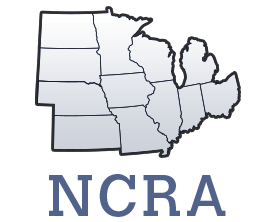
NC1179: Food, Feed, Fuel, and Fiber: Security Under a Changing Climate
(Multistate Research Project)
Status: Inactive/Terminating
NC1179: Food, Feed, Fuel, and Fiber: Security Under a Changing Climate
Duration: 10/01/2014 to 09/30/2019
Administrative Advisor(s):
NIFA Reps:
Non-Technical Summary
Statement of Issues and Justification
Climate change is in the forefront of the agricultural community. Current predictions on crop and animal performance are based on research literature and accepted understanding of the current biological systems. At present, published research is one the few options available to policy makers and producers alike to predict the potential impacts of climate change over the next 30 years. Many of these issues are extremely complex and cannot be evaluated in the field because of not only this complexity, but also because the changes will be gradual. In addition, the magnitude of these changes is uncertain and difficult to predict, especially at the local scale. Also masking some of the overall changes are the large variabilities that exist within the climate system with often large inter-annual changes. In reaction to the potential impacts caused by climate change USDA has started the process of developing regional climate hubs. These hubs are being developed to help guide research and outreach for dealing with climate change issues: USDAs regional hubs will deliver science-based knowledge and practical information to farmers, ranchers and forest landowners within each region of the US to support decision-making related to climate change. These hubs are needed to maintain and strengthen agricultural production, natural resource management, and rural economic development under increasing climate variability. The Hubs will build capacity within USDA to deliver information and guidance on technologies and risk management practices at regional and local scales. (http://www.usda.gov/oce/climate_change/regional_hubs.htm). NOAA and USDA have recently renegotiated a Memorandum of Understanding to work together on climate and agriculture issues as a result of the 2012 drought. Members of the committee had an opportunity to engage in this discussion and comment on the MOU. The current committee members as state climatologists and applied climate researchers are well positioned to engage in both of these activities, the USDA Climate Hubs and the NOAA-USDA interaction. Because of the cross-disciplinary efforts of this committee for many years, the members are positioned to engage well in these activities. A range of climate scenarios exist that attempt to describe how the climate will change over the next three to four decades (Backlund et al., 2008b). These scenarios vary in basic interpretation, but all tend to predict increased temperatures in the range of 1 to 2°C for most of the Great Plains and Midwest. The current literature is replete with results regarding the impact of temperature or drought on crop performance, but seldom are the two evaluated simultaneously and few research frameworks exist that allow for climate changes to be evaluated on a large scale, both geographically and temporally. The recent release of the latest National Climate Assessment concurs with the general trend over the Corn Belt and Plains regions. Numerous groups have sought to evaluate the impact of climate change on crop performance and the subsequent impacts that this may have on global food, fiber and fuel supplies. Hatfield et al. (2008) indicated that high night temperatures will reduce crop yields as a result of higher respiration requirements and carbon loss. Higher temperatures are also likely to cause more rapid crop development which may result in lower yields by shortening the grain filling period (Muchow, 1990). In fact, Lobell and Asner (2003) predict a 17 percent yield reduction in corn and soybean yield for each 1°C increase in air temperatures when temperature effect was confounded with rainfall limitation. However, when the effect of rainfall deficit was accounted for separately, then Lobell and Field (2007) reported a smaller 8.3% reduction in corn yield and a 1.3% decline in soybean yield per 1ºC rise in temperature. Backlund et al. (2008) also predict that precipitation variability will increase along with the increased incidence of heat waves. This rainfall pattern will likely result in longer periods of water stress followed by intense rainfall events. Wolfe et al. (2008) estimate that crops in the Northeast US will experience higher frequency of temperature stress, perennial crops will have inadequate chilling in the winter and all crops will be exposed to increase pest and weed pressure. Crop, soil, pest, and economic models that are used singularly or simultaneously exist in systems that can rapidly test the impacts of drought and temperature stress on crop species. Corn yields are currently increasing at a rate of approximately 95 kg ha-1 year-1. Recent trends in yields over the last four years have seen below trend yields occurring in the corn belt as a whole because of combinations of droughts, extreme heat and late planting because of wet spring conditions. Predictions suggest that because of increased night temperatures and greater incidence of water stress, this trend will decline to little or no annual improvement. Such predictions assume that plant adaptation, both natural and through human interaction (breeding), will not occur as climate change is occurring. Evaluating the impact of a breeding program, which may focus on performance under high temperatures, is difficult with current field and growth chamber technology, because most controlled environment systems, like SPAR or FACE, are too small to allow for germplasm evaluation or traditional breeding techniques (Hatfield et al., 2008). Crop models exist in systems that can rapidly test the impacts of improved drought or temperature tolerance on crop productivity as climate change occurs or they can be used to provide plant breeders with areas of focus to address this challenge. Along with increased variability in precipitation, it has been predicted that total rainfall amounts in the Midwest and Great Plains will be reduced approximately 10% with a shift toward more precipitation being received during the winter months and less during the summer months (Backlund et al., 2008). Increased precipitation variability will increase the risk associated with crop production through longer periods of drought and higher intensity thunderstorms. These events result in lower yields as well as greater opportunities for soil erosion and crop damage from hail and damaging winds. Increased intensity of heavy rainfall events will continue to cause excess run-off, flooding and increased soil erosion. Soil organic matter levels affect water infiltration rates, soil water holding capacity (Hudson, 1994), crop productivity, and is a regarded as one of the primary measures of soil quality. Increases in temperatures, reduced crop productivity and increased soil water deficits (because of increased precipitation variability) could reduce soil organic matter levels if management practices such as reduced tillage and the use of cover crops are not adopted. The effects of reduced tillage and the use of cover crops [cite] are well documented as methods of increasing soil organic matter, but the effects of increased temperatures and reduced soil water content are not and will be difficult to assess with current field and growth chamber techniques and technology. Integrated or coupled crop, soil, and economic models can be deployed to address these problems. The advantage of a modeling approach is the range of treatments that can be tested is nearly unlimited and is only constrained by the model functionality. A great deal of focus has been placed on the impacts that increased temperatures and precipitation variability may have on crop productivity. However, these climate scenarios may equally affect disease, insect and weed pests. Increased temperatures will likely alter insect, disease and weed lifecycles as well. Recent research has illustrated that some weed species require greater quantities of herbicide to control them when CO2 increases (Ziska et al., 1999). However the change for many pests can be positive, negative or neutral depending on the local environment change (Coakley et al., 1999). The impact is even more complicated since climate change not only affects the pest response, but equally affects the host response to disease and insect attack (Garrett et al., 2006). Until climate change scenarios can be down-scaled to the level where pest impact can be modeled and assessed, uncertainty will remain (Seem, 2004). To complicate the challenges associated with climate change, the Renewable Fuel Standard Program mandate to quadruple bioenergy contributions to the U.S. fuel supply by 2010 (U.S. Dep. of Energy, 2007; http://www.epa.gov/oms/renewablefuels/). Biofuel production has many production challenges, but protecting soil and water simultaneously is also important to society as a whole. Many agricultural practices developed over the past decade (nutrient and manure management, cropping systems, reduced tillage systems) have made great strides in improving and protecting soil and water resources that benefit the general public as well as the crop producer. However, if pressure to remove residue from production fields to produce bioenergy is not properly balanced, then these soil quality gains could be lost along with soil through erosion and water quality through impairments via runoff. Developing crop management systems that provide bioenergy feedstocks while protecting soil and water resources will be difficult to study in the field because runoff studies require large watersheds, numerous locations and many years to encompass a wide range of environments. These types of studies require a large amount of financial support and will span five to ten years before tangible results will be available. Once again, integrated models can address these questions rapidly and can evaluate a larger number of treatments than field experiments. Coupled or integrated crop, soil and economic models are poised to address questions that may arise because of expected climate change. Other questions related to bioenergy production include the most appropriate species to be produced at a given location and the evaluation of perennial, annual or mixed cropping systems to address the needs of the bioenergy industry. Perennial crops have the advantage that after establishment, annual production costs and energy requirements are lower than annual crops. Traditional perennial grass production has largely occurred in marginal areas, which means that there is not a clear understanding of their production potential if grown in higher production environments (i.e. switchgrass on deep silt loam soils or under irrigation). It would be within the framework of the existing databases and crop models to evaluate switchgrass or other perennial crops both spatially and temporally across the ten-state region. The advantage of annual crop systems for bioenergy production is they allow producers flexibility to respond to the food, fiber and fuel needs of society rapidly. It is conceivable that future bioenergy systems that adequately balance food and fuel needs while simultaneously protecting soil and water resources will include both food and fuel crops with the use of cover crops during fallow periods to reduce the negative impacts of annual crop production. Developing such a system can be easily evaluated with integrated models across a wide range of environments and soils types (Hoogenboom et al., 2004). Earlier iterations of this committee, (North Central Regional Committee 1018) have not only developed a spatial-temporal database of soils, weather, and crop information for the ten north central states, it has developed a research model that can easily be replicated in other regions of the U.S. or the world. The methodologies used to aggregate these data to a county resolution could be applied to other regions to develop similar databases. The system also has the ability to create input files for use in integrated crop-soil-environment suites such as the Decision Support System for Agrotechnology Transfer (DSSAT; Hoogenboom et al., 2004), but also has lower level soil and crop simulation models programmed within the Modeling Applications Integrated Framework (MASIF) database at the Computational Ecology and Visualization Laboratory at Michigan State University. The future plans are to use the existing measured weather data to develop climate change scenarios which will add a new suite of capabilities to this system that can aid in answering production, economic and policy oriented questions. In addition several current NC-1179 members run state networks through then own auspices ranging from the Pacific Northwest, to the Plains and Corn Belt. These networks have been developed to enhance monitoring and forecasting for disease, insect and irrigation applications. The networks also provide a unique ability for cooperation on monitoring lesser-noted atmospheric data such as humidity, wind, soil temperature and soil moisture. In some cases these stations provide the only data available in regions. Collaboration among the members will allow for better development and monitoring of changes and variability in these additional types of data. Long term data sets for temperature and precipitation at higher spatial density (roughly county level), but are not available for the other data sets. The continuing monitoring of these types of data in their states will continue to show the trends and variability existing in these data. The existence of these state networks was the basis for a soil moisture and snow monitoring proposal for the Upper Missouri Basin which is currently part of a WRDA (Water Resources Development Act S.R 601) which passed out of the Senate in the spring of 2013. The committee also has a long history of developing new and innovative measurement standards, data manipulation and quality control methods. Most climate change scenario predictions are at very large scales (several 1000 km2); however, the NC-94/1018 database has a county level spatial resolution. It has an existing temporal resolution of 30 year with the tools and templates in place to expand temporally as more data is measured. The importance of these data is they first can serve as baseline for measured data comparisons over the next 30 to 40 years AND can serve as a baseline from which to develop climatologies from the climate change model predictions. An integrated modeling approach is needed in order to develop effective policies, management programs that secure our food, fuel, and fiber supply as well as protect our natural resources. Often these systems need to be evaluated simultaneously for the biological, agronomic, ecologic and economic impacts. Since all of these variables are intertwined, one model type (crop model, soil model, etc) will seldom accomplish -the objectives set forth here. The scientists who participate in NC-1018 encompass a wide range of disciplines and include crop modelers, soil scientists, climatologists, and agricultural economists. This integrated team has the capabilities to address all of the issues described above and objectives stated below through large scale, integrated modeling efforts. The committee is also well positioned in working with currently-funded USDA and NOAA grants to develop tools for producers. These projects include the AFRI-funded (U2U) Useful to Usable Project developing tools for producers in the Corn Belt, the Corn CAP, working on climate change issues and tools in the Corn Belt and the NOAA Regional Integrated Sciences Assessment -The Southeast Climate Consortium. Six state climatologists are also involved. All are using climate information to help develop decision-making in agriculture in some form to help users make decisions. These tool applications are excellent methods of connected current research to tools and applications for producers.
