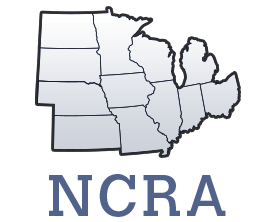
NC1211: Precision Management of Animals for Improved Care, Health, and Welfare of Livestock and Poultry
(Multistate Research Project)
Status: Active
NC1211: Precision Management of Animals for Improved Care, Health, and Welfare of Livestock and Poultry
Duration: 10/01/2021 to 09/30/2026
Administrative Advisor(s):
NIFA Reps:
Non-Technical Summary
Statement of Issues and Justification
Precision Management of Animals, application of technology within the animal space, is one of the most powerful developments among many new innovations in agriculture and has the potential to revolutionize the livestock industries. Today’s livestock producers are faced with many competing challenges including improving animal welfare, decreasing access to antibiotics, managing waste sustainably, reducing or eliminating odor emission, controlling the thermal environment, and educating and employing qualified animal caretakers. In addition, producers are faced with shrinking profit margins, which has fueled the expansion of existing livestock production systems in an attempt to preserve economic viability. As a result, current commercial farms typically house large numbers of animals in relatively confined conditions managed by only a few animal caretakers. This combination makes it hard for workers to identify and monitor individual animals, particularly when they all look similar, as is the case with laying hens, broilers or pigs. Current labor and demographic circumstances challenge livestock producers to find employees, especially employees with farm or animal care backgrounds (Carlin et al., 2008). The result is fewer people with less experience caring for more animals. Under these conditions, it is more difficult for caretakers to identify problems (health, production, and/or safety concerns) of individual animals within the facility. This lack of individual attention can lead to welfare problems and result in less than optimal production.
In modern livestock production, the management unit is typically a herd or flock (animals housed within a facility); little is known about an individual animal (health, well-being, weight gain, behavior, etc.). On a daily basis, the workers note the general condition of individual animals; however, with large groups of animals and a shortage of time, it is difficult to spot individuals with minor or developing health and/or welfare problems.
The application of technology within the animal space can provide information about not only the behavior of the groups of animals but ideally, will monitor individuals. Monitoring animals using precision management of animals (PMA) technology will be used to improve animal welfare, and the data stream can also help guide new facility design, and genetic evaluation and selection. The greatest opportunity with PMA is its potential to alert the animal caretakers to deliver individual care to an animal showing altered behavior as a result of disease, injury, or a stressor. PMA could also be used to manage animals in ways that more closely approximates their natural state—such as delivering feed multiple times a day to simulate the nearly continuous grazing performed by cattle. To use PMA to improve welfare, however, we need systems that integrate data related to production, health and behavior measures into comprehensible outputs that producers will trust and use.
Precision management of animals has the potential for disease detection, treatment, and prevention. In a system where the individual animal is the management unit, electronic monitoring of animals will provide earlier, and more precise, detection of disease and conditions related to welfare. This capability would reduce the consequences of disease by first minimizing the impact on the individual and by preventing or slowing the spread of the disease through the herd/flock. Additional benefits include reducing the total cost of the medication, and the amount of antibiotic residue entering the waste handling system and potentially the environment. Thus, the resulting benefits are leveraged beyond the animals and the facility.
Beyond managing health and welfare, the individual animal data stream captured by a PMA system can be used for the development of novel phenotypes and gain insights into the consequences of engineering design of the animal spaces. Novel phenotypes like disease resistance, heat tolerance, and mothering ability, can aid in genetic selection. Not only would this information would assist geneticists in selecting breeding stock, but also identify traits of economic importance for genomic evaluation. Thus, providing information about relevant production traits that is otherwise unobtainable. The collection of individual animal data within a commercial facility will allow engineers an in-depth look at the interaction between environment (stocking density, lighting, temperature, etc.) and behavior and performance of individual animals within the space. This new perspective on facility design will help engineers develop and test different animal facilities in the future.
Precision Management of Animals will change animal production from a primarily manual labor industry to a technology-based industry with targeted management careers. To develop these integrated systems, a truly multi-interdisciplinary approach is needed. The key to PMA is real-time data collection on individual animals with little or no disruption of the animal activities, which requires small wearable sensors integrated with animal identification and video or image analysis of the animals. Meeting this need requires input from electrical engineers and computer scientists with animal scientists to help inform and guide the process so data collection is targeted for the needs of the producer or genetic company. Additionally, disease detection models will require input from veterinarians to detect symptoms of different diseases. Finally, agricultural and biological systems engineers are needed to provide an understanding of animal housing systems and knowledge of modeling biological processes to turn a raw data stream into management information, thus creating novel systems. Using this multi-interdisciplinary approach will ensure that the research generates targeted solutions, and PMA will have a real and sustainable impact on the future of animal production.
