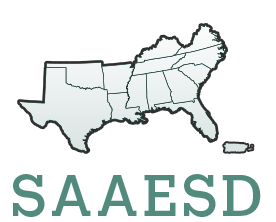
S1078: Cognitive Influence on Teaching, Learning, and Decision Making Around Critical Agricultural and Natural Resource Issues
(Multistate Research Project)
Status: Inactive/Terminating
S1078: Cognitive Influence on Teaching, Learning, and Decision Making Around Critical Agricultural and Natural Resource Issues
Duration: 10/01/2018 to 09/30/2023
Administrative Advisor(s):
NIFA Reps:
Non-Technical Summary
Statement of Issues and Justification
The agricultural and natural resource industry must address a variety of significant, and often controversial, issues because it consists of a multitude of complexly intertwined societal functions — food, fuel, and fiber — all of which “have to be fulfilled, simultaneously, in a framework in which inputs (i.e., soil, water, air) are increasingly tight” (Aertset al., 2009, p. 331). Determining how these functions are carried out individually and collectively is an equally complex mixture of politics, economics, science, ethics, culture, and consumerism. Unfortunately, people are either incapable or unwilling to engage in civil discourse to develop efficient action as “opportunities for honest discussion are reduced in today’s polarized environment” (Epstein & Graham, 2007, p. 1). As society becomes more nationally and globally connected, the need for people to communicate and work collaboratively to address agriculture and natural resource issues is becoming increasingly important.
Cognition plays a large role in how people engage in teaching and learning experiences, and ultimately make decisions, including how they work collaboratively and communicate with one another. Cognitive styles, or an individual’s preferred way of organizing and retaining information (Keefe, 1979), need to be studied to understand the influence of teaching and learning on decision making and encouraging civil discourse. The resulting findings will then inform the development of educational programming that integrates critical agricultural and natural resource issues into formal and non-formal learning environments. Critical issues include (a) food security, (b) climate variability and change, (c) water, (d) bioenergy, (e) childhood obesity, and (f) food safety (U.S. Department of Agriculture, National Institute of Food and Agriculture, n.d.).
The overall purpose of this project is to develop a teaching and learning decision support system to aid in (a) the identification and stakeholder implementation of new production and resource management technologies and practices (including those that may be contentious such as gene editing, unmanned aerial systems, and those addressing climate change), (b) the ability to integrate holistic perspectives on agriculture and natural resources issues into formal and non-formal educational programs, and (c) to ensure cultural consideration in the decision making process. Ultimately, this will increase stakeholder adoption of information and technologies that will lead to systems that ensure the sustainability of agriculture and natural resources.
There is little research examining cognition’s effect on how cognitive styles impact teaching and learning, including how that influences decision making with respect to critical agricultural and natural resources issues. Therefore, it is essential that researchers explore this connection in order to develop a decision support system that is effective both regionally and nationally. Without this research and the collaboration of researchers across the country, the adoption of information and technologies related to critical agriculture and natural resource issues may be slowed or ceased, leading to unnecessary inefficiencies in agricultural systems and degradation of natural resources.
Advantages of a Multistate Effort
Agricultural and natural resource issues can be local, regional, national or international in scope. Advantages of the multistate effort include an effective forum for utilizing distinct expertise, local and regional knowledge, and building collaboration among agricultural scientists. The effort expands the ability to investigate the feasibility of differing agricultural education and communication strategies in rural and urban centers and across economic, demographic, and commodity foci. Collaborative approaches are likely to formulate stronger solutions when compared to a single state or single researcher effort. Collaboration in a multistate effort will streamline and focus research that bridges agricultural education, extension education, communication and leadership. Past research has lacked congruence across research instruments. This project will also allow longitudinal data and replication of studies across the United States. With the exception of a few nationwide efforts, current research related to the scholarship of agricultural education and communication is conducted without a focused effort. Without the structure of a multistate project, this proposed research would be more challenging to manage and would create a need for more effort on behalf of the faculty involved. The project is significant to the National Research Agenda for Agricultural Education and Communication (Roberts et al., 2016) that called for holistic approaches to identifying what education and communication methods, models and practices are most effective in leading change and diffusing agricultural innovations. The research agenda set forth by the USDA NIFA complements the National Research Agenda for Agricultural Education and Communication (Roberts et al., 2016) by prioritizing not just the development of new agricultural innovations but recognizing the level to which they are adopted (and retained) is just as crucial, and can be improved upon through agricultural education and communication efforts developed using research-driven best practices (e.g., wording and graphics that are tailored to region, race, identity, culture, socioeconomic status, etc.).
Likely Impacts from Successful Completion
The successful completion of this research project is expected to yield several impacts crucial to the adoption of innovations needed to solve agricultural and natural resource issues at the local, regional, national and even global level. Educational programs and communication efforts disseminating information about agricultural innovations will be developed based on best practices identified by research to capitalize on the effects of cognition. Agricultural and extension professionals will have an increased awareness of how cognition influences the adoption and critical thinking/problem-solving skills within their audiences and scientists will have a better understanding of how their audiences’ cognition affects the acceptance of their innovations. Individuals participating in agricultural and extension education programs designed using best practices capitalizing on the effects of cognition will be more likely to process the information and increase their knowledge levels; thereby becoming more informed. Informed individuals will encourage and support the adoption of new innovations. Increased adoption will lead to agricultural systems that ensure the sustainability of natural resources.
