conducted at the end of the annual meeting. It is generally expected that the annual meeting will be hosted by the current Chair, so incoming Secretaries should be advised of this expectation when elected, three years in advance of the time they would serve as host. Project members will decide at the first annual meeting if objective coordinators are needed for each of the three objectives. If so, objective coordinators will be selected at that meeting for at least a two-year term, and will be added to the project Executive Committee.
All project members will meet face-to-face (or virtually face-to-face) at least once per year, either as a stand-alone meeting or in conjunction with an appropriate conference. Members working on individual objectives may have conference calls periodically throughout the year to coordinate activities and the Executive Committee may also schedule a project conference call if needed. Leadership will look for additional partners, including scientific societies and universities to grow and strengthen the group, as well as expand its reach.
Literature Cited
Adak, A., Murray, S. C., Božinović, S., Lindsey, R., Nakasagga, S., Chatterjee, S., ... & Wilde, S. (2021). Temporal Vegetation Indices and Plant Height from Remotely Sensed Imagery Can Predict Grain Yield and Flowering Time Breeding Value in Maize via Machine Learning Regression. Remote Sensing, 13(11), 2141. https://doi.org/10.3390/rs13112141.
Anderson S.L., Murray S.C., Chen Y., Malambo L., Chang A., Popescu S., Cope D., Jung J., 2021. (In Preparation) Unmanned Aerial Systems Enabled Functional Modeling of Maize (Zea mays L.) Height Revealing Dynamic Expression of Loci Associated with Temporal Growth Trajectories.
Anderson S.L., Murray S.C., Malambo L., Ratcliff C., Popescu S., Cope D., Chang A., Jung J., Thomasson J.A., 2019. Prediction of Maize Grain Yield before Maturity Using Improved Temporal Height Estimates of Unmanned Aerial Systems. The Plant Phenome Journal 2:190004. DOI: 10.2135/tppj2019.02.0004.
Anthony D., Elbaum S., Lorenz A., Detweiler C., 2014. On crop height estimation with UAVs, 2014 IEEE/RSJ International Conference on Intelligent Robots and Systems, IEEE. pp. 4805-4812.
Anthony, D., Elbaum, S., Lorenz, A., Detweiler, C., 2014. On crop height estimation with UAVs. Presented at the 2014 IEEE/RSJ International Conference on Intelligent Robots and Systems, IEEE, pp. 4805–4812.
Aquilina, C.A. 2020. Estimating Floodplain Vegetative Roughness using Drone-Based Laser Scanning and Structure from Motion Photogrammetry. MS Thesis, Biological Systems Engineering, Virginia Tech, Blacksburg, VA.
Balota, M., Oakes, J., 2017. UAV remote sensing for phenotyping drought tolerance in peanuts. Presented at the Autonomous air and ground sensing systems for agricultural optimization and phenotyping II, International Society for Optics and Photonics, p. 102180C.
Bendig J., Bolten A., Bennertz S., Broscheit J., Eichfuss S., Bareth G., 2014. Estimating biomass of barley using crop surface models (CSMs) derived from UAV-based RGB imaging. Remote Sensing 6:10395-10412.
Borra-Serrano, I., De Swaef, T., Aper, J., Ghesquiere, A., Mertens, K., Nuyttens, D., Saeys, W., Somers, B., Vangeyte, J., Roldán-Ruiz, I., 2018. Towards an objective evaluation of persistency of Lolium perenne swards using UAV imagery. Euphytica 214, 1–18.
Breda, N.J., 2003. Ground‐based measurements of leaf area index: a review of methods, instruments and current controversies. Journal of experimental botany 54, 2403–2417.
Carter, G.A., 1991. Primary and secondary effects of water content on the spectral reflectance of leaves. American Journal of Botany 78, 916–924.
Certified Crop Advisors, 2020. 2020 Certificant Survey. https://www.certifiedcropadviser.org/files/certifiedcropadviser/boards/2020-icca-survey-report.pdf. Last accessed April 27, 2021.
