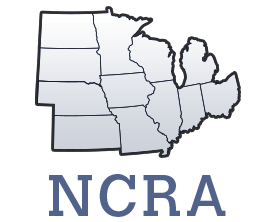
NC1195: Enhancing nitrogen utilization in corn based cropping systems to increase yield, improve profitability and minimize environmental impacts
(Multistate Research Project)
Status: Active
NC1195: Enhancing nitrogen utilization in corn based cropping systems to increase yield, improve profitability and minimize environmental impacts
Duration: 10/01/2021 to 09/30/2026
Administrative Advisor(s):
NIFA Reps:
Non-Technical Summary
Statement of Issues and Justification
Nitrogen, food, and environment
Nitrogen (N) fertilizer is one of the greatest inventions in human history. Smil (2001) estimates that 40% of the current human population would not be alive if the process for turning atmospheric N gas into fertilizer had not been invented. Around half of current global food production can be attributed to the use of N fertilizer (Smil, 2011). Along with its tremendous impact on food production, N fertilizer has potent effects when it escapes to water and air from the agricultural systems where it was applied. For example, plants in coastal waters are, like plants on land, usually N-limited in their growth rate (Vitousek and Howarth, 1991). Coastal waters receiving drainage from intensive agricultural regions (e.g., Gulf of Mexico, Yellow Sea, Baltic Sea) have excessive plant growth that can sometimes be severe enough to lead to dissolved oxygen-depleted as low as to harm marine life (Burkart and James, 1999; Rabalais et al., 2002; Guo et al., 2020; Conley et al., 2011). Nitrogen fertilizer is the main agricultural contributor to global warming potential in the U.S., both through CO2 produced during its manufacture and N2O released after its application to farm fields (USEPA, 2016; Davidson, 2009). Although CO2 from transport and other industries have a much larger global warming impact, the most important reduction advances in agriculture are likely to be from N fertilizer management. In addition, the N2O from agriculture is now the largest human-driven source of compounds that deplete stratospheric ozone (Ravishankara et al., 2009). Thus, N fertilizer is essential to the U.S. and world food supply but has substantial negative impacts on the environment. There is currently great potential to maintain or increase N benefits in food production while reducing the negative environmental consequences. That potential is the main interest of this committee.
Improving nitrogen use in the U.S.
The U.S. was one of the earliest widespread users of N fertilizer following the conversion of munitions (high in N) factories to fertilizer factories after World War II. In the U.S., more N fertilizer is applied to corn than to all other crops combined; however, it is also used on nearly all non-legume crops. A large proportion of the N fertilizer used in the U.S. is for crops fed to animals (especially corn), and most of that N is excreted by those animals as manure. Efficient management of manure N is also important, both to support crop production and in terms of negative consequences in the environment. Increasing N use efficiency (NUE) from fertilizer and manure has been a key driver increasing crop production efficiency in the U.S. (Houlton et al., 2012). However, the manure N source is often not accounted for and applying fertilizer N to crops in amounts than is needed reduces profitability and increases environmental impact and costs (Hong et al., 2007; Shcherbak et al., 2014).
Considering the availability of the various N sources; “How much N fertilizer is needed?”. This turns out to be a difficult question, and one that is of great interest to this research group. Corn production in the U.S. today relies on large inputs of N fertilizer to meet N demand by the crop (Simons et al., 2014), but also soil N supply. Nitrogen mineralization from soil organic matter (SOM), of which manure can greatly increase, provides a substantial portion of corn’s total N need in many areas (Lynch, 2013), and is supplemented as needed with inorganic N fertilizer, manures, and co-products (Yan et al., 2019). Although more total N is needed by corn as yield increases (Ciampitti and Vyn, 2011), more fertilizer N may not be needed (Shapiro and Wortmann, 2006; Scharf et al., 2006b). The amount of N supplied by the tremendous reserves in soil organic matter can vary widely—this is a purely biological process that is very sensitive to both inorganic and organic inputs, soil temperature, moisture, and oxygen (Sierra, 1997; Fernández et al., 2017). The release of N from organic compounds in manure is similarly complex. Complicating the matter further is the impact of temperature, moisture, and oxygen on N loss via leaching, denitrification, and ammonia volatilization (Scharf and Alley, 1988). The result is that even the optimal N fertilization rate can vary widely from place to place even in a single field (Mamo et al., 2003; Scharf et al., 2005). There is still much to learn and predict both spatially and temporally and spatially about these fundamental processes that control plant N availability and, thus, optimal N fertilizer management.
A key component to improved fertilizer N efficiency and reduced environmental impact is a better understanding and quantification of N mineralization from all sources coupled to crop uptake. Fertilizer N efficiency is normally based on N uptake/yield of unfertilized (check) plots. An important, possibly incorrect, assumption in this approach is that release of organic soil N is unaffected by N fertilization (Jenkinson et al., 1985; Mahal et al., 2019). Not accounting for N fertilizer’s impact on soil organic N release can lead to over-fertilization and increased N loss. While it is commonly accepted that N fertilizer influences soil N mineralization by “priming” processes (Jenkinson et al., 1985; Mahal et al., 2019) little has been done to quantify these effects and incorporate this knowledge into our fertilizer N recommendations or calculations of NUE. Thus, quantifying uptake of fertilizer N by the crop and associated changes in soil N mineralization are paramount to developing sound management approaches that maintain high crop yields while minimizing N losses. Thus, methods to predict the best N fertilizer rate that can account for spatially and temporally variable N loss and release of organic N from soil and manure are a key step toward improved N management. This research group has collected data and published three papers addressing this issue (Scharf et al., 2006a; Laboski et al., 2008; McDaniel et al., 2020). Each of these papers benefited from the regional nature of the group, producing conclusions that were much more robust than can be achieved by a few investigators in limited geographies. The relatively recent addition of microbiological and modeling expertise to the committee adds new approaches that can be used in pursuit of this goal.
However, improved understanding of soil N processes only addresses one side of the equation in the larger picture of environmental sustainability under a changing climate. With the adoption of new cropping systems, including more diverse crops grown in rotation with corn as well as the use of cover crops and integrated livestock systems, a more thorough understanding is needed of N cycle processes to maximize NUE, minimize environmental impact, and protect soil and water resources.