Related, Current and Previous Work
A search of the NIMSS system revealed one active multistate project related to decision making and a number related to specific agriculture and natural resource issues. NEERA1501: University-community intermediaries: Supporting informed decision-making around polarized issues focuses on decision making around polarized issues, specifically the theory and practice of university/local government engagement. Those related to agricultural education--W2006: Multistate agricultural literacy research and S1071: A framework for secondary schools agriscience education programs that emphasizes the STEM content in agriculture--focus on formal secondary and higher education. This project would augment the practical application of findings from W2006 and S1071, and recruit members from each.
Research in the discipline has focused on the influences of cognitive styles on teaching and learning, specifically its influence on decision making, for quite some time (Witkinet al., 1977). Educational literature overwhelmingly conveys the importance of cognitive styles of students as an important function of their everyday lives (Witkin et al., 1977; Thomas, 1992; Torres & Cano, 1995; Parr & Edwards, 2004; Myers & Dyer, 2006). Keefe (1979) defined cognitive style as a learner’s preferred way of organizing and retaining information. Within the realm of cognitive styles, critical thinking style has been recurrently identified as necessary for students in the 21st century and crucial for individuals to be able to deal with decisions faced every day (Myers & Dyer, 2006; Torres & Cano, 1995). Critical thinking and problem-solving skills have also been identified as essential for employment, particularly in technical areas and the agricultural industry (Alstonet al., 2009; Graham, 2001; Robinson & Garton, 2008; Robinson et al., 2007). Gokhale (1995) stated that skills associated with critical thinking and problem solving are highly desired due to the highly structured components of today's society.
Critical thinking is an area that can be challenging to define and there are many definitions available. Rudd et al. (2000) defined critical thinking as “a reasoned, purposive, and introspective approach to solving problems or addressing questions with incomplete evidence and information, and for which an incontrovertible solution is unlikely” (p. 5). An individual’s critical thinking ability is essential to their success; however, it should be noted that ability changes constantly as the student continues to learn and grow (Lamm et al., 2011; Irani et al., 2007). Further, critical thinking has been conveyed as one of the most important cognitive traits that leads to a person’s success (Rudd et al., 2000; Irani et al., 2007; Lamm et al., 2012). Irani et al. (2007) utilized critical thinking dispositions to help identify the deeper preferences that affect the way individuals critically think rather than ability as the unit of measurement. These measurements can help educators assess students’ abilities and then develop a tailored educational curriculum to meet the needs of their particular students (Lamm et al., 2011).
Along with critical thinking, problem solving style and learning style are the primary cognitive styles being examined within the field of education and of special interest to agricultural educators. Much like critical thinking, problem solving has also been a challenging area to define. Anderson (1980) defined problem solving as “any goal-directed sequence of cognitive operation” (p. 257). In more recent literature, problem solving has been defined “as one's ability to solve critical, complex problems in challenging environments” (Kirton, 2003, p.1). Jonassen (2000) added that in order for problem-solving to occur there must be social, cultural, and intellectual value. With that being said, in order for a problem to be solved there must be an individual that believes that there is worth in finding and solving the unknown (i.e. problem). However, if no individual sees worth in finding the unknown then there should be no problem that needs solved. Jonassen (2000) stated that “finding the unknown is the process of problem solving” (p. 65). Kirton’s Adaption-Innovation Theory (2003) identified individual cognitive styles based on a continuum ranging from more adaptive to more innovative. According to this Theory individuals whose tendencies were more adaptive prefer a more structured environment when solving problems. However, individuals whose tendencies were more innovative preferred less structure to solve the problem (Lamm et al., 2011; Kirton, 2003). Often, those individuals falling more on the innovator side tend to be novel and find different means to solve problems. However, adaptors tend to be safer, more predictable, conforming, and less ambiguous when solving problems (Kirton, 1999, 2003). The qualities between adaptors and innovators are quite different, as are there perceptions of each other (Kirton, 2003). However, because of different individual cognitive learning styles, the approach to critical thinking and problem solving are often quite different (Kirton, 2003).
While it is known that there are a number of individual factors that influence a student’s cognitive development, individual learning styles are factors that can play a large role on performance during problem solving and critical thinking (Dunn et al., 1979; Claxton & Murrell, 1987). Gregorc (1979) described learning style as “consisting of distinctive behaviors which serve as indicators of how a person learns from and adapts to his/her environment. It also gives clues as to how a person’s mind operates” (p. 234). Previous literature has also revealed that learning style could be an extremely important element in improving the teaching and learning process (Claxton & Murrell, 1987; Dunn et al., 1979; Fisher & Grant, 1983; Miller, 1989; Pickford, 1988; Thomas, 1992). Researchers in agricultural education are rapidly increasing the amount of research and education focused on understanding and utilizing cognitive function in an attempt to improve educational programs (Boone, 1990; Cano, 1993; Dyer & Osborne, 1996a; Gay et al., 2015; Parr & Edwards, 2004) and improve the integration of cultural perspectives (Bunch et al., 2015; Cater et al., 2016; Danjean et al., 2012014, 2015; McClure et al., 2014; Sandlin, 2015; Sandlin et al., 2013).
