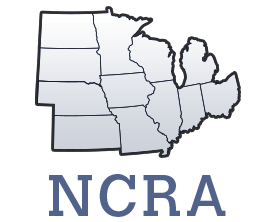
NC1191: Weeds as Phytometers in a Changing Environment
(Multistate Research Project)
Status: Inactive/Terminating
NC1191: Weeds as Phytometers in a Changing Environment
Duration: 10/01/2011 to 09/30/2016
Administrative Advisor(s):
NIFA Reps:
Non-Technical Summary
Statement of Issues and Justification
Weed management strategies that have been developed over the last fifty years will be substantially challenged by anticipated changes to the climate. It is critical that adaptive strategies be developed to meet new realities facing U.S. growers. Weeds are found in all agricultural systems, and although they must be managed to prevent crop losses, their wildness offers unique ecosystem services to aid climate mitigation and adaptation. The keen response of weeds to environmental signals makes them inexpensive yet powerful environmental sensors, or phytometers. This characteristic of weeds may be used, in combination with bioclimatic scaling functions, to assist precision timing of weed management practices. Improved timing of weed management has been demonstrated to reduce crop yield losses to weed interference. We propose to demonstrate that Hopkins' Bioclimatic Law can be used to easily and accurately predict weed phenology across the North Central region of the U.S., thereby improving weed management outcomes.
The North Central Weed Biology Working Group has a decades-long history (under such project numbers as NC202 and NC1026) of productive regional experimentation with many highly-cited publications to its credit. Our group has successfully completed many projects of the scale and scope described in the current project plan. Distributing measurements of weed phenology across the landscape at a regional scale is a critical part of verifying the use of Hopkins' Law as a predictive tool for timing weed management in the North Central Region.
The research proposed here will support the long-range improvement in and sustainability of U.S. agriculture and food systems by improving the ability of agriculture to adapt to, and mitigate, global climate change and maintain agroecosystem function and biodiversity in the face of a changing global environment. A novel outcome of this project in support of these goal is the development of an accurate bioclimatic scaling function in support of precision timing of weed management in current and future climates.
Related, Current and Previous Work
As anthropogenic climate change subjects global agricultural production to increasing levels of environmental variability (Intergovernmental Panel on Climate Change, 2007), much attention is focused on the ability of cereal crops to adapt to novel environmental conditions. We believe that an equally important contribution to climate adaptation and mitigation in agriculture may come from non-crop agricultural flora: weeds.
Weeds are ubiquitous in agricultural ecosystems (Holm et al., 1997), reviled yet grudgingly admired for their ability to adapt to a seemingly endless variety of environmental signals and stresses (Clements et al., 2004). The key to the superior ability of weeds to adapt to natural and anthropogenic environmental stresses is their enormous genetic variability (Wang et al., 1995, Tranel et al., 2004), undiminished by selective breeding efforts that have narrowed genetic diversity in their crop counterparts over the decades. Weeds are wild plants that happen to inhabit agricultural ecosystems. Humans need to manage weeds to protect crop productivity, but we may also take advantage of their wildness to deliver novel ecosystem services that help agriculture adapt to, and mitigate, climate change.
Phenology. Recently, global climate change has intensified scientific interest in historical phenological records. Because temperature is a primary driver of plant phenology (especially seed germination and flowering) (Rathcke & Lacey, 1985), records of plant phenology can function as proxies for records of temperature (Menzel, 2000). Numerous studies of plant phenological records have documented earlier onset of spring phenological events in response to gradual global warming (Sparks & Carey, 1995, Menzel, 2000, Schwartz & Reiter, 2000, Parmesan & Yohe, 2003). Analysis of first leaf appearance and first bloom for common lilac (Syringa vulgaris) and Red Rothomagensis lilac clones (Syringa chinensis) showed an average 5-6 d earlier onset of spring in North America from 1959-1993 (Schwartz and Reiter 2000). These observations agree with the assessment of the Intergovernmental Panel on Climate Change (2007) that global temperatures are rising as a result of anthropogenic influences on the earths atmosphere.