Chandel, A. K.,Molaei, B., Khot, L. R., Peters,R. T., Stöckle, C. O., Jacoby, P. W., 2021. High-resolution spatiotemporal water use mapping of surface and direct-root-zone drip irrigated grapevines using UAS-based thermal and multispectral remote sensing. Remote Sensing, 13, 954. https://doi.org/10.3390/rs13050954
Chandel, A.K., Khot, L.R., Sallato, B.C., 2020. Towards rapid detection and mapping of powdery mildew in apple orchards. IEEE Xplore, Proceedings of IEEE 2020 International Workshop on Metrology for Agriculture and Forestry, November 4–6, 2020, pp. 268–272.
Chapman, S.C., Merz, T., Chan, A., Jackway, P., Hrabar, S., Dreccer, M.F., Holland, E., Zheng, B., Ling, T.J., Jimenez-Berni, J., 2014. Pheno-copter: a low-altitude, autonomous remote-sensing robotic helicopter for high-throughput field-based phenotyping. Agronomy 4, 279–301.
Chen, J.M., Black, T., 1992. Defining leaf area index for non‐flat leaves. Plant, Cell & Environment 15, 421–429.
Chu T., Starek M., Brewer M., Murray S., Pruter L., 2017. Assessing lodging severity over an experimental maize (Zea mays L.) field using UAS images. Remote Sensing 9:923.
Ciampalini, R., Follain, S., Le Bissonnais, Y., 2012. LandSoil: a model for analysing the impact of erosion on agricultural landscape evolution. Geomorphology 175, 25-37.
Davis, A., 2017. Venture Capital: Drone startups draw big guns. Wall Street Journal. Online at: https://blogs.wsj.com/briefly/2017/03/14/venture-capital-drone-startups-draw-big-guns-at-a-glance/
DroneDeploy, 2021. State of the Drone Industry Report 2021. DroneDeploy, 12 pp.
Engelbrecht, B.M., Tyree, M.T., Kursar, T.A., 2007. Visual assessment of wilting as a measure of leaf water potential and seedling drought survival. Journal of Tropical Ecology 497–500.
Erickson, B., Lowenberg-DeBoer, J., 2020. 2020 Precision Ag Dealership Survey: Moving the Needle on Decision Agriculture, Crop Life Survey.
Evans, K.S., Athearn, K., Chen, X., Bell, K.P., Johnson, T., 2016. Measuring the impact of pollution closures on commercial shellfish harvest: The case of soft-shell clams in Machias Bay, Maine. Ocean & Coastal Management 130, 196–204.
Evett, S.R., Howell, T.A., Schneider, A.D., Upchurch, D.R., Wanjura, D.F., 1996. Canopy temperature based automatic irrigation control. Presented at the Proc. Intl. Conf. Evapotranspiration and Irrigation Scheduling, pp. 207–213.
Fang, H., Wei, S., Liang, S., 2012. Validation of MODIS and CYCLOPES LAI products using global field measurement data. Remote Sensing of Environment 119, 43–54.
FAO, 2018. The State of World Fisheries and Aquaculture 2018-Meeting the sustainable development goals. Fisheries and Aquaculture Department, Food and Agriculture Organization of the United Nations, Rome.
Farfan, I.D.B., Murray, S.C., Labar, S., Pietsch, D., 2013. A multi-environment trial analysis shows slight grain yield improvement in Texas commercial maize. Field Crops Research 149, 167–176.
Giles, D. K. 2019. Remotely piloted aircraft for agricultural spraying: multiple-season results in commercial-scale applications. In Linscott, D.: Pesticide Formulation and Delivery Systems, V. 39, Innovative Formulation, Application and Adjuvant Technologies for Agriculture. West Conshohocken, Pa: ASTM International, https://doi.org/10.1520/STP161920180082.