A variety of research examining how critical thinking style, problem solving style, and learning style as individual concepts impact decision making, specifically in learning environments, has been conducted within agricultural education (Blackburn & Robinson, 2016; Blackburn, et al., 2014; Boone, 1990; Cano, 1993, 1999; Cano & Martinez, 1991; Dyer & Osborne, 1996a, 1996b; Garton et al., 1999; Parr & Edwards, 2004; Rudd et al., 1998; Sandlin & Price, 2016; Torres & Cano, 1994). Dyer and Osborne (1996b) studied the effect of teaching approach on problem solving ability of students with varying learning styles. The results from this study indicated those students who were taught by the problem-solving approach produced significantly higher problem solving ability than those taught by the subject matter approach. Also, no significant differences were present between pretest and posttest problem solving ability scores of students with the same learning style. However, students of different learning styles benefited from the instruction using the problem-solving approach. Similarly, Torres and Cano (1994) found that learning style has an effect on the learning success of students in specific kinds of situations and different types of learning environments (i.e. labs). Recently, Blackburn et al. (2014) conducted a study to determine the effects of cognitive style and problem solving complexity. The findings from this study also found a statistically significant effect existed between cognitive style and problem complexity.
In addition, researchers have examined how critical thinking disposition and problem-solving style may be linked through creative thinking. Studies have identified creative thinking as an essential part of critical thinking (Maltzman, 1960; Newell et al., 1962; Russell, 1956; Torrance & Torrance, 1973; Vinacke, 1952). At the same time, there is some debate as to whether creative thinking and problem solving are significantly different concepts. Kirton (2003) argued that problem solving style does not differentiate whether an individual is creative or not, but rather the differences in the way they express their creativity. Friedel et al. (2008) found low levels of correlation between critical thinking and problem solving, but concluded the two are probably more independent than previously thought and may be impacting decision making separately. An understanding of how these two concepts interrelate around decision making is still unanswered.
Cognitive relationships between critical thinking style and learning style have also been explored (Friedel et al., 2008; Myers & Dyer, 2006; Lamm et al., 2011; Rudd et al., 2000; Torres & Cano, 1995). While studying these relationships in undergraduate students, Rudd et al. (2000) reported no significant correlation between learning style and critical thinking disposition. Torres and Cano (1995) also expressed the need for further study when they discovered learning style only accounted for 9% of the variance in critical thinking ability. Lamm et al. (2011), however, found a relationship between learning style and critical thinking disposition; while overall problem solving style scores and learning style scores were not strongly related, connections between constructs within the styles were uncovered and found to be significant. The finding leaves open a question of how these two concepts work independently and collaboratively in influencing decision making.
Further, cognitive relationships between problem solving and learning style have been examined in agricultural education (Blackburn & Robinson, 2016; Blackburn et al., 2014; Torres & Cano, 1994; Dyer & Osborne, 1996a; Parr & Edwards, 2004; Friedel et al., 2008; Pate et al., 2004; Lamm et al., 2012).
More recently, researchers have started to focus on how cognitive styles influence team dynamics during the decision-making process in order to enhance educational initiatives, communication strategies, and leadership programming around critical agricultural and natural resource issues. In a recent study, Lamm et al. (2016) found that problem solving style influenced how opinion leaders worked collaboratively in teams while building agendas around critical agricultural and natural resource issues. In this study, the findings suggested that teams representing diverse problem-solving styles enhanced the consensus building process. These results imply that when building teams around critical issues educators should focus on establishing well-structured groups that will allow participants to “share their conceptual and procedural knowledge . . . so that all [participants] are actively engaged in the problem-solving process and differences of opinion are resolved in a reasonable manner” (Heller & Hollabaugh, 1992, p. 637).
While research examining how cognitive styles impact decision making in general is abundant, research focusing on how cognitive styles impact the teaching and learning process and how decisions are made around critical agricultural and natural resources issues has just begun. Therefore, the subject matter area is ripe for further investigation. The researchers believe developing a national teaching and learning decision support system will aid and foster: (a) the widespread stakeholder adoption of new technologies and practices, (b) educators’ integration of holistic perspectives on complex agriculture and natural resources issues into formal and non-formal environments, and (c) cultural consideration in the decision-making process.
Objectives
-
Identify diverse audiences’ problem-solving style, critical thinking style, learning style, and behavioral style. Each multistate research collaborator will identify audiences related to their specific work. Extension educators, in particular, may identify multiple audiences based on their communities of practice.
-
Determine how diverse audiences’ problem-solving style, critical thinking style, learning style, and behavioral style influence decision making regarding the adoption of new innovations in agriculture and natural resources in their region. The multistate research collaborators, particularly the agriculture and extension educators, will participate in the data analyses and/or interpretation to audit the relevance, appropriateness, and validity of the outputs, as they pertain to their identified audiences
-
Determine how--in the aggregate--problem solving style, critical thinking style, learning style, and behavioral style influence decision making regarding the adoption of new innovations in agriculture and natural resources with diverse audiences. The multistate research collaborators, particularly the agriculture and extension educators, will participate in the data analyses and/or interpretation of the results. All research collaborators will engage in the testing of outputs related to this objective.