Plant phenology is primarily governed by temperature, photoperiod, soil moisture and complex interactions between these factors (Rathcke & Lacey, 1985). Under anthropogenic climate change, plant phenology will be impacted by changes in these environmental factors, along with changes in atmospheric CO2 concentrations, alterations in species interactions caused by differential responses to environmental factors, and changes in nutrient cycling (Badeck et al., 2004). Plant phenological responses effectively integrate numerous environmental factors. Thus, predicting plant phenology may provide a way to track and predict key environmental perturbations occurring as a result of climate change (Cleland et al., 2007).
Plant phenology can be predicted, or modeled, using a variety of approaches that range from empirical to mechanistic and encompass scales from individual plant to continental. For annual plants, including many crops and weeds, mechanistic models often describe seed germination and seedling emergence as function of thermal time (TT) or hydro-thermal time (HTT) (Forcella et al., 2000, Bradford, 2002, Rowse & Finch-Savage, 2003). For perennial species, phenological events such as appearance of first leaf have also been modeled based on accumulated TT (Hickin & Vittum, 1976). Mechanistic models have provided reasonable retrospective fits to data collected at specific site-years (Grundy et al., 2003, Schutte et al., 2008); however, such models have seldomly been tested at broader spatio-temporal scales. Also, mechanistic models based on biophysical responses to environmental variables often require inputs that can be difficult to obtain, particularly as the sophistication and complexity of the model increases (Cardina et al., 2007).
Consequently, empirical approaches may provide more tractable predictions of plant phenological events for many species and across wide spatio-temporal scales. Bioclimatic scaling functions based on latitude, longitude, and elevation have been developed to interpolate plant phenological events such as first leaf appearance or initiation of flowering across broad spatio-temporal scales. Examples of such scaling approaches include the spring index (SI), an empirical scaling factor based on variation of lilac phenological events developed from 100 years of data from numerous US locations (Schwartz & Reiter 2000) and Hopkins' Bioclimatic Law (HBL), which spatially interpolates relative bioclimatic phenology as a function of latitude, longitude, and elevation (Hopkins 1919). Hopp (1976) used HBL to predict phenophases (first bloom, full bloom) for Red Rothamagensis lilac phenology data collected in the US from 1968-1974. For five out of seven years of the study, HBL resulted in predicted dates of phenological events within two days of the actual event for broad geographical area. Spatial statistical analyses of HBL predictions of lilac phenology indicate structural problems with the function (see 'Preliminary results'). We hypothesize that an improved bioclimatic scaling function, HBL.2, may i) achieve a greater degree of accuracy in predicting lilac phenology, and ii) be used to accurately predict weed phenology in cereal crops.
Preliminary results. Almost a century ago, Hopkins (1919) described a procedure for calculating the relative timing of two phenological events which has become known as Hopkins Bioclimatic Law (HBL). Using maps with temperature-sum contours called isophanes he could determine an exchange rate between geographical variables, latitude, longitude and elevation, and event timing: a shift of 4 days occurred for every 1 deg latitude (q), 5 deg of longitude (f) and 400 ft of elevation (r), later northward, eastward and upward in the spring and early summer and the reverse in late-summer and autumn. Mathematically, if is the day of year of an event taking place at position , then HBL predicts:
This law originally allowed for farmers to time the planting of winter wheat to minimize Hessian fly invasions, by extrapolating the information on Hessian fly emergence/ disappearance at one location to other locations in the Eastern US.
For organisms adapted to develop and grow in relation to accumulated thermal units (i.e. as a linear and cumulative function of temperature above some base or threshold, growing-degree days or GDD), the linearity of HBL stems from the approximate flatness of the GDD surface with respect to each of the coordinates. In the case of latitude and altitude, changes are due to the relative positions of the earth and the sun and the adiabatic cooling of air respectively. The linear response of GDD to longitude reflects North American continental effects on local climate.