Current knowledge of N-cycle processes and their management
Although the biological underpinnings of most N cycle processes are well characterized, less is known about how these processes interact spatially and temporally within the soil to control fate of N. Because soils are spatially heterogeneous and include biological ‘hot spots’ such as the rhizosphere and decomposing plant residues, the interaction of applied N with SOM pools as mediated by the soil microbiome is rarely considered where sufficient labile N is added as fertilizer. This can lead producers to over-apply N fertilizer. Adequate N supply is required to achieve producer acceptable economic outcomes. Because the relationship between yield and N uptake is usually tightly conserved, achieving ever-higher yields often depends on greater N uptake, which in turn requires greater amounts of available N. However, overapplication of N fertilizer to ensure consistent crop yields has led to decreased NUE (Tilman et al., 2002). Excessive N fertilizer use threatens environmental quality and human health. Emission of greenhouse gases, specifically N2O, is also attributed to inefficient use of N fertilizers. Fertilizer N also typically accounts for approximately 50% of the fossil energy input into intensively managed crops like corn. Environmental degradation and rising energy costs have become major impediments to both the profitability and sustainability of intensively managed cropping systems.
Climate change science suggests a slight increase in overall precipitation in the U.S. Corn Belt, with a significant increase in intensity and frequency of large rainfall events in the spring/early summer corn growing season (Kellner and Niyogi, 2015). This pattern is consistent with weather events over the past several years. These changes in precipitation patterns will drive increased loss of both mineralized and fertilizer N from soils, via denitrification and leaching (Bowles et al., 2018). Loss directly from soils via denitrification, and in-stream denitrification of nitrate leached to surface waters, will also increase emissions of N2O. Therefore, one potential indirect consequence of precipitation changes and N loss could be increased N-based greenhouse gas production. Mitigation of these losses requires improved understanding of N release from soil organic N pools to provide better N rate recommendations which can account for variation in N mineralization between years; improved N management practices to reduce N loss and better synchronize soil N availability with crop N demand; and an increase in NUE. Implementation of more diverse crop rotations, cover crops, application of biosolids (manures, biochars etc.) to address climate variability will necessitate updated management practices including N recommendations, timing and placement of fertilizer N, selection of N source and additives to reduced nitrate formation and loss to better synchronize N supply with crop demand. Improved N management practices may also include using crop sensors or other decision tools to guide in-season application, and fertilizer products and additives that reduce loss; all of these practices will aid producers in adapting to climate change, provide environmental benefits, improve crop yields, and give a better economic return to N.
Regional project goals
The long-term goals of this regional project are to better understand how the interactions of soil, weather, climate, and cropping system influence N availability and optimal N management from all N containing inputs. Additionally, we plan to develop tools that help farmers translate this understanding into practice. Over the next five years, we aim to develop one key new piece of knowledge and/or one new management tool that moves N management toward reduced environmental impact while maintaining production benefits. The ultimate success of the project – reduced N loss, efficient N fertilizer use and continued increase in crop yield - lies in grower adoption of N recommendations and management practices developed. This will require a thorough understanding of how practices within a cropping system impact N availability and yield, understanding the producer and adviser decision making process, and development/enhancement of decision tools that will inform N fertilization decisions. Thus, a strong, transformative extension education/outreach program targeted to producers and crop advisors (in conjunction with extension educators, local/state/federal regulatory personnel, and policymakers), is central to this project.
Related, Current and Previous Work
Management practices and optimum nitrogen use
For the past few decades, agronomists and soil scientists have made recommendations to farmers for N additions that include rate, source, timing, and placement for optimum economic returns and environmental protection. Nitrogen rate recommendations are often based on expected crop yield multiplied by a N fertilizer factor, which is usually a regionally-specific quantity of N fertilizer required per unit yield (Morris et al., 2018). In several Midwestern states, N rate recommendations are now based on empirical data relating economic returns to N fertilizer rate averaged over a large number of site-years within a particular region (MRTN approach, Sawyer et al., 2006).
Recent research has focused on understanding how environmental factors influence the economic optimum N rate (EONR) so that they may be integrated into recommendation systems to make N applications more precise, particularly in the face of environmental change. For example, Tremblay et al. (2012) evaluated corn N response as impacted by soil texture and weather, finding that corn response to added N was greater in fine-textured than medium-textured soils and that abundant and well-distributed rainfall increased the N response. Puntel et al. (2019) evaluated the role of 54 static and dynamic variables on the EONR, and found that soil depth, number of rainy days, residue amount, soil nitrate at planting, and heat stress around silking were the most valuable predictors of the EONR.
A number of innovative tools have recently been developed to rapidly assess crop N need and make fertilizer N recommendations. Sensors or remote sensing of crop N needs are the current frontier. For example, in-situ soil and plant sensors, rapid leaf chlorophyll measurements, and canopy spectral reflectance measurements from proximal or remote sensors can be used to detect actual in-season soil or crop N status as well as capture spatial variability. Soil and plant tissue tests have been used for decades, but improvements are rapidly being made for these as well. New soil health tests are being developed to measure ‘N-supplying power’, a soil ecosystem service directly that has shown a promising relationship to EONR (Yost et al., 2018; Wade et al., 2020, Franzluebbers, 2018). For example, the extraction protocol developed for “glomalin” has been recognized to more broadly reflect soil protein from a variety of sources and can be used as an indicator of potentially available N (Hurisso et al., 2018). Permanganate oxidizable C and mineralizable C have also been proposed as indicators of biological N cycling processes (Culman et al., 2012). Another approach is using multiple tests, or even advanced data analytics (like machine learning), to determine what test(s) best predict EONR. Members of this committee have explored (Yost et al., 2018), and are also currently further exploring, the potential of mineralizable C (i.e. CO2 Burst) to predict corn EONR. Using a dataset generated by this multi-state committee, McDaniel et al. (2020) evaluated 30 chemical and biological soil tests, along with environmental and management data, to predict maize response to N fertilizer in the Midwest U.S. The authors found that a 14-day aerobic N mineralization assay combined with tetraphenyl borate extraction (extraction accounting for fixed NH4-N) increased predictability of non-responsive sites compared to the traditional pre-sidedress nitrate test. Our multi-state committee member Li is now expanding on the large dataset from this study to publish an economic analysis.