Methods
Quantitative and qualitative research methods will be used to accomplish the objectives of this multistate project. The researchers involved in this project will determine the most appropriate methods and statistical tools based on targeted research objectives, design, and selected population. The researchers involved in this project will employ three specific data collection protocols (i.e., cognitive assessments, willingness and intention assessments, and eye-tracking system) to meet the three objectives.
Objective one will be assessed by using four different instruments. The first instrument will be the Kirton’s Adaption Innovation Inventory (KAI). The KAI measures an individual’s problem-solving style. The instrument assigns values to their rule/group conformity, efficiency and sufficiency of originality which is then aggregated into an overall score that places an individual on a continuum between innovation and adaption. An innovator is someone who can come up with a lot of ideas, does not conform and is not limited by structure but may not be efficient in solving a complex problem. When their ideas do work, it will create radical change within a system. An adaptor is someone who likes to understand a system and come up with a few succinct ideas that will work within what already exists creating small changes that will lead to larger change over time.
The second instrument, The University of Florida Critical Thinking Inventory (UFCTI), measures critical thinking style on a continuum between engaging and seeking information. Information seekers are hungry learners and open to opinions of others. They take an objective view to look at things and make decisions. Engagers anticipate and look for situations where good reasoning will be needed. They are confident in their ability to reason and are good at explaining their critical thinking process.
The third instrument to be used by the researchers is Kolb’s learning style inventory (LSI). The LSI is designed to measure the level to which an individual displays their preferred learning style. Kolb has identified four different learning styles, (1) diverging, (2) assimilating, (3) converging, and (4) accommodating. Diverging learners are best at viewing concrete situations with several viewpoints. The assimilating learner prefers to take a wide range of information and covert the information into succinct logical form. The converging learners are best at taking information in and making practical and useful sense of the information. Lastly, the accommodating learners learn predominantly from hands-on experience. This instrument will allow the researchers to determine an individual’s learning style.
The fourth instrument to be used is the DISC Behavioral Style Assessment (DISC). The DISC measures an individual’s behavioral style. It categorizes behavior into four dimensions: Dominance (D); Influence (I); Steadiness (S); and Compliance (C). The dimensions are measured on a continuum from low to high expression of each dimension’s characteristics. An individual’s behavioral profile is the combination of an individual’s four dimensions; the letters are listed in descending order of the dimension scores (Bonnstetter & Suiter, 2011). While individuals may have the same behavioral profiles (order of letters), each profile is unique in terms of expression level in each of the dimensions, as well as characteristics not measured by this instrument (e.g., personality, culture, environmental conditions, life events, etc.).
These instruments used for objective one will be provided in two forms, paper questionnaires and online questionnaires depending on researcher inclination of best practices for data collection given their chosen population. Dr. J.C. Bunch (University of Florida), Dr. Alexa Lamm (University of Florida), Dr. Joey Blackburn (Louisiana State University), Dr. M’Randa Sandlin (University of Hawaii), Dr. Rochelle Sapp (University of Georgia), Dr. Summer Odom (Texas A&M University), Dr. Sandra Thompson (Florida A&M University) and Dr. Tre Easterly (University of Florida) will lead efforts on objective one and provide other multistate group members with questionnaires. Populations to be assessed will be defined by factors such as formal education, non-formal education, commodity group, government official, and general public. Each member of the multistate research group will be responsible for recruiting targeted populations.
Objective two will be assessed through the development of several quantitative instruments to measure individuals’ willingness and intention to adopt new innovations. The scales included in these instruments will be semantic differential scales, social distance scales, Likert-type scales, and ranking scales. Questions developed for these instruments will be based on a thorough review of relevant literature and consultation with expert panels of researchers in agricultural education, agricultural communications, Extension education, and leadership education. Once instruments are developed to measure willingness and intention to adopt new innovations, the multistate research group will analyze data collected in objective one with objective two to determine how diverse audiences’ problem-solving style, critical thinking style, learning style, and behavioral style influence decision making regarding the adoption of new innovations in agriculture and natural resources. In addition to using willingness and intention instruments to measure the influence cognition has on innovation adoption, biometrics will be used to further understand cognitive processing and behavior. Biometric measures, like eye-tracking, allow us to understand where an individual looks (fixations) and how long they look (fixation duration) among other behaviors to offer insight into cognition and information processing. Increased frequency of fixations and fixation duration are indicators of higher cognitive load and processing. This project will utilize a goggle-based eye-tracking unit and a screen-based unit to track foveal fixations and fixation duration as measures of cognitive processing and visual literacy. Visual Literacy is a teachable and learnable cognitive skill set that includes processing and understanding images and other forms of visual communications. Therefore, as the team investigates decision making and adoption of new technologies, understanding how they process visual information related to these decisions and innovations is of increasing importance in defining the totality of cognition. Biometric data will be complemented by quantitative performance measures like the Visual Literacy Index (VLI) and researcher-developed qualitative protocols to assess intention and recall. This effort will be led by Dr. Tobin Redwine (Texas A&M University), Dr. J.C. Bunch (University of Florida) and Dr. Nicole Stedman (University of Florida) because they have the technology available.