As a first step in evaluating the suitability of HBL as a continental-scale bioclimatic scaling function, our group (the NC1026 Regional Weed Biology Working Group, with over 30 years of collaborations) compared empirical observations of lilac first bloom (Syringa x chinensis "Red Rothomagensis") from the National Phenology Network Spring Index data archive (Schwartz & Reiter, 2000) with predictions of deviations in the timing of this event relative to Madison, WI. There was good agreement between the observed and predicted values (c2621 = 1629; P > 0.05), and the least squares linear regression between these variables was strong (Predicted Value = 0.85*Observed, F1,621 = 1574, P < 0.0001, R2 = 0.72). Inspection of the residuals of this regression indicated that the fit was well-behaved with respect to latitude, but there was still information left in the residuals with respect to longitude and elevation. The residuals were significantly correlated with longitude (r = -0.49, P< 0.0001) and elevation (r = 0.79, P < 0.0001); factoring out elevation effects from the relationship between the residuals and longitude did not entirely account for the correlation between them (r = 0.14, P < 0.05).
These preliminary results suggest that an improved version of HBL (hereafter referred to as HBL.2) may be created which more fully accounts for elevation and longitude effects on phenology.
Objectives
-
Improve predictions of weed phenology in the U.S., particularly emergence and flowering, using bioclimatic models to provide ecosystem services in cereal production agriculture supporting adaptation to a changing climate. These services are based on precision-timed weed management to reduce crop yield losses due to weed interference.
-
Develop preliminary phenology data set with which to seek further extramural funding for the research activities of the NC1026 Working Group (a proposal was submitted by the group to the FY 2010 AFRI competition, and is currently in review) to increase the inference space and utility of the phenological predictions (both number of weed species represented and geographic area included).
Methods
The proposed work spans a range of empirical and modeling activities in support of more sensitive environmental signal detection with weed phytometers, with the central goal of enabling agricultural systems to mitigate and adapt to anthropogenic climate change. Objective 1: Improve local and regional predictions of weed phenology under current and future climates. Hypotheses H1: Weed phenology is spatiotemporally autocorrelated in a predictable way at regional and continental scales. H1a: A bioclimatic scaling function using latitude, longitude and elevation as its inputs may be used to predict weed phenological benchmarks at numerous satellite sites from empirical observations of weed phenology at a core site. Activity 1.1. Quantify weed phenological responses to environmental signals at broad geographic scales. Description. This activity consists of empirical studies of the phenology of 4 weed species over a three year period at 10 locations within 10 states in the northern half of the USA. Weed phenology has been quantified for one or two species over many site-years, or several species across few site years, but not for many species over many site-years. The NC1026 working group has used the basic study design described below for several successfully completed projects (Forcella et al., 1992, Lindquist et al., 1996, Forcella et al., 1997, Davis et al., 2005), therefore we have good reason to believe the methods are feasible at the intended scale and project scope. Methods. At a regional scale, the study will be performed as a nested design with the following nesting structure: state(year(replication))). Each of the 10 participating states (IL, IN, KS, MI, MN, ND, NE, OR, SD, WI) will host a site on a university agricultural research farm in a field previously planted to corn. At each site, we will record the phenology of common and local accessions of 2 winter annual and 2 summer annual weed species common to all locations in four replications of a common garden study laid out in a randomized complete block design. This treatment structure results in a total of 32 experimental units per site (4 reps x 2 life histories x 2 species/life history x 2 accessions/species). Experimental units will consist of 1 m2 quadrats with two types of measurements taken within: seedling emergence, and monitoring of flowering and seed maturation within the quadrat as a whole. The 1 m2 quadrat will also contain four evenly spaced rows (0.25 m between rows) of a given experimental species seeded at a species-specific number of seeds row-1 (based on literature reports of typical 1st year germination percent, to achieve a target population of 30 plants per row). Small-seeded species will be suspended in a laponite gel prior to planting, and extruded from a syringe to produce even seeding rates. Experimental blocks will be 9 m wide by 11 m long, with 1 m2 quadrats centered on a 1 m grid pattern of 4 rows by 5 columns. Since the main point of Objective 1 is to determine whether a bioclimatic scaling function can be used to accurately predict relative shifts between the timing of phenological events at different locations for a given weed species under uniform management conditions, we will maintain experimental areas in a bare fallow. Weed species will be included in the study on the