Related, Current and Previous Work
The current NCR committee, NC-1179, and its predecessors NC-1018/NC-94, have been in existence for nearly 60 years working on research activities related to the role of weather and climate on agriculture in the North Central Region and other member states. The committee has helped move forward several major agro-climatological innovations, including some of the first successful efforts to collect and electronically enter data for agroclimatological studies and as well as proposing and championing the formation of regional climate centers which have now successfully provided data to the general public and performed research activities on regional climate for almost 30 years. They have also conducted research activities on regional climate and its impact on agricultural production and resource use. Over the course of this past reassignment, the committee has been responsible for the publication of over 80 scientific publications to communicate and disseminate their efforts. The committee has also expanded to increase coverage with representatives from across the country. The scientists in the group have experience in analyzing large scale climate data and potential climate change scenarios. Growing season precipitation variations in central and western U.S. have been examined and found that the interannual variations in summer rainfall in these regions have been strongly influenced by the SST anomalies associated with the Atlantic Multidecadal Oscillation (Hu et al,, 2008; Feng et al., 2008; Feng and Hu, 2007; Feng and Hu, 2008). Data collection remains a responsibility of many member of NC-1179. Over the past five years, the weather station networks have expanded in North Dakota, Michigan, South Dakota, Kansas, Nebraska and Washington and Missouri. However, a more significant accomplishment has been the development of web sites to deliver the information to clientele. Web sites are available for data retrieval from North Dakota, South Dakota, Iowa, Missouri, and Kansas. All of these web sites enable users to down load raw data, but many have weather-related products that are also available. These include advisories for planting, pest control and irrigation scheduling routines that use measured weather data to assist clientele in their daily lives. These networks have proved especially useful in K-12 education programs where students can monitor the local weather and climate conditions to learn more about the world around them. The committee members as state climatologists and climate scientists have expanded to discuss climate change to general audiences and deliver appropriate climate change information to those audiences particularly about agricultural impacts. Many members of the committee have been involved in a USDA AFRI funded project U2U which has sought to take various available climatological datasets and develop and evaluate tools (http://www.agclimate4u.org). This has expanded the efforts of the regional committee and state climatologists to develop tools and do full evaluations on tools and how users utilize the information in agricultural decision-making. Partial goals of the 5 year project are to continue work from the U2U project in an on-going basis. This regional committee has a long history of collecting and aggregating spatial and temporal data with regards to crop production, soil physical and chemical properties, and weather variables important to crop growth and development. During the past five years, this group has continued this mission as well as the mission of utilizing these data as inputs for crop, soil and pest models, to analyze crop responses to the environment and to develop risk management tools for crop producers and policy makers. In coordinating data, four state climate offices representing IL, KY, MI and MO met in Champaign, IL in March 2013 for 1 .5 day regional meeting to discuss technical aspects and feasibility of assimilating weather data from their state mesonets into the Midwestern Regional Climate Centers Applied Climate System (MACS). Initially, each state presented an overview on the operation of their mesonet to the Midwestern Regional Climate Center Director (MRCC), Dr. Beth Hall, and MACS technical staff. A discussion followed on developing a uniform platform for development of a regional mesonet database, including metadata and quality control. The MRCC identified formatting and other technical requirements for ingesting data, including development of applications using mesonet data. Discussion also extended to developing options for uniform instrumentation and development of one or more test beds for comparing the performance of different sensors used by various mesonets. Members in the High Plains continue coordination on networks though the High Plains Regional Climate Center and are part of a regional proposal to expand soil moisture monitoring in the Missouri Basin as a pilot project for a national soil moisture network. Significant progress was made in the simulation of two representative crops growing in the region, corn, and wheat, under historical and projected future climates. The simulations included areas within the region where agricultural activities historically have been limited by climatological and soil constraints, but which could become more favorable for agriculture in the future given a warmer climate. CERES-Maize and CERES-Wheat crop models, part of the Decision Support for Agrotechnology Transfer (DSSAT) model software system were used for all simulations. Five locations across the Great Lakes region (1 in WI, 3 in MI, and 1 in NY) were chosen for the study on the basis of climatological series record length and homogeneity, as well as geographical coverage across the region and its major land use zones. The study was divided into two major categories; historical and future. In general, mean annual temperatures across the region warmed 2-6°C (relative to the 1990-2009 period) in the ensemble of projected future time series by the 2090-2099 decade. Projected annual precipitation totals in the ensembles generally remained in a range between 70-140 percent of the control period totals, with a slight overall average increase by the 2090-2099 decade. Regionally, low precipitation and moisture stress were chief limitations to simulated crop yields during the historical period. Simulated corn and wheat yield series were found to increase with time since the late 1930's at most of the study sites, largely the result of wetter, less stressful growing season weather conditions. In the projected future simulations, the warmer climate suggested by the GCMs led to some initial early-century increases in simulated non-CO2 enriched crop yields relative to historical yields. By late in the century, however, relative yield declines were found for most of the projected scenarios. With CO2 enrichment included, increases in simulated wheat yield were noted for most scenarios. Largest percentage increases in yield during 2010-2099 were found at northern locations. The ratios of the future scenarios with and without increases in CO2 concentration suggest that the majority of wheat yield increases during this period are due to CO2 enrichment. In the analysis we downscaled the present-day control simulations and predictions of future climate in the 21st century from 16 fully coupled atmosphere-ocean models included in the IPCC AR4 from the models grid resolutions to a 1/8 degree grid system. This statistically-downscaled dataset covers the continental U.S. for the period from 1950 to 2099. The predictions of future climate were made with low, median and high greenhouse gas emission scenarios (SRES B1, A1b, and A2). Using the downscaled temperature and precipitation data we calculated the PDSI and used it to evaluate the drought variability. Major results suggest that the High Plains will become drier in the future warmer climate. Significant increases in drought intensity and durations are projected for this region. An article on the changes in drought variability in the continental U.S. is currently in preparation. We modified a hybrid soil temperature model by combining empirical and mechanistic approaches, and evaluated the model in an agroecosystem and also a tallgrass prairie in the Great Plains. This model simulated soil temperatures on a daily basis from meteorological inputs of maximum and minimum air temperatures and soil and plant properties. The agroecosystem consisted of a no-till corn (Zea mays L.) and soybean (Glycine max Merr. (L)) rotation system. In the agroecosystem, the root mean square error of the modified model simulation varied from 1.41 to 2.05 oC for the four depths of 0.1, 0.2, 0.3 and 0.5 m beneath the surface. The mean absolute error varied from 1.06 to 1.53 oC. The root mean square error and mean absolute error of the modified model were about 0.1-0.3 oC less than the original model at the 0.2-0.5m depths. For the tallgrass prairie, the