Objective three will be accomplished by multistate group members sharing data collected from chosen populations. Data will be aggregated and matched based on populations from across the nation. The aggregated data will allow the multistate group to build large data sets to better generalize the influence cognition has on decision making regarding the adoption of new innovations in agriculture and natural resources. Further, data specific to identified cultural characteristics will be analyzed to determine if there are considerations that must be taken into account when creating educational programs or communication efforts about agriculture and natural resource issues. Ultimately, the aggregated data will be used to create a teaching and learning decision support system to aid in (a) the identification and stakeholder implementation of new technologies and practices, (b) the ability to integrate holistic perspectives on agriculture and natural resources issues into formal and non-formal educational programs, and (c) to ensure cultural consideration in the decision-making process. The teaching and learning decision support system will be tested by the multistate collaborators in their respective contexts with their respective audiences. This effort will be led by Dr. J.C. Bunch (University of Florida), Dr. John Diaz (University of Florida), Dr. Joey Blackburn (Louisiana State University), Dr. Rochelle Sapp (University of Georgia), Dr. Kristi Farner (University of Georgia), Dr. Tobin Redwine (Texas A&M University), and Dr. Kim Dooley (Texas A&M University). The outputs from this objective will be representative of the data collected from all of the multistate group members and will be a product of their collaborative efforts. Because the group members of this project are from multiple regions, there are regional and national implications for the project outputs and outcomes.
Measurement of Progress and Results
Outputs
- Analyzed problem-solving style, critical thinking style, learning style, and behavioral style assessment results (Obj. 1)
- Validated educational and communication materials based on identified best practices (Obj. 1 & 2)
- Publication of quantitative instruments measuring individuals’ willingness and intention to adopt new innovations (Obj. 2)
- Workshops, webinars, e-learning materials and other delivery methods to disseminate findings to agricultural education, extension, and scientists (Obj. 2 & 3)
- Publication of research and practice implications based on biometric data (Obj. 2 & 3)
- Publication of a teaching and learning decision support system to aid in stakeholder implementation of new technologies and practices, the ability to integrate systems perspectives into educational programs, and to ensure cultural consideration in the decision making process (Obj. 3)
- Series of reports reflecting recommended practices resulting from project efforts (Obj. 1, 2, & 3)
- Public website that includes related materials and results (Obj. 1, 2, & 3)
Outcomes or Projected Impacts
- Agricultural and extension professionals will have an increased awareness of how cognition influences the adoption and critical thinking/problem-solving skills within their audiences and scientists will have a better understanding of how their audiences’ cognition affects the acceptance of their innovations (Obj. 1, 2, & 3). This project will identify best practices in education and information dissemination as they pertain to target audiences’ cognitive characteristics. As a result, the project will develop a teaching and learning decision support system to aid educators and scientists in their efforts.
- Modified agricultural and extension education approaches and materials (Obj. 1, 2, & 3). The identified best practices in education and information dissemination will be developed into educational and communication lessons and materials. These will enhance focus on holistic perspectives on agriculture and natural resources issues into formal and non-formal educational program. Content and materials will be made available through workshops, webinars, e-learning materials and other delivery methods. A public website will also be created to share the information.
- Increased educator and scientist effectiveness with target audiences (Obj. 2 & 3). Identified best practices can be used to guide development in these areas. The application of these principles will hopefully lead to similar results for those that implement the practices. Further research projects are planned to assess the success of the implementation of these best practices.
- Increased ability of target audiences’ ability to process the information presented at programs designed using best practices capitalizing on the effect of cognition (Obj. 2 & 3). While the best practices are geared to aid educators present information in a cognitively appropriate method for their target audience, this project also has implication for the target audience’s ability to better process the information and, therefore, increase knowledge and application skills.
- Long term - Informed individuals will encourage and support the adoption of new innovations. Increased adoption will lead to agricultural systems that ensure the sustainability of natural resources (Obj. 2 & 3). With increased knowledge and abilities in agriculture and natural resources, informed individuals from target audiences can become change agents in their communities and sphere of influence. Effective education of these individuals can impact the long-term sustainability of agriculture and natural resources.
- Long term - Increased awareness and ability for educators, extension professionals, and scientists to consider cultural influences in the decision making process (Obj. 3). By sampling from diverse audiences, the researchers will be able to analyze culture-specific information and make recommendations based on the findings. This increased awareness will allow for larger, more diverse audiences to be reached with agriculture and natural resource programs.
Milestones
(2019):Identification and recruitment of diverse audiences as identified by members of the multistate research group (Obj. 1). Distribution of problem-solving style, critical thinking style, learning style, and behavioral style assessments for implementation with identified audiences (Obj. 1).(2020):Analyze and aggregate problem-solving style, critical thinking style, learning style, and behavioral style assessment data (Obj. 1 & 2). Develop educational and communication content and materials to disseminate findings to agricultural education, extension, and scientists (Obj. 1 & 2). Test developed materials using biometric measurements (Obj. 2 & 3).