basis of a) economic importance in cereal cropping systems, and b) wide distribution in the northern U.S. according to the USDA PLANTS database. Summer annual weed species will include: common lambsquarters (Chenopodium album L.), and velvetleaf (Abutilon theophrasti Medik.). Winter annual weed species will include: field pennycress (Thlaspi arvense L.) and horseweed (Conyza canadensis L.). For each weed species, a common and local seed accession will be buried at each location. Viable seed of local accessions will be collected by gently shaking ripe inflorescences of mature plants from a single population within 50 km of each site. Common seed accessions will be collected from the research site where a given species is judged by the group to be the most prolific, distributed to all sites, and immediately buried (a sample of the seed will also be sent to Morris, MN, for seed lot characterization). Because we have winter and summer annual weeds in this study, several species within each life history, and numerous locations, we will have locally-dictated burial times. We have chosen this approach, rather than standardizing seed burial times by storing seeds, as this allows the plant life cycle to proceed with as few interruptions, and potential alterations of phenology, as possible. To accommodate this approach, we will accumulate environmental signals, such as thermal time, from seed burial as T0. We will initiate Activity 1.1 in Spring 2011 with seed collection and burial for winter annual weed cohort 1, the first of three annual cohorts to be followed for phenology measurements. Summer annual cohort 1 will be collected and buried in Fall 2011. Cohorts 2 and 3 will follow a similar temporal pattern in years 2 and 3 (2012 and 2013) of the study. Phenology measurements will include seedling emergence over time, floral initiation (onset of anthesis), and species-specific measures of seed maturation (Table 1). Weed seedling emergence will be recorded non-destructively twice a week for the first 12 emerging seedlings, after which seedling counts will be destructive. The first 12 emerging seedlings will be marked with a toothpick to which a waterproof label with an identification number has been attached, and seedling mortality noted. Floral initiation and seed maturation for each marked individual in each plot will be recorded using the measures indicated below. Species-specific events denoting phenological benchmarks: SPECIES: A. theophrasti - LIFE HISTORY: summer annual - ANTHESIS: 1st open petals - SEED MATURATION: 1st abscission layer SPECIES: C. album - LIFE HISTORY: summer annual - ANTHESIS: 1st bloom - SEED MATURATION: 1st brown or black seed SPECIES: C. candaensis - LIFE HISTORY: winter annual - ANTHESIS: 1st fuzzball - SEED MATURATION: shake test SPECIES: T. arvense - LIFE HISTORY: winter annual - ANTHESIS: 1st open flower - SEED MATURATION: brittle silique Each study site has a university-operated weather station recording hourly air temperature, soil temperature, precipitation, wind and solar radiation. These inputs can be converted into various accumulators for measuring phenological time, including growing degree days, hydrothermal time, and day length. Data on soil physical properties (including bulk density, organic matter, and percent sand and silt) will be collected for each location in support of thermal time calculations. Analysis. Linear mixed effects models, in combination with variance partitioning, will be used to determine the factor level at which most of the variation in phenology for a given species occurs. We will also develop multiple regression models to determine which environmental signals (thermal time, hydrothermal time, or day length) a particular species is most sensitive to. Data for use in tests of the original and revised bioclimatic scaling function (Activity 1.3) will be aggregated for each species within site-years. Pitfalls/Limitations. There are two potential pitfalls associated with this objective. First, for the purposes of bioclimatic scaling, we need all locations to collect data in the same study-years. If a particular site has difficulty in finding personnel, they may be short staffed during a given growing season. However, all co-PDs have agreed to collect and establish the first cohort regardless of their staffing status. Second, not all sites may have all 4 species. If a species is not present within 50 km of a given location, seed accessions will be obtained from the nearest available population. Activity 1.2. Refining Hopkins' Law, a bioclimatic scaling function. Description. We will fine-tune HBL through an iterative statistical modeling approach, using the North American Lilac Phenology data archive, hosted by the National Phenology Network (NPN) (http://www.usanpn.org/about/phenology?q=content/lilachoneysuckle-program), as our training data set. This data set contains several hundred site-years of phenology data distributed across many locations in the conterminous U.S. over a 50 yr period. The data achieve a balance between long time series and spatial coverage needed to detect widespread climate-driven changes in phenological events (Jackson, 1966, Schwartz & Reiter, 2000). Because there is a common clonal lilac cultivar used at all observer stations, genotype by environment interactions will not confound phenology predictions tuned to the training data. Methods. We will fit competing versions of the HBL model to the