To continue to move animal agriculture forward into the future, a combined effort from multiple land grant universities (LGU) is needed. As animal production sometimes varies by region, having people from multiple LGU working on a single effort will allow the research to be guided to ensure that solutions being developed can be applied to animal systems in multiple regions. Housing the PAM multistate committee in the North Central Region takes advantage of the $125 billion agriculture-driven industry which employees 2.4 million people in the region. The North Central Region has large and diverse livestock and poultry industries and is responsible for over 36% of the US food animal production. This region is responsible for 33% of eggs produced, 34% of all milk produced, 43% of beef produced, 75% of pork produced, and over 40% of turkey produced in the USA. Further, the Cooperative Extension Services and Agricultural Experiment stations in this 12-state region have the industry support and the expertise to lead the PMA effort in the USA. The need for PMA is highest within the states that have the largest animal populations.
Within the several years, several activities have occurred to promote and inform researchers about PMA. The 1st US Precision Livestock Farming Symposium was held in Omaha, NE, September 2018 and, Iowa State University held a Precision Livestock Workshop in December 2018. Universities within the North Central Region are looking to hire faculty in this area; in the last year, Nebraska, Illinois, and the Ohio State University hired faculty into newly created positions in Precision Management of Animals. In 2018, efforts at Michigan State, University of Wisconsin Madison and Kentucky have involved current faculty expanding their research into this area. The development of a PMA committee is a very timely effort for the North Central Region, because it will link key researchers across universities and disciplines, that otherwise would not converge into a common ground to develop the discipline.
Related, Current and Previous Work
A review of CRIS data suggests there is little duplication of NC-1211 research activities. This multistate NC-1211 is a combination of engineering, animal science, and data science. According to the CRIS database, there are two multistate projects with aspects related to our proposed project.
NC1029 Animal Behavior and Welfare Committee emphasizes using PMA to measure animal behavior and welfare and provide new quantitative ways of measuring each. Under the current NC1029 Objective 1: (To develop novel behavioral and physiological indicators of animal welfare), there is a stated aim of 'developing and validating automated methods for measuring individual animals' welfare.' Under Objective 2 (To strengthen the scientific basis of animal welfare assessment and auditing programs), there is a stated aim of "identifying animals with compromised welfare" (with an emphasis on using precision dairy technologies already commercially available on-farm). In this project rewrite, the scope changed a bit is focused on using technology to automatically record behavior and how PMA data can be used to automate welfare measures and better assess welfare. While this project has some overlap and potentially synergetic ideas, NC-1211 is focused on all aspects of animal production, not only welfare measures.
NE-1942: Enhancing Poultry Production Systems through Emerging Technologies and Husbandry Practices also incorporates technology specifically into their multistate. Objective 1 (Integrating technological advances into poultry systems) emphasizes collaborative and translational research incorporating science, engineering, and technology to enhance system efficiency and sustainability through infrastructure development of blockchain production. While similar and synergistic, NE-1942 objectives are clearly different and broader in some ways (ie blockchain) and narrower (poultry only) than NC-1211 objectives.
Objectives
-
Develop, validate, and evaluate sensor, instrumentation, controls and related hardware systems applied to livestock and poultry production.
-
Apply data science, data analytic, and other software techniques to big data collected from animal facilities to create usable information for assessments and improvements in welfare, engineering, genetic, and management of animals.
Methods
There is a shift to housing animals in larger social groups in the commercial livestock and poultry industries. Thus, it has become increasingly difficult for caretakers to manage animals. In addition, labor shortages and high labor turnover make it difficult to care for animals effectively and efficiently. Precision management of animals offers methods to improve animal care using technology-based tools. This project aims to address Theme 1 Sustainable Ag Intensification in USDA's newly released blueprint for their priorities over the coming five years. Outlined in these methods are ongoing and proposed research areas to address labor shortages in animal production, improve illness surveillance in group-housed animals, develop technologies to capture and evaluate animal behavior through the development, validation, and application of new or enhanced technologies for both sensors and data analytics.
Methods for Objective 1.
The development of precision management tools requires testing different technologies and system development and validation for using these technologies under different commercial conditions and with different species. No single technology offers all the solutions; therefore, many different technologies need to be critically evaluated. Under this objective, we anticipate working in three broad classes of technology:
- Remote sensors or sensors that are located in the vicinity of the animals to gather animal data.
- Wearable or attachable sensors, place on the animal that can identify, locate, classify movement or behavior, or record physiological responses.
- Environmental sensors to capture data from the housing environment can encompass the entire facility or focus on a targeted section to provide information at the animal level.
Remote Sensors:
Computer vision and other methods for remotely detecting animals and their behavior can provide data from animals housed in large groups that can be used to monitor, manage, and assess animal welfare. Remote sensing technologies include recording devices that can detect sounds of animals and their environment as well as a variety of vision-based technologies (e.g., monochromatic, color, 3D depth-sensing, infrared, and thermal cameras). These technologies can provide information about animal distribution in space, animal postures and behaviors, interactions among animals, and information about animals' physical characteristics such as shape, color, body condition, lameness, or animal temperature (Ellen et al., 2019; Wurtz et al., 2019).
Vision is one of the most used technologies for animal monitoring, and 2D red-green-blue (RGB) digital cameras have been used to determine animal characteristics such as weight, behavior, and critical welfare indicators (Berckmans 2017). While 2D RGB cameras are useful, there can be automation issues due to lighting, occlusion, and background color. In addition, some producers express privacy concerns related to RGB video data. Work at UNL and UT will continue to develop methods to overcome challenges with RGB video to track animals to capture behaviors and activity patterns.