mean absolute errors of the simulated soil temperatures were slightly greater than the agroecosystem varying from 1.48-1.7 oC for all years and from 1.09-1.37 oC during the active growing seasons for all years. Advantages of this model are its relative simplicity using readily available daily temperature data. The new model can be incorporated into a larger crop model where soil temperatures are required. Given the applied nature of this hybrid model, it would be well suited to simulate soil temperatures in the first 50 cm of soil over a vegetated surface for processes related to soil respiration, soil organic matter decomposition and soil-borne pests. Increased crop production and expansion of irrigated acreage in the USA have increased agricultural water use during the past two decades. To optimize irrigation water use, it is important to know when to irrigate and how much water should be applied. We evaluated the Cropping System Model (CSM)-CERES-Maize model with measured data for the amount of water required for supplemental irrigation. Then both monthly and annual water demand for maize was determined for each county. The results from the evaluation showed that the model was able to simulate the amount of water required for maize irrigation in good agreement with the observed data. This demonstrated the potential application of the CSM-CERES-Maize model as a tool for estimating water demand for irrigation. The estimated water requirements for supplemental irrigation can be used by both policy makers and local farmers for planning the amount of water required for supplemental irrigation as well as for improvements in irrigation management for water conservation. The history of this group began with data collection and archiving. The use of the archived data was later added as an additional focus for the group, but continuing to collect and archive data remains one of the objectives for this group and their methods have also evolved. Improving existing data sets (Nelson et al., 2005) or improving the accuracy of soil input data (Gijsman et al., 2007; Gunal and Ransom, 2006 a and b; Karlstrom et al., 2007; Olson et al., 2005 a and b; and Staggenborg et al., 2007) and weather data estimations (Bannayan and Hoogenboom, 2008 a and b; Garcia y Garcia et al., 2005; Garcia y Garcia et al., 2008; Garcia y Garcia and Hoogenboom. 2005; Shank et al., 2008a and b; Soltani and Hoogenboom, 2007; White et al., 2007) continue to be a focus of this group. These techniques will be useful in developing the daily climates based on climate change scenarios. Data collection methods continue to be evaluated to improve model input data available, its quality and resolution (Alfieri et al., 2007; Chen et al., 2007). In more recent years, socioeconomic data were included in the NC 94/1018 database so that temporal impacts of crop yield fluctuations can be evaluated for their impacts on farm size, number and profitability. Crop based food, fiber, and fuel production is determined by human manipulation of the crop-soil-environment interaction, which is often referred to as crop management . Decades of field research have been dedicated to studying this complex interaction as a means to maximize natural resource use efficiency. This approach will also be important in studying the impact of expected climate change over the next decade. In recent years, biological models have matured to levels capable of being important tools in this research area (Staggenborg and Vanderlip, 2005). In the past five years, the many efforts by NC-1018 have focused in the area of crop-soil-environment interaction. The current NC-94/1018 database was used to asses the impact of climatic factors on crop productivity and yield trends across the region (Todey and Shukla, 2005; Staggenborg et al., 2008). Attempts have been made to predict the impact of drought and frost events on crop production (Boken et al., 2007; Lopez-Cedron et al., 2005, 2008; Jain et al., 2006; Prabhakaran and Hoogenboom, 2008; Sau et al., 2004; Smith et al., 2006) as well as the effects of management decisions on yields of maize (Fang et al., 2008; Tojo Soler et al., 2007), sweet corn (Lizaso et al., 2007), pearl millet (Tojo Soler et al., 2007), peanut (Dangthaisong et al., 2006), and grain sorghum (Staggenborg et al., 2008). Models have also been used in crop improvement and plant breeding programs (Anothai et al., 2008; Boote et al., 2003; Suriharn et al., 2007; White and Hoogenboom, 2005). More recently, crop models have been used to assess yield risk that results from year to year crop yield fluctuations, an important tool in the face of increased uncertainty as a result of global warming. Deng et al. (2008) used crop models to develop crop indices for alternative crops and crop models have been used to evaluate the risk associated with irrigation (Lin et al., 2008). Crop models have been used to evaluate yield stability has been evaluated (Banterng et al., 2006; Garcia y Garcia et al., 2006) and if forecast information can be used as a risk management tool. (Fraisse et al., 2006). Results from these efforts pave the way for continued assessment of crop responses to climate change with the use of crop models. Other efforts in this area include evaluating the impacts of estimated weather variables (Garcia y Garcia et al., 2005; Garcia y Garcia et al., 2008; Garcia y Garcia and Hoogenboom, 2005; Shank et al., 2008a and b; White et al., 2007) and other potential climate impacts such as increased aerosols (Greenwald et al., 2006) and increased CO2 levels (Heinemann et al., 2006). As climate change unfolds, crop simulation models will assist producers in altering their crop management practices to maintain crop productivity and profitability. Crop models will be key in developing these new management systems through crop selection (Mullen et al., 2005; Pathak et al., 2007; White et al., 2007), evaluation of the new management strategy before capital is invested in it adoption (Soltani and Hoogenboom, 2007), the evaluation of integrated production systems (Herrero et al., 2007), and potential land use changes (Ashish et al., 2008) that may occur. We have completed several projects contributing to system optimization for management of plant pathogens under global change. For example, Garrett et al. (2013) evaluated the effects of climate variability and the color of weather time series on agricultural diseases and pests, and also evaluated the effects of these patterns on the efficacy of decision making for disease management. They also developed a computer program for evaluating the effects of these patterns. Garrett et al. (2012) evaluated the prospects for managing plant health under climate change and proposed new experimental options for designing research spillover from plant genomics to understand the role of microbial communities. Skelsey et al. (in review) have developed a new approach for evaluating climate change impacts in maps of plant disease risk. In summary, NC-1179 has continued its history of collecting and aggregating spatial and temporal data throughout the region. Other efforts have focused on improving the quality of the data, expanding the data available, and developing new techniques to exploit existing datasets. Modeling efforts continued in the development and evaluation of crop and pest models as well as the development of soil carbon models. One area of new innovation is integration of models from different disciplines. Several outcomes from this group will enable them to provide insight on the impacts of climate change on food, fiber, and fuel production. These outcomes include experience in risk management assessment, new techniques for predicting environmental stresses and the ability to assist producers in managing inputs such as irrigation water and selecting the most appropriate crop for a given scenario to achieve the goals of securing adequate food and fiber supplies. These results will also serve as the framework to expand these efforts to other regions of the U.S. and other regions of the world.
Objectives
-
Enhance the understanding of the effects of climate variability and change on crop and livestock production systems
-
Develop and evaluate innovative observational technologies through regional collaboration of observational networks and partnerships to develop new data and products for agriculture
-
Develop predictive systems and risk assessment and decision tools at various spatial and temporal scales to support climate smart crop, hydrologic and livestock production systems
-
Enhance the understanding of how stakeholders use weather and climate information in their management decision process
-
Disseminate the research outcomes and educate stakeholders on the potential effects of climate variability and climate change on agricultural production resource use and adaptation options through cooperation with university extension services, state climate offices and various federal partners.
