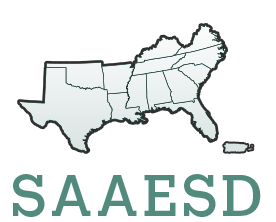
OLD S1069: Research and Extension for Unmanned Aircraft Systems (UAS) Applications in U.S. Agriculture and Natural Resources
(Multistate Research Project)
Status: Inactive/Terminating
OLD S1069: Research and Extension for Unmanned Aircraft Systems (UAS) Applications in U.S. Agriculture and Natural Resources
Duration: 10/01/2016 to 09/30/2021
Administrative Advisor(s):
NIFA Reps:
Non-Technical Summary
Statement of Issues and Justification
There is currently great interest in developing unmanned aerial systems (UAS) applications to benefit agricultural production and research. For this endeavor to be successful, it is essential that research and extension/education be conducted (a) to clarify requirements related to data quality and quantity, (b) to discover and develop common practices and methodologies that are consistently useful for a given crop, and (c) to discover and make known capabilities and limitations related to detection of stresses in crops with UAS remote sensing.
Determining appropriate data resolutions for UAS-data collection is of particular importance for accuracy, usefulness, and efficiency. Optimal resolutions vary by application and can differ by region and production practice. Key factors that need to be identified include spatial resolution, spectral bands, timing and re-visit, and post-processing necessary to produce answers within an acceptable timeframe for a decision by a producer.
Currently, practical applications for producers and service providers are limited. A wide range of platforms and sensors are available, but utility is not well documented in research literature; at the same time, the rapid pace of UAS development provides new opportunities and challenges for testing, calibration, and validation of these systems. Cost-effective, crop-specific processes and workflows that are robust across regions must be identified, tested, and evaluated for their ability to enable decision making in production agriculture.
It would be extremely valuable to agricultural producers and natural resource managers if UAS methods were available for identifying specific causal agents of plant stress, rather than just confirming its existence. A great deal of research is needed to determine stress-detection effectiveness of current and developing sensor technology as it is used from the UAS platform.
Finally, there is a need to increase capacity and materials for the transfer of critical information and developments to the agricultural production, crop improvement, and natural resources communities. Potential users need a better understanding of the capabilities of modern UAS platforms and the sensors they carry, as well as how to effectively apply these to specific applications. At the same time, extension and outreach educators need a better understanding of what information is required and how best to facilitate information to constituent groups they serve.
Related, Current and Previous Work
Unmanned aircraft systems (UAS) based remote sensing has potential to be useful at a variety of scales; from research studies working with small plots to providing actionable information in large field operations for growers. Much work has been done on the science behind remote sensing of crops, although studies have used manned aircraft or satellite imagery and thus may not be directly applicable at the extremely high-resolution, low altitude, frequent re-visit scales that UAS enable (Zhang and Kovacs 2012). Methods and approaches for different field scales, different crops, and different measurements are expected to have different requirements and challenges and need to be evaluated on a case-by-case basis. Most studies using UAS to date have looked at a single field, a single crop and a single physiological phenotype or stress factor to evaluate the technology. The requirements and challenges of using UAS in agriculture are not well understood, and there has thus far been little coordination between different research groups in addressing them.
At present, UAS methods are application-specific, requiring translation and calibration between crops and climates (Ishimwe et al. 2014), as well as varying light conditions, soil conditions (Muller and Décamps 2001), crop moisture (Carter 1991), etc. This matter is further complicated by observed differences in sensors and payloads (von Bueren et al. 2015). These factors combine to make development of universally repeatable routines difficult. In light of this fact, research is needed to determine the appropriate data collection protocols for targeting imaging efforts. Key factors to be identified include spatial resolution, spectral bands, timing and re-visit, and post-processing necessary to produce answers within an acceptable timeframe for a decision by a producer. These factors should also be investigated for geographic and climatological variability. This information is necessary if turn-key solutions will ever be possible for producers.
Currently Used Remote Sensing Technologies
Currently there are a number of sensing technologies being used by growers in the field. Tractor-mounted infared (IR), near-IR (NIR), and red, green, and blue (RGB) sensors were recently used to improve the efficiency of field evaluations (Munns et al., 2010), but the time required to take these measurements is too long. Stationary wireless IR sensors (O’Shaughnessy, 2011) can provide real-time data on crop conditions, but these data are limited by the cost of the large number of sensors needed for hundreds of plots. Manned-aerial IR imagery measuring canopy temperature depression (CTD) has been shown to be a good method ifor screening wheat varieties for yield in droughty conditions, but aircraft flying at 800 m above plots have encountered problems like intermittent cloud cover and low resolution (Reynolds et al., 1999). Modern field evaluations can benefit from low-level aerial imaging platforms that are increasingly available and affordable.
Canopy imaging to improve crop yield can be advanced by UAS imagery, allowing more efficient data capture over the course of the growing season and potentially at several times during the day for large numbers of plots. Algorithms have been developed and successfully tested on wheat and other crops to estimate biomass, leaf area index (LAI), and yield from RGB image indices (Casadesus and Villegas, 2014; Kefauver et al., 2015; Balota and Oakes, 2016). Images can be taken at the ground or from UAS platforms (Casadesus and Villegas, 2014) and used not just for selection of phenotypes in breeding programs, but also in genomic applications (Condon et al., 2013).