Depth cameras are a recent development and an alternative to expensive laser scanners to create 3D images (Khoshelham & Elberink, 2012). Depth images have been used in several different livestock species to predict weight (Condotta et al., 2018, Kongsro, 2014; Mortensen et al., 2016; Song et al., 2018) and postures (Lao et al., 2016, Leonard et al., 2020; Zheng et al., 2018; Viazzi et al., 2014). Depth video has been used to detect gait changes associated with lameness (Jabbar et al., 2017; Condotta et al., 2020) and behaviors (Chen et al., 2019, 2020 a,b,c). These publications illustrated the usefulness of depth cameras; however, ongoing work is needed to enhance current methods and develop new and innovative methods of capturing behavior and production traits. University of Illinois (U of I), University of Nebraska- Lincoln (UNL), University of Wisconsin (UW), and Michigan State University (MSU) are developing methods to capture and analyze depth images\video to detect behaviors, condition scores, and animal movement in cattle, poultry, and pigs.
Wearable sensors:
Wearable sensors can encompass a range of technologies from sensors that record physiological variables to devices that detect motion, such as pedometers and accelerometers, or provide animal identification or tracking (RFID).
Stand-alone wearable sensors that provide information on the physiological responses have been developed (Brown-Brandl et al., 2003; Eigenberg et al., 2007). Small wireless body-worn sensors capable of detecting general levels of activity, specific behaviors, and location of hens relative to other hens as well as ambient and skin temperatures have been developed and validated at Michigan State University (Quwaider et al., 2010; Banerjee et al., 2012, 2014; Daigle et al., 2012, 2014). Such systems represent an approach to detecting specific information about individual animals' behavior and physiology while linking this to the environmental context.
Systems that include wearable sensors can provide information about animal location, movement, or proximity to critical resources such as feeders and drinkers. Several researchers have documented the use of RFID systems to help determine the feeding behavior of group-housed pigs (Adrion et al., 2018; Brown Brandl et al., 2013; Maselyne et al., 2014) and of group-housed hens and broilers (Li et al., 2017; Li et al., 2019). Furthermore, other parameters, such as drinking behavior, nesting behavior, social interaction between the animals, and general activity, are currently being evaluated (Kapun et al., 2017; Oliveira et al., 2019). RFID data streams contain valuable information that can be used for various needs, including pen design, livestock management, identification of sick animals, and genetic differences within a herd. One aim of this type of research is to predict illness and impairments to animal welfare (Brown-Brandl et al., 2016; Kapun et al., 2017; Maselyne et al., 2017). Research at MSU and UNL will be utilizing RFID technology to understand patterns of feeding and drinking.
Ultrawideband or active RFID sensors are have been used for tracking livestock and poultry. These sensors have been used in swine (Zhuang et al., 2020; Barnes et al., 2017), broilers (Van Der Sluis, 2019), and cattle (Arcidiacono et al., 2018; Meunier et al., 2017; Tullo et al., 2016). The ability to track livestock over a period has applications to detect health-related issues, aid in better-engineered animal facilities, among other applications.
Accelerometers measure the change in velocity and the static acceleration component of gravity in either two or three axes. Three-axis accelerometers and gyroscopes can also be used to measure precise movement trajectories using sufficient sample rates. In the last decade, the use of accelerometers attached to animals has become more popular for a diverse range of purposes, including recording position, general levels of activity, acceleration, movement in space, and even specific behaviors. Improvements in battery life (Marchioro et al., 2011) coupled with decreases in device size have resulted in sensors small enough for poultry (Broell et al., 2013). UNL, UW and UT will be evaluating the use of accelerometers to detect motion and changes in posture in pigs, cattle and poultry.
Environmental sensors:
Robust and sustainable controlled environment agriculture is critical to achieving optimal animal production efficiency with the least impact on animal welfare and our environment. Historically, environmental control has been based on a classical engineering approach to provide optimal thermal comfort and proper ventilation. The conventional approach to environmental sensing and control focuses only on one or two main environmental parameters and neglects other potentially relevant ones. Air temperature and humidity are the most commonly measured to assess interior thermal conditions. However, measurements are generally taken at only a few points in the barn and not general at the animal level, leading to uncomfortable conditions for some animals in the barn.
Another deficiency in this approach is that it takes a one-size-fits-all approach to the animals' needs under a given set of conditions. Advances in technology are moving quickly toward viable on-farm capabilities to offer real-time monitoring and potential to tailor animal management, which simultaneously increases our ability to address individuals' welfare needs and while more effectively directing resource inputs (Norton and Berckmans, 2018).
Methods of measuring aerial gas concentration in a barn can help assess the interior air quality.
Measures of CO2, NH3, and dust have been used in animal buildings. These measures can also help capture the changes in animals' heat and moisture production related to changes in modern housing designs (Brown-Brandl et al., 2014). University of Kentucky (UK), UNL, and Iowa State University (ISU) will be working together to capturing temperature distribution estimates of heat and moisture production in group-housed sows.
These data need to be processed using novel computer analytics (Objective 2) to fully capture the information contained with the data collected with these three different sensor types described in Objective 1.