Members of this multi-state committee have been and will continue to be involved in important evaluations of traditional and innovative N management approaches. Some research shows that site- and season-specific data or model simulations of soil and crop N status can increase accuracy of EONR predictions relative to static recommendations or grower selections, thereby minimizing unnecessary N applications (Scharf et al., 2011; Sela et al., 2018). On the other hand, Ransom et al. (2020) showed that the relatively static MRTN approach was among the best decision tools for predicting the EONR, and performed similarly to in-season soil tests and crop sensing. More research is needed to identify where dynamic N management approaches are profitable and how to increase their reliability and reduce their costs.
Increasingly, soil scientists and agronomists recognize that precise fertilizer management is only part of the solution to reducing N losses from agroecosystems (Bowles et al., 2018). This is captured currently in mainstream agronomy as the ‘4R-Plus’, meaning there are management practices beyond fertilizer that can increase NUE in agroecosystems. Several recent studies have investigated the effects of some of these innovative soil and cropping system management practices on EONR, and their interaction with the environment. For example, Castellano et al. (2019) used model simulations to show that the expansion and intensification of artificial drainage as an adaptation to climate change may reduce the EONR by increasing N mineralization, decreasing denitrification, and increasing crop NUE. The authors hypothesized that artificial drainage would make cropping systems more compatible with cover crops, a proven tool for reducing N losses (Thapa et al., 2018). Two members of our multistate committee - Castellano and Fernández - are now performing studies to evaluate the effect of innovative artificial drainage systems and combinations of drainage and cover crops, on EONR. Recently, members of our committee begun to investigate how cover crops affect soil N cycling, crop N demand, and optimum N fertilization. For example, a study led by Sawyer found that a rye cover crop marginally reduced corn yield but did not affect the EONR relative to no cover crop (Pantoja et al., 2015). Jagadamma and Poffenbarger are also performing research to understand how legume and non-legume cover crops influence the EONR of the subsequent crop, and how cover crops may differentially affect N cycling depending on topographic position.
Interaction of soil biological processes, plant available nitrogen, and soil health
The prediction of plant available N (PAN) from organic sources, green cover crops and reservoirs in soil organic matter has been an ongoing challenge due to the inconsistent turnover of these soil pools (Wade et al., 2016; Miller and Geisseler, 2018; Sullivan et al., 2020). Ideally, we need to bridge the gap between research and practical solutions if ecological intensification strategies are to be accepted by farmers (Kleijn et al., 2019; Li et al., 2019). New focus on soil health and interactions with N availability are of current interest for both practical (applied) and fundamental research into the relationship between the soil microbiome, soil organic matter and PAN by members of this multi-state committee.
Crop plants primarily assimilate N as nitrate and ammonium and these are often assumed to comprise the majority of PAN but this ignores the important role of organic N turnover. Mineralization is the general term for the conversion of organic N to inorganic N as ammonium and nitrate, ammonification is the conversion of organic N to the ammonium form, while nitrification is the oxidation of reduced N (ammonium or organic N) to nitrate. Microbial assimilation or immobilization converts inorganic N back to organic N as microbial biomass (part of soil organic N). The soil internal N cycle often changes the less mobile organic N and ammonium forms to nitrate which is mobile and subject to loss from the plant-soil system. Predictions of the soil’s capacity to supply PAN remain difficult but will likely require a combination of soil carbon and N pools by chemical methods with biologically based testing (Wade et al., 2016, McDaniels et al., 2020).
Our ability to manage N in agroecosystems requires that we manage and predict the fertilizer and manure applied, the mineralization of organic N from cover crops, manures and soil organic N (SON), and inorganic N stored in the soil profile. Characterization of these complex soil N resources and predicting their dynamic process rates is one critical step towards managing for improved NUE and the prevention of N loss from agroecosystems under conventional or organic management. Assessment and prediction of plant available N in agricultural soils may involve soil testing, crop and field history and interpretations from yield response curves (Ransom et al., 2020; Sawyer et al., 2006). Studies undertaken by group members include PAN assessment using 15N-labeled fertilizer combined with cover crops and or 15N pool dilution experiments under contrasting N management systems. The effects of N management on microbial communities and their activities have been addressed through functional metagenomics and community structural equation modeling (Ouyang et al., 2018; Ouyang and Norton, 2020; Chen et al., 2020).
Best practices for crop productivity will support biodiversity, facilitate nutrient cycling, promote soil fertility from multiple N sources, and contribute to soil health, and promote agroecosystem resiliency (Pretty et al., 2010; Smith et al., 2015). One avenue that holds great and largely untapped potential for supporting plant productivity while minimizing environmental impact is characterization of soil and crop-associated microbiomes (Bhowmik et al., 2017; Dignac et al., 2017; Finn et al., 2017). Management of the soil microbiome to control the timing and amount of N mineralization from soil organic matter; however, is not a simple matter given the complexity of habitats (e.g. rhizosphere, soil aggregates, plant residue hot-spots, faunal fecal material) and added N interactions that are controlled by microbial activity, their access to nutrient pools of varying bioavailability, their bioactivity in light of abiotic (e.g. temp., pH, water) and biotic stressors, and ultimately N demand by the growing crop. Thus, the soil microbiome spans a myriad of physiological states across spatial and temporal scales making predictive models of in-season PAN difficult.
Microbe-mediated PAN can be either direct via symbiotic associations with mycorrhizal fungi and N2-fixing bacteria or indirect through microbial-mediated mineralization of organic N. Both are driven by microbial C demand, albeit from differing sources. Current approaches to simplify this system often focus on operationally defined delineations of ‘rhizosphere’ vs ‘bulk soil’ to characterize the communities therein, their functions and how soil-crop management can impact microbe-mediated PAN. Characterizing the influence of management history on initial recruitment and assembly of the maize rhizobiome (our work) will contribute to improved recommendations for indirect management of soil microbiomes (e.g. fertilization, cover crops or crop rotation regimes) and/or the identification of potential targets for direct manipulation of the maize rhizobiome (e.g. biocontrol agents or biostimulants). Furthermore, plant breeding efforts may be able to take advantage of information about the processes of exclusion and selective colonization in the rhizosphere in order to optimize crops for given management histories. In-season N management also impacts beneficial plant symbioses important to N supply. For example, N-fertilization of soybean may decrease symbiotic N fixation (Friel and Friesen, 2019) while N-fertilization of maize depresses extramatrical mycorrhizal biomass in soil (Jeske et al., 2018), an important conduit for plant N and P uptake as well as the formation of soil organic matter (Frey, 2019). Thus, better understanding of the impact of management practices on the structure and function of soil and crop-associated microbial communities, as well as the elucidation of ecological processes mediated by plant-soil-microbe interactions may provide novel solutions to improving PAN supply and NUE.