(2021):Continue measuring problem-solving style, critical thinking style, learning style, and behavioral style of identified audiences to strengthen data set (Obj. 1 & 2). Continue developing (Obj. 1 & 2) and testing (Obj. 2 & 3) educational and communication content and materials to disseminate findings to agricultural education, extension, and scientists Develop a public website for knowledge management and the sharing of information and data (Obj. 1, 2, & 3). Conduct workshops webinars, e-learning materials, and other delivery methods to disseminate findings/best practices to agricultural education, extension, and scientists (Obj. 2 & 3).
(2022):Continue conducting workshops, webinars, e-learning materials, and other delivery methods to disseminate findings/best practices to agricultural education, extension, and scientists (Obj. 2 & 3). Draft teaching and learning decision support system (Obj. 3)
(2023):Release teaching and learning decision support system (Obj. 3)
Projected Participation
View Appendix E: ParticipationOutreach Plan
The project’s results will be disseminated to interested stakeholders through the project’s website, presentations at research conferences (e.g., American Association of Agricultural Education, NACTA, Association for International Agricultural and Extension Education), publication in research journals (e.g., Journal of Agricultural Education, Journal of Extension), and local extension publications for agriculture industry partners, technical reports, and workshop delivery for researchers and practitioners. Relationships with preservice teacher preparation programs and extension education programs will be sought in the construction and dissemination of project outputs. Professional development opportunities will be designed and delivered at preservice teacher preparation institutions, identified extension education venues, and at conferences that target both of these audiences. Input from these audiences will guide the development of the outreach plan to ensure the dissemination method/approaches best meet the needs of those who will use the information and products. Members of the project team have strong current relationships with these audiences. These relationships will be used to leverage opportunities to share the information and outputs of this project with the target audiences.
We have identified three primary categories of stakeholders: (a) the community of agriculture education professionals; (b) the community of extension educators; and (c) the community of agriculture and natural resource scientists who create new technologies in these fields. Our goal for (a) and (b) is to create a community engaged with every aspect of the work. Category (a) will focus primarily on formal education contexts and category (b) will focus primarily on non-formal education contexts. Our goal for (c) is to involve them less intensively but in the same ways as categories (a) and (b) but with a more purposeful focus on the creation and usability of the created content/materials.
In each of the states involved in this multistate research project, the land grant system reaches into a variety of communities that represent their own unique blend of diverse demographics. As agriculture and natural resource information and technologies are diffused into these communities, local diversity will influence the context and outcomes of these efforts. Our focus is on supporting those educators and agents to effectively navigate these cultural landscapes.
Organization/Governance
An executive committee consisting of a chair, chair-elect, and a secretary will be established to maintain the direction this project. The past-chair will also be considered a part of the executive committee. All executive committee members will be elected for two-year terms to provide continuity. Ad hoc committees will be appointed for specific functions as they arise. The executive committee will meet quarterly (either face-to-face or via conference call) to assess progress. An annual meeting of all participants will be held.
Literature Cited
Aerts, S., De Tavernier, J., Decuypere, E., & Lips, D. (2009). The 6 F’s of agriculture. In: Miller, K., & Nerlich, B. (Eds.). Ethical futures: bioscience and food horizons. Wageningen Academic Publishers Wageningen, Netherlands.
Alston, A. J., Cromartie, D., Wakefield, D., & English, W. C. (2009). The importance of employability skills are perceived by the employers of United States’ land-grant college and university graduates. Journal of Southern Agricultural Education Research, 59, 56-69. Retrieved from http://www.jsaer.org/pdf/vol59Whole.pdf
Anderson, J. R. (1980). Cognitive psychology and its implications. New York: Freeman.
Boone, H. N. (1990). Effect of level of problem solving approach to teaching on student achievement and retention. Journal of Agricultural Education, 31(1), 18–26. doi: 10.5032/jae.1990.01018
Blackburn, J. J., & Robinson, J. S. (2016). Determining the effects of cognitive style, problem complexity, and hypothesis generation on the problem solving ability of school-based agricultural education students. Journal of Agricultural Education, 57(2), 46–59. doi: 10.5032/jae.2016.02046
Blackburn, J. J., Robinson, S., & Lamm, A. J. (2014). How cognitive style and problem complexity affect preservice agricultural education teachers' abilities to solve problems in agricultural mechanics. Journal of Agricultural Education, 55(4), 133–147. Doi: 10.5032/jae.2014.04133.