Methods for Objective 2.
All the PMA technologies described under Objective 1 deliver highly dimensional data (sound, images, etc.) that need to be processed and distilled into useful indicators and summaries that can aid in making management decisions. Typical characteristics of PMA datasets are:
- Big Data. Images, video, and sound streams can quickly become unmanageable file sizes. Also, the sheer size of the data makes "direct visual inspection" of complete data impractical.
- Heterogeneous Data. Data from the same biological or environmental outcomes collected with different methods can be vastly different and even vary over time due to technical reasons as well as biological reasons.
- Continuous data streams need to be processed and distilled in real-time into useful management flags.
Thus, data analytic methods for PMA need to be flexible and scalable. Moreover, data analytics methods commonly used in PMA encompass classical statistical methods, machine learning, and deep learning methods. However, regardless of the paradigm used for data analysis, we can generally classify the uses and goals of data analytics techniques implemented in PMA into supervised learning and unsupervised learning algorithms.
In supervised learning, an algorithm learns a system's features (dataset) to predict or classify observations of a relevant outcome set (these are called labels or response variables depending on the application). In contrast, in unsupervised learning, the data's intrinsic structure is learned without considering any predictive or classificatory outcome.
In these contexts, we anticipate that the main data analytic challenges that will be faced when implementing PMA are dimension reduction (mostly for unsupervised learning) and penalization to avoid overfitting (but not exclusively, for supervised learning). In dimension reduction, a reduced set of variables is generated from the multi-dimensional data that retains the data's relevant characteristics while shedding unnecessary noise and detail. Penalization consists of imposing constraints of the parameter solutions in the analysis models to avoid overfitting.
We anticipate working in three broad data analytic tasks under this objective: 1) transformation of raw sensor data into meaningful metrics, 2) generating and sharing labeled data, 3) validation of existing and novel algorithms.
Transform raw sensor data into meaningful measurements:
Part of this committee's research will be focused on the elicitation, implementation, and validation of algorithms that transform raw sensor data into meaningful metrics for animal production. This will include computer vision (Berckmans, 2017), analysis of radio frequency signals (Adrion et al., 2018), and analysis of sound streams (Berckmans, 2017) used to detect and quantify behaviors and activities, to identify animals, to detect disease, to quantify growth and performance measures, etc.
To achieve on-farm tool development, we anticipate the need for analytic methods suitable for processing massive amounts of raw data in real-time. These methods will use dimension-reduction techniques to generate easy to process variables or features that will then be used to train prediction models for various production-relevant outcomes.
Dimension reduction will be accomplished through various approaches depending on the specific production system, sensor technology, and production outcome pursued. For instance, feature design and extraction will be used whenever possible. Feature design and extraction require understanding biological and technical details of the data generation process (Norton et al., 2019). But this approach gives the advantage of producing intermediate reduced data that is easily interpretable and repeatable over time and across farms. Also, reducing raw data to a small number of biologically and technically meaningful features facilitates accounting for heterogeneous sources of variation in the subsequent supervised analysis. Specifically, as features are generated and extracted, hierarchical statistical models can be used to dissect systematic and non-systematic sources of variation that moderate the relation between the predictive extracted features and the target production variables.
When feature design and extraction are not readily possible or when the extracted features fall short in their predictive ability of the target outcomes, unsupervised learning will be used directly or in combination with redundancy measures (Birodkar et al., 2019). Entropy computation (Shi et al., 2015; Yin et al., 2017) to reduce dimensionality and to produce a set novel intermediate variable. The resulting variables will not be readily interpretable, but they will retain optimal predictive properties. Classical statistical methods, machine learning, and deep learning will be used for these purposes. The resulting reduced set of variables will be validated over a wide range of conditions to ensure that they are valid for use across farms. An advantage of this approach to dimension reduction over the classic feature design and extraction paradigm is that through unsupervised learning new features of a system may be uncovered and learned that would be otherwise lost in the feature extraction process.
After dimension reduction, supervised learning methods will be used to generate predictive models. The main theme in these models will be the use of regularization to avoid overfitting. This will be achieved with classic statistical methods and with artificial intelligence methods such as machine learning and deep learning. Members of this group have ample experience utilizing all these techniques for generating predictive and forecasting models (Chen et al., 2020c, Condotta et al., 2018, Cairo et al., 2020). Still, we anticipate that the main bottleneck to implementing these methods will reside in the availability of properly labeled ground truth datasets. We describe this in the following sections.
All the proposed methods, including dimension reduction and prediction, will be optimized for computational efficiency. We anticipate that cloud computing and supercomputing will not be readily accessible on production farms. Thus, computational optimization of the analysis algorithm will be another area of research under this task. For instance, generating scalable methods that can take advantage of GPU computing available in personal gaming machines will be considered under this task. Moreover, our groups routinely use GPU computing for statistical, machine, and deep learning.
Generation and sharing of reference (labeled) data from production and experimental farms:
As mentioned above, supervised learning methods to train predictive models rely on the availability of reference data. In the case of sound and images, this usually requires extensive labeling. Manual data labeling is so far the current reference data generation standard in PMA. But manual labeling is not scalable over time and across farms.