Methods
Objective 1 Enhance the understanding of the effects of climate variability and change on crop and livestock production systems The specific assumptions the project is making are that: - If the project is to be successful, stakeholders need to be constructively engaged in a dialogue about climate variability and change that is i) presented using non-threatening language, ii) identifies immediate issues that are relevant to producers and iii) offers benefits that are tangible for producers in both the short- and long-term. - When presented with science-based information in experiential ways, producers will both increase their knowledge and their willingness to adopt alternative management practices. The challenge to develop and disseminate adaptation and mitigation strategies with an increased chance of adoption is to overcome lack of knowledge, misconceptions, and the sometimes existing cultural and political barriers to constructive action related to climate change. We need to provide diverse stakeholders with trusted, useful, and science-based information and tools to aid them in making informed decisions. Much current work of members of the committee is based around understanding and discussing the current varying and changing climate and the impacts on agricultural production systems. Long term databases exist for temperature and precipitation over the United States. Thus, linkages between these data and yield relationships (for example) have been previously illustrated. The development of new climatologies such as humidity and soil moisture are only possible through ongoing and improved monitoring as is discussed in objective We will continue work relating new observations to changes in agriculture and current impacts to changes in yield and cattle losses for example. We will discuss potential future changes relating new predictive capabilities to disease and other crop production issues. The Kansas State University agronomy researchers (Holman, Lin, Ransom and Roozeboom) will provide an extensive and robust knowledge base for water-efficient cropping systems and crop and livestock production systems. a.) Water-efficient cropping systems i)Grain sorghum – Grain sorghum’s yield stability in drought-prone environments makes it a key component of cropping systems in the Great Plains as water resources become scarcer and less predictable. Current emphasis is on optimizing sorghum management to improve performance of a subsequent wheat crop. ii)Cropping system intensification – Intensifying cropping systems can mitigate climate change via increased carbon fixation and greater system-wide water use efficiency as well as potentially increase crop production from a declining land resource. Research will focus on finding ways to increase cropping system intensity while maintaining productivity to improve annual cropping system water use efficiency. We will investigate the soil and environmental effects of cover crops in the semiarid central Great Plains. b.) Crop and livestock production systems i. Using soil moisture and precipitation outlook together may increase the success of increasing cropping intensity during favorable years or farming conservatively in dry years. ii. In order to stabilize crop yields, dryland crop rotations have typically included fallow to accumulate precipitation in the soil profile. However, fallow is relatively inefficient at utilizing precipitation when compared to a growing crop. iii. Our project will determine if the integration of forages into the crop rotation when conditions are favorable could reduce the amount of fallow in the rotation, increase precipitation storage and reduce evaporation, reduce wind and water soil erosion, improve soil health, provide additional forage for the livestock industry, and increase profitability. iv. Livestock systems that depend on pasture and rangeland are very vulnerable to weather variability and climate change. Combining soil moisture and precipitation outlook with grass productivity measurements would benefit livestock producers by predicting grass productivity. v. Long-term precipitation records and grass productivity are available for many regions across the United States. Grass productivity is correlated to weather the predominant forage type is cool-season or warm-season and when most of the precipitation generally occurs. Past research did not include soil water measurement, but by combining soil water status and early season precipitation better management decisions for season-long stocking can be made early in the grazing season. Objective 1a. Increase climate science literacy of stakeholders (particularly related to agriculture) including extension faculty and producers across the regions of interest to the project. Educational modules will be produced and made available on the Internet as well as in printed form; they will become a continuous source for information for the stakeholders as well as others across the country and under similar climate and production conditions. Additionally, these products will serve as a resource to be used during the workshops and training sessions offered as part of this project, thus providing the opportunity to improve the products for mass distribution and effectiveness. Objective 1b. Integrate extension and research communities to facilitate the transfer of technologies and information about research gaps and needs of the industry Integration of our Extension activities with research faculty is of paramount importance for a successful outcome of this project. Our challenge is to provide stakeholders with trusted, useful, science-based information so that they in turn can make informed decisions. In addition, there is a need to inform the research community of existing knowledge gaps concerning the impact of climate change on agriculture across the USA. Objective 2. Develop and evaluate innovative observational technologies through regional collaboration of observational networks and partnerships to develop new data and products for agriculture Weather and climate have numerous impacts on agricultural production and farm management. Several NC-1179 participants are closely affiliated with automated weather station networks in their state - or mesonets. Over the years a large database of environmental variables has emerged from these mesonets; the value of this database grows with each additional day of monitoring. The weather station networks provide opportunities for educational programs, teaching, research, innovation, discovery and service to the agricultural community as well as numerous other sectors. Objective 2a. These weather stations are key to the development of long-term climatological databases in order to be able to document climate change and climate variability. At the same time these weather stations provide data that are being used in models and decision support systems (Objective 3) to provide information to stakeholders for decision makers. We propose to collaborate and exchange information with respect to operation and maintenance of the environmental monitoring systems, including sensor calibration and maintenance, new sensor evaluation and performance and data management. Output will be enhanced environmental monitoring systems that provide accurate weather data. All mesonet participants will collaborate in the development and evaluation of technologies to integrate and apply the detailed environmental data collected by the project’s mesonet systems. Such an effort would ultimately increase the value of the base data, allow direct integration of the data into larger regional scale applications, and lead to more useful applications. Objective 2b. Development of data and products for agriculture Based on the activities under objective 2a, it is important to develop databases and data products that can be readily accessed by users, including colleagues in academia, as well growers and other stakeholders. With the rapid changes in information and communication technology, we propose to share state-of-the-art technologies that are being implemented in the participating states and to explore further options for collaboration. Output will be improved data and weather-based data products that can be easily accessed by stakeholders. All mesonet participants will actively work to maintain and enhance their networks and integrate weather and climate databases to develop solutions across different time scales. We will continue work on tools from the USDA Funded U2U project to complete the 5 year goals and establish method to carry-on tools developed via U2U through the NOAA Regional Climate Centers. We will seek additional funding to improve and enhance tools from U2U to add to the tool suite. Regional collaboration on monitoring of atmospheric data and soil moisture information is existing in the states from North Dakota to Kansas. Additional collaboration on monitoring and tools developed from the data will occur through interaction on the project. Todey(South Dakota) will cooperate on the monitoring and development of improved soil moisture monitoring. Dr. Ransom (Kansas State University) is a soil scientist and will provide expertise for the project in the application and use of various soil databases for crop modeling and research on water-efficient cropping systems. He has been an active participant in NC1179 and earlier iterations of the project. Objective 3: Develop predictive systems and risk assessment and decision tools at various spatial and temporal scales to support climate smart crop, hydrologic and livestock production systems Climate variability is a major source of crop production risk. The majority of crop failures in the USA are associated with either a lack (e.g. 2012) or excess of rainfall (e.g. early 2013). Climate variability is also associated with other sources of production risks such as pest and diseases. According to the Intergovernmental Panel on Climate Change (IPCC, 2007), projected changes in mean climate conditions and in climate variability are likely to increase disruptions in food security at local, regional, and global scales unless cropping systems are resilient and adapted to those changes. Increases in human populations and demand for food, energy, and water combined with an uncertain future climate that is very likely to have higher temperatures and increased frequencies of extreme events are certain to lead to increased food shortages unless cereal cropping systems become more resilient to those changes. The IPCC estimated that by 2080, there could be between 100 and 230 million undernourished people under the low to moderate greenhouse gas emission scenarios. It is clear that we need to increase the climate resilience of the agricultural industry in the USA by co-developing and communicating strategies to help producers reduce crop, hydrologic and livestock production risks associated with climate variability and long-term climate change. However, developing strategies is not enough, they need to be communicated and informed to stakeholders in a way that facilitates their effective application across different geographies and production systems. Risk assessment tools are an important component of climate smart production systems as they help evaluating risk levels under different scenarios. Previous work of the committee has emphasized developing tools and products to support agricultural systems. What is necessary looking to the future is to develop better predictive tools for agricultural production and risk management. Such an objective is also the interest of recent Memorandum of Understanding between NOAA and USDA and is also a goal of the proposed USDA Climate Hubs. Thus, the committee proposes to continue working to develop and refine tools and predictive systems for agricultural and hydrologic decision-making. Objective 3a. Crop Model Improvement One of the most widely used tools for understanding the impact of climate change and climate variability on crop and livestock production system are crop and forage simulation models. However, current studies have shown that model evaluation and improvement is required to deal with the complex issues of the interaction between the plant and soil and weather, especially under extreme conditions. We propose to evaluate the current simulation models with existing data sets from prior research and use this information to help improve the crop simulation models. Output will be improved models that can better address crop production under a changing climate. Recent climate change impact research has suggested that differences in various crop simulation models may lead to levels of uncertainty as high as those associated with different climate models (Rosenzweig et al., 2012). Andresen (Michigan State Univ.) will collaborate with other PIs to examine the relative differences between three major process-based crop simulation model ensembles for a corn cropping system: 1) The Decision Support System for Agrotechnology Transfer (DSSAT) system (Jones et al. 2003); 2) The Hybrid-Maize model (Yang et al., 2004); and 3) The Systems Approach for Land Use Sustainability (SALUS) model (Basso et al. 2006). These modeling systems account for the impacts of microclimatic variability on crop growth, development, and yield as well as underlying hydrological processes and water cycle dynamics. Nearly all agronomic and other technology-related input variables in the simulations will be held constant so as to isolate the effects of weather and climate. At the beginning of the project we will undertake validation of the simulation models with a comparison of simulated yields versus observed county-levels yields with independent data sets. The AgWeatherNet Program at Washington State University (Hoogenboom) currently coordinates the development of the Decision Support System for Agrotechnology Transfer (DSSAT). We will continue with the development and advancement of the DSSAT crop modeling system to address current and future scientific challenges. We will also organize training workshops on a regular basis to facilitate knowledge exchange with those interested in crop model development and applications. The Kansas agronomy researchers will use crop models to study cropping system performance within the semi-arid Great Plains. The results from the simulations will allow producers and policy makers to develop programs aimed at maintaining rural economic viability under a changing climate coupled with a decline in the availability of ground water. Hu (Nebraska) et al. will participate in a study to understand how maize and soybean production in each of the participating states may have been changing as a result of regional climate variations and change. Each representative from their state will assist in obtaining state crop production data and practice data. Participants will also retrieve climate data from each state in addition to the reanalysis datasets, and design a set of analyses to quantify the variations/changes in those crop productions. Each participating state will take the procedure and complete their analyses for their state. The results from each state will be summarized and reported in publications. Objective 3b. Pest and Disease