Several new sensor-based methods are now available for the detection, identification, and quantification of various types of plant and ecosystem stresses (Hillnhutter et al. 2010; Mahlein 2016; Mahlein et al. 2012; Sankaran et al. 2010; and West et al. 2003, 2010). Current sensor-based methods rely mainly on optical properties of plants and soil within different wavelengths of the electromagnetic spectrum, with particular emphasis on use of wavelengths outside the visible range. These sensors include multispectral, hyperspectral, thermal, chlorophyll-flourescence, and three-dimensional imaging systems. Multispectral and hyperspectral sensor systems assess the spectral information of objects in the red, green, blue and infrared bands of the spectrum, or in the wavelengths of 350 to 2500 nm. Space-based, or airborne multispectral or hyperspectral systems are currently suitable for the detection of large areas (field scale or sub-field scale) that may be stressed due to diseases and other factors (Hillnhutter et al. 2011). However, additional research is needed to develop the sensing methodologies and data processing algorithms for high spatial and temporal resolution,. Thermal infrared sensors are able to assess plant temperature, which is correlated with plant water status, crop microclimate, and changes in transpiration due to developing stress in the plant (Jones et al. 2002; Lenthe et al 2007; and Oerke et al 2006). Flourescence imaging has been used to study differences in photosynthetic activity caused by biotic and abiotic stresses over the leaf area (Burling et al. 2011; Scholes and Rolfe 2009). Current disadvantages of fluorescence imaging is that it is difficult to implement in a field setting, therefore, additional research is needed to determine if solar reflectance in the field can be used to induce the appropriate fluorescence parameters (Rossini et al. 2014).
Use of UAS in High-throughput Field-based Phenotyping (HTFBP)
In contrast to monitoring phenomena at the field-scale, the use of UAS in agricultural small plot research to measure the effects of genetics and management treatments in crops is promising. High-throughput phenotyping with UAS, in which field researchers use imaging technology to make phenotypic measurements, is of emerging interest (Anthony et al. 2014; Aruas and Cairnes 2014; White et al. 2012). The measurements of primary interest to field researchers are two-fold; to automate phenotypes already collected in the field manually, and to develop new phenotypes that may be useful in improving yield or illuminating biological processes. Variety choice is also the most important production decision farmers make, because a good variety can significantly increase farmer profits with no additional production costs. The main purpose for breeding programs worldwide is to regularly supply farmers with better varieties. UAS and remote sensing technologies can boost the benefits of breeding and variety selection for the benefit of the farmers. To accelerate the rate of genetic gain, breeding for target traits is needed, because the superiority of trait-based vs yield selection has been documented (Nigam et al., 2005; Reynolds et al., 2009; Passioura, 2012). There are several target traits that breeders can measure to increase the rate of genetic gain.
Plant height is a primary example of a phenotype often measured manually in small plot studies that could be automated through UAS remote sensing. Measuring plant height manually is labor intensive, but it can be automated with more complex measurement systems. Plant height is of practical importance for Texas corn as it is 40% to 60% correlated with yield (Farfan et al. 2013) and likely useful throughout the stressful conditions of the Southern U.S. in an assortment of crops. Plant height is currently measured with a measuring stick at the end of the season before harvest, providing only a terminal growth measurement. Plant height has been measured with moderate to high precision using imaging (Chapman et al. 2014) and LiDAR (Anthony et al. 2014), which can be improved with unmanned aircraft. A new phenotype that could also be measured at high precision with UAS is the temporal changes in plant height growth or biomass (e.g. measuring the maximum height of a plant or plot throughout the growing season and then fitting growth curve models to these values) (Fig. 1; Murray et al. 2016). The different genetic varieties or agronomic treatments could then be compared within experiments for their growth trajectories. It is hypothesized that different abilities of plants to grow tall throughout different periods of stress would be more valuable than the single terminal plant height measurement. New genetics could be developed by crossing varieties that demonstrate different growth patterns resistant to stress at different times.
See attached diagram
Figure 1. Example of temporal plant height measurement (lines) compared to terminal height measurement (circle and inset table). This approach will allow identification of genetic and agronomic growth pattern variation and/ or stress resistance (Figure from Murray et al. 2016).
In addition to its use for plant height, plant structure imaging could be used to look at biomass of small plots, or predict yield of small research plots in wheat, sorghum, cotton or tree fruit (where the grain, fiber or fruit is exposed), reducing the need for more laborious forms of yield measurement. Under controlled conditions, digital imaging can monitor plant biomass production in real time (Rajendran et al. 2009), but research tests have been performed under controlled conditions and do not reflect field conditions. Temporal measurements using multispectral, hyperspectral, and thermal imaging could provide insight into differences between genetics or treatments for stress, disease or transpiration.
In addition to structural plant measurement for HTFBP, a number of physiological measurements have already been successfully demonstrated in the field and could be deployed on UAS. CTD measured with ground-based IR thermometers has been described as an integrated canopy response derived from whole plant stomatal conductance (Reynolds et al., 1994, 1999), CO2 assimilation rate (Fischer et al., 1998), leaf architecture (leaf width and specific leaf area) (Balota et al., 2008), and growth rate (Babar et al., 2006). CTD has been proposed as a proxy for yield, water use, and general crop fitness within given environments (Balota et al., 2007; Hatfield et al., 1987; Howell et al., 1984; Reynolds et al., 1999), but its use for routine variety selection has been hampered by the time required to walk hundreds of plots. In addition, CTD presented a challenge of confounded measurements due to environmental changes that affect crop physiological state. An alternate variable is spectral reflectance, which has been measured in the visible to near NIR region (520 – 900 nm) and used to monitor plant water status, chlorophyll content, leaf area, canopy temperature, green-leaf area retention, and biomass and yield (Araus, 1996, 2009; Babar et al., 2006 a, b; Barnes, 2000). Reflectance spectrometers rely on radiation at leaf and canopy surfaces which is sensitive to temporary changes in cloudiness, sun angle, and the distance between crop and sensor (Jones and Vaughan, 2010). Similar to the situation with plant structure measures like plant height, field testing measurements with spectral reflectance is challenging due to the long time required for testing and changes in environmental conditions. Evaluations in the field are essential for quantitative traits for which the expression depends on the environment (Pieruschka and Poorter, 2012), and technological advances such as simultaneous thermal image of a breeding nursery taken with a UAS could overcome this barrier.
UAS presents an unprecedented opportunity to collect high-volume remote sensing data. Such measurements could allow better varieties and agronomic procedures to be identified. However, the spatial, temporal, and spectral resolutions at which remote sensing data must be collected for optimal use is unknown.