Bunch, J. C., Blackburn, J. J., Danjean, S. E., Stair, K. S., & Blanchard, L. D. (2015). Examining Louisiana State University College of Agriculture students’ perceived motivators and barriers to participation in international experiences. Journal of International Agricultural and Extension Education, 22(3). doi:10.5191/jiaee.2015.22305
Cano, J. (1993). An assessment of the level of cognitive performance and critical thinking ability of selected agricultural education students. Journal of Agricultural Education, 34(2), 25–30. doi: 10.5032/jae.1993.02025
Cano, J. (1999). The relationship between learning style, academic major, and academic performance of college students. Journal of Agricultural Education, 40(1), 30–37. doi: 10.5032/jae.1999.01030
Cano, J., & Martinez, C. (1991). The relationship between cognitive performance and critical thinking abilities among selected agricultural education students. Journal of Agricultural Education, 32(1), 24–29. doi: 10.5032/jae.1991.01024
Cater, M., Bunch, J. C., & Danjean, S. E. (2016). Assessing the prior intercultural experiences, motivating factors and deterrents influencing 4-H members’ intention to participate an international experience program. Journal of International Agricultural and Extension Education, 23(2). DOI: 10.5191/jiaee.23202
Claxton, C. S., & Murrell, P. H. (1987). Learning Styles: Implications for Improving Educational Practices. ASHE-ERIC Higher Education Report No. 4, 1987. Association for the Study of Higher Education, 1 Dupont Circle, Suite 630, Washington, DC 20036.
Danjean, S., Bunch, J. C., & Blackburn, J. J. (2015). Examining the motivations and barriers influencing the decisions of Louisiana State University College of Agriculture freshmen to participate in international experiences. Journal of International Agricultural and Extension Education, 22(1), 49–62. doi: 10.5191/jiaee.2015.22104
Danjean, S., McClure, C., Bunch, J. C., Kotrlik, J. W., & Machtmes, K. (2014). Louisiana secondary agricultural educators' perceptions of an international experience toward their teaching career. Journal of Agricultural Education, 55(2), 1–15. doi: 10.5032/jae.2014.02001
Dunn, R. S., Dunn, K. J., & Price, G. E. (1979). Learning style inventory. Lawrence, KS: Price Systems.
Dyer, J. E., & Osborne, E. W. (1996a). Effects of teaching approach on achievement of agricultural education students with varying learning styles. Journal of Agricultural Education, 37(3), 43–51. doi: 10.5032/jae.1996.03043
Dyer, J. E., & Osborne, E. W. (1996b). Effects of teaching approach on problem solving ability of agricultural education students with varying learning styles. Journal of Agricultural Education, 37(4), 38–45. doi: 10.5032/jae.1996.04038
Epstein, D., & Graham, J. D. (2007). Polarized politics and policy consequences. Rand Corporation, Pittsburgh, PA.
Friedel, C. R., Irani, T. A., Rhoades, E. B., Fuhrman, N. E., & Gallo, M. (2008). It’s in the genes: Exploring relationships between critical thinking and problem solving in undergraduate agriscience students’ solutions to problems in mendelian genetics. Journal of Agricultural Education, 49(4), 25–37. doi: 10.5032/jae.2008.04025
Garton, B. L., Spain, J. M., Lamberson, W. R., & Spiers, D. E. (1999). Learning styles, teaching performance and student achievement: A relational study. Journal of Agricultural Education, 40(3), 11–20. doi: 10.5032/jae.1999.03011
Gay, K., Terry, B., & Lamm, A. J. (2015). Identifying critical thinking style to enhance volunteer development. Journal of Extension, 53(6): 6TOT2. Retrieved from http://www.joe.org/joe/2015december/tt2.php
Gokhale, A. A. (1995). Collaborative learning enhances critical thinking. Journal of Technology Education, 7(1), 22-30.
Graham, D. L. (2001). Employer perception of agricultural and extension education graduates. Journal of Southern Agricultural Education Research, 51(1), 88-101. Retrieved from http://www.jsaer.org/pdf/Vol51/51-00-088.pdf
Gregorc, A. F. (1979). Learning/teaching styles: Their nature and effects. Student learning styles: Diagnosing and prescribing programs, 19-26.
Heller, P., & Hollabaugh, M. (1992). Teaching problem solving through cooperative grouping, part 2: Designing problems and structuring groups. American Journal of Physics, 60(7), 637–644. Doi: 10.119/1.17117.
Irani, T., Rudd, R., Gallo, M., Ricketts, J., Friedel, C., & Rhoades, E. (2007). Critical thinking instrumentation manual. Gainesville: University of Florida. Retrieved from http://step.ufl.edu/resources/critical_thinking/ctmanual.pdf
Jonassen, D. H. (2000). Toward a design theory of problem solving. Educational Technology: Research and Development, 48(4), 63-85. Retrieved from http://link.springer.come/article/10.007%2FBF02300500LI=true#page-1
Keefe, J. W. (1979). Learning style: An overview. In NASSP's student learning styles, Diagnosing and prescribing programs (pp. 1–17). Reston, VA: National Association of Secondary School Principals.
Kirton, M. J. (1999). Kirton adaption-innovation inventory feedback booklet. Newmarket, Suffolk, UK: Occupational Research Center.
Kirton, M. J. (2003). Adaption-Innovation: In the context of diversity and change. New York, NY: Routledge.