Guzmán, G., Quinton, J.N., Nearing, M.A., Mabit, L., Gómez, J.A., 2013. Sediment tracers in water erosion studies: Current approaches and challenges. Journal of Soils and Sediments 13, 816-833.
Hamidou, F., Ratnakumar, P., Halilou, O., Mponda, O., Kapewa, T., Monyo, E., Faye, I., Ntare, B., Nigam, S., Upadhyaya, H., 2012. Selection of intermittent drought tolerant lines across years and locations in the reference collection of groundnut (Arachis hypogaea L.). Field Crops Research 126, 189–199.
Han, X., Thomasson, J. A., Wang, T., & Swaminathan, V. (2020c). Autonomous mobile ground control point improves accuracy of agricultural remote sensing through collaboration with UAV. Inventions, 5(1), 12.
Ishimwe, R., Abutaleb, K., Ahmed, F., 2014. Applications of thermal imaging in agriculture - a review. Advances in Remote Sensing 3, 128–140.
Jenkins, D., Vasigh, B., 2013. The economic impact of unmanned aircraft integration in the United States. Association for Unmanned Vehicle Systems International, Arlington, VA, p. 38.
Ju, J. and Roy, D.P., 2008. The availability of cloud-free Landsat ETM+ data over the conterminous United States and globally. Remote Sensing of Environment, 112(3), pp.1196-1211. https://doi.org/10.1016/j.rse.2007.08.011.
Koparan, C., Koc, A.B., Privette, C.V., Sawyer, C.B., 2018. In situ water quality measurements using an unmanned aerial vehicle (UAV) system. Water 10, 264.
Lane, H. M., Murray, S. C., 2021. High throughput can produce better decisions than high accuracy when phenotyping plant populations. Crop Science (in press).
Lane, H. M., Murray, S. C., Montesinos López, O. A., Montesinos López, A., Crossa, J., Rooney, D. K., ... & Morgan, C. L., 2020. Phenomic selection and prediction of maize grain yield from near‐infrared reflectance spectroscopy of kernels. The Plant Phenome Journal, 3(1), e20002.
Luis, J., Ozias-Akins, P., Holbrook, C., Kemerait, R., Snider, J., Liakos, V., 2016. Phenotyping peanut genotypes for drought tolerance. Peanut Science 43, 36.
Ma, L., Gardner, F., Selamat, A., 1992. Estimation of leaf area from leaf and total mass measurements in peanut. Crop science 32, 467–471.
Maimaitijiang, M., Sagan, V., Sidike, P., Daloye, A.M., Erkbol, H. and Fritschi, F.B., 2020. Crop monitoring using satellite/UAV data fusion and machine learning. Remote Sensing, 12(9), p.1357. https://doi.org/10.3390/rs12091357.
Malambo L., Popescu S., Horne D., Pugh N., Rooney W., 2019. Automated detection and measurement of individual sorghum panicles using density-based clustering of terrestrial lidar data. ISPRS journal of photogrammetry and remote sensing 149:1-13.
Milberg, P., Bergstedt, J., Fridman, J., Odell, G., Westerberg, L., 2008. Observer bias and random variation in vegetation monitoring data. Journal of Vegetation Science 19, 633–644.
Montgomery, D.R., 2007. Soil erosion and agricultural sustainability. Proceedings of the National Academy of Sciences 104, 13268-13272.
Muller, E., Decamps, H., 2001. Modeling soil moisture–reflectance. Remote Sensing of Environment 76, 173–180.
Nigam, S., Chandra, S., Sridevi, K.R., Bhukta, M., Reddy, A., Rachaputi, N.R., Wright, G., Reddy, P., Deshmukh, M., Mathur, R., 2005. Efficiency of physiological trait‐based and empirical selection approaches for drought tolerance in groundnut. Annals of Applied Biology 146, 433–439.