Applications of UAS to Growers and Landscape Management
Biotic and abiotic plant stressors are key limiting factors in all crop production and managed ecosystems. Reducing the impact of these stressors is a priority of researchers across the country. Detecting and mapping plant stress response or individual plant stressors with UAS will provide plant health experts and landscape managers the ability to rapidly respond with appropriate mitigation strategies. Currently, studies have primarily focused on general crop response to a single stress factor such as nutrient deficiency, pesticides, or water stress. These studies have been conducted with little or no collaboration between research teams, therefore collaboration to determine appropriate sensors for various field scales, crops, and platforms will be needed.
While the UAS will be a critical element in rapid data collection, the ability to determine plant stress is ultimately dependent on the data quality. While the literature has described ground-based sensors used to indicate relative levels of plant stress, soil moisture, nutrient deficiencies, etc., additional research is needed to determine the effectiveness of current and developing sensor technology as it is used from the UAS platform. Further research is also needed to relate UAS sensor data to ground-based measurements. Best practices and standards are needed to guide the research community and practitioners in the use and reporting of UAS sensor data.
Research has been conducted to detect plant response to nutrient deficiency, water stress, weed competition, herbicide stress, disease presence, and temperature stress, primarily with manned aircraft and satellite data. Mapping variability allows producers to determine where differences occur within their fields, but unless a specific causal agent is under investigation, determining the underlying causes of variability is still a matter of field data collection and field history. This means that UAS-collected data for production agriculture is hardly a panacea for enabling all production decisions. At present, methods are very specific, requiring translation and calibration between crops and climates (Ishimwe et al. 2014), as well as varying light conditions, soil conditions (Muller and Décamps 2001), crop moisture (Carter 1991), etc. This matter is further complicated by observed differences in sensors and payloads (von Bueren et al. 2015). These factors combine to make development of universally-repeatable routines difficult. In light of this, what is needed is research which determines the appropriate data collection protocols for targeting imaging efforts. Key factors to be identified include spatial resolution, spectral bands, timing and re-visit, and post-processing necessary to produce answers within an acceptable timeframe for a decision by a producer. These factors should also be investigated for geographic and climatological variability. This information is necessary for turn-key solutions to be possible for producers.
Rapid detection and management of biotic and abiotic plant stress will be a key factor in ensuring maximum crop yield and food security in the future. Therefore, it is important to develop the tools to differentiate various plant stressors using UAS. UAS present an unprecedented opportunity to collect tremendous amounts of remote sensing data; however, our understanding is currently very limited on the spatial, temporal, and spectral resolutions at which remote sensing data must be collected for optimal use.
Evaluation of UAS Sensors and Platforms
Producers and service providers need practical procedures for incorporating UAS into production agriculture. Researchers and producers need better understanding of the capabilities of modern UAS platforms and the sensors they carry as they relate to specific applications. A wide range of platforms and sensors are available, but functionality is not well documented in the literature. The rapid pace at which UAS is developing will provide opportunities for testing, calibration, and validation of new systems.
Training and Extension Opportunities in UAS
It is clear that UAS is a novelty to many producers. Many have flown a UAV but weren’t really sure how to get the information they needed or they crashed the aircraft. The end result is a UAV collecting dust on a shelf. The principle objective for multistate training and Extension opportunities is to help producers, consultants and retailers understand that UAS is a system of components that must fit together like pieces of a puzzle to extract the needed decision making information. The components include the aircraft (fixed wing or multirotor), training, image acquisition, image processing, image interpretation and finally turning the image into information for machine variable or broad rate applications.
The Extension Service of land-grant universities provides research-based information, educational programs, and technology transfer focused on issues and needs of producers. The vast base of the Extension Service will be heavily relied upon to deliver the peer-reviewed educational content from the multistate research project. Each component of the UAS puzzle can be quite technical but in reality it’s more common sense. Delivering that technical knowledge in a practical way to educate producers and others so they know how UAS works as a whole and which system is best for their farm operation.
Knowledge outreach technology has changed since the early days of Extension. A century ago nearly one third of the population was involved in agriculture. Few farmers had a high school education (~7%) and many fewer went to college. Universities conducted the research, and then Extension agents worked with farmers to implement new agricultural practices (Lubell et al. 2013).
Today, almost 82% of farmers have a high school diploma, and nearly 16% have four or more years of college. Most have internet access and use social media to connect with their local agricultural networks, sharing their farming issues via texting, twitter, the internet and Facebook. There are also many agricultural specialists outside Extension who are in the social media farming network. These specialists work for retailers, equipment companies, seed and chemical companies, and service providers of remote sensing, data analysis, etc. Many of these are connected to local farmer social networks and provide information and promote their company brands (Lubell et al. 2013). The traditional method of transferring information through print and face-to-face meetings is now often supplemented and/or replaced by web-based outlets (Winstead et al. 2009). Tapping into this knowledge network from local up to multistate regions will be important in delivering knowledge, techniques and innovation learned from this multistate research project.
How UAS will benefit the agricultural industry is still largely unknown. A multistate collaboration can verify the effectiveness of UAS in agriculture through fundamental science and engineering research. How will Extension best manage the transfer of multistate knowledge and expertise to farmers across a wide region? What do producers already know? How can multistate research be applied to local producer knowledge networks? What is their network and how to help them apply knowledge on UAS at the right time and place? Extension must answer these questions to teach the skills necessary for successful adoption of UAS into farm operations
Social media surveys show that Extension still provides a critical amount of valuable information to producers. Over years of work and involvement, county Extension agents form a bond with their producers and people in the county through face-to-face dialogue. But the traditional top-down approach of universities to Extension agents and then down to local producers needs adjusting to meet a more bottom-up networked approach. Producers are a hands-on visual workforce. They may read a journal paper but they do read blogs, use Facebook and Twitter and watch YouTube videos (Saravanan et al. 2013). Producers like to experiment and know how things work. UAS is no different. Once producers have the required skills unmanned aerial systems offer can unparalleled view of the landscape and the potential of new ways in managing large parcels of farm land. Extension will demonstrate to and educate producers on the results of this multistate project and how UAS can be another tool in farm operations.