NPN lilac data, as linear mixed multiple regressions. Competing models will explore the following questions: are additional parameters necessary for interactions between latitude, longitude and elevation? Are the coefficients for HBL out of date? When bi are allowed to float, do parameter values change? We will also fit alternate bioclimatic scaling functions to the lilac data that explicitly account for changes in the values of thermal time, hydrothermal time and day length. The environmental data necessary to derive these variables for observer sites will be obtained through NOAA weather stations associated with each of the locations. Model selection will proceed by maximum likelihood methods, with minimization of AIC and BIC as criteria for model parsimony (Burnham & Anderson, 2002). Once a candidate model for HBL.2 is selected for best fit to the lilac data, we will further evaluate its fit by statistical examination of the residuals to make sure no more information remains. Pitfalls/Limitations. It is possible that, despite our best efforts, we won't be able to improve the prediction accuracy of Hopkins' Law for any of the species included in the study. It is also possible that it will work quite well for species attuned to some environmental signals but not those that are attuned to others. For example, the phenology of a species may be very sensitive to day length, but not to temperature. Hopkins Law is built around the spatial autocorrelation between these two signals through its use of latitude and altitude as inputs. In cases where the autocorrelation between these signals breaks down, as with high altitudes, it may be that the prediction accuracy of Hopkins Law is not acceptable. To address this possibility, we will create alternate bioclimatic scaling functions that take specific environmental accumulators, including thermal time, hydrothermal time and day length, as inputs. These functions will allow us to determine whether specific environmental signals provide a more accurate predictive capacity for phenological benchmarks than their proxies, as represented by geographic location. Activity 1.3. Evaluate performance of original and revised bioclimatic scaling functions for predicting weed phenology of various species at a regional scale. Description. In this activity, we will evaluate the ability of HBL and HBL.2 to make predictions of the relative time of occurrence of various phenological events for common and local accessions of 2 summer annual and 2 winter annual weeds, using the 30 site-years of geographically distributed phenology data collected in the northern half of the U.S. in Activity 1.1 as a verification data set. Methods. Depending upon the inputs required by HBL.2, we will generate a data frame with the necessary environmental variables for all site-years of data in the weed verification data set. We will then use HBL and HBL.2 to make predictions of the relative time at which different phenological events (50% seedling emergence, anthesis, seed maturation) occur. These predictions will be compared to empirical measurements of the timing of these events using model selection criteria (Pearson's chi-squared, Root Mean Square Error, AIC) across several evaluative dimensions. First, how well do observed and predicted values compare across the entire data set, including all species and accessions? Second, can the phenology of every species in the data set be described with either HBL or HBL.2 (i.e., on the basis of geographic coordinates alone) or are more specific accumulator functions required for some species? Do the best-fit functions for individual species perform equally well across the sampling area, or are there geographic sub-areas where a given function performs particularly well? Finally, is there an important effect of genetic variation due to accession on the predictive capacity of the bioclimatic scaling functions? The revised bioclimatic scaling function(s) will be used to re-parameterize the Weedometer (http://weedometer.net), an online application for predicting weed phenology at any given location in the U.S. The Weedometer operates in three stages. First, it aggregates weather data (temperature and precipitation) for Madison, WI for a given growing season. Second it uses hydrothermal time models to generate real-time estimates of weed phenology for 70 economically important weed species. Third, it uses HBL to scale the predictions of weed phenology to other locations in the U.S. Depending upon the results of our study, we will either continue to use HBL in the Weedometer or replace it with HBL.2, should it prove superior. Pitfalls/Limitations. The 4 weed species used in this evaluation make up only a small subset of the total number of species currently included in the Weedometer. If the approach outlined in this project proves successful, we will conduct future studies to verify phenological predictions for the other species. Hazards. There are no hazards associated with objective 1. Objective 2: Prepare and submit a proposal for extramural funding of the research Activities of the NC1026 Weed Biology Working Group Activity 2.1: Use preliminary phenological data gathered in Activities 1.1 through 1.3 to refine the proposal submitted to AFRI in the FY 2010 funding cycle.Measurement of Progress and Results
Outputs
- Obtain data for quantification of weed phenological responses to environmental signals at broad geographic scales.