Under this task we will investigate methods to semi-automate labeling (Yik et al., 2020). This could include pre-processing of data streams using simpler feature extraction algorithms to perform pre-annotation or segmentation that results in smaller data units (short snippets of video, pre-selected images or image regions, etc) that can be easily and consistently manually annotated.
Crowdsourcing annotation and labeling will also be tested. Crowdsourcing is potentially appealing for generating reference data in PMA (Fernandes et al., 2020), but it is also challenging to implement it, for instance, when untrained individuals must label complex behaviors or activities. Thus, crowdsourcing of PMA data labeling will require design of labeling schemes that allow for estimating inter-labeler reliabilities and repeatability while optimizing the use of resources.
Members of this committee have generated substantial amounts of labeled data for a number of funded PMA projects (Brown-Brandl et al., 2016; Leonard et al., 2019; Chen et al., 2019, 2020a). Thus, this committee will facilitate the sharing of such data and outputs of existing analysis for re-using them and for strategic relabeling. For instance, image data from feeding pigs has been generated by groups at MSU and at UNL, and cross-sharing those data can further help to improve the predictive ability of existing models. One limitation to sharing some data (e.g., images) is obtaining consent from farm managers and owners. Part of the work of this committee under this task will focus on the anonymization of raw data and in providing guidelines for establishing agreements with farmers to facilitate the sharing of data collected in commercial farms.
Validation of analytical approaches for PMA:
There is currently an explosion of published data analytic methods for supervised and unsupervised learning applied to PMA (Halachmi et al., 2019; Garcia et al., 2020). In most cases, methods are developed under a limited set of experimental or production conditions. Assessing the scope of the validity of those methods is critical for generating robust PMA technologies.
Members of this committee will perform validation of newly generated and existing PMA algorithms. Sharing labeled data across groups will be instrumental for this purpose.
Standards and metrics for validation will be generated for distinct types of predictive models applicable to a variety of production systems and sensing technologies. For instance, metrics for validation of continuous and automatic behavior detection have to be developed separately in beef cattle, pork, and poultry production systems and for RFID, accelerometer, and computer vision data. Also, validation metrics to assess the goodness of data integration will be pursued.
Developing validation protocols will require the development of visualization techniques and interfaces to quickly diagnose predictive problems. And an important side benefit of this is that some visualization tools will also be useful for on-farm PMA applications, to facilitate human-technology interactions for improved management decisions (Vranken and Berckmans, 2017).
Finally, algorithms and sensors themselves rapidly change over time, making it difficult to analyze data longitudinally. Having standards and open-source algorithms capable of dealing with PMA data streams in areas such as computer vision are necessary to fully realize the potential of PMA data analytics. A way to foster the development of novel algorithms in this field is the distribution of relevant datasets as described in the previous section.
Our members of our committee are working multiple areas of data analytics. Many of these techniques are still developing applications to precision animal management techniques. Therefore, meetings to share data and analysis techniques are critical to further the development of PMA tools.
Measurement of Progress and Results
Outputs
- Publication of results in peer-reviewed manuscripts that will provide researchers and educators tools and information on how to assess animal welfare.
- Development and/or validation of automated measures of animal activity, health and well-being.
- Development and/or validation of data processing methods to process large datasets Sharing labeled data for methods development.
- Dissemination of information to farmers and other people involved in animal production to translate science into practices that improve the development of precision management technologies for on farm use.
- Next generations of highly qualified personnel will be mentored and taught about PMA using knowledge gained by this project.
Outcomes or Projected Impacts
- Development and/or validation of automated measures of welfare, including sensors and machine vision technologies, that provide indication of health and behavior will lead to uptake of these technologies in commercial situations.
- Development and/validation of new data processing methods for large data sets will increase the use of technology in the animal sector.
- Findings of this committee have potential to impact the sustainability of animal agricultural by easing the labor burden, improving traceability and transparency, and increasing animal welfare—thus increasing the productivity and competitiveness of US producers.
- Results from this project will provide science-based information to automate the detection of illness in animals to aid in the judicious use of antimicrobials.
- Electronic data collected on every animal in the facility will aid in better engineering design of animal environments.
- Continuous data streams from individual animals will be used to develop novel phenotypes.
Milestones
(2022):Recruit members from experiment stations to participate in the objective and tasks delineated to ensure deep expertise. Complete inventory of past, ongoing, and future work in each objective; identify commonalities and areas for synergy.(2023):Apply collaboratively for funding. Conduct planned studies using the methods outlined above. Share raw data between committee members for re-labeling.
(2024):Continue conducting planned studies; prepare data for manuscripts and conference proceedings. Share labeled reference datasets with the research community.
(2025):Continue conducting planned studies; prepare data for manuscripts and conference proceedings.
(2026):Complete publication of findings.
Projected Participation
View Appendix E: ParticipationOutreach Plan
Various members of our development committee hold extension or outreach appointments and will contribute to dissemination of project findings to other researchers, producers, and specialized groups who are using this kind of practical information to assess the welfare of agricultural and captive animals. There are also members involved in teaching activities so that we can education the upcoming generation of researchers, industry and producers.