Decision-making tools and educational resources
Successful translation of N research into reliable decision tools for farmers will improve agroecosystem resiliency and allow for economic and environmental sustainability. It is widely recognized that managing N fertilizer uniformly in all fields (or even all parts of one field) is not the most effective method. However, creating accurate corn N recommendations for individual fields, or within fields, is difficult because of the complexity of the N cycle, weather, variability in both static and dynamic soil properties across a field, and the various interactions among these factors. Many difficult-to-predict factors affect plant N needs and internal use efficiency; such as soils, weather, irrigation, crop variety, previous crop, N timing, landscapes, other nutrients, and interactions of these factors. As a result, few farmers vary N rate across different fields on their farm, and varying rates within a field is even less common. Many committee members have a strong interest in developing evidence-based N decision-making tools that can be used by farmers and their agronomists, and it has been a historical strength of this committee for decades. There is great potential to both leverage existing data and data from ongoing projects of participants in an effort to develop new decision tools or, potentially, to improve the old tools.
Commonly used N recommendation tools are based on soil and plant tests, and yield response trials. The committee has evaluated various soil tests designed to assess potentially mineralizable N. Laboski et al. (2008) determined that the Illinois soil N test was not predictive of corn N need. More recently, two separate studies concluded that a combination of biological and chemical soil tests may improve N recommendations (McDaniel et al., 2020; Clark et al., 2020).
A different strategy to improve corn N recommendations is to use the plant as an integrator of soil N processes and dynamics. Nitrogen sufficiency can be assessed using the crop’s chlorophyll status or light reflectance. An earlier committee (NC-218) found that a hand-held chlorophyll meter was a good predictor of optimal N fertilizer rate; whereas many soil tests performed poorly (Scharf et al., 2006a). Subsequent research provided independent confirmation of the predictive relationship between meter readings and optimal fertilizer rate (Hawkins et al., 2007). A weakness of the hand-held meters is that they are not well-suited to help farmers manage the high levels of spatial variability in N status and dynamics that are found in their fields (Mamo et. al., 2003; Scharf et al., 2005). Canopy reflectance sensors apply the same concept but are more useful as decision tools because measurements are automated and continuous as a fertilizer applicator is driven through a field. Committee members have contributed to a number of important developments with using sensors as decision tools (Khanal et al., 2018; Li et al., 2016; Pena-Yewtukhiw et al., 2015; Raper et al., 2013; Barker and Sawyer, 2012; Scharf et al., 2011), including the recognition that sensor algorithms can be improved using static soil properties and weather information (Bean et al., 2018).
Several committee members were instrumental in developing the Maximum Return to Nitrogen (MRTN) recommendation system which is based on accumulated N response data from each state or region within a state (Sawyer et al., 2006). A major advantage of MRTN is that it easily allows inclusion of new research and enables the inclusion of price and cost information in optimal N calculation. The method is widely used by Land Grant Universities in the Midwest Corn Belt.
In recent years, computer simulation models and weather databases have been gaining popularity in building N decision tools. Some private companies have also shown interest in this effort (such as Monsanto’s FieldView Pro, Granular Agronomy, Adapt-N, etc.). The committee is interested in this area, too. We have had several discussions regarding the possibility of evaluating these models relative to other decision tools, and several committee members have already undertaken such evaluations (Laboski et al., 2014). Also, committee members are interested in development of such tools. These simulation models seek to mathematically describe N and water behavior in soils in real-time to predict availability of N to crops. In a similar vein, committee members have also contributed to the greater understanding of how soil properties and weather affect optimal N rate decisions through their effects on N mineralization and N loss (Tremblay et al., 2012).
A limitation of the numerous, current N recommendation systems is the inaccuracy in predicting N rates for individual corn fields (Morris et al., 2018). While spatial and temporal variability in optimal N rate is widely recognized, there currently are few reliable approaches to incorporate such variability (primarily caused by environment and management conditions) into field-specific rate guidelines. The MRTN approach uses past field-based N response data from many sites and years to estimate N need on a state or within state regional basis (Morris et al., 2018; Sawyer et al., 2006). However, MRTN does not incorporate real-time soil or weather conditions. Some states have MRTN recommendations that vary based on static soil properties and/or region within a state (Laboski and Peters, 2012; Sawyer et al., 2020). For canopy reflectance sensor-based and soil test-based N recommendation tools, many parameters were not calibrated for specific soil properties or environmental conditions but approximated by general average values. Therefore, refinement of N recommendations on an individual field (or subfield) basis is a critical research need. The committee is interested in exploring methods to improve the generalized N recommendations for individual fields (or subfields) by incorporating more field-specific information such as static and dynamic soil properties and management history. Several committee members have conducted research evaluating how soil and weather information can be incorporated into corn N recommendations (Ransom et al., 2020; Clark et al., 2019; Bean et al., 2018).
Another topic that has not been extensively studied, but is drawing more research attention, is the economic performances of different N decision tools. Previously, the performance of N recommendation tools was often measured by the correlation of predicted N rates and EONR. However, that assessment metric does not directly evaluate the subsequent profitability improvements by the predicted N rates. In particular, all costs associated with use of those tools should be considered in the profitability evaluations. Nor does this assessment directly assess environmental outcomes associated with use of a tool. Recently, some committee members conducted an economic performance evaluation of 31 N recommendation tools using field N response data in eight states (Ransom et al., 2020). They found that, although only 10 tools, primarily ones using soil nitrate tests, predicted N rates that were weakly correlated to EONR, almost all tools, except the Maize-N crop growth model, had similar economic returns. That finding suggests the correlation metric and profitability metric of N decision tool evaluation are not necessarily the same. Additional effort should be placed on evaluating the overall profitability of N decision tools relative to other factors that influence growers use of a tool, such as convenience and simplicity. Assessment of a tool’s robustness to predict profitability under a wide array of soil properties and environmental conditions would also be advantageous.
Objectives
-
Determine the roles of innovative management practices, the environment, and their interactions on optimum use of nitrogen in agroecosystems.
-
Understand the role of soil biological processes in controlling plant available nitrogen and their relationships with soil health and system resilience.