- Develop a revised and improved Hopkins' Bioclimatic Law, a bioclimatic scaling function for improved predictions of weed phenology in the U.S.
- Evaluate performance of the original and revised bioclimatic scaling functions for predicting weed phenology of various species at a regional scale.
- Prepare and submit a proposal for extramural funding (AFRI) of the research Activities of the NC1026 Weed Biology Working Group. Preliminary data for this larger proposal will be developed from this regional project.
Outcomes or Projected Impacts
- Improved predictions of weed phenology in the U.S., particularly emergence and flowering, using bioclimatic models to provide ecosystem services in cereal production agriculture supporting adaptation to a changing climate. These services will help in developing precision-timed weed management to reduce crop yield losses due to weed interference.
- We expect to have an evaluation of Hopkins Bioclimatic Law (HBL) in comparison to the revised Hopkins Bioclimatic Law (HBL.2) by year four of the project, and a revised version of the Weedometer soon thereafter. The Weedometer is currently used by producers in support of weed management decisions, commercial applicators in support of scouting efforts and scientists for research purposes. We anticipate the improved Weedometer will receive 50% more hits per season by a wider range of clientele, and that the affected growers will improve the timeliness of their weed management operations, resulting in a 10% decrease in crop yield losses due to weeds.
- Development of preliminary phenology data set with which to seek further extramural funding for the research activities of the NC1026 Working Group (i.e., AFRI) to increase the inference space and utility of the phenological predictions (both number of weed species represented and geographic area included).
Milestones
(1):tivity 1.1 - spring: collect/bury winter annual weed seeds (cohort I); fall: collect/bury summer annual seeds (cohort I), monitor winter annual weed seedling emergence (cohort I) Activity 1.2 (ongoing): statistical modeling activities to improve HBL and create HBL.2(2):tivity 1.1 - spring: collect/bury winter annual weed seeds (cohort II), monitor winter annual weed flowering and seed development (cohort I) and summer annual seedling emergence (cohort I); fall: collect/bury summer annual seeds (cohort II), monitor winter annual weed seedling emergence (cohort II) and summer annual flowering and seed development (cohort I). Activity 1.2 Milestones: statistical modeling activities to improve HBL and create HBL.2 finished by end of Year 2. Preparation of related manuscript for peer-reviewed journal.
(3):tivity 1.1 - spring: collect/bury winter annual weed seeds (cohort III), monitor winter annual weed flowering and seed development (cohort II) and summer annual seedling emergence (cohort II); fall: collect/bury summer annual seeds (cohort III), monitor winter annual weed seedling emergence (cohort III).
(4):tivity 1.1 - spring: monitor winter annual weed flowering and seed development (cohort III) and summer annual seedling emergence (cohort II); fall: monitor summer annual flowering and seed development (cohort III). Milestones: weed phenology measurements complete. Preparation of related peer-reviewed manuscripts. Activity 1.3 (ongoing): Compare performance of HBL and HBL.2 in predicting temporal shifts in weed phenology events among experimental locations.
