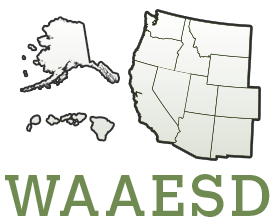
W1009: Integrated Systems Research and Development in Automation and Sensors for Sustainability of Specialty Crops
(Multistate Research Project)
Status: Inactive/Terminating
W1009: Integrated Systems Research and Development in Automation and Sensors for Sustainability of Specialty Crops
Duration: 10/01/2008 to 09/30/2013
Administrative Advisor(s):
NIFA Reps:
Non-Technical Summary
Statement of Issues and Justification
The Need: The continuing trend of declining available labor, combined with an increasing consumer desire for a safe and high quality food supply, the pressure of global competition, and the need to minimize the environmental footprint, represents challenges for specialty crop sustainability in the US. Producers and processors are urgently seeking new devices and systems which will aid them during production, harvesting, sorting, storing, processing, packaging, marketing, and transportation while also minimizing input costs.
Currently, there is a lack of effective and efficient sensors and automation systems for specialty crops (fruits, vegetables, tree nuts, dried fruits and nursery). This is because many of the underlying biological processes related to quality and condition of fruits and vegetables are difficult to translate into engineering concepts. Biological variability, coupled with the variable environmental factors, makes it difficult to develop sensors and automation systems for effective implementation at various stages of the production, harvest and postharvest handling chain. Additionally, obtaining measurement of biological factors internal to the commodity is difficult using external, nondestructive sensors, as such devices or processes used must adapt to a wide variation in shape, size, and maturity of the commodity being processed. It is a challenge for any single specialty crop sector to afford the cost of research, development, and commercialization of this complex level of automation. It is thus important for public agency entities to assist this economically vital agricultural sector with sensor and automation research and development. A CRIS search conducted in Fall 2007 failed to identify and USDA project focused on the development of automated equipment for specialty crop operations.
Importance of the Work: Previous incarnations (NE179 and NE1008) of this project have made considerable contributions in the development of sensors and the quantification of material properties to the fruit and vegetable industries. However, the steady increase in global competition and the recent decrease in available labor has increased the need for new technologies. A system-wide approach to developing automation for the specialty crop industry is critically needed to address economic and environmental sustainability challenges.
Related, Current and Previous Work
In the 1800's, US agriculture was powered by human and animal labor. With the introduction of the steam engine and internal combustion engine, mechanical devices rapidly became the power source for agricultural operations, and by the early 1900's there was a strong economic case for mechanical power (Ahndschin et al., 1921, Long 1931, Reynoldson 1933). The need for mechanization of agricultural crops (primarily row crops) intensified after World War II ( Cooper et al., 1947, Barlow and Fenske 1947, Lanham 1947). By the later half of the 20 century, grain and fiber agriculture employed very few farm laborers (National Rural Center 1980).
In contrast, specialty crops (fruits, nuts, vegetables, berries, nursery, dried fruits and herbs) continued to rely on human labor for most operations. Production operations were diverse, difficult, and required judgment - attributes which were well suited for human labor and poorly suited for mechanization (Grise and Johnson 1973). There were a few examples of successful mechanization in production systems such as tree shakers (Pellerin et al., 1978), fruit conveyers (Rehkugler et al., 1976, Schulte et al., 1989) and orchard sprayers (Burton et al., 1989, Byers 1989, Giles et al., 1987). But most of the automation effort was in postharvest handling and processing. There were many research and development efforts in quality inspection of fruits such as apples (Brown et al., 1989, Brown et al., 1993, Cavalieri et al., 1998, Guyer et al., 1991), berries (Boyette 1996, Okamura et al., 1993, Timm et al., 1998) and other fruits and vegetables (leafy greens - Boyette et al., 1992, fruit - Crowe and Delwiche 1996, fruit handling Deck and Strikeleather 1994, peaches - Miller and Delwiche 1989, cherries - Thompson et al., 1997 citrus - Miller and Drouillard 2001, fruit juices - Singh et al., 1996, prunes - Tan et al., 1990 and flowers Gautz and Wong 1993).