Objectives
-
Determine the optimal spatial, temporal and spectral resolution needed for actionable decisions from farmers (economic) and researchers (discovery) for the following specific applications:
Comments: a. High-throughput field-based phenotyping b. Crop management (e.g. moisture, disease/infestation, nutrients) c. Livestock management (e.g. biometrics, tracking) d. Forest management (e.g. inventory, disease/infestation) -
Test applications of UAS in specific real-world, production agriculture situations in multiple locations to determine:
Comments: a. Appropriate platforms and sensors b. Methods of calibration c. Detailed protocols for specific applications d. Appropriate data management strategies e. Benefits to researchers and producers -
Use UAS to detect stress and determine stressors in plants and related ecosystems:
Comments: a. Develop a broader understanding of how UAS-based sensors can be used to detect biotic and abiotic stressors of plants and related ecosystems i. Create a knowledge base for key plant stress-response traits, which are detectable by UAS-based sensors, and which indicate common stresses of plants and ecosystems of interest ii. Identify sensor platforms that are most effective in detecting important forms of plant/ecosystem stress (e.g. moisture, nutrient, pest, disease, or chemical) b. Develop models and calibration techniques that relate UAS-derived sensor data to the ground-truthed data for the various plant species of interest c. Deliver the best measurement standards for detecting stress in plants and related ecosystems. -
Develop sustainable, decision-making information that can cross boundaries from multistate down to local agricultural communities in the Information Age.
Methods
Objective 1 –
- Data Aggregation: collect and categorize UAS related research methods and results. Data on UAS related research methods and results will be collected and categorized in effort to enable broad application of UAS to agricultural and natural resource management. The data source will primarily consist of examples from existing literature and include a survey of current research and production practices during professional meetings (e.g. parameter V estimated in application W using sensor X, platform Y, and operated under conditions Z). The aggregated data will serve as a roadmap for pairing the correct tools and methodology with a particular application. An online database will be developed to facilitate data entry from investigators participating in the multi-state project. A common memorandum of understanding will be developed allowing all institutions to access the database.
- Standardization: standardize UAS research methodology across disciplines to better enable multi-investigator collaborations. UAS methods and results that demonstrate consistency across disciplines will be targeted for the development of standard research methods and operating procedures. For example, a standard defining the optimal spatial, temporal, and spectral resolution needed for a specific application will help ensure future research efforts are conducted in a consistent manner such that results can be compared. Standard development will be pursued through professional societies at the national and potentially international level.
- Proposal Development: develop competitively funded research proposals that use UAS for agricultural research that address regional, national, and global problems. Research proposals with investigators across multiple states will be developed to test the viability of standardized UAS methodology for addressing challenges across large geographical regions. Proposals will be categorized by the specific applications including high-throughput phenotyping, crop management, livestock management, and forest management. It is anticipated that the list of specific applications will grow as new technologies and opportunities are identified. Proposals developed as a result of this multi-state project will be tracked and used to map the collaborations between investigators.
- Disseminating Results: facilitate multi-disciplinary meetings to share research findings. Results from this objective relating to research methods will be disseminated through peer-reviewed publications and conference presentations. Results relating to production practices will be disseminated through extension publications and popular press. Publications developed as a result of this multi-state project will be tracked and used to map the collaborations between investigators.
Objective 2 –
- Protocol Development: Multistate teams will work together to develop common protocols for common applications. For example, researchers in Texas and Georgia may collaborate to determine robust and repeatable ways – i.e., a specific series of steps – that UAS remote sensing data can be used to benefit cotton producers.
- Data Aggregation: collect and categorize UAS and ground truth data collection methods and results. Gather appropriate data from individual and joint projects managed by participants and build database of methods and results. As much as possible, data will be uploaded to and maintained on a centralized database, such as the Agricultural Data Cooperative.
- Calibration: research and report procedures and acceptable minimums for data calibration. Identify appropriate projects managed by participants, and in those projects compare results of maximum-level calibration for research purposes with minimum-level calibration for applied purposes and with levels in between the two. This work will pertain to geographic registration and spectral-reflectance calibration, at a minimum. The projects identified must rigorously collect ground-control-point (GCP) and reflectance-target data.
- Platforms: evaluate and report on UAS performance for specific applications. All included projects should, as much as possible, collect data on UAS performance. These data should include a record of planned versus actual activities along with reasons for the differences; e.g., “we planned to fly 50 acres between 11:00 and 12:00 local time, but the aircraft control system failed, so we rescheduled the mission on the following day.”
- Sensors: evaluate and report on UAS-deployed sensor performance for specific applications. All included projects should, as much as possible, collect data on sensor performance. These data should include a record of planned versus actual activities along with reasons for the differences; e.g., “we planned to collect images on 50 acres, but the data-storage card failed, so even though we flew no data were collected and we rescheduled the mission on the following day.”
- Proposal Development: develop competitively funded research proposals that use UAS for agricultural research that address regional, national, and global problems. This project, through its meetings and other group and individual communications, should encourage submission of proposals through formal efforts of the project and through informal “grassroots” efforts of individual collaborators. It is desirable to keep a record of proposal submissions and results.
- Disseminating Results: facilitate multi-disciplinary meetings to share research findings. This project will hold an annual meeting to facilitate coordination, networking, and information sharing among participants. Focused Research: two or more included project participants in different states will work together to develop broadly applicable solutions. These groups of members will work on common crops to develop lists of common practices that could benefit from UAS images and lists of current practices with UAS images. They will also test, using common protocols, procedures that could be beneficial across multiple states
Objective 3 –
- Develop investigator teams with common research interests on specific types of plant stresses to investigate optimal sensing techniques for these stresses. Development of expert teams is critically important to enable effective applications for detection and control of plant stress responses. Researchers within this project and other potential investigators with known expertise and interest in the area of plant stress will be identified. In this way, outstanding expert teams will be gathered to cover the entire spectra of biotic and abiotic stresses. Depending on the investigators’ expertise and interest, multiple working groups will be developed. For example, working teams on disease, insect, and weed problems as part of the biotic; and water and temperature extremes, and nutrient stress as part of the abiotic plethora of stresses mostly recurrent to the Southern USA can be formed for in depth research. However, constant communication of the results across the teams will be maintained to facilitate multi-disciplinary and multi-investigator collaborations.