(5):tivity 1.3 (ongoing): Finish comparing performance of HBL and HBL.2 in predicting temporal shifts in weed phenology events among experimental locations. Milestones: evaluation of bioclimatic scaling function performance complete. Preparation of related peer-reviewed manuscript. Revised version of Weedometer, making use of HBL.2, posted to http://weedometer.net.
Projected Participation
View Appendix E: ParticipationOutreach Plan
We have been, and will continue to be, very visible to a wide range of scientific and end-user groups in the North Central Region and across the country. Our group has sponsored symposiums at the regional and national Weed Science Society meetings and has actively presented results of our research to various user groups through the hard work of individuals within each state. Data have been presented at North Central Weed Science Society annual meetings each year by individual state representatives, some presenting state-specific data, other presenting a regional summary of data. We will also continue to actively write manuscripts for peer reviewed publications.
Our primary goal is to support the development of applied weed management decision support systems through innovative regional research that addresses long-term decision making. Members of NC 1026 have worked with the decision support system, WeedSOFT in the past and will now work on improving the phenological model Weedometer. The Weedometer is currently used by producers in support of weed management decisions, commercial applicators in support of scouting efforts and scientists for research purposes to help improve grower weed management decisions.
Most notably, NC 1026 has provided the framework for cooperative research among weed scientists, microbiologists, economists, and modelers. This type of multi-disciplinary interaction is critical for addressing complex questions such as the objectives of NC 1026. This interaction has stimulated numerous research ideas and has broadened many of the scientists' views of their individual research efforts. This type of interaction would not have occurred without the support of NC 1026.
The success of NC 1026 serves as a model for regional research efforts. The participating scientists developed research protocols that were conducted in multiple environments, leading to a database much more powerful than would have been possible working independently. The result is the generation of valuable biological and environmental data in a very efficient manner. This group has also been active in trying to obtain extramural funding from other sources, such as AFRI.
Organization/Governance
The project will be planned and executed by a Technical Committee composed of one representative from each state. However, each experiment station and federal agency may have more than one representative on the committee where the scope of the project involves more than one subject matter discipline. The administrative advisor and CSRS-USDA representatives are non-voting but retain veto power.
Members of the technical committee of this project will meet annually. In addition to addressing routine business matters, the general purpose and motivating exercise will be to critique the adequacy and effectiveness of established research procedures, to discuss and debate the meaning and interpretation of data, suggest avenues to follow for best objective achievement, and to discuss the regional implication that the research will have. This process is viewed as critical and contributory to the regional research concept.
The officers shall consist of a chairperson, vice chairperson, and secretary. The vice chairperson and secretary will be elected annually, and the vice chairperson shall become chairperson, in consultation with the administrative advisor. The chairperson presides over the Technical Committee and Executive Committee and is responsible for preparing or supervising the preparation of the annual report of the regional project. The Chairperson is also responsible for site selection and coordination of the annual meeting and other meetings held at regional and national discipline meetings. The secretary records and distributes the minutes and performs other duties assigned by the Technical Committee.
Subcommittees will also be organized around each objective. A sub-committee chairperson will be appointed by the Technical Committee chair and will provide oversight responsibilities for activities related to the designated objective. The sub-committee chair will also coordinate meetings outside of the annual meeting, if necessary.
Literature Cited
BADECK FW, BONDEAU A, BOTTCHER K et al. (2004) Responses of spring phenology to climate change. New Phytologist 162, 295-309.
BRADFORD K (2002) Applications of hydrothermal time to quantifying and modeling seed germination and dormancy. Weed Science 50, 248-260.
BURNHAM KP & ANDERSON DR (2002) Model selection and inference: a practical information-theoretic approach, 2nd edn. Springer Verlag, New York. 32-74.
CARDINA J, HERMS CP, HERMS DA & FORCELLA F (2007) Evaluating phenological indicators for predicting giant foxtail (Setaria faberi) emergence. Weed Science 55, 455-464.
CLELAND EE, CHUINE I, MENZEL A, MOONEY HA & SCHWARTZ MD (2007) Shifting plant phenology in response to global change. Trends in Ecology & Evolution 22, 357-365.