One of the most promising technologies was the mechanical harvest of processing tomatoes (Sullivan et al., 1974). This effort was a combination of breeding, management practices and mechanization to produce an integrated system for tomato harvest. Development of the tomato harvester and other specialty crop production equipment halted once the effect of mechanization on migrant labor was examined by society (De Janvry et al., 1980). The plight of displaced farm labor was examined for many crops (Peterson and Kislev 1981, Krause 1982, Price 1983, Fridley and Holtman, 1974), and even became a topic of popular culture (Baez 1984).
US automation and mechanization research for specialty crop production all but ceased in the late 1980's and early 1990's. Research and development continued in Europe and Japan, outpacing the US in production operations such as pruning, harvesting and handling specialty crops. In the late 1990's and continuing to the present, the farm labor supply for specialty crops has continued to shrink, and there emerged an urgent and on-going need for automation to replace human labor.
Understanding biology: Postharvest needs to measure quality and inspect for damage have directed the physiological and metabolic study of specialty crops. The parameters of firmness, color and bruise damage are reoccurring themes in reported work.
Firmness is associated with mechanical properties, and many researchers looked for connections between mechanical properties and sensory firmness. Puncture tests as used by Timm and Guyer 1998, are the most common means to measure firmness, but are destructive. Nondestructive assessment of firmness is highly desirable, and multiple approaches have been studied to measure the mechanical properties in a nondestructive manner, such as impact loading (Finney et al., 1978, Reyes et al., 1996, Lu and Abbott 1997) and vibration modal response (Wu et al., 1994, Lu and Abbott 1996). A few indirect measures of firmness have been determined; near infrared reflectance (Lu et al., 2000) and viscoelastic behavior (Gautz and Bhambare 1990).
Bruising is a common defect in fruits, and understanding the type and severity of mechanical loads which would cause bruising is important to the design of fruit and vegetable handling systems. Schulte et al. (1994) measured threshold levels of peach impact damage. Slaughter et al. (1993) quantified vibration injury to pears. Bajema et al. (2000) measured impact waves and resulting stresses in apple tissue. Upchurch et al. (1990) developed a method to measure bruise volume in situ using spectrophotometers.
One of the most important consumer quality attributes in fruits and vegetables is color. Color is also a maturity and harvest indicator. Delwiche et al. (1987) quantified color and maturity in peaches. Timm et al. (1993) made a similar harvest tool for tart cherries. Thai et al. (1990) monitor changes to tomatoes in storage.
Many other biological attributes have been studied. Delwiche and Baumgardner (1986) and Pitts et al. (1987) quantified the size of peaches and potatoes, respectively. Watercore (a potential quality factor in apples) was studied by Hung et al. (1989) and Tollner et al. (1992). Ikediala et al. (2000) investigated dielectric properties of apple and codling moth larvae to develop a method to eradicate the insect from apples. Sapers et al. (1977) measured to volatiles from a MacIntosh apple to estimate maturity. Thai and Shewfelt (1990) monitored changes in peach quality during storage; Anderson and Abbott (1975) monitored apple quality in various storage environments. Allison et al. (1987) measured internal pressures in maturing pecans. Pearson et al. (1996) studied hull adhesion forces in pistachio nuts.
Sensors and sensing systems: In postharvest operations, a rapid, accurate and nondestructive sensor is most desirable. Watada et al. (1976) and Delwiche et al. (1987) tested devices to measure peach firmness. Davis and Pitts (1985) and Timm et al. (1996) developed portable devices to measure cherry firmness. Multiple investigators developed more universal fruit firmness sensors (Delwiche et al., 1987, Delwiche et al., 1996, Ozer et al., 1998, Hung et al., 1999).