NOAA, 2018. 2017 Aquaculture Production Highlights, National Oceanic and Atmospheric Administration Fisheries. Online at: https://www.fisheries.noaa.gov/national/aquaculture/us-aquaculture
Nutter Jr, F.W., Littrell, R.H., 1996. Relationships between defoliation, canopy reflectance and pod yield in the peanut-late leafspot pathosystem. Crop Protection 15, 135–142.
Palmer, S.C., Gernez, P.M., Thomas, Y., Simis, S., Miller, P.I., Glize, P., Barillé, L., 2020. Remote sensing-driven Pacific oyster (Crassostrea gigas) growth modeling to inform offshore aquaculture site selection. Frontiers in Marine Science 6, 802.
Pampolini, L.F., 2020. An Assessment of 2d and 3d Spatial Accuracy of Photogrammetry for Livestock Health Monitoring (M.S. Thesis). University of Kentucky, Lexington, KY.
Parajuli, P., Zhao, Y., Tabler, T., Chesser, G.D., 2019. Evaluating avoidance distance of broilers exposed to aerial automated systems. Presented at the 2019 ASABE Annual International Meeting, American Society of Agricultural and Biological Engineers.
Passioura, J., 2012. Phenotyping for drought tolerance in grain crops: when is it useful to breeders? Functional Plant Biology 39, 851–859.
Prince Czarnecki, J. M., van der Zwaag, J., Ramirez-Avila, J. J., Linhoss, Anna C., Schauwecker, T. J., 2020. Augmented reality as a tool for technology-driven conservation. 75th Soil and Water Conservation Society International Annual Conference, Virtual. Available online: https://bit.ly/Czarnecki_2020SWCS
Prior, E.M., Aquilina, C.A. Czuba, J.A., Pingel, T.J., Hession, W.C., 2020. Estimating floodplain vegetative roughness using drone-based laser scanning and structure from motion photogrammetry. American Geophysical Union (AGU) 2020 Fall Meeting.
Pugh N., Horne D.W., Murray S.C., Carvalho G., Malambo L., Jung J., Chang A., Maeda M., Popescu S., Chu T., 2018. Temporal estimates of crop growth in sorghum and maize breeding enabled by unmanned aerial systems. The Plant Phenome Journal 1.
Pullanagari, R. R., Kereszturi, G., & Yule, I. (2018). Integrating airborne hyperspectral, topographic, and soil data for estimating pasture quality using recursive feature elimination with random forest regression. Remote Sensing, 10(7), 1117.
Rajendran, K., Tester, M., Roy, S.J., 2009. Quantifying the three main components of salinity tolerance in cereals. Plant, Cell & Environment 32, 237–249.
Research and Markets, 2020. The Drone Market Report 2020-2025. Research and Markets, 225 pp.
Resop, J.P., Lehmann, L., Hession, W.C., 2019. Drone laser scanning for modeling riverscape topography and vegetation: Comparison with traditional aerial lidar, Drones, 3(35). doi:10.3390/drones3020035
Reynolds, M., Manes, Y., Izanloo, A., Langridge, P., 2009. Phenotyping approaches for physiological breeding and gene discovery in wheat. Annals of Applied Biology 155, 309–320.
Rincent R., Charpentier J.-P., Faivre-Rampant P., Paux E., Le Gouis J., Bastien C., Segura V., 2018. Phenomic Selection Is a Low-Cost and High-Throughput Method Based on Indirect Predictions: Proof of Concept on Wheat and Poplar. G3: Genes, Genomes, Genetics 8:3961-3972.
Rowland, D.L., Faircloth, W.H., Payton, P., Tissue, D.T., Ferrell, J.A., Sorensen, R.B., Butts, C.L., 2012. Primed acclimation of cultivated peanut (Arachis hypogaea L.) through the use of deficit irrigation timed to crop developmental periods. Agricultural Water Management 113, 85–95.