Research scientists will be targeted through the American Society of Agricultural and Biological Engineers (ASABE), which is the international professional organization for agricultural and biological engineers and International commission of Agricultural and Biosystems Engineering (CIGR) which is the global network of agriculture and biological engineers. In addition, appropriate scientific animal societies, such as American Society of Animal Science (ASAS), American Dairy Science Association (ADSA), American Association of Swine Veterinarians (AASV) and Poultry Science Association (PSA). The final research results will be presented at the ECPLF biannual conferences before an international audience. Members will also present research results at the ASABE, ASAS, ADSA, and PSA annual meetings. Publications will be published in a variety of journals including Frontiers in Animal Science, Biosystem Engineering, Computers and Electronics in Agriculture, Transactions of the ASABE, and the Journals of Animal Science, Dairy Science, and Poultry Science.
In general, this project is expected to result in collaborative, peer-reviewed scientific publications and reviews, as well as abstracts presented at national and international meetings, and extension publications. This project also provides unique opportunities for interdisciplinary training of graduate students and other research personnel.
Organization/Governance
The Executive Committee of NCDC235 shall consist of the Chair and Secretary.
Chair: The chair of the committee is responsible for organizing the meeting agenda, conducting the meeting, preparing the final version of the annual report, and assuring that tasks and assignments are completed.
Secretary: The secretary is responsible for keeping records on decisions made at meetings (a.k.a. keeping the minutes) and assisting in the preparation of the annual report by collecting and combining station reports.
The Chair is elected for a 1-year term. The term of Office of the Chair will end at the adjournment of the regular annual meeting. The previous Secretary will become the Chair for 1 year. A new secretary will be elected each year by those attending the Committee meeting.
Members: Committee membership requires active participation and information exchange (including the submission of a station report) at the annual meetings. In addition to carrying out the agreed information exchange, project members are responsible for contributing to the ongoing progress of any committee activity and communicating their accomplishments to the committee's members and their respective employing institutions. Regular attendance is vital for a committee to be successful. Therefore, members that do not attend the annual meeting or send a substitute 3 years in a row will be removed from the committee.
Literature Cited
Adrion, F., Kapun, A., Eckert, F., Holland, E.-M., Staiger, M., Götz, S., & Gallmann, E. (2018). Monitoring trough visits of growing-finishing pigs with UHF-RFID. Comput. Electron. Agric., 144, 144–153. https://doi.org/10.1016/j.compag.2017.11.036
Arcidiacono, C., Porto, S. M., Mancino, M., & Cascone, G. (2018). A software tool for the automatic and real-time analysis of cow velocity data in free-stall barns: The case study of oestrus detection from Ultra-Wide-Band data. Biosystems Engineering, 173, 157-165.
Banerjee D, Daigle C, Biswas S and Siegford JM. 2012. Remote activity classification of hens using wireless body mounted sensors. Body Sensor Networks. 2012: 107-112.
Banerjee D, Daigle CL, Dong B, Wurtz K, Newberry RC, Siegford J and Biswas S. 2014. Detection of jumping and landing force in laying hens using wireless wearable sensors. Poultry Science. 93: 1-10.
Barnes, B. L., Keshwani, D. R., & Brown-Brandl, T. M. (2016). Deployment and evaluation of an active RFID tracking system for precision animal management. In Proceedings of the 8th European conference on precision livestock farming (pp. 614-621).
Berckmans, D. (2017). General introduction to precision livestock farming. Animal Frontiers, 7(1), 6-11.
Birodkar V., Mobahi H., and Bengio S., “Semantic redundancies in image-classification datasets: The 10% you don’t need,” arXiv [cs.CV], 2019
Broell F, Noda T, Wright S, Domenici P, Steffensen JF, et al. 2013. Accelerometer tags: detecting and identifying activities in fish and the effect of sampling frequency. Journal of Experimental Biology. 216:1255–64.
Brown-Brandl TM, Yanagi J, Xin H, Gates RS, Bucklin RA and Ross GS. 2003. A new telemetry system for measuring core body temperature in livestock and poultry. Applied Engineering in Agriculture. 19: 583-589.
Brown-Brandl, T. M., Hayes, M. D., Xin, H., Nienaber, J. A., Li, H., Eigenberg, R. A., ... & Shepherd, T. (2014). Heat and moisture production of modern swine.
Brown-Brandl, T. M., Jones, D. D., & Eigenberg, R. A. (2016). Modelling Feeding Behavior of Swine to Detect Illness. In Int. Conf.Proc. International Conference on Agricultural Engineering, CIGR – AgEng 2016. Retrieved from http://conferences.au.dk/uploads/tx_powermail/2016cigr_tmb_05292016_.docx
Brown-Brandl, T. M., Rohrer, G. A., & Eigenberg, R. A. (2013). Analysis of feeding behavior of group housed growing–finishing pigs. Comput. Electron. Agric., 96, 246–252. https://doi.org/10.1016/j.compag.2013.06.002
Cairo, F. C., Pereira, L. G. R., Campos, M. M., Tomich, T. R., Coelho, S. G., Lage, C. F. A., ... & Dorea, J. R. R. (2020). Applying machine learning techniques on feeding behavior data for early estrus detection in dairy heifers. Computers and Electronics in Agriculture, 179, 105855.