Bruise detection is an important quality attribute for fruit and vegetables in terms of consumer acceptance and in storing the produce. Upchurch et al. (1987) developed a detection device based on ultrasound reflection. Hyde et al. (1990) used a combination of an instrumented sphere and camera to identify handling systems components which were likely to bruise fruit. Rehkugler et al. (1971) and Upchurch (1991) used optical devices to locate bruises on fruit.
Color, both visible and infrared, has been the basis for numerous fruit and vegetable quality sensors. Delwiche (1987) used color to estimate peach maturity. Kleynen et al. (2003) used selected frequencies of light to sort Jonagold apples. Infrared light has been used to measure firmness and sugar content (Lu, 2001), internal quality in peaches, nectarines and kiwifruit (Slaughter 1995 and Slaughter and Crisosta, 1998), and vegetable quality (Xie et al., 2007).
Image analysis has formed the basis of many sensor designs. Machine vision system have been used to detect defects in prunes (Delwiche et al., 1990), peaches (Miller and Delwiche, 1991), and pistachio nuts (Pearson and Slaughter, 1996). Vision systems have also been used to grade various fruits and vegetables; potatoes (Tao et al., 1995), apples (Rehkugler and Throop, 1986, Heinemann et al., 1994), roses ( Steinmetz et al., 1994), mushrooms (Heinemann et al., 1994) and raisins (Okamura et al., 1993). Vision systems coupled with light energy transmitted through fruit has been used to detect watercore (Throop et al., 1994), and internal quality of mango fruit (Reyes et al., 2000).
Machine vision systems will be an integral part of automated equipment, and some progress has been made in vision for robotic systems. Slaughter and Harrell (1987) defined a color vision system for harvesting fruit. Cho et al. (2000) designed a real time tracking system in three dimensional space. Heinemann and Morrow (1986) developed a frost detector for use in tree fruit.
Some researchers have used novel methods to measure fruit quality. Cho and Krutz (1989) used NMR techniques as did Ray et al. (1993). Pitts and Cavalieri (1988) used images of iodine stained starch locations in apples as a measure of maturity. Zhao et al. (1993) used the apparent density of air-water mixtures to identity apples with watercore. Timm et al. (1991) evaluate various technologies to detect pits in tart cherries. Marrazzo et al. (2007) adapted an electronic nose design to differentiate between apple cultivars. Li et al. (2007) used sensor data fusion techniques to distinguish between damaged and healthy apples utilizing an electronic nose and a "zNose".
Developing nondestructive quality evaluation systems which operate at speeds comparable with orchard and/or packinghouse operations is a critical need. Design automation systems: There is a great deal of domestic and international interest in the design concepts related to robotic design in specialty crops. Krutz (1984) described a vision for robotics at the Conference on Robotics and Intelligent Machines in Agriculture. Cheng et al. (1999) described a distributed network to mange the automation. Kondo and Ting (1998) and Tillett (2003) described different aspect of automation on the farmstead.
Greenhouses are a more controlled environment for automation. Belforte et al. (2006) outline design concepts for greenhouse automation. Rodriguez et al. (1996) described a computer controlled automation system using a hertzian link.
Planting and transplanting operations has been studied by a number of researchers. Kutz et al. (1987) described a method for transplanting bedding plants. Gautz and Wong (1993) described a system to open flower pollen vessels during micropropagation. Sakaute (1996) developed an automated transplanter in Japan. In nursery operations, reducing labor used for transplanting is a critical need.
There is a great interest in automating harvest operations. Harvesting is one of the most labor intensive operations and has a high timeliness cost associated with delay (Oliveira et al., 1993). Sarig (1993) provided a state-of-review in the mid 1990's. Arima et al. (1996) developed a cucumber harvester in Japan. Kondo et al. (1996) developed a guided robotic system for cherry tomato harvesting. Pilarski et al. (2002) described a harvesting system using the demeter system. Robinson et al. (1990) discussed the potential benefits of pruning trees to ease automated harvest operations. Kondo et al. (1996) described a vision system for cutting chrysanthemums. Wang et al. (1997) developed a vision-guided robotic system for separating and transplanting sugar cane shoots from tissue culture.