- Investigate sensing strategies and identify new sensing technologies for detecting various plant and related ecosystem stresses. Data on the current sensing strategies for detecting plant and ecosystem stresses will be collected from the existing literature and direct communication with researchers during professional meetings. Aggregating the data to include precise reference to the conditions in which they were collected, application type, and what sensor, parameter and UAS platform were used is essential for determining the best methods for stress detection applications. Plant response to stress changes with the growing conditions, growth stages, and throughout the day in response to weather fluctuations. UAS methods that show consistency across these factors would seem the best candidate for use as standard methods in plant stress detection.
- Develop a database of stresses and available sensor strategies to detect major categories of plant and related ecosystem stresses. Aggregated and new data obtained through this project will be made available online via a database of stresses and sensing strategies for stress detection. All investigators participating in the multi-state project will have access to the database and constantly update with new data. The database information will be used for multiple purposes including development of research protocols, grant proposals, instruction of undergraduate and graduate students, and extension education.
- Develop algorithms and models to predict stresses from the various types of sensors. Multi-disciplinary teams will develop algorithms and models to predict plant performance under stress within this multi-state project. Using multiple environments, these models will further be validated for suitability with agronomic crops and natural resources, and regional versus large geographical areas applicability. Results will be presented at national and international meetings and used to map further research projects related to prediction and alleviation of plant stresses.
- Identify best practices and standard techniques for sensor use and data collection.Research proposals in multiple environments will be developed to validate the consistency of the available UAS methods for stress detection and identify standard techniques that can be used on large geographical areas. Whether each type of stress and/or plant species will need different standard techniques and sensors for best stress detection will be determined through this multi-state project. As the list of specific applications is anticipated to increase, collaborative research between investigator teams will continue to be developed. Results will be disseminated through peer-reviewed publications and presentations at professional meetings.
Objective 4 –
Extension is fortunate to have professional staff and faculty trained and educated in the latest outreach methods of social media technology. Their expertise will be an integral part of the UAS Multistate Project. Extension Technology Centers will be used in producing several outreach formats such as YouTube videos, webinars and developing smart phone apps if applicable. Not to be overlooked is the traditional county agent who will need the latest UAS information packets produced from Extension Publication department to distribute in their face-to-face meetings with clients.
- Include outreach professionals in the multistate project.Extension Social Media Strategists will develop an educational outreach program that will disseminate results from research both locally and across the Multistate Project region.
- Focus on integrating into producer social networks and UAS businesses by a robust social media presence. Consistently posting engaging and informational content producers and industry professionals can trust will be key to gaining traction in established networks. It will also be important to interact with people in existing social networks and listen to what the real conversation is about in UAS research. Extension will conduct surveys of producers and retailers on which social media like Facebook, Twitter, Instagram they use most and integrate the Multistate Project into those social media. Producers need informative information and social media is a unique platform to meet that information need.
- Training to Extension agents on results from multistate UAS research. Focus on county agent training through face-to-face workshops and webinars on best practices for UAS technology in agriculture learned from the Multistate Project.
- Develop real-time decision support tools - Smartphone Apps. The younger producers especially expect an app. It will also be especially helpful when farmers are on the go and looking at a mobile device. Mississippi State University Extension Center for Technology Outreach has developed numerous apps and would be a good source of information on what steps need to be taken to make an app happen. If results from the Multistate Project can be developed into a smart phone app then the Extension Centers for Technology Outreach will be involved in developing the functional app for release across the Multistate Project region.
- Digital newsletter to connect with a niche audience. It does not need to be a stagnant PDF. The newsletter will be more enlightening if it becomes a truly interactive experience. It should include video, hyperlinks, infographics—even places for feedback from readers.
- Extension Centers for Technology Outreach will again be used in digital content development from the results of the Multistate Project and be distributed both in state and across the Multistate Project region.
- YouTube How-To-Videos. People would rather watch a video than read a huge essay on what they need to know. More and more social media platforms are making it easier to upload raw video directly to their site. However, the how-to videos shouldn’t be very long because many social media sites restrict video length. Work with Extension instructors and Extension video production departments in developing short YouTube videos on the results and best practices developed from the Multistate research.
- Webinars to address a regional audience. Webinars can be a great question and answer platform for producers and retailers with research scientist.
- Field day activities will include fliers and signs showcasing social media handles and hashtags that attendees should know about. It would also be helpful to have UAS social media cards that can be passed out that can connect attendees to the resources they need even after they leave the field day. Field days are a great opportunity to meet with producers and retailers and discuss the activities and purpose of the Multistate Project. Extension Publication can produce posters, information packets and other handout literature.
- Hands-on workshops. Once the research has shown that UAS technology has a proven benefit the hands-on workshops is a valuable tool in getting potential users that experience they need so they can take UAS technology and implement it in their agricultural operations.
- Face-to-face producer visits should always have some sort of packet of information to leave with someone.
- Dynamic infographics tend to do well on social media. Graphics showing Return-On-Investment benefits in producers adopting UAS technologies from methods developed through the Multistate Project.