CLEMENTS DR, DITOMMASO A, JORDAN N et al. (2004) Adaptability of plants invading north American cropland. Agriculture Ecosystems & Environment 104, 379-398.
DAVIS AS, CARDINA J, FORCELLA F et al. (2005) Environmental factors affecting seed persistence of 13 annual weeds across the U.S. corn belt Weed Science 53, 860-868.
FORCELLA F, BENECH-ARNOLD RL, SANCHEZ R & GHERSA CM (2000) Modeling seedling emergence. Field Crops Research 67, 123-139.
FORCELLA F, WILSON RG, DEKKER J et al. (1997) Weed seed bank emergence across the corn belt. Weed Science 45, 67-76.
FORCELLA F, WILSON RG, RENNER KA et al. (1992) Weed seedbanks of the U.S. cornbelt: magnitude, variation, emergence, and application. Weed Science 40, 636-644.
GRUNDY AC, PETERS NCB, RASMUSSEN IA et al. (2003) Emergence of Chenopodium album and Stellaria media of different origins under different climatic conditions. Weed Research 43, 163-176.
HICKIN RP & VITTUM MT (1976) The importance of soil and air temperature in spring phenoclimatic modeling. International Journal of Biometeorology 20, 200-206.
HOLM L, DOLL J, HOLM E, PANCHO J & HERBERGER J (1997) World weeds: natural histories and distribution. John Wiley and Sons, Inc., New York, NY. 1129.
HOPKINS AD (1919) Periodical events and natural law as guides to agricultural research and practice., 5-41. U.S. Bureau of Entomology, Washington, D.C.
HOPP RJ (1976) Modeling lilac development. International Journal of Biometeorology 20, 256-260.
INTERGOVERNMENTAL PANEL ON CLIMATE CHANGE (2007) Climate change 2007: Synthesis report. http://www.ipcc.ch/pdf/assessment-report/ar4/syr/ar4_syr.pdf, (Last accessed on Dec. 2, 2009.)
JACKSON MT (1966) Effects of Microclimate on Spring Flowering Phenology. Ecology 47, 407-415.
LINDQUIST JL, MORTENSEN DA, CLAY SA et al. (1996) Stability of corn (Zea mays)-velvetleaf (Abutilon theophrasti) interference relationships. Weed Science 44, 309-313.
MENZEL A (2000) Trends in phenological phases in Europe between 1951 and 1996. International Journal of Biometeorology 44, 76-81.
PARMESAN C & YOHE G (2003) A globally coherent fingerprint of climate change impacts across natural systems. Nature 421, 37-42.
RATHCKE B & LACEY EP (1985) Phenological Patterns of Terrestrial Plants. Annual Review of Ecology and Systematics 16, 179-214.
ROWSE HR & FINCH-SAVAGE WE (2003) Hydrothermal threshold models can describe the germination response of carrot (Daucus carota) and onion (Allium cepa) seed populations across both sub- and supra-optimal temperatures. New Phytologist 158, 101-108.
SCHUTTE BJ, REGNIER EE, HARRISON SK et al. (2008) A hydrothermal seedling emergence model for giant ragweed (Ambrosia trifida). Weed Science 56, 555-560.
SCHWARTZ MD & REITER BD (2000) Changes in North American spring. International Journal of Climatology 20, 929-932.
SPARKS TH & CAREY PD (1995) The Responses of Species to Climate over 2 Centuries - an Analysis of the Marsham Phenological Record, 1736-1947. Journal of Ecology 83, 321-329.
TRANEL PJ, JIANG WL, PATZOLDT WL & WRIGHT TR (2004) Intraspecific variability of the acetolactate synthase gene. Weed Science 52, 236-241.
WANG RL, WENDEL JF & DEKKER JH (1995) Weedy adaptation of Setaria spp. I. Isozyme analysis of genetic diversity and population genetic structure in Setaria viridis. American Journal of Botany 82, 308-317.