Automated systems in the orchard require mobility. Torii (2000) described the development of autonomous vehicles in Japan; Stentz et al. (2002) described a semi autonomous vehicle developed in the US. Guidance is a critical part of vehicle mobility (Reid at al., 2000). A number of researchers have investigated ways to map the route of an autonomous vehicle using GPS (Bell et al., 1998, Freeland et al., 2002) and remote mapping (Tao and Li, 2007). Other systems rely on machine vision to move in the orchard (Slaughter et al., 1999, Pinto et al., 2000). Developing a commercially viable, and widely accepted mobile platform as a base for specific crop operations is a critical need.
Grasping, or otherwise interacting with the crop or branches is essential to many production operations. Sivaraman and Burks (2006) developed performance indices for manipulators. Cho et al. (2002) adapted three degree of freedom robotic arms for harvesting lettuce. Edan et al. (1992) developed a finite element model of a gripper. There have been many designs for grippers of fruit in general (Kondo et al., 1992), melons (Cardenas-Weber et al., 1991), tomatoes (Kondo et al., 1995), and in the vineyard (Monta et al., 1995), but there is not wide acceptance of any particular design. A common gripper design would greatly advance the development of harvesting equipment.
Objectives
-
Adapt biological concepts associated with specialty crop production, harvest, and postharvest handling into quantifiable parameters which can be sensed
-
Develop sensors and sensing systems which can measure and interpret the parameters
-
Design and evaluate automation systems which incorporate varying degrees of mechanization and sensors to assist specialty crop industries with labor, management decisions, and reduction of production costs
-
Work in partnership with equipment and technology manufacturers to commercialize and implement the outcomes of this project
Methods
Multiple Crop and Cross Platform Integration: A key and continuing theme in this project is a two dimensional integration of research activities; integration from the adaptation of biological concepts to measurable parameters through the commercialization of new products; and integration within each objective among different specialty crops. Although the objectives seem sequential in execution, we anticipate a swirl of concurrent activities centered about and driven by the needs of specialty crop growers for automation of growing, harvesting and postharvest operations. A major task for the project leadership is to facilitate communication and collaboration among the members of this project, and between project members and other stakeholders in specialty crop agriculture.Objective 1: Adapt biological concepts into parameters which can be sensed.
{CA (Delwiche, Slaughter), CO (Khosla), GA (Li), HI (Gautz), IA (Steward), MI (Lu, Guyer), NY (Hang), AL (Chin), PA (Heinemann, Baugher), TX (Sui, Thomasson), WA (Pierce, Pitts, Sablani, Tang, Lewis)}
Every automation system for specialty crops will depend on sensing both the immediate environment and the "biological state" (the combination of genetics, growth history including pesticide pressures, and current environmental conditions which influence future growth and quality factors) of the plant and produce. Developing the knowledge relating the biological state to parameters which can be externally measured is central to sensor design.
Relating the biological state into to measurable parameters is a direct continuation of the research conducted under NE-179 and NE-1008, the predecessor of the proposed project, and there is a deep level of expertise among the proposed members of W_TEMP 2401 in the areas of physical properties, enzymatic reactions, internal quality and plant response to insect or disease attack and environmental stress.
Defining how external loads are transferred to cells, and under what conditions will those loads cause mechanical failure and/or injury response from the plant has been studied in almost every experiment station represented in this project. Continuing and future research will define the cellular mechanical properties, and how these cell-scale properties integrate to form tissue-scale mechanical properties which can be linked to quality parameters such as firmness and crispness.