Model Improvement In current climate change and climate variability studies biotic stresses are mainly ignored. We propose to continue to develop and enhance pest and disease models that are capable of evaluating crop production systems responses to environmental challenges. We will also explore options for linking pest and disease models with crop simulation models in order to improve our understanding of the interaction of biotic and abiotic stresses with crop production systems under a changing climate. Output will be improve pest and disease models as well as linked crop-pest modeling systems. Garrett (Kansas State University) will develop and publish disease models, for integration with crop simulation models, to evaluate crop yield loss under climate change scenarios. Garrett will also develop and implement a framework for analysis of how decision support systems for pest and disease management will become more or less useful, and may need to be adjusted, under changing patterns of weather variability and trends. The Garrett lab will work to develop generic platforms for evaluating crop disease risk under climate change. We (Sridhar, Virginia) will integrate human-induced climate and land use changes to quantify the surface energy balance under rainfed and irrigated agricultural systems. Todey (South Dakota) will continue providing work on climate-yield relationships and the changing impacts under changing climate conditions. Todey (SD) will continue work on tracking regional climate changes and their impact to agriculture in the state including increased moisture conditions and impacts on disease occurrence. Objective 3c. Risk Assessment Tools Risk assessment tools will be developed based on models and climate scenarios developed under the activities described above. We propose to develop web-based risk assessment tools that can be used to evaluate management strategies under different seasonal and long-term climate scenarios. Our goal is to enhance the ability of producers to adapt to seasonal climate shifts as they prepare for long-term climate change. We want to investigate the combined effects of annual and lower frequency cycles and long-term climate change scenarios with alternative management practices on diseases and insects, crop development and yield, and water use. Ultimately we want to identify management strategies that can help reduce crop and animal production risks associated with enhanced climate variability and change. Andresen (Michigan State Univ.) will apply the results from the modeling efforts in Objective 3a to examine seasonal patterns of crop water use and soil moisture and estimate the potential impacts of irrigation on corn production and weather-related production risks. Fraisse (Florida) will continue to maintain and improve AgroClimate (http://agroclimate.org), which is the Southeast Climate Consortium main mechanism for delivering climate information to growers in the region. Their focus will be in developing and implementing tools focusing on water and pest management under a changing climate. First, they will develop map-based tools to complement existing tools that are based on weather stations with detailed temporal resolution but limited spatial coverage. Second, they will focus on developing smartphone-based tools that better fit the information delivery preferences of our stakeholders. Logan (University of Tennessee) will assist in the development of some tools, knowing their potential for use in education and outreach, and she will evaluate existing tools for redundancy. Angel (Illinois) will work with other members of the committee to develop observation and model based products. Develop more products based on data collected from the Illinois Climate Network. Akyuz (North Dakota) will utilize the North Dakota Agricultural Weather Network observation capabilities: http://ndawn.ndsu.nodak.edu/ Objective 3d. Hydroclimatology response assessment. In order to better inform the predictive tools, historic analysis of hydrologic impacts due to climate variability and change at a local scale is very important. We (Sridhar, Virginia) will integrate climate data from observational and GCMs to quantify streamflow, soil moisture and other fluxes at the field scale and evaluate the impacts of warming trends. Our hypothesis is that if we are able to capture hydroclimatology and crop production using climate model outputs, it can then be extended to predict future conditions. Objective 4: Enhance the understanding of how stakeholders use weather and climate information in their management decision process Many NC1179 members have close ties to agriculture and interact with stakeholders frequently. Climate scientists on the committee are eager to share their knowledge of weather and climate with their constituents and, similarly, they are excited to learn from stakeholders who use weather and climate information. We propose to enhance our discussion with agricultural stakeholders in learning how weather and climate information can be integrated into their management decisions for application and value. Our duties and responsibilities provide unique opportunities for interaction and collaboration with diverse audiences and stimulate us to seek and provide creative methods of educating and learning from stakeholders. The state climatologists (and other climate scientists) on the committee are frequently asked to deliver talks at various meetings. These will be used to deliver new tools and findings. We will use surveys as these meetings specifically targeted at tools displayed to determine usage and feedback to contribute to further development of products. We will use information collected from surveys of attendees at various webinars within regions to drive potential tool development and feedback to the development. Fraisse (Florida) will continue to work with extension faculty and producers to understand their needs and learn about research gaps as well as provide basic climate literacy. They plan to do this at the regional level, in collaboration with land grant universities across the region. The AgWeatherNet Program (Washington State University, Hoogenboom) currently maintains a web portal (www.weather.wsu.edu) to disseminate weather data and weather data products to stakeholders. We continue to enhance this user interface based on the needs expressed by the stakeholders. Angel (Illinois) will continue to work closely with Extension and ag advisors on understanding their needs, as well as bring back data and research to address those needs. As a climate change speaker (Climate Reality Project and Climate Voices), Logan (University of Tennessee) will seek speaking engagements in the agriculture and horticulture sector. She will assist in developing a unified message and survey, as well as relevant audio-visuals. Objective 5: Disseminate the research outcomes and educate stakeholders on the potential effects of climate variability and climate change on agricultural production resource use and adaptation options through cooperation with university extension services, state climate offices and various federal partners. In spite of numerous investigations conducted during the last few years to evaluate the impact of climate change on crop performance and the subsequent impacts that this may have on global food, fiber and fuel supplies, the dissemination of research results in a way that offer solutions and a pathway to reducing vulnerability to climate change and variability has been lacking. In addition, climate science literacy must be elevated to help build sustainable agricultural communities that are resilient to climate variability and change and its extremes. The USDA is in process of developing seven new climate hubs throughout the United States to enhance work on climate-related issues for agriculture including short term and longer term climate services for people at local levels. Many of the members of this committee are state climatologists or other climate service providers. We propose to work directly with appropriate USDA Climate Hubs offering current research and existing climate services including more detailed automated station data, decision tools and additional summary and outreach products aimed at disseminating adaptation and mitigation strategies. Dennis Todey (South Dakota) has been in regular communication with Justin Derner, director of the Northern Plains Climate Hub. Justin Derner has also attended several recent meetings with NC-1179 members where regular communication of needs and abilities has been shared. We will also invite Dr. Derner and Dr. Jerry Hatfield from the Midwest Climate Hub to attend the annual meeting to continue planning how NC-1179 can interact with the existing structure of the climate hubs. Garrett (Kansas State University) will interact with USDA Climate Hubs to provide crop disease modeling expertise, building on on-going work with the USDA Climate Indicators project. Fraisse (Florida) is actively engaged in the discussions taking place under the recently established USDA – Southeast Regional Climate hub (SERCH: http://globalchange.ncsu.edu/serch/) to determine research agendas, dissemination methods, development of tools and stakeholder engagement. The Southeast Climate Consortium is a SERCH partner and AgroClimate is being contemplated as a platform to expand climate tools across the hub footprint. The Kansas agronomy researchers provided a support letter to the USDA Climate Hub in January 2014. They participated in teleconferences in April and May 2014 to explore the opportunities of mutual interest. Their next task is to visit the USDA Climate Hub in Oklahoma in June or July 2014. The Climate Hub for the Pacific Northwest is just getting started and has been assigned to the US Forest Service, based in Corvallies, Oregon. The AgWeatherNet Program at WSU (Hoogenboom) has been in discussion with the US Forest Service on how we can contribute and actively participate in the Climate Hub. Angel et al. (Illinois) will work closely with the USDA Midwest Climate Hub as well as the Midwestern Regional Climate Center. We will continue to engage stakeholders during off-growing season meetings and through webinars and workshops. Objective 5.1 Develop and conduct workshops and field days with producers to engage stakeholders and demonstrate adaptation and mitigation strategies Workshops will be designed to engage participants by discussing past production experiences and the impacts of climate variability to initiate discussions on how to prepare to an uncertain future. We will also explore past responses and/or adaptation strategies to past climatic conditions and discuss how climate information can support the development of adaptation strategies to projected climate change. These can also be used as evaluation capabilities for tools as mentioned in Objective 4. Additionally, delivery of tools, climate information and overall climate services will occur via collaborations among the state climatologists, regional climate centers, and others involved in the project. The AgWeatherNet Program at Washington State University (Hoogenboom) is funded by both research and extension. As such, it provides a unique opportunity to directly deliver research outcomes to stakeholders using the AgWeatherNet web portal as well as other traditional methodologies including presentations at local and regional meetings. Objective 5.2 Develop and deliver educational products such as FAQs, publications, and Web-based materials Educational materials to be developed will include: i) fact sheets, ii) posters on climate fundamentals made available for display, and iii) in-service extension and Certified Crop Advisors (CCA) training sessions. Emphasis will be placed on the changes that impact prevailing agricultural practices and row crops (soybean, corn) though much of the information will be widely applicable to other common row crops (wheat, sorghum, cotton, and peanuts) and vegetables. Akyuz (North Dakota) will continue to use a blog to communicate with stakeholders daily: http://www.ndsu.edu/ndscoblog/ Table summarizing participating stations and the Objectives they will work on: https://uwmadison.app.box.com/s/jrbvzgy2xyeg64worcjo (NIMSS not allowing attachments)Measurement of Progress and Results
Outputs
- Enhanced environmental monitoring systems will provide more accurate local weather data.
- New variables and climatologies will be developed that will enhance its utility as an input source for crop, soil, economic, and pest models.
- Improved data and weather-based data products that can be easily accessed by stakeholders including more regional products
- A reassessment of crop responses to soil and environmental influence based on new measured data
- Improved models that can better address crop production under a changing climate
- Output 6: Potential impact of climate change on food, fiber, and fuel production systems Output 7: New information on how producers and advisors access and utilize climate and weather information Output 8: New fact sheets and web sites will be developed for producers to use for crop decisions tools
Outcomes or Projected Impacts
- Improved yield forecasts will improve crop marketability and ultimately lead to better economic decision-making based on better information
- New/improved crop and disease models will provide producers and crop advisors tools to improve yield and protect crop loss
- Provide decision tools for better soil and water conservation practices to protect soil from erosion and water from impairments
- Knowledge of how climate change could potentially affect crop yields
- Educate policy-makers by providing scientifically-based assessments of climate change impact on major US food, fiber and fuel crops
- Outcome/Impact 6: Educate stakeholders and students about climate and climate change through access to the agricultural weather monitoring networks in participant states and the array of resources available on the network web sites
Milestones
(2015): Determine potential models for comparison in Objective 3 Examine potential regional collaboration opportunities on regional data collection Choose potential new tools for development Plan discussions with new USDA Climate Hubs for interaction Develop fact sheets and information on climate changes impacting agriculture Update NC 1179 web site(2016): Incorporate new variables into models that forecast disease, insect, weed and other stresses. Deliver information form new tools and modeled data at various workshops Gather evaluation information from workshops to feed further tool development Evaluation and comparison of modeled crop responses to environmental stress and responses to predicted climate change.
(2017): Continue inter-model comparisons Develop new products from regional network comparisons for web site and other delivery Update tools and products based on information gathered from workshops in first years of 5-year project
(2018): Evaluation and comparison of modeled crop responses to environmental stress and responses to predicted climate change. Evaluation of the socioeconomic responses to crop production systems in the region and the change dynamics that impact where people live and work.