-
Translate field and laboratory research into nitrogen management decision-making tools and educational resources promoting improved profitability and sustainability of corn-based cropping systems.
Methods
We will use field studies that evaluate how corn responds to N inputs in different edaphoclimatic settings and innovative management systems, such as cover crop-based systems and alternative drainage designs. Our methods will also include soil characterization, including soil health testing. To understand environment by management interactions, we will likely use traditional statistical approaches as well as spatial statistics and perhaps multivariate statistics as well.
We will use a wide array of methods to assess soil microbial biomass, activity, community structure and diversity from soil samples collected during field, greenhouse and laboratory experiments. Methods to include soil microbial biomass by chloroform extraction and fatty acid based lipid approaches, e.g. fatty acid methyl esters (FAMEs), gas fluxes for CO2, N2O and CH4, soil extracellular enzyme activities, and molecular based approaches for microbial community composition, estimates of diversity, and functional genes (e.g. N-cycling). Field and laboratory studies undertaken by group members will include plant available N assessment using 15N labeled fertilizer combined with cover crops and or 15N pool dilution experiments under contrasting N management systems. The effects of N management on microbial communities and their activities will be addressed through functional metagenomics and community structural equation modeling.
We will develop new N recommendation algorithms to incorporate soil properties, weather information, and other field data collected into the existing N recommendation tools with the goal of improving farm profitability and sustainability. One strategy is to combine the existing trial data the committee members already have, and match with location, soil, weather, and management factors of the trial fields to build a large meta-dataset. Multivariate regression approach will be employed to fit the meta-data and identify the models that statistically best explain the field-level N responses. The ex ante field-specific N recommendations will then be predicted using available field information. Another newer strategy is to build a comprehensive multi-year, multi-location response trial dataset that consistently collects the yield-impacting factors for each trial plot. That new dataset can be developed as part of trial designs of Objectives 1 and 2, where the impacting factors are suggested by the strategy one modeling results. This large plot-level primary dataset will enable the fitting of more advanced varying coefficient regression models, both parametrically and non-parametrically, to better explain the spatial and temporal variability in N responses. More accurate N rates can be recommended based on the estimated varying N responses.
Educational materials such as extension bulletins and videos will be developed to educate farmers and their crop advisors about corn N decision tools.
Measurement of Progress and Results
Outputs
- Refereed scientific papers are the primary output. The committee has a good history in this regarding nearly all of the papers in the Literature Cited section are important recent contributions from the committee. Comments:
- Presentations to professional scientific and non-scientific audiences are another important output. Committee members have been highly active in this area.
- Written communications aimed at non-scientific audiences is a third important output, helping to increase understanding and awareness of N dynamics and management.
- Development and refinement of decision tools is critical output area. The tools can be put in the hands of farmers and farm advisors to help them improve the management of N.
- Development of educational tools for traditional and non-traditional audiences
Outcomes or Projected Impacts
- Scientific papers and presentations from the committee will substantially improve scientific understanding related to one or more project objectives.
- Farmers and farm advisors will have a better understanding of N dynamics and management due to committee research and outreach.
- Nitrogen management practices will improve in some agricultural sectors due to increased understanding, or due to the development or refinement of N decision tools.
Milestones
(2022):Organize into interest sub-groups to plan either 1) grant proposals, 2) organizing and mining existing databases, 3) economic analysis, and 4) N recommendation tool enhancement/development.(2023):Joint studies initiated here or earlier based on successful proposals (proposals may continue, but probably only studies initiated by 2023 will be completed during the life of the project.
(2024):Synthesis of data for publication from groups producing research results, mining existing long-term data set, or enhancing recommendation tools.
(2025):Extension promotion of increased understanding of soil N mineralization systems, N sensing systems, modelling and incorporation into N recommendation tool enhancements.
(2026):Successful scientific publication from efforts in new research and database analyses.
Projected Participation
View Appendix E: ParticipationOutreach Plan
Several committee members (Fernández, Laboski, Mengel, Sawyer, Scharf, and Steinke) have formal Extension appointments and are regularly engaged in outreach as an important part of their job duties. Other committee members participate in ad hoc Extension/Outreach activities. The members with formal Extension appointments will take the lead in outreach activities, particularly with outcomes that are of importance to farmers and farm advisors. Decision tools are expected to fall into this category. Face-to-face meetings, field days, newsletters, farm press, radio interviews, webinars, and individual websites are all likely to be used as outreach media.
Organization/Governance
The voting membership of the Technical Committee will consist of the official representative from each participating AES or cooperating agency group. The Administrative Advisor and NIFA representative will serve as non-voting, ex-officio members of the committee. The participation of additional interested people from member AES and agencies is encouraged. Membership in the Technical Committee is not limited to states within the North Central Region.
The Executive Committee of the technical committee will consist of a Chairperson, Secretary, and committee Member-at-Large. Members of the Executive Committee must be official members of the Technical Committee. Each year the Technical Committee will elect a new Member-at-Large for a three-year leadership term. In year two the member-at-large will automatically move up to secretary, and in year three will become Chair. At the discretion of the chair, the Administrative Advisor and other additional members can be designated as ex-officio members of the Executive Committee.
The chair sets the agenda and presides at the annual meeting, and any other meetings as deemed necessary. The current chair is responsible for preparing the annual report and seeing it is posted on the NIMSS website (www.nimss.org). In addition, the current chair is responsible for preparation of any documents required for the mid-term review, preparation and up-loading of the five-year project proposal, and appointing working committees as needed.
The secretary will keep the official minutes of all meetings of the Technical Committee and see that they are uploaded to the official NCRA project website in a timely manner. They will preside over any meetings the chair is unable to attend and will become chair of the Technical Committee in the event that the elected committee chair is for any reason unable to continue in this capacity.
The Member-at-Large is responsible for all local arrangements for meetings of the committee. They will also assume the duties of the secretary in the event that the secretary is unable to attend a meeting, or is required to assume the responsibilities of the chair.
The Executive Committee will review and make recommendations concerning the conduct of business by the technical Committee. The Technical Committee will have a regularly scheduled annual meeting. In addition, the Chair may call other meetings of the Technical Committee and the Administrative Adviser as deemed necessary.
Literature Cited
Barker, D.W., and J.E. Sawyer. 2012. Using active canopy sensing to adjust nitrogen application rate in corn. Agron. J. 104: 926-933.