Chen C, Zhu W, Liu D, Steibel J, Siegford J, Wurtz K, Han J and Norton T. 2019. Detection of aggressive behaviours in pigs using a RealSense depth sensor. Computers and Electronics in Agriculture. 166: 105003.
Chen C, Zhu W, Steibel J, Siegford J, Han J and Norton T. 2020a. Classification of drinking and drinker-playing in pigs by a video-based deep learning method. Biosystems Engineering. 196: 1-14.
Chen C, Zhu W, Steibel J, Siegford J, Han J and Norton T. 2020c. Recognition of feeding behaviour of pigs and determination of feeding time of each pig by a video-based deep learning method. Computers and Electronics in Agriculture. 176: 105642.
Chen C, Zhu W, Steibel J, Siegford J, Wurtz K, Han J and Norton T. 2020b. Recognition of aggressive episodes of pigs based on convolutional neural network and long short-term memory. Computers and Electronics in Agriculture. 169: 105116.
Condotta, I. C., Brown-Brandl, T. M., Rohrer, G. A., & Silva-Miranda, K. O. (2020). Development of method for lameness detection based on depth image analysis. In 2020 ASABE Annual International Virtual Meeting (p. 1). American Society of Agricultural and Biological Engineers.
Condotta, I. C., Brown-Brandl, T. M., Silva-Miranda, K. O., & Stinn, J. P. (2018). Evaluation of a depth sensor for mass estimation of growing and finishing pigs. Biosystems Engineering, 173, 11-18.
Daigle C, Banerjee D, Biswas S and Siegford JM. 2012. Non-caged laying hens remain unflappable while wearing body-mounted sensors: levels of agonistic behaviors remain unchanged and resource use is not reduced after habituation. Poultry Science. 91: 2415-2423.
Daigle CL, Banerjee, D, Montgomery RA, Biswas, SK and Siegford JM. 2014. Moving GIS indoors: spatiotemporal analysis of agricultural animals. PLOS One. 9(e104002): 1-11.
Eigenberg, R. A., R. A. Bucklin, and T. M. Brown-Brandl. (2009). Instrumentation for research and management in animal agriculture. In J. A. DeShazer (Ed.), ASABE Monograph No. 25: Livestock energetics and thermal environment management (1st ed., pp. 113 - 126). St. Joseph, MI: ASABE.
Ellen ED, van der Sluis M, Siegford J, Guzhva O, Toscano M, Bennewitz J, van der Zande L, van der Eijk J, de Haas EN, Norton T, Piette D, Tetens J, de Klerk B, Visser B and Rodenburg TB. 2019. Review of sensor technologies in animal breeding: phenotyping behaviors of laying hens to select against feather pecking. Animals. 9: 108.
Fernandes, Arthur FA, et al. "Deep Learning image segmentation for extraction of fish body measurements and prediction of body weight and carcass traits in Nile tilapia." Computers and Electronics in Agriculture 170 (2020): 105274.
García, R., Aguilar, J., Toro, M., Pinto, A., & Rodríguez, P. (2020). A systematic literature review on the use of machine learning in precision livestock farming. Computers and Electronics in Agriculture, 179, 105826.
Halachmi, I., Guarino, M., Bewley, J. and Pastell, M., 2019. Smart animal agriculture: application of real-time sensors to improve animal well-being and production. Annual Review of Animal Biosciences, 7, 403-425.
Jabbar, K. A., Hansen, M. F., Smith, M. L., & Smith, L. N. (2017). Early and non-intrusive lameness detection in dairy cows using 3-dimensional video. Biosystems engineering, 153, 63-69.
Kapun, A., Adrion, F., & Gallmann, E. (2017). Behaviour and activity monitoring of growing-finishing pigs with UHF RFID. In D. Berckmans & A. Keita (Eds.), Precision Livestock Farming ‘17: Papers presented at the 8th European Conf. on Precision Livestock Farming (pp. 605–613). Self-published.
Khoshelham, K., & Elberink, S. O. (2012). Accuracy and resolution of kinect depth data for indoor mapping applications. Sensors, 12(2), 1437-1454.
Lao, F., Brown-Brandl, T., Stinn, J. P., Liu, K., Teng, G., & Xin, H. (2016). Automatic recognition of lactating sow behaviors through depth image processing. Computers and Electronics in Agriculture, 125, 56-62.
Leonard, S. M., Xin, H., Brown-Brandl, T. M., & Ramirez, B. C. (2019). Development and application of an image acquisition system for characterizing sow behaviors in farrowing stalls. Computers and Electronics in Agriculture, 163, 104866.
Leonard, S. M., Xin, H., Ramirez, B. C., Stinn, J. P., Dutta, S., Liu, K., & Brown-Brandl, T. M. (2020). Static and Dynamic Space Usage of Late Gestation Sows. Transactions of the ASABE, 0.
Li, G., Zhao, Y., Hailey, R., Zhang, N., Liang, Y., Purswell, J.L. (2019). An ultra-high frequency RFID system for studying individual feeding and drinking behaviors of group-housed broilers. Animal, 13(9): 2060-2069.