Sankaran S., Khot L.R., Carter A.H., 2015. Field-based crop phenotyping: Multispectral aerial imaging for evaluation of winter wheat emergence and spring stand. Computers and Electronics in Agriculture 118:372-379.
Shi Y., Thomasson J.A., Murray S.C., Pugh N.A., Rooney W.L., Shafian S., Rajan N., Rouze G., Morgan C.L., Neely H.L. (2016) Unmanned aerial vehicles for high-throughput phenotyping and agronomic research. PloS One 11:e0159781.
Stanton C., Starek M.J., Elliott N., Brewer M., Maeda M.M., Chu T. (2017) Unmanned aircraft system-derived crop height and normalized difference vegetation index metrics for sorghum yield and aphid stress assessment. Journal of Applied Remote Sensing 11:026035.
Temu, V.W.; Hession, W.C., Sforza, P. and Wang, H. In Press. Enhanced Grazing Management Assessment Using Drone-Based Lidar Measurements. Joint International Grassland & Rangeland Congress 2021, October 13-22, Karibu Kenya.
von Bueren, S.K., Burkart, A., Hueni, A., Rascher, U., Tuohy, M.P., Yule, I.J., 2015. Deploying four optical UAV-based sensors over grassland: challenges and limitations. Biogeosciences 12, 163–175.
Walker, D., Kurth, T., Van Wyck, J., Tilney, M., 2016. Lessons from the Frontlines of the Agtech Revolution. bcg.perspectives. Online at: https://www.bcgperspectives.com/content/articles/process-industries-strategy-lessons-frontlines-agtech-revolution/
Wang, L., Yue, X., Wang, H., Ling, K., Liu, Y., Wang, J., Hong, J., Pen, W., Song, H., 2020d. Dynamic Inversion of Inland Aquaculture Water Quality Based on UAVs-WSN Spectral Analysis. Remote Sensing 12, 402.
Wang, T., Thomasson, J. A., Isakeit, T., Yang, C., & Nichols, R. L. (2020b). A Plant-by-Plant Method to Identify and Treat Cotton Root Rot Based on UAV Remote Sensing. Remote Sensing, 12(15), 2453.
Wang, T., Thomasson, J. A., Yang, C., Isakeit, T., Nichols, R. L., Collett, R. M., ... & Bagnall, C. (2020a). Unmanned aerial vehicle remote sensing to delineate cotton root rot. Journal of Applied Remote Sensing, 14(3), 034522.
Watson, D.J., 1947. Comparative physiological studies on the growth of field crops: I. Variation in net assimilation rate and leaf area between species and varieties, and within and between years. Annals of Botany 11, 41–76.
Wiesner-Hanks T., Stewart E.L., Kaczmar N., DeChant C., Wu H., Nelson R.J., Lipson H., Gore M.A., 2018. Image set for deep learning: field images of maize annotated with disease symptoms. BMC research notes 11:440.
Woldt, W., Martin, D., Lahteef, M., Kruger, G., Wright, R., McMechan, J., ... & Jackson-Ziems, T. (2018). Field evaluation of commercially available small unmanned aircraft crop spray systems. In 2018 ASABE Annual International Meeting (p. 1). American Society of Agricultural and Biological Engineers.
Yeom J., Jung J., Chang A., Maeda M., Landivar J., 2018. Automated Open Cotton Boll Detection for Yield Estimation Using Unmanned Aircraft Vehicle (UAV) Data. Remote Sensing 10:1895.
Zhou , J., Khot, L.R., Peters, T. , Whiting, M.D., Zhang, Q., Granatstein, D., 2016. Efficacy of unmanned helicopter in rainwater removal from cherry canopies. Computers and Electronics in Agriculture 124, 161-167.
Zhou, J., Zhou, J., Ye, H., Ali, M.L., Nguyen, H.T., Chen, P., 2020. Classification of soybean leaf wilting due to drought stress using UAV-based imagery. Computers and Electronics in Agriculture 175, 105576.