Bean, G.M., N. Kitchen, J.J. Camberato, R.B. Ferguson, F.G. Fernández, D.W. Franzen, C.A.M. Laboski, E.D. Nafziger, J.E. Sawyer, P.C. Scharf, J.S. Schepers, and J.F. Shanahan. 2018. Improving an active-optical reflectance sensor algorithm using soil and weather information. Agron. J. 110: 2541-2551.
Bhowmik, A., M. Cloutier, E. Ball, and M. Ann Bruns. 2017. Underexplored microbial metabolisms for enhanced nutrient recycling in agricultural soils. AIMS Microbiology, 3(4): 826–845.
Bowles, T.M., S.S. Atallah, E.E. Campbell, A.C.M. Gaudin, W.R. Wieder, and A.S. Grandy. 2018. Addressing agricultural nitrogen losses in a changing climate. Nat. Sustain. 1: 399–408.
Burkart, M.R., and D.E. James. 1999. Agricultural nitrogen contribution to hypoxia in the Gulf of Mexico. J. Environ. Qual. 28: 850–859.
Castellano, M.J., S.V. Archontoulis, M.J. Helmers, H.J. Poffenbarger, and J. Six. 2019. Sustainable intensification of agricultural drainage. Nat. Sustain. 2019 210 2: 914–921.
Chen, M., Y. Huang, C. Wang, and H. Gao. 2020. The conversion of organic nitrogen by functional bacteria determines the end-result of ammonia in compost. Bioresour. Technol. 299: 122599.
Ciampitti, I.A. and T.J. Vyn. 2011. A comprehensive study of plant density consequences on nitrogen uptake dynamics of maize plants from vegetative to reproductive stages. Field Crops Res. 121: 2–18.
Clark, J.D., K.S. Veum, F.G. Fernandez, J.J. Camberato, P.R. Carter, R.B. Ferguson, D.W. Franzen, D.E. Kaiser, N.R. Kitchen, C.A.M. Laboski, E.D. Nafziger, C.J. Rosen, J.E. Sawyer, J.F. Shanahan. 2019. United States Midwest soil and weather conditions influence anaerobic potentially mineralizable nitrogen. Soil Sci. Am. J. 83: 1137-1147.
Clark, J.D., F.G. Fernandez, K.S. Veum, J.J. Camberato, P.R. Carter, R.B. Ferguson, D.W. Franzen, D.E. Kaiser, N.R. Kitchen, C.A.M. Laboski, E.D. Nafziger, C.J. Rosen, J.E. Sawyer, J.F. Shanahan. 2020. Soil-nitrogen, potentially mineralizable-nitrogen, and field condition information marginally improves corn nitrogen management. Agron. J. 112: 4332-4343.
Conley D.J., J. Carstensen, J. Aigars, P. Axe, E. Bosdorff, T. Eremina, B-M. Haahti, C. Humborg, P. Jonsson, J. Kotta, C. Lännegren, U. Larsson, J. Walve, S. Wilhelms, and L. Zillén. 2011. Hypoxia is increasing in the coastal zone of the Baltic Sea. Environ. Sci. Technol. 45: 6777–6783.
Culman, S.W., S.S. Snapp, M.A. Freeman, M.E. Schipanski, J. Beniston, R. Lal, L.E. Drinkwater, A.J. Franzluebbers, J.D. Glover, A.S. Grandy, and J. Lee. 2012. Permanganate oxidizable carbon reflects a processed soil fraction that is sensitive to management. Soil Sci. Soc. Am. J. 76: 494– 504.
Davidson, E.A. 2009. The contribution of manure and fertilizer nitrogen to atmospheric nitrous oxide since 1860. Nat. Geosci. 2: 659-662.
Dignac, M. F., D. Derrien, P. Barré, S. Barot, L. Cécillon, C. Chenu, T. Chevallier, G.T. Freschet, P. Garnier, B. Guenet, M. Hedde, K. Klumpp, G. Lashermes, P.A. Maron, N. Nunan, C. Roumet, and I. Basile-Doelsch. 2017. Increasing soil carbon storage: mechanisms, effects of agricultural practices and proxies. A review. Agron. Sustain. Dev. 37: 14.
Finn, D., P.M. Kopittke, P.G. Dennis, and R.C. Dalal. 2017. Microbial energy and matter transformation in agricultural soils. Soil Biol. Biochem. 111: 176–192.
Franzluebbers, A.J. 2018. Soil‐test biological activity with the flush of CO2: III. Corn yield responses to applied nitrogen. Soil Sci. Soc. Am. J. 82: 708-721.
Frey, S.D. 2019. Mycorrhizal fungi as mediators of soil organic matter dynamics. Annu. Rev. Ecol. Evol. Syst. 50: 237-259.
Friel, C.A., and M.L. Friesen. 2019. Legumes modulate allocation to rhizobial nitrogen fixation in Response to factorial light and nitrogen manipulation. Front. Plant Sci. 10: 1316.
Guo, J., H. Yuan, J. Song, X. Li, and L. Duan. 2020. Hypoxia, acidification and nutrient accumulation in the Yellow Sea Cold Water of the South Yellow Sea. Sci. Total Environ. 745: 141050.
Hawkins, J.A., J.E. Sawyer, D.W. Barker, and J.P. Lundvall. 2007. Using relative chlorophyll meter values to determine nitrogen application rates for corn. Agron. J. 99:1034-1040.
Hong, N., P.C. Scharf, J.G. Davis, N.R. Kitchen, and K.A. Sudduth. 2007. Economically optimal nitrogen rate reduces residual soil nitrate. J. Environ. Qual. 36: 354-362.
Houlton, B.Z., E. Boyer, A. Finzi, J. Galloway, A. Leach, D. Liptzin, J Melillo, T.S. Rosenstock, D. Sobota, and A.R. Townsend. 2012. Intentional versus unintentional nitrogen use in the United States: trends, efficiency and implications. Biogeochem. 114: 11-23.
Hurisso, T.T., D.J. Moebius-clune, S.W., Culman, B.N. Moebius-clune, J.E. Thies, and H.M. van Es. 2018. Soil protein as a rapid soil health indicator of potentially available organic nitrogen. Agric. Environ. Lett. 1–5.
Jenkinson, D.S., R.H. Fox, and J.H. Rayner. 1985. Interactions between fertilizer nitrogen and soil nitrogen - the so-called ‘priming’ effect. J. Soil Sci. 36: 425-444.