Li. L., Zhao, Y., Oliveira, J., Verhoijsen, W., Xin, H. (2017). A UHF RFID system for studying individual feeding and nesting behaviors of group-housed laying hens. Transactions of the ASABE, 60(4): 1337-1347.
Marchioro GF, Cornou C, Kristensen AR, Madsen J. 2011. Sows’ activity classification device using acceleration data—a resource constrained approach. Computers and Electronics in Agriculture. 77:110–17
Maselyne, J., Saeys, W., De Ketelaere, B., Mertens, K., Vangeyte, J., Hessel, E. F., van Nuffel, A. (2014). Validation of a High Frequency Radio Frequency Identification (HF RFID) system for registering feeding patterns of growing-finishing pigs. Comput. Electron. Agric.,. 102, 10–18. https://doi.org/10.1016/j.compag.2013.12.015
Maselyne, J., van Nuffel, A., Briene, P., Vangeyte, J., Ketelaere, B. de, Millet, S.,. . . Saeys, W. (2017). Online warning systems for individual fattening pigs based on their feeding pattern. Biosyst. Eng. Advance online publication. https://doi.org/10.1016/j.biosystemseng.2017.08.006
Meunier, B., Delval, E., Cirie, C., Richard, M. M., Pradel, P., Gaudron, Y., ... & Veissier, I. (2017, September). Automated measurement of dairy cow grooming behaviour from real time location system. In 8. European Conference on Precision Livestock Farming (ECPLF).
Mortensen, A. K., Lisouski, P., & Ahrendt, P. (2016). Weight prediction of broiler chickens using 3D computer vision. Computers and Electronics in Agriculture, 123, 319-326.
Norton, T., Chen, C., Larsen, M. L. V., & Berckmans, D. (2019). Precision livestock farming: building ‘digital representations’ to bring the animals closer to the farmer. animal, 13(12), 3009-3017.
Oliveira, J., Xin, H., Wang, K., Zhao, Y. (2019). Nesting behavior of individual laying hens in an enriched colony housing. International Journal of Agricultural and Biological Engineering, 12 (6): 7-15.
Quwaider M, Daigle CL, Biswas SK, Siegford JM and Swanson JC. 2010. Development of a wireless body-mounted sensor to monitor activity and location of laying hens in a non-cage housing system. Transactions of the American Society of Agricultural and Biological Engineers. 53: 1705-1713.
Shi J., Jiang Q., Zhang Q., Huang Q., and Li X., “Sparse kernel entropy component analysis for dimensionality reduction of biomedical data,” Neurocomputing, vol. 168, pp. 930–940, 2015.
Song, X., Bokkers, E. A. M., van der Tol, P. P. J., Koerkamp, P. G., & van Mourik, S. (2018). Automated body weight prediction of dairy cows using 3-dimensional vision. Journal of dairy science, 101(5), 4448-4459.
Tullo, E., Fontana, I., Gottardo, D., Sloth, K. H., & Guarino, M. (2016). Validation of a commercial system for the continuous and automated monitoring of dairy cow activity. Journal of Dairy Science, 99(9), 7489-7494.
Van Der Sluis, M., De Klerk, B., Ellen, E. D., De Haas, Y., Hijink, T., & Rodenburg, T. B. (2019). Validation of an ultra-wideband tracking system for recording individual levels of activity in broilers. Animals, 9(8), 580.
Viazzi, S., Bahr, C., Van Hertem, T., Schlageter-Tello, A., Romanini, C. E. B., Halachmi, I., ... & Berckmans, D. (2014). Comparison of a three-dimensional and two-dimensional camera system for automated measurement of back posture in dairy cows. Computers and Electronics in Agriculture, 100, 139-147.
Vranken, E., & Berckmans, D. (2017). Precision livestock farming for pigs. Animal Frontiers, 7(1), 32-37.
Wurtz K, Camerlink I, D’Eath RB, Peña Fernández A, Norton T, Steibel J and Siegford J. 2019. Recording behaviour of indoor-housed farm animals automatically using machine vision technology: A systematic review. PLOS ONE. 14(12): e0226669.
Yik, S., Benjamin, M., Lavagnino, M., & Morris, D. DIAT (Depth-Infrared Image Annotation Transfer) for Training a Depth-Based Pig-Pose Detector. 2020 IEEE/RSJ International Conference on Intelligent Robots and Systems (IROS)October 25-29, 2020, Las Vegas, NV, USA (Virtual)978-1-7281-6211-9/20/$31.00 ©2020 IEEE1080
Yin S., Qian Y., and Gong M., “Unsupervised hierarchical image segmentation through fuzzy entropy maximization,” Pattern Recognit., vol. 68, pp. 245–259, 2017.
Zheng, C., Zhu, X., Yang, X., Wang, L., Tu, S., & Xue, Y. (2018). Automatic recognition of lactating sow postures from depth images by deep learning detector. Computers and Electronics in Agriculture, 147, 51-63.
Zhuang, S., Maselyne, J., Van Nuffel, A., Vangeyte, J., & Sonck, B. (2020). Tracking group housed sows with an ultra-wideband indoor positioning system: A feasibility study. Biosystems Engineering, 200, 176-187.