Measurement of Progress and Results
Outputs
- Objective 1 Comments: • An on-line database to facilitate data entry from investigators within the multi-state project which collects necessary for development of standard research methods and operating procedures (e.g. parameter V estimated in application W using sensor X, platform Y, and operated under conditions Z) • Standard research methods and operating procedures which define appropriate spatial, spectral, and temporal resolutions for specific applications of UAS (e.g. parameter V estimated in application W using sensor X, platform Y, and operated under conditions Z) • Collaborative research proposals to test standard research methods and operating procedures across diverse geographies • Dissemination of research via scientific presentations and peer-reviewed publications, in addition to extension and outreach
- Objective 2 Comments: • A database of UAS and ground truth data collection methods and results • Acceptable minimums for data calibration based on existing research which compares maximum- and minimum-level calibration and for which ground control points and reflectance target data are available • Compilations of performance data for specific applications on both UAS platforms and UAS-deployed sensors, including notations of planned versus actual activities and reasons why these activities may differ (e.g., technical difficulties, weather difficulties). • Collaborative research proposals to address use of UAS for regional, national, and global agricultural problems • Annual meeting to facilitate coordination of research efforts, networking, and information sharing among project participants • Broadly applicable solutions that involve geographically-diverse teams
- Objective 3 Comments: • Expert teams focused on specific biotic and abiotic stresses • An exhaustive literature review to determine the current state of knowledge for specific applications being addressed by expert teams • An on-line database to aggregate research data collected on strategies targeted as specific applications of UAS for biotic and abiotic stresses • Algorithms and models to predict plant performance under specific biotic and abiotic stresses • Collaborative research proposals to validate consistency of UAS methodology for specific biotic and abiotic stressors across diverse geographies
- Objective 4 Comments: • An educational outreach program focused on disseminating results from local, regional, and multistate research • Producer and retailer surveys to gauge social media use • Multimedia products such as dynamic infographics, apps for mobile devices to produce real-time decision support, digital newsletters to connect to niche audiences, and YouTube videos to illustrate best practices and explain research results • Workshops and webinars to train County Agents • Informational field tours and workshops for producers, scouts and retailers
Outcomes or Projected Impacts
- Objective 1 Standard research methods and operating procedures will benefit the scientific community by unifying research programs, creating consistency in data collection and reporting that facilitates meaningful comparisons between studies. This additionally builds on foundational knowledge about the applicability of UAS technology to agriculture stresses. The combined effect of this activity will push the commercial market towards turnkey solutions for producers who desire incorporation of UAS technology into their agricultural operation
- Objective 2 Identification of key performance indicators for UAS platforms and sensors will allow development and validation of minimum standards for UAS data collection. This will increase the accessibility of UAS-enabled research and decision making in both the scientific community and the producer arena.
- Objective 3 Collaborative efforts of leading experts will provide guidance for future development of investigations into UAS applications for biotic and abiotic stresses. This will provide research outcomes which enable the development of new technologies which target specific problems in agriculture, offering opportunity for industry to move into new market directions where targeted solutions can be offered to producers.
- Objective 4 Transition of research findings to end users will benefit both researchers and producers. As more people understand the application and function of UAS in agriculture, interest will increase. Increased interest by the public creates incentives to fund innovative research solutions to producer problems.
Milestones
(0):Objective 1 • On-line database – 1 year to stand up for use and put into operation • Standard research methods and operating procedures – 3 years for development and validation before transfer to market; on-going thereafter • Proposals – on-going • Dissemination – 2 years to start producing meaningful documentation on findings; on-going thereafter(0):Objective 2 • Database – 1 year to stand up for use and put into operation • Acceptable minimums – on-going; yearly updates based on recent literature • Compilations – on-going; yearly updates based on recent literature • Proposals – on-going • Annual meeting – 2 years to plan and facilitate, on-going thereafter • Solutions – 3 years for testing and research before transfer to market; on-going thereafter
(0):Objective 3 • Expert teams – 1 year to confirm teams and develop work plans • Literature review – on-going; yearly updates based on recent literature • On-line database – 1 year to stand up for use and put into operation • Algorithms/models – 3 years for development and validation before transfer to market; on-going thereafter • Proposals – on-going
(0):Objective 4 • Educational outreach program – 1 year to develop; on-going thereafter • Surveys – 1 year to develop; yearly updates to keep up with changing technology trends • Multimedia products – on-going • Workshops/webinars – 1 year to develop; on-going thereafter • Field tours – on-going
Projected Participation
View Appendix E: ParticipationOutreach Plan
Outreach and Extension education will be a significant portion of this project and is considered one of the four objectives. Details of planned outreach and Extension activities are described above under Objective 4.
Organization/Governance
The project governance structure will consist of a Chair, Chair-elect, and Secretary, each with a one-year term. The Chair-elect will become Chair, and Secretary will become Chair-elect, at the end of each annual meeting. Also at that meeting a new Secretary will be selected by the project membership in attendance. These three officers will make up the project Executive Committee.
Project members will decide at the first annual meeting if Objective Coordinators are needed for each of the four objectives. If so, they will be selected at that meeting for at least a two-year term, and will be added to the project Executive Committee.
All project members will meet face-to-face at least once per year, either as a stand-alone meeting or in conjunction with an appropriate conference. Members working on individual objectives may have conference calls periodically throughout the year to coordinate activities and the Executive Committee may also schedule a project conference call if needed.
Literature Cited
Anthony, D., Elbaum, S., Lorenz, A. and Detweiler, C., 2014. “On crop height estimation with UAVs,” Intelligent Robots and Systems (IROS 2014) 4805-4812.
Araus, J.L., G.A. Slafer, M.P. Reynolds, and C. Royo. 2009. Breeding for yield potential. p. 449-478. In: S. Ceccarelli et al. (ed.) Plant breeding and farmer participation, FAO, Rome.
Araus, J.L. 1996. Integrative physiological criteria associated with yield potential. P. 150-166. In M.P. Reynolds et al. (ed.). Increasing yield potential in wheat: Breaking the barriers. CIMMYT, Mexico, D.F.
Araus, J.L. and Cairns, J.E., 2014, “Field high-throughput phenotyping: the new crop breeding frontier,” Trends in Plant Science, 19(1), 52-61.
Babar, M.A., M.P. Reynolds., M. Van Ginkel, A.R. Klatt, W.R. Raun, and M.L. Stone. 2006a. Spectral reflectance to estimate genetic variation for in-season biomass, leaf chlorophyll, and canopy temperature in wheat. Crop Sci. 46:1046-1057.