Jeske, E.S., H. Tian, K. Hanford, D.T. Walters, and R.A. Drijber. 2018. Long-term nitrogen fertilization reduces extraradical biomass of arbuscular mycorrhizae in a maize (Zea mays L.) cropping system. Agric. Ecosyst. Environ. 255: 111-118.
Kellner, O., and D. Niyogi. 2015. Climate variability and the US Corn Belt: ENSO and AO episode-dependent hydroclimatic feedbacks to corn production at regional and local scales. Earth Interact. 19: 1-32.
Khanal, S., J. Fulton, N. Douridas, A. Klopfenstein, and S. Shearer. 2018. Integrating aerial images for in-season nitrogen management in a corn field. Comput. Electron. Agric., 148: 121-131.
Kleijn, D., R. Bommarco, T.P.M. Fijen, L.A. Garibaldi, S.G. Potts, and W.H. van der Putten. 2019. Ecological intensification: bridging the gap between science and practice. Trends Ecol. Evol. 34: 154-166.
Laboski, C.A.M., and J.B. Peters. 2012. Nutrient application guidelines for field, vegetable, and fruit crops in Wisconsin. UWEX Publ. A2809. p 88.
Laboski, C.A.M., J.E. Sawyer, D.T. Walters, L.G. Bundy, R.G. Hoeft, G.W. Randall, and T.W. Andraski. 2008. Evaluation of the Illinois soil nitrogen test in the North Central region of the United States. Agron. J. 100: 1070-1076.
Laboski, C.A.M., J.J. Camberato, and J.E. Sawyer. 2014. Evaluation of Adapt-N in the corn belt. Proc. North Central Extension-Industry Soil Fertility Conf. 30: 7-14.
Li, A., B.D. Duval, R. Anex, P. Scharf, J.M. Ashtekar, P.R. Owens, and C. Ellis. 2016. A case study of environmental benefits of sensor‐based nitrogen application in corn. J. Environ. Qual. 45: 675-683.
Li, M., C.A. Peterson, N.E. Tautges, K.M. Scow, and A.C.M. Gaudin. 2019. Yields and resilience outcomes of organic, cover crop, and conventional practices in a Mediterranean climate. Sci. Rep. 9: 12283.
Lynch, J.P. 2013. Steep, cheap and deep: an ideotype to optimize water and N acquisition by maize root systems. Ann. Bot. 112: 347-357.
Mahal, N.K., W.R. Osterholz, F.E. Miguez, H.J. Poffenbarger, J.E. Sawyer, D.C. Olk, S.V. Archontoulis, and M.J. Castellano. 2019. Nitrogen fertilizer suppresses mineralization of soil organic matter in maize agroecosystems. Front. Ecol. Evol. 7: 59.
Mamo, M., G.L. Malzer, D.J. Mulla, D.R. Huggins, and J. Strock. 2003. Spatial and temporal variation in economically optimum nitrogen rate for corn. Agron. J. 95: 958-964.
McDaniel, M.D., D.T. Walters, L.G. Bundy, X. Li, R.A. Drijber, J.E. Sawyer, M.J. Castellano, C.A.M. Laboski, P.C. Scharf, and W.R. Horwath. 2020. Combination of biological and chemical soil tests best predict maize nitrogen response. Agron. J. 2020: 1–16.
Miller, K.S., and Geisseler, D. 2018. Temperature sensitivity of nitrogen mineralization in agricultural soils. Biol. Fertil. Soils 54(7): 853-860.
Morris, T.F., T.S. Murrell, D.B. Beegle, J.J. Camberato, R.B. Ferguson, J. Grove, Q. Ketterings, P.M. Kyveryga, C.A.M. Laboski, J.M. McGrath, J.J. Meisinger, J. Melkonian, B. Moebius-Clune, E.D. Nafziger, D. Osmond, J.E. Sawyer, P.C. Scharf, W. Smith, J.T. Spargo, H.M. van Es, and H. Yang. 2018. Strengths and limitations of nitrogen rate recommendations for corn and opportunities for improvement. Agron. J. 110: 1–137.
Ouyang, Y., and J.M. Norton. 2020. Short-term nitrogen fertilization affects microbial community composition and nitrogen mineralization function in an agricultural soil. Appl. Environ. Microbiol. 86: e02278-19.
Ouyang, Y., J.R. Reeve, and J.M. Norton. 2018. Soil enzyme activities and abundance of microbial functional genes involved in nitrogen transformations in an organic farming system. Biol. Fertil. Soils 54: 437-450.
Pantoja, J.L., K.P. Woli, J.E. Sawyer, and D.W. Barker. 2015. Corn nitrogen fertilization requirement and corn– soybean productivity with a rye cover crop. Soil Sci. Soc. Am. J. 79: 1482–1495.
Pena-Yewtukhiw, E.M, J.H. Grove, and G.J. Schwab. 2015. Fertilizer nitrogen rate prescription, interpretational algorithms, and individual sensor performance in an array. Agron. J. 107: 1691-1700.
Pretty, J., W.J. Sutherland, J. Ashby, J. Auburn, D. Baulcombe, M. Bell, J. Bentley, S. Bickersteth, K. Brown, J. Burke, H. Campbell, K. Chen, E. Crowley, I. Crute, D. Dobbelaere, G. Edwards-Jones, F. Funes-Monzote, H.C.J. Godfray, M. Griffon, … and S. Pilgrim. 2010. The top 100 questions of importance to the future of global agriculture. Int. J. Agric. Sustain. 8(4): 219–236.
Puntel, L.A., A. Pagani, and S.V. Archontoulis. 2019. Development of a nitrogen recommendation tool for corn considering static and dynamic variables. Eur. J. Agron. 105: 189-199.
Rabalais, N.N., R.E. Turner, and D. Scavia. 2002. Beyond science into policy: Gulf of Mexico Hypoxia and the Mississippi River. Biosci. 52: 129–142.
Ransom, C.J., N.R. Kitchen, J.J. Camberato, P.R. Carter, R.B. Ferguson, F.G. Fernandez, D.W. Franzen, C.A.M. Laboski, D.B. Myers, E.D. Nafziger, J.E. Sawyer, P.C. Scharf, and J.F. Shanahan. 2020. Corn nitrogen rate recommendation tool’s performance across eight US Midwest corn belt states. Agron J. 2020: 112:470-492.