(2019): Finalize tools and usage information for web site Determine long term residence for tools and data from project Update fact sheets with new data gathered
Projected Participation
View Appendix E: ParticipationOutreach Plan
Several members of the committee are state climatologists (in large agricultural states) or have extension appointments which demand significant outreach as part of their appointments. Inclusion of these results will be done through individual outreach work by each member is his or her state as part of their local work. On a wider-spread regional basis, we will utilize several outreach efforts. A new NC-1179 web site will be developed to update existing databases and illustrate new tools and forecast methods. This web site will link with web sites developed for the AFRI-funded U2U Useful to Usable (http://www.agclimate4u.org) and Corn Systems CAP (http://www.sustainablecorn.org) which include some related efforts. We will develop new extension fact sheets based on new forecast and tools. All members will publish in appropriate journals in the agriculture and climate communities. Outreach efforts will also be coordinated with the new USDA Climate Hubs in appropriate regions and existing outreach efforts among the state climatologists, NOAA, NOAA Regional Climate Centers and the National Drought Mitigation Center on monthly climate outlook webinars and quarterly climate update publications.
Organization/Governance
The recommended Standard Governance for multistate research activities include the election of a Chair, a Chair-elect, and a Secretary. All officers are to be elected for at least two-year terms to provide continuity. Administrative guidance will be provided by an assigned Administrative Advisor and a CSREES Representative.
Literature Cited
Literature Cited: Anonymous. 2007. Cooperative States Research, Education and Extension Service Strategic Plant for 2007-2012. USDA, Washington, 45 pp. Backlund, P., Janetos, A., and Schimel, D. 2008a. The Effects of Climate Change on Agriculture, Land Resources, Water Resources, and Biodiversity in the United States. Synthesis and Assessment Product 4.3, US Climate Change Science Program and Subcommittee on Global Change Research. 193 pp. Backlund, P., D. Schimel, A. Janetos, J. Hatfield, M.G. Ryan, S.R. Archer, and D. Lettenmaier, 2008b. Introduction. In: The effects of climate change on agriculture, land resources, water resources, and biodiversity in the United States. A Report by the U.S. Climate Change Science Program and the Subcommittee on Global Change Research. Washington, DC., USA, 362 pp. Bulatewicz, T., Yang, X., Peterson, J. M., Staggenborg, S., Steward, D. R. and Welch, S. M., Integration of agriculture, groundwater and economic models using the Open Modeling Interface (OpenMI): Application to the Ogallala Aquifer, AgSAP (Integrated Assesment of Agriculture and Sustainable Development) Conference 2009, Egmond aan Zee, The Netherlands, 2p, March 10-12, 2009. Coakley, S.M., Scherm, H., and Chakraborty, S. 1999. Climate change and plant disease management. Ann. Rev. Phytopathol. 37:399-426. Garrett, K.A., Dendy, S.P., Frank, E.E., Rouse, M.N., and Travers, S.E. 2006. Climate change effects on plant disease: Genomes to Ecosystems. Ann. Rev. Phytopathol. 44:489-509. Hatfield, J., K. Boote, P. Fay, L. Hahn, C. Izaurralde, B.A. Kimball, T. Mader, J. Morgan, D. Ort, W. Polley, A. Thomson, and D. Wolfe, 2008. Agriculture. In: The effects of climate change on agriculture, land resources, water resources, and biodiversity in the United States. A Report by the U.S. Climate Change Science Program and the Subcommittee on Global Change Research. Washington, DC., USA, 362 pp. Hudson, B.D. 1994. Soil organic matter and available water capacity. J. Soil and Water Conserv. 49:189-195. Kim, K.S., T.C. Wang, and X.B. Yang. 2005. Simulation of apparent infection rate to predict severity of soybean rust using a fuzzy logic system. Phytopathology. 10:1122-1131. Lobell, D.B., and G.P. Asner, 2003: Climate and management contributions to recent trends in U.S. agricultural yields. Science, 299, 1032. Lobell, D.B., and C. B. Field. 2007. Global scale climate-crop yield relationships and the impact of recent warming. Environ. Res. Lett. 2:1-7. McCornack, B.P., D.W. Rasdale, and R.C. Venette. 2005. Demography of soybean aphid (Homoptera: Aphididae) at summer temperatures. J. Econ. Entom. 97: 854-861. Onstad, D.W., S. Fang, D.J. Voegtlin. 2005. Forecasting seasonal population growth of Aphis glycines (Hemiptera: Aphididae) in soybean in Illinois. J. Econ. Entom. 98:1157-1162. Peterson, J. M and Steward, D. R., Groundwater economics: object oriented, integrated studies using the AEM, 5th International Conference on the Analytic Element Method, Manhattan, KS, pp.26-31, May 14-17, 2006. Muchow, R. C., T. R. Sinclair, and J. M. Bennett, 1990: Temperature and solar-radiation effects on potential maize yield across locations. Agronomy Journal, 82, 338-343. Sau, F., K. J. Boote, W. M. Bostick, J. W. Jones, and M. I. Minguez. 2004. Testing and improving evapotranspiration and soil water balance of the DSSAT crop models. Agron. J. 96: 1243-1257. Wolfe, D.W., Ziska, L., Petzoldt, C., Seaman, A., Chase, L., and Hayhoe, K. 2008. Projected change in climate thresholds in the Northeastern U.S.: Implications for crops, pests, livestock, and farmers. Mitig. Adapt. Strat. Glob. Change 13:555575. Ziska, L.H., J.R. Teasdale, and J.A. Bunce, 1999: Future atmospheric carbon dioxide may increase tolerance to glyphosate. Weed Sci, 47, 608-615. Literature Cited from NC 1018 participants Abrahamson, D.A., D.E. Radcliffe, J.L. Steiner, M.L. Cabrera, D.M. Endale and G. Hoogenboom. 2006. Evaluation of the RZWQM for simulating tile drainage and leached nitrate in the Georgia Piedmont. Agronomy Journal 98(3):644-654. Abrahamson, D.A., D.E. Radcliffe, J.L. Steiner, M.L. Cabrera, J.D. Hanson, K.W. Rojas, H.H. Schomberg, D.S. Fisher, L. Schwartz, and G. Hoogenboom. 2005. Calibration of the Root Zone Water Quality Model for simulating tile drainage and leached nitrate in the Georgia Piedmont. Agronomy Journal 96(6):1584-1602. Alfieri J G, D. Niyogi, M. A. LeMone, F. Chen, S. Fall, 2007, A Simple Reclassification Method for Correcting Uncertainty in Land Use/Land Cover Datasets Used with Land Surface Models, Pure and Applied Geophysics (Invited), 164, 1789 - 1809. DOI 10.1007/a00024-007-0241-4. Anothai, J., A. Patanothai, K. Pannangpetch, S. Jogloy, K.J. Boote, and G. Hoogenboom. 2008. Reduction in data collection for determination of cultivar coefficients for breeding applications. Agricultural Systems 96(1-3):195-206. Ashish, D., G. Hoogenboom, and R.W. McClendon. 2008. Land-use classification of mutispectral aerial images using artificial neural networks. International Journal of Remote Sensing. (Accepted for publication). Bannayan, M., and G. Hoogenboom. 2008a. Predicting realizations of daily weather data for climate forecasts using the non-parametric nearest-neighbor re-sampling technique. International Journal of Climatology 28(10):1357-1368. Bannayan, M., and G. Hoogenboom. 2008b. Weather Analogue: A tool for lead time prediction of daily weather data realizations based on a modified k-Nearest Neighbor approach. Environmental Modeling 23(6):703-713. Banterng, P., A. Patanothai, K. Pannangpetch, S. Jogloy, and G. Hoogenboom. 2006. Yield stability evaluation of peanut lines: a comparison of an experimental versus a simulation approach. Field Crops Research 96(1):168-175. Boken, V.K., C. E. Haque, and G. Hoogenboom. 2007. Predicting drought using pattern recognition, Annals of the Arid Zone 46(2):133-144. Boote, K. J., J. W. Jones, W. D. Batchelor, E. D. Nafziger, and O. Myers. 2003. Genetic coefficients in the CROPGRO-soybean model: Links to field performance and genomics. Agron. J. 95: 32-51. Bostick, W.M., V.B. Bado, A. Bationo, C. Tojo Soler, G. Hoogenboom, and J.W. Jones. 2007. Soil carbon dynamics and crop residue yields of cropping systems in the Northern Guinea Savannah of Burkina Faso. Soil and Tillage Research 93(1):138:151. Chen F., K. W. Manning, M. A. LeMone, S.B. Trier, J. G. Alfieri, R. Roberts, M. Tewari, D. Niyogi, T. W. Horst, S. P. Oncley, J. B. Basara, P. D. Blanken, 2007, Description and Evaluation of the Characteristics of the NCAR High-Resolution Land Data Assimilation System, Journal of Applied Meteorology and Climatology, 46, 694-713, DOI: 10.1175/JAM2463.1 Colunga-Garcia M, S Gage, and G Safir. 