Babar, M.A., M.P. Reynolds, M. Van Ginkel, A.R. Klatt, W.R. Raun, and M.L. Stone. 2006b. Spectral reflectance indices as potential indirect selection criteria for wheat yield under irrigation. Crop Sci. 46:578-588.
Balota, M., Payne, W. A., Evett, S. R., and Lazar, M. D., 2007. Canopy temperature depression sampling to assess grain yield variation and genotypic differentiation in winter wheat. Crop Sci. 47:1518-1529.
Balota, M., W.A. Payne, S.R. Evett, and T.R. Peters. 2008. Morphological and physiological traits associated with canopy temperature depression in three closely related wheat lines. Crop Sci. 48: 1897-1910. Doi: 10.2135/cropsci2007.06.0317.
Balota, M., Oakes, J. (2016). Exploratory use for a UAV platform for variety selection in peanut. Proc. Ann. Soc. Photographic Instrumentation Engineers (SPIE) 18-19 April, 2016 Baltimore, MD (in press).
Barnes, E.M., T.R. Clarke, S.E. Richards, P.D. Colaizzi, J. Haberland, M. Kostrzewski, P. Waller, C. Choi, E. Riley, and T. Thompson. 2000. Coincident detection of crop water stress, nitrogen status and canopy density using ground based multispectral data. In Unpaginated CD-ROM(13.pdf). In Proc. 5th Int. Conf. on Precision Agric., Bloomington, MN. 16–19 July 2000. ASA, CSSA, and SSSA, Madison, WI.
Burling, K., Hunsche, M., and Noga, G. 2011. Use of blue-green and chlorophyll fluorescence measurements for differentiation between nitrogen deficiency and pathogen infection in wheat. J. Plant Physiol. 168:1641-1648.
Carter, G.A., 1991, "Primary and secondary effects of water content on the spectral reflectance of leaves," American Journal of Botany 78(7), 916-924.
Casadesus, J., Villegas, D. (2014) Conventional digital cameras as a tool for assessing leaf area index and biomass for cereal breeding. J. Integrative Plant Biol. 56, 7-14.
Chapman, S. C., Merz, T., Chan, A., Jackway, P., Hrabar, S., Dreccer, M. F., Holland, E., Zheng, B., Ling, T. J. and Jimenez-Berni, J., “Pheno-copter: a low-altitude, autonomous remote-sensing robotic helicopter for high-throughput field-based phenotyping,” Agronomy 4(2), 279-301 (2014).
Condon, A., Tattaris, M., Molero, G., Lopez-Castaneda, C., Murchie, E., Pridmore, T., Jensen, O., Preston, S., Pound, M., Zhu, X-G., Song, Q., Furbank, R.T., Jimenez-Berni, J., Deery, D., Sirault, X., Reynolds, M., (2013) Optimizing and modeling canopy photosynthesis and photosynthetic duration. In Reynolds M, Braun H. Eds. Improving crop biomass. Proc. 3rd International Workshop of the Wheat Yield Consortium, 5-7 March, 2013 CIMMYT Cd. Obregon, Sonora, Mexico.
Farfan, I.D.B., Murray, S.C., Labar, S. and Pietsch, D., 2013, “A multi-environment trial analysis shows slight grain yield improvement in Texas commercial maize,” Field Crops Research, 149, 167-176.
Fischer, R.A., D. Rees, K.D. Sayre, Z.-M. Lu, A.G. Condon, and A. Larque-Saavedra. 1998. Wheat yield progress associated with higher stomatal conductance and photosynthetic rate, and cooler canopies. Crop Sci. 38:1467-1475.
Hatfield, J.L., J.E. Quisenberry, and R.E. Dilbeck. 1987. Use of canopy temperatures to identify water conservation in cotton germplasm. Crop Sci. 27 (2): 269-273.
Hillnhutter, C., Schweizer, A., K¨uhnhold, V., and Sikora, R. A. 2010. Remote sensing for the detection of soil-borne plant parasitic nematodes and fungal pathogens. Pages 151-165 in: Precision crop protection—The challenge and use of heterogeneity. E.-C. Oerke, R. Gerhards, G. Menz, and R. A. Sikora, eds. Springer, Dordrecht.
Hillnhutter, C., Mahlein, A.-K., Sikora, R. A., and Oerke, E.-C. 2011. Remote sensing to detect plant stress induced by Heterodera schachtii and Rhizoctonia solani in sugar beet fields. Field Crops Res. 122:70-77.
Howell, T.A., J.L. Hatfield, H. Yamada, and K.R. Davis. 1984. Evaluation of cotton canopy temperature to detect crop water stress. Transactions of the ASAE 27(1):84-88.
Ishimwe, R., Abutaleb, K., Ahmed, F., 2014, "Applications of thermal imaging in agriculture – a review," Advances in Remote Sensing 3, 128-140.
Jones, H. G., Stoll, M., Santoa, T., de Sousa, C., Chaves, M. M., and Grant, O. M. 2002. Use of infrared thermography for monitoring stomatal closure in the field: Application to grapevine. J. Exp. Bot. 53:2249-2260.
Jones, H.G. and Vaughan, R.A. 2010. Remote sensing of vegeration: Principles, techniques, and applications, Oxford University Press.
Kefauver, S.C., El-Haddad, G., Vergara-Diaz, O., Araus, J.L. (2015). RGB picture indexes for high-throughtput phenotyping platform (HTPPs). In Neale, C.M.U., Maltese, A. eds. Remote sensing for agriculture, ecosystems and hydrology XVII. SPIE Proc. Vol 9637.
Lenthe, J.-H., Oerke, E.-C., and Dehne, H.-W. 2007. Digital infrared thermography for monitoring canopy health of wheat. Precis. Agric. 8:15-26.
Lubell. M, M. Niles, M. Hoffman, 2013. Extension 3.0: Agricultural Education and Outreach In the Age of Connectivity, University of California Davis Center for Environmental Policy and Behavior.