Lamm, A. J., Carter, H., Settle, Q., & Odera, E. (2016). The influence of problem solving style on team dynamics when building consensus. Journal of Human Sciences and Extension, 4(1), 18–33. Retrieved from http://www.jhseonline.com/#!current-issue-1/yg31h
Lamm, A. J., Rhoades, E., Irani, T., Roberts, T. G., Snyder, L., & Brendemuhl, J. (2011). Utilizing natural cognitive tendencies to enhance agricultural education programs. Journal of Agricultural Education, 52(2), 12–23. doi: 10.5032/jae.2011.02012
Lamm, A. J., Shoulders, C., Roberts, T. G., Irani, T. A., Unruh, L. J., & Brendemuhl, J. (2012). The influence of cognitive diversity on group problem solving strategy. Journal of Agricultural Education, 53(1), 18–30. doi: 10.5032/jae.2012.01018
Maltzman, I. (1960). On the training of originality. Psychological Review, 38, 332–346.
McClure, C., Danjean, S. E., Bunch, J. C., Machtmes, K., & Kotrlik, J. W. (2014). Louisiana extension educators’ perceptions of the benefit and relevance of participating in an international extension experience toward their career. Journal of Agricultural Education, 55(1), 92–105. Doi: 10.5032/jae.2014.01092
Myers, B. E., & Dyer, J. E. (2006). The influence of student learning style on critical thinking skill. Journal of Agricultural Education, 47(1), 43–52. doi: 10.5032/jae.2006.01043
Newell, A., Shaw, J. C., & Simon, H. A. (1962). The process of creative thinking. New York, NY: Atherton.
Pate, M. L., Wardlow, G. W., & Johnson, D. M. (2004). Effects of thinking aloud pair problem solving on the troubleshooting performance of undergraduate agriculture students in a power technology course. Journal of Agricultural Education, 45(4), 1–11. doi: 10.5032/jae.2004.04001
Parr, B., & Edwards, M. C. (2004). Inquiry-based instruction in secondary agricultural education: Problem solving – an old friend revisited. Journal of Agricultural Education, 45(4), 106–113. doi: 10.5032/jae.2004.04106
Roberts, T. G., Harder, A., & Brashears, M. T. (2016). American Association for Agricultural Education national research agenda: 2016-2020. Gainesville: Department of Agricultural Education and Communication, University of Florida.
Robinson, J. S., & Garton, B. L. (2008). An assessment of the employability skills needed by graduates in the college of agriculture, food, and natural resources at the University of Missouri. Journal of Agricultural Education, 49(4), 96–105. doi: 10.5032/jae.2008.04096
Robinson, J. S., Garton, B. L., & Terry, Jr., R. (2007). Identifying the employability skills needed in the workplace according to supervisors of college of agriculture, food, and natural resources graduates. Journal of Southern Agricultural Education Research, 57(1): 1-15. Retrieved from http://www.jsaer.org/pdf/Vol57/57-01-095.pdf
Rudd, R. D., Baker, M., & Hoover, T. (1998). Student and faculty learning styles within academic units in the University of Florida's College of Agriculture. NACTA Journal, 42(3), 18–24.
Rudd, R. D., Baker, M., & Hoover, T. (2000). Undergraduate agriculture student learning styles and critical thinking abilities: Is there a relationship? Journal of Agricultural Education, 41(3), 2–12. doi: 10.5032/jae.2000.03002
Russel, D. (1956). Children’s thinking. New York, NY: Ginn and Co.
Sandlin, M. R. (2015). The agricultural industry in Trinidad and Tobago: Perspectives of industry professionals. Journal of International Agricultural and Extension Education, 22(2).: 6-19. doi:10.5191/jiaee.2015.22201
Sandlin, M. R., Murphrey, T. P., Lindner, J. R., & Dooley, K. E. (2013). Impacts of a faculty abroad experience on teaching style and technology use in a College of Agriculture and Life Sciences. Journal of Agricultural Education, 54(3), 186–197. doi: 10.5032/jae.2013.03186
Sandlin, M. R., & Price, M. (2016). Planning for success: Student preflection on the impacts of behavioral styles on the success of a course project. Abstract presented at the 2016 NACTA Conference, Honolulu, HI.
Schunk, D. H. (2012). Learning theories an educational perspective sixth edition. Pearson.
Sivan, E. (1986). Motivation in social constructivist theory. Educational Psychologist, 21(3), 209-233.
Thomas, R. G. (1992). Cognitive Theory-Based Teaching and Learning in Vocational Education. Information Series No. 349. ERIC Institute of Education Sciences.
Torrance, E. P., & Torrance, P. (1973). Is creativity teachable? Bloomington, IN: Phi Delta Kappa.[SL1]
Torres, R. M., & Cano, J. (1994). Learning styles of students in a College of Agriculture. Journal of Agricultural Education, 35(4), 61–66. doi: 10.5032/jae.1994.04061
Torres, R. M., & Cano, J. (1995). Critical thinking as influenced by learning style. Journal of Agricultural Education, 36(4). 55–62. doi: 10.5032/jae.1995.04055
Vinacke, W. E. (1952). The psychology of thinking. New York, NY: McGraw-Hill.
Witkin, H. A., Moore, C. A., Goodenough, D. R., & Cox, P. W. (1977). Field-dependent and field-independent cognitive styles and their educational implications. Review of Educational Research, 47(1), 1–64.
U.S. Department of Agriculture, National Institute of Food and Agriculture. (n. d.). Challenge areas. Retrieved from https://nifa.usda.gov/challenge-areas