Raper, T.B., J.J. Varco, and K.J. Hubbard. 2013. Canopy-based normalized difference vegetation index sensors for monitoring cotton nitrogen status. Agron. J. 105: 1345-1354.
Ravishankara, A.R., J.S. Daniel, and R.W. Portmann. 2009. Nitrous oxide (N2O): The dominant ozone-depleting substance emitted in the 21st century. Sci. 326(5949): 123–125.
Sawyer, J., G. Nafziger, L. Randall, G. Bundy, B. Rehm, C. Joern, R. Laboski, R. Hoeft, R. Mullen, R. Killorn, and S. Brouder. 2006. Concepts and rationale for regional nitrogen rate guidelines for corn. Iowa State University, University Extension Publ. PM 2015. p 27.
Sawyer, J., E. Nafziger, J. Camberato, K. Steinke, D. Kaiser, S. Culman, and C. Laboski. 2020. Corn N rate calculator website. http://cnrc.agron.iastate.edu
Scharf, P.C., and M.M. Alley. 1988. Nitrogen loss pathways and nitrogen loss inhibitors: a review. J. Fert. Issues 5(4): 109-125.
Scharf, P.C., N.R. Kitchen, K.A. Sudduth, J.G. Davis, V.C. Hubbard, and J.A. Lory. 2005. Field-scale variability in optimal nitrogen fertilizer rate for corn. Agron. J. 97: 452-461.
Scharf, P.C., S.M. Brouder, and R.G. Hoeft. 2006a. Chlorophyll meter readings can predict nitrogen need and yield response of corn in the north-central U.S. Agron. J. 98: 655–665.
Scharf, P.C., N.R. Kitchen, K.A. Sudduth, and J.G. Davis. 2006b. Spatially variable corn yield is a weak predictor of optimal nitrogen rate. Soil Sci. Soc. Am. J. 70: 2154-2160.
Scharf, P.C., D.K. Shannon, H.L. Palm, K.A. Sudduth, S.T. Drummond, N.R. Kitchen, L.J. Mueller, V.C. Hubbard, and L.F. Oliveira. 2011. Sensor-based nitrogen applications out-performed producer-chosen rates for corn in on-farm demonstrations. Agron. J. 103: 1683–1691.
Sela, S., H.M. van Es, B.N. Moebius-Clune, R. Marjerison, and G. Kneubuhler. 2018. Dynamic model-based recommendations increase the precision and sustainability of N fertilization in midwestern US maize production. Comput. Electron. Agric. 153: 256–265.
Shapiro, C.A., and C.S. Wortmann. 2006. Corn response to nitrogen rate, row spacing, and plant density in Eastern Nebraska. Agron. J. 98: 529-535.
Shcherbak, I., N. Millar, and G.P. Robertson. 2014. Global metaanalysis of the nonlinear response of soil nitrous oxide (N2O) emissions to fertilizer nitrogen. Proc. Natl. Acad. Sci. U.S.A 111(25): 9199-9204.
Sierra, J. 1997. Temperature and soil moisture dependence of N mineralization in intact soil cores. Soil Biol. Biochem. 29: 1557-1563.
Simons, M., R. Saha, L. Guillard, G. Clement, P. Armengaud, and R. Canas, et al. 2014. Nitrogen-use efficiency in maize (Zea mays L.): from 'omics' studies to metabolic modelling. J. Exp. Bot. 65: 5657-5671.
Smil, V. 2001. Enriching the Earth. MIT Press, Cambridge, Massachusetts.
Smil, V. 2011. Nitrogen cycle and world food production. World Agriculture 2: 9-13.
Smith, J.L., H.P. Collins, A.R. Crump, and V.L. Bailey. 2015. Management of soil biota and their processes. In: Soil Microbiology, Ecology and Biochemistry (Ed: E Paul): pp. 539–572.
Sullivan, D., A. Moore, E. Verhoeven, and L. Brewer. 2020. Baseline soil nitrogen mineralization: measurement and interpretation. Oregon State University Extension Service https://catalog.extension.oregonstate.edu/em9281
Thapa, R., S.B. Mirsky, and K.L. Tully. 2018. Cover crops reduce nitrate leaching in agroecosystems: A global meta-analysis. J. Environ. Qual. 47: 1400–1411.
Tilman, D., K.G. Cassman, P.A. Matson, R. Naylor, and S. Polasky. 2002. Agricultural sustainability and intensive production practices. Nature 418: 671-677.
Tremblay, N., Y.M. Bouroubi, C. Bélec, R.W. Mullen, N.R. Kitchen, W.E. Thomason, S. Ebelhar, D.B. Mengel, W.R. Raun, D.D. Francis, E.D. Vories, and I. Ortiz-Monasterio. 2012. Corn response to nitrogen is influenced by soil texture and weather. Agron. J. 104: 1658–1671.
U.S. Environmental Protection Agency. 2016. U.S. Greenhouse Gas Emissions and Sinks: 1990–2016. Available at https://www.epa.gov/ghgemissions/inventory-us-greenhouse-gas-emissions-and-sinks-1990-2016
Vitousek, P.M., and R.W. Howarth. 1991. Nitrogen limitation on land and in the sea: How can it occur? Biogeochem. 13: 87–115.
Wade, J., W.R. Horwath, and M.B. Burger. 2016. Integrating soil biological and chemical indices to predict net nitrogen mineralization across California agricultural systems. Soil Sci. Soc. Am. J. 80(6): 1675-1687.
Wade, J., S.W. Culman, J.A.R. Logan, H. Poffenbarger, M.S. Demyan, J.H. Grove, A.P. Mallarino, J.M. McGrath, M. Ruark, and J.R. West. 2020. Improved soil biological health increases corn grain yield in N fertilized systems across the Corn Belt. Sci. Rep. 10, 1–9.
Yan, M., G. Pan, J.M. Lavallee, and R.T. Conant. 2019. Rethinking sources of nitrogen to cereal crops. Glob. Chang. Biol. 1–9.
Yost, M.A., K.S. Veum, N.R. Kitchen, J.E. Sawyer, J.J. Camberato, P.R. Carter, R.B. Ferguson, F.G. Fernandez, D.W. Franzen, C.A. Laboski, and E.D. Nafziger. 2018. Evaluation of the Haney soil health test for corn nitrogen recommendations across eight Midwest states. J. Soil Water Conserv. 73: 587-592.