2005. Development and integration of temporal/spatial information into plant pest and disease forecasting systems. Survey Detection & Identification & Biological Control National Science Program/Center for Plant Health Science & Technology Annual Report 2004. p. 9-12. Colunga-Garcia M., P.R. Grace, S.H. Gage, G.P. Robertson, G.R. Safir. 2005. Urbanization and its Impact on the Carbon Sequestration Potential of Agroecosystems in the North Central Region. Third USDA Symposium on Greenhouse Gases & Carbon Sequestration in Agriculture and Forestry, March 21 - 24, 2005, Baltimore, MD. Dangthaisong, P., P. Banterng, S. Jogloy, N. Vorasoot, A. Patanothai and G. Hoogenboom. 2006. Evaluation of the CSM-CROPGRO-Peanut model in simulating responses of two peanut cultivars to different moisture regimes. Asian Journal of Plant Sciences 5(6):913-922. Deng, X., B.J. Barnett, G. Hoogenboom, Y. Yu, and A. Garcia y Garcia. 2008. Alternative crop insurance indices. Journal of Agricultural and Applied Economics 40(1): 223-237. Fang, H., S. Liang, G. Hoogenboom, J. Teasdale and M. Cavigelli. 2008. Corn yield estimation of remotely sensed data into the CSM-CERES-Maize model. International Journal of Remote Sensing 29(10):3011-3032. Feng, S., R. Oglesby, C. Rowe, D. Loope, and Q. Hu, 2008: Pacific and Atlantic SST influences on Medieval drought in North America simulated by Community Atmospheric Model. J. Geophys. Res. J. Geophys. Res., 113, D11101, doi:10.1029/2007JD009347. Feng, S, and Q. Hu, 2008: How the North Atlantic multidecadal oscillation may have influenced the Indian summer monsoon during the past two millennia. Geophys. Res. Lett., 35, L01707, doi:10.1029/2007GL032484. Feng, S., and Q. Hu, 2007: Changes in winter snowfall/precipitation ratio in the contiguous United States. J. Geophys. Res. 112, D15109, doi:10.1029/2007JD008397. Fraisse, C.W., N.E. Breuer, D.Zierden, J.G. Bellow, J. Paz, V.E. Cabrera, A. Garcia y Garcia, K.T. Ingram, U. Hatch, G. Hoogenboom, J.W. Jones and JJ. O'Brien. 2006. AgClimate: A climate forecast information system for agricultural risk management in the southeastern USA. Computers and Electronics in Agriculture 53(1):13-27. Gage, S.H., M. Colunga-Garcia, P.R. Grace, H. Yang, G.R. Safir, G.P. Robertson, A. Shortridge, A Prasla, A. Ali, S. Del Grosso, P. Wilkins, S. Rowshan. 2005. A Modeling Application Integrative Framework for Regional Simulation of Crop Productivity, Carbon Sequestration and Greenhouse Gas Emissions. Third USDA Symposium on Greenhouse Gases & Carbon Sequestration in Agriculture and Forestry, March 21 - 24, 2005, Baltimore, MD. Garcia y Garcia. A., L.C. Guerra, and G. Hoogenboom. 2008. Impact of generated solar radiation on simulated crop growth and yield. Ecological Modeling 210(3):312-326. Garcia y Garcia, A., and G. Hoogenboom. 2005. Evaluation of an improved daily solar radiation generator for the southeastern USA. Climate Research 29:91-102. Garcia y Garcia, A., G. Hoogenboom, L.C. Guerra, J.O. Paz, and C.W. Fraisse. 2006. Analysis of the interannual variation of peanut yield in Georgia using a dynamic crop simulation model. Transactions of the American Society of Agricultural and Biological Engineers 49(6):2005-2015. Gijsman, A.J., P.K. Thornton, and G. Hoogenboom. 2007. Using the WISE database to parameterize soil inputs for crop simulation models. Computers and Electronics in Agriculture 56:85-100. Gijsman A. J., G. Hoogenboom, W. J. Parton, and P. C. Kerridge. 2002. Modifying DSSAT crop models for low-input agricultural systems using a soil organic matter-residue module from CENTURY. Agron. J. 94:462-474. Grace, P.R., M. Colunga-Garcia, S.H. Gage, G.R. Safir, G.P. Robertson. 2005. The potential impact of climate change on North Central Regions soil organic carbon resources. Ecosystems. Grace, P.R., S.H. Gage, M. Colunga-Garcia, G.P. Robertson, G.R. Safir. 2005. Maximizing Net Carbon Sequestration in Agroecosystems of the North Central Region. Third USDA Symposium on Greenhouse Gases & Carbon Sequestration in Agriculture and Forestry, March 21 - 24, 2005, Baltimore, MD. Grant, R.H. and W. Gao. 2006. Distribution of diffuse UV-B radiation in a maize canopy. 17th Conf. on Biometeorol. and Aerobiology, Amer. Meteorol. Soc. Grant, R.H. and J.R. Slusser. 2005. Estimation of ultraviolet-A irradiance from measurements of 368-nm spectral irradiance. J. Atmos. & Ocean. Technology 22: 2853-2863. Grant, R.H. and J.R. Slusser. 2005. The measurement and modeling of broadband UV-A irradiance. In: G. Bernhard, J.R. Slusser, J.R. Herman and W. Gao, Eds., Symposium on UV Ground- and Space-based Measurements, Model, and Effects V, Proceedings of SPIE Vol. 5886. Green, M., Wang, D., Murphy, M., and J. Almendinger. 2005. Sensitivity of simulated stream water N and P concentrations and N:P ratios to precipitation regimes in a central Minnesota watershed. Eos Trans. AGU, 86(52), Fall Meet. Suppl., Abstract H31B-1302, 2005 AGU winter meeting, San Francisco, CA. Greenwald, R., M.H. Bergin, J. Xu, D. Cohan, G. Hoogenboom and W.L. Chameides. 2006. The influence of aerosols on crop production: A study using the CERES model. Agricultural Systems 89(2-3):390-413. Guerra, L.C., A. Garcia y Garcia, J.E. Hook, K.A. Harrison, D.L. Thomas, D.E. Stooksbury, and G. Hoogenboom. 2007. Irrigation water use estimates based on crop simulation models and kriging. Agricultural Water Management 89(3):199-207. Guerra, L.C., G. Hoogenboom, J.E. Hook, D.L. Thomas, V.K. Boken, and K.A. Harrison. 2005. Evaluation of the model EPIC for simulating on-farm irrigation applications. Irrigation Science 23:171-181. Gunal, H., and M.D. Ransom. 2005. Clay mineralogy, specific surface area and micromorphology of polygenetic soils from eastern Kansas. Archives of Agronomy and Soil Science 51:459-468. Gunal, H., and M.D. Ransom. 2006. Clay illuviation and calcium carbonate accumulation along a precipitation gradient in Kansas. Catena 68:59-69. Gunal, H., and M.D. Ransom. 2006. Genesis and micromorphology of loess-derived soils from central Kansas. Catena 65:222-236. Hartley, Paul, DeAnn Presley, and Michel D. Ransom. 2007. Mineralogy of polygenetic soils from the Bluestem Hills of East-Central Kansas, USA. In Annual Meetings Abstracts [CD-ROM]. ASA, CSSA, and SSSA, Madison, WI. Heinemann, A.B., A.de H.N. Maia, D. Dourado_Neto, K.T. Ingram and G. Hoogenboom. 2006. Soybean (Glycine Max [L.] Merr.) growth and development response to CO2 enrichment under different temperature regimes. European Journal of Agronomy 24(1):52-61. Heisler, G., B. Tao, J. Walton, R. Grant, R. Pouyat, I. Yesilonis, D. Nowak, and K. Belt 2006. Land cover influences on below-canopy temperatures in and near Baltimore, MD., In: Proceedings of the 6th Symposium on the Urban Environment, American Meteorological Soc. (In press). Herrero, M., E. Gonzalez-Estrada, P.K. Thornton, C. Quiros, M.M. Waithaka, R. Ruiz and G. Hoogenboom. 2007. IMPACT- Generic household-level databases and diagnostic tools for integrated crop-livestock analysis. Agricultural Systems 92 (1-3):240-265. Hoogenboom, G. 2006. Plant/soil interface and climate change: carbon sequestration from the production perspective. In: p. 93-126. [J.S. Bhatti, R. Lal, M. J.. Apps and M.A.. Price, editors] Climate Change and Managed Ecosystems. CRC Press. Boca Raton, Florida. Hoogenboom, G., J.W. Jones, P.W. Wilkens, C.H. Porter, W.D. Batchelor, L.A. Hunt, K.J. Boote, U. Singh, O. Uryasev, W.T. Bowen, A.J. Gijsman, A. du Toit, J.W. White, and G.Y. Tsuji. 2004. Decision Support System for Agrotechnology Transfer Version 4.0 [CD-ROM]. University of Hawaii, Honolulu, HI. Hosamane. S., 2005. Using National Weather Service forecasts and model output statistics (MOS) to forecast evapotranspiration. Masters Thesis. South Dakota State University. 120pp. Hu, Q., and S. Feng, 2008: Variation of the North American summer monsoon regimes and the Atlantic Multidecadal Oscillation. J. Climate, 21, 2371-2383. Hu, Q. and S. Feng, 2007: Decadal variation of the southwest U.S. summer monsoon circulation and rainfall in a regional model. J. Climate, 20, 4702-4716. Isard, S. A., Gage, S.H., Comtois, P. and Russo, J. 2005. Principles of the atmospheric pathway for invasive species applied to soybean rust. BioScience: 851-861. Jain, A., R.W. McClendon, and G. Hoogenboom. 