Mahlein, A.K. 2016. Plant disease detection by imaging sensors – parallels and specific demands for precision agriculture and plant phenotyping. Plant Disease 100(2):241-251.
Mahlein, A.-K., Oerke, E.-C., Steiner, U., and Dehne, H.-W. 2012. Recent advances in sensing plant diseases for precision crop protection. Eur. J. Plant Pathol. 133:197-209.
Muller, E., Décamps, H., 2001, "Modeling soil moisture-reflectance," Remote Sensing of Environment 76(2), 173-180.
Murray, S.C., L. Knox, B. Hartley, M.A. Méndez-Dorado, G. Richardson, J.A. Thomasson, Y. Shi, N. Rajan, H. Neely, M. Bagavathiannan, X. Dong, and W.L. Rooney. 2016. High clearance phenotyping systems for season-long measurement of corn, sorghum and other row crops to complement unmanned aerial vehicle systems. SPIE 9866-4
Nigam, S. N., S. Chandra, K. R. Sridevi, M. Bhukta, A. G. S. Reddy, N. R. Rachaputi, G. C. Wright, P. V. Reddy, M. P. Deshmukh, R. K. Mathur, M. S. Basu, S. Vasundhara, P. Vindhiya Varman, and A. K. Nagda. 2005. Efficiency of physiological trait-based and empirical selection approaches for drought tolerance in groundnut. Ann. Appl. Biol. 146:433-439.
Oerke, E.-C., Steiner, U., Dehne, H.-W., and Lindenthal, M. 2006. Thermal imaging of cucumber leaves affected by downy mildew and environmental conditions. J. Exp. Bot. 57:2121-2132.
O'Shaughnessy, S.A., M. A. Hebel, S. R. Evett, and P. D. Colaizzi. 2011. Evaluaetion of a wireless infrared thermometer with a narrow field of view. Comput. Electron. Agric. 76:56-68.
Passioura, J.B. 2012. Phenotyping for drought tolerance in grain crops: when it is useful to breeders? Functional Plant Biol. 39:851-859.
Pieruschka, R., and Poorte, H. 2012. Phenotyping plants: genes, phenes and machines. Functional Plant Biology 39:813-820.
Rajendran, K., Tester, M., and Roy, S.J. 2009. Quantifying the three main components of salinity tolerance in cereals. Plant, Cell, Environ. 32:237-249.
Reynolds, M.P., M. Balota, M.I.B. Delgado, I. Amani, and R.A. Fischer. 1994. Physiological and morphological traits associated with spring wheat yield under hot, irrigated conditions. Aust. J. Plant Physiol. 21:717-730.
Reynolds, M.P., Rajaram, S., and Sayre, K.D. 1999. Physiological and genetic changes of irrigated wheat in the post-green revolution period and approaches for meeting projected global demand. Crop Sci. 39:1611-1621.
Reynolds, M., Maners, Y., Izanloo, A., and Langridge, P. 2009. Phenotyping for physiological breeding and gene discovery in wheat. Annals of Applied Biology: 155: 309–320
Rossini, M., Alonso, L., Cogliati, S., Damm, A., Guanter, L., Julietta, T., Meroni, M., Moreno, J., Panigada, C., Pinto, F., Rascher, U., Schickling, A., Sch¨uttemeyer, D., Zemek, F., and Colombo, R. 2014. Measuring sun induced chlorophyll fluorescence: an evaluation and synthesis of existing field data. Pages 1-5 in: 5th International Workshop on Remote Sensing of Vegetation Fluorescence, 22-24 April 2014, Paris.
Sankaran, S., Mishra, A., Ehsani, R., and Davis, C. 2010. A review of advanced techniques for detecting plant diseases. Comput. Electron. Agric. 72:1-13.
Saravanan, R., & Bhattacharjee, S. 2013. Mobile Phone and Social Media for Agricultural Extension: Getting Closer to Hype & Hope?
Scholes, J. D., and Rolfe, S. A. 2009. Chlorophyll fluorescence imaging as tool for understanding the impact of fungal diseases on plant performance: a phenomics perspective. Funct. Plant Biol. 36:880-892.
Suchiradipita, R, and Saravanan, R., 2016. Social Media: Shaping the Future of Agricultural Extension and Advisory Services. GFRAS interest group on ICT4RAS discussion paper, GFRAS: Lindau, Switzerland
West, J. S., Bravo, C., Oberti, R., Lemaire, D., Moshou, D., and McCartney, H. A. 2003. The potential of optical canopy measurement for targeted control of field crop diseases. Annu. Rev. Phytopathol. 41:593-614.
West, J. S., Bravo, C., Oberti, R., Moshou, D., Ramon, H., and McCartney, H. A. 2010. Detection of fungal diseases optically and pathogen inoculum by air sampling. Pages 135-149 in: Precision Crop Protection—The Challenge and Use of Heterogeneity. E.-C. Oerke, R. Gerhards, G. Menz, and R. A. Sikora, eds. Springer, Dordrecht, Netherlands.
White, J. W., Andrade-Sanchez, P., Gore, M. A., Bronson, K. F., Coffelt, T. A., Conley, M. M., Feldmann, K. A., French, A. N., Heun, J. T., Hunsaker, D. J. and Jenks, M. A., “Field-based phenomics for plant genetics research,” Field Crops Research, 133, 101-112 (2012).
Winstead. A, J. Fulton, A. Adams, S. Norwood, 2009. Precision Agriculture Education: Using Social Media. Alabama Cooperative Education System.
von Bueren, S.K., Burkhart, A., Hueni, A., Rascher, U., Tuohy, M.P., Yule, I.J., 2015, "Deploying four optical UAV-based sensors over grassland: challenges and limitations," Biogeosciences, 12, 163-175.
Zhang, C., Kovacs, J.M., 2012, "The application of small unmanned aerial systems for precision agriculture: a review," Precision Agriculture 13, 693-712.