2006. Freeze prediction for specific locations using artificial neural networks. Transactions of the American Society of Agricultural and Biological Engineers 49(6):1955-1962. Jones, J.W., G. Hoogenboom, C.H. Porter, K.J. Boote, W.D. Batchelor, L.A. Hunt, P.W. Wilkens, U. Singh, A.J. Gijsman, and J.T. Ritchie. 2003. DSSAT Cropping System Model. Europ. J. Agron. 18:235-265. Karlstrom, E.T., Oviatt, C.G., and. Ransom, M.D. 2007. Paleoenvironmental interpretation of multiple soil-loess sequence at Milford Reservoir, northeastern Kansas. Catena 72:113-128. Kim, K.R., Seem. R.C., Park. E.W., Zack, J.W., and Magarey, R.D. 2005 Simulation of grape downy mildew across geographic areas based on mesoscale weather data using supercomputer. Plant Pathol. J. 21:111-118. Lin, S., J.D. Mullen, and G. Hoogenboom. 2008. Farm-level risk management using irrigation and weather derivatives. Journal of Agricultural and Applied Economics 40(2):485-492. Lizaso, J.I. , K.J. Boote, C.M. Cherr, J.M.S. Scholberg, J.J. Casanova, J. Judge, J.W. Jones, and G. Hoogenboom. 2007. Developing a sweet corn simulation model to predict fresh market yield and quality of ears. American Journal of Horticultural Science 132(2):415-422. Lopez-Cedron, F. X, K. J. Boote, B. Ruiz-Nogueria, and F. Sau. 2005. Testing CERES-Maize versions to estimate maize production in a cool environment. Europ. J. of Agronomy 23: 89-102. Lopez-Cedron, F. X, K. J. Boote, J. Pineiro, and F. Sau. 2008. Improving the CERES-Maize model ability to simulate water deficit effects on maize production and yield components. Agron. J. 100:296-307. Ma, L., G. Hoogenboom, L. R. Ahuja, J.C. Ascough, and S.A. Saseendran. 2006. Development and evaluation of the RZWQM-CERES-Maize hybrid model for maize production. Agricultural Systems 87(3):274-295. Ma, L.,G. Hoogenboom, L.R. Ahuja, D.C. Nielsen and J.C. Ascough II. 2005. Evaluation of the RZWQM-CROPGRO Hybrid model for soybean production. Agronomy Journal 97(4):1172-1182. Magarey, R.D., Russo, J.M., Seem, R.C., and Gadoury, D.M. 2005. Surface wetness duration under controlled environmental conditions. Ag. For. Meterol. 128:111-122. Mullen, J.D., C. Escalante, G. Hoogenboom and Y. Yu. 2005. Determinants of irrigation farmers crop choice and acreage allocation decisions: Opportunities for extension delivery service. Journal of Extension [on-line] 43(5). Available at http://www.joe.org/joe/2005october/rb3.shtml. Nelson, B.R., W.F. Krajewski, J.A. Smith, E. Habib and G. Hoogenboom. 2005. Archival precipitation data set for the Mississippi river basin: evaluation. Geophysical Research Letters 32: L19403,doi:10.1029/2005GL023334. Olatinwo R.O., J.O. Paz, S.L. Brown, R.C. Kemerait, A.K. Culbreath, J.P. Beasley, Jr., and G. Hoogenboom. 2008. A predictive model for spotted wilt epidemics in peanut based on local weather conditions and the tomato spotted wilt virus risk index. Phytopathology 98(10):1066-1074. Olson, K.R., T.E. Fenton, N.E. Smeck, R.D. Hammer, M.D. Ransom, C.W. Zanner, R. McLeese, and M.T. Sucik. 2005. Identification, mapping, classification, and interpretation of eroded Mollisols in the U.S. Midwest. Soil Survey Horizons 46:23-35. Olson, K.R., T.E. Fenton, N.E. Smeck, R.D. Hammer, M.D. Ransom, C.W. Zanner, R. McLeese, and M.T. Sucik. 2005. Proposed modifications of mollic epipedon thickness criteria for eroded conditions and potential impacts on existing soil classifications. Soil Survey Horizons 46:39-47. Pathak, T.B., C.W. Fraisse, J.W. Jones, C.D. Messina, and G. Hoogenboom. 2007. Use of global sensitivity analysis for CROPGRO cotton model development. Transactions of the American Society of Agricultural Engineers 50(6):2295-2302. Paz, J.O., C.W. Fraisse, L.U. Hatch, A. Garcia y Garcia, L.C. Guerra, O. Uryasev, J.G. Bellow, J.W. Jones, and G. Hoogenboom. 2007. Development of an ENSO-based irrigation decision support tool for peanut production in the southeastern US. Computers and Electronics in Agriculture 55(1):28-35. Prabhakaran, T., and G. Hoogenboom. 2008. Evaluation of the weather research and forecasting model for two frost events. Computers and Electronics in Agriculture 64:234-247. Presley, DeAnn Ricks. 2007. Ph. D. Dissertation. Genesis and spatial distribution of upland soils in east central Kansas. Kansas State Univ. Presley, DeAnn, Michel D. Ransom, and Paul Hartley. 2007. Mineralogy and stratigraphy of polygenetic soils on different geomorphic surfaces of the Bluestem Hills of East-Central Kansas. In Annual Meetings Abstracts [CD-ROM]. ASA, CSSA, and SSSA, Madison, WI. Saseendran, S.A., L. Ma, R. Malone, P. Heilman, L. R. Ahuja, R. S. Kanwar , D. L. Karlen, and G. Hoogenboom. 2007. Simulating management effects on crop production, tile drainage, and water quality using RZWQM-DSSAT. Geoderma 140:297-309. Schmitz, H. and R.H. Grant 2006. Precipitation and dew in soybean canopies: An In depth look at the differences in wetness with canopy height.. 17th Conf. on Biometeorol. and Aerobiology, Amer. Meteorol. Soc. Seem, R.C. 2004. Forecasting plant disease in a changing climate: A question of scale. Can. J. Plant Pathol. 26:274-283. Shank, D.B., G. Hoogenboom, and R.W. McClendon. 2008. Dew point temperature prediction using artificial neural networks. Journal of Applied Meteorology and Climatology 47(6):1757-1769. Shank, D.B., R.W. McClendon, J.O. Paz, and G. Hoogenboom. 2008. Ensemble artificial neural networks for prediction of dew point temperature. Applied Artificial Intelligence 22(6):523-542. Smith, B.A., R.W. McClendon and G. Hoogenboom. 2006. Improving air temperature prediction with artificial neural networks. International Journal of Computational Intelligence 3(3):179-186. Soltani, A., and G. Hoogenboom. 2007. Assessing crop management options with crop simulation models based on generated weather data. Field Crops Research 103:198-207 Staggenborg, S.A., M. Carignano, and L. Haag. 2007. Predicting soil pH and buffer pH with a real-time sensor. Agron. J. 99:854-861. Staggenborg, S.A., W.B. Gordon, K.C. Dhuyvetter. 2007. Grain sorghum and corn comparisons: Yield, economic and environmental responses. Agron. J. (accepted). Staggenborg, S.A., and R.L. Vanderlip. 2005. Crop simulation models can be used as dryland cropping systems research tools. Agron. J. In Press. Suleiman, A.A., and G. Hoogenboom. 2007. Comparison of Priestley-Taylor and Penman-Monteith for daily reference evapotranspiration estimation in Georgia. Journal of Irrigation and Drainage Engineering 133(2):175-182. Suleiman, A..A., C.M. Tojo Soler, and G. Hoogenboom. 2007. Evaluation of FAO-56 crop coefficient procedures for deficit irrigation management of cotton in a humid climate. Agricultural Water Management 91(1-3):33-42. Suriharn, B., A. Patanothai, K. Pannangpetch, S. Jogloy, and G. Hoogenboom. 2008. Yield performance and stability evaluation of peanut breeding lines with the CSM-CROPGRO-Peanut model. Crop Science 48(4):1365-1372. Todey, D.P. and C. Shukla, 2005. Climate factors impacting productivity and yield trends in the Midwest. 15th Annual Conference on Applied Climatology. Savanna, GA. American Meteorological Society. Tojo Soler, C.M., P.C. Sentelhas, and G. Hoogenboom. 2007 Application of the CSM-CERES-Maize model for planting date evaluation and yield forecasting for maize grown off-season in a subtropical environment. European Journal of Agronomy 27(2-4):165-177. Tojo Soler, C.M., N. Maman, X. Zhang, S.C. Mason and G. Hoogenboom. 2008. Determining optimum planting dates for pearl millet for two contrasting environments using a modeling approach. Journal of Agricultural Science 146(4):445-459. United States Department of Energy. 2007. Fact Sheet: Energy Independence and Security Act of 2007 http://www.whitehouse.gov/news/releases/2007/12/20071219-1.html (Accessed on October 24, 2008) White, J.W., K.J. Boote, G. Hoogenboom, and P.G. Jones. 2007. Regression-based evaluation of ecophysiological models. Agronomy Journal 99(2):419-427. White, J.W., and G. Hoogenboom. 2005. Integrated viewing and analysis of phenotypic, genotypic, and environmental data with GenPhEn arrays. European Journal of Agronomy 23:170-182. White, J.W, G. Hoogenboom, and L.A. Hunt. 2005. A structured procedure for assessing how crop models respond to temperature. Agronomy Journal 96(2):426-439. White, J.W., G. Hoogenboom, P.W. Stackhouse and, J. M. Hoell. 2008. Evaluation of NASA satellite- and assimilation model-derived long-term daily temperature data over the continental US. Agricultural and Forest Meteorology 148(10):1574-1584.