Enzymes are the messengers and initiators of most biological activities in plants. Understanding the function of a particular enzyme, and the biochemical reactants of the enzymatic activity will provide significant insight into the biological state of the plant. These reactants are complex and present in low concentrations, but can be measured with third and fourth generation biosensors. Continuing research efforts will focus on enzyme-based biochemistry, the kinetics of these reactions, reaction products, and the mass transfer of these products to the exterior of the fruit or vegetable.
Internal quality factors, such as the amount soluble sugar, give clear indications of the maturity level of many fruits and vegetables. There has been considerable research conducted on the relationship between internal quality factors and consumer acceptance of various produce using optical, NMR, X-ray, infrared and other electromagnetic properties, but much work remains in using the concentration and location of internal quality factors, measured using nonintrusive means, as indicators of fruit and vegetable maturity.
Optical and electrical properties are useful for assessing internal quality factors, such as the amount of soluble sugar, and the maturity level of many fruits and vegetables. There has been considerable research conducted on the relationship between internal quality factors and consumer acceptance of various produce using optical, NMR, X-ray, infrared and other electromagnetic properties, but much work remains in development of rapid, cost effective, and more accurate methods and techniques for quantification of the electromagnetic properties and their relationship with internal quality parameters of fruits and vegetables.
Plant response to insect/disease attack and environmental stress is often the first and best indicator that intervention is required.
The biological concepts described above do not act in isolation to each other. Causal relationships such as enzyme activity and cell mechanical properties exists. Abnormal "biological states", such as water deficient stress, often cause multiple responses among the concepts described above. Future research is needed to look at using combinations of these concepts to gain more precise and insightful understanding of the "biological state".
Objective 2: Develop sensors and sensing systems
{CA (Delwiche, Slaughter), FL (Ehsani, Lee), GA (Li, Daley), HI (Gautz), IA (Darr, Steward, Tang, Yu), MA (Tao), MI (Lu, Alocilja, Guyer), OK (Weckler, Wang), AL (Chin), PA (Heinemann), TX (Sui, Thomasson), WA (Pierce, Pitts, Tang), WV (Tabb)}
Sensors gather the information needed by automation systems. There is a long and rich history of sensor research and development aiming toward sensing the "biological state". Most of the sensors developed to date are used in the comparatively controlled environment of the packing / sorting shed. Automated operations on the growing crop, as well as harvest, handling, and transport processes will require a class of sensors rugged and adaptable enough for field and packing house use. The multitude of sensor designs can be roughly classified by three human senses; touch, sight and smell.
Many of the interactions between the crop and machinery (such as pruning, thinning, harvesting, sorting, storage, and transportation) will involve physical contact. Most of these interactions must be nondestructive to the plant. Knowing the maximum forces which may be applied without damage will require sensing the mechanical properties of the plant. Research and design is required to develop sensors which can nondestructively, rapidly and accurately determine the inherent strength of a plant tissue.
Image and vision-based sensors will provide the bulk of the information to an automation system. Vision systems (including cameras, NMR devices, IR devices and other devices based on radiated energy) can easily focus on a variety of targets, work non-invasively, provide information in the visible and non-visible spectrums, and provide a large amount of data. The challenge in using vision systems is to extract the required information from the collected data. For some imaging devices such NMR and X-ray, moving to a cost effective, portable system in an outdoor environment will be a challenge in itself. New concepts such as stereoscopic images, coupled with an ever increasing amount of portable computing power can provide new techniques to extract the information, but there is a significant challenge in designing effective vision-based sensing systems at an acceptable cost.
Biosensors, which can selectively detect small concentrations of airborne organics, have the potential to provide information concerning on-going biological processes in the plant. There are significant design issues regarding informed placement of the sensors, building sensors rugged enough for outdoor use, and stable enough for use during an automated operation. The research task is to bring the biosensor outdoors, and make it an effective sensor in an automated system.
Much of the automated equipment in the field, and some automated equipment operating in a packing / sorting shed environment, will move and perform its operations in an autonomous manner. The equipment will require a sensing system tasked to safe and efficient movement. Many commercial sensors (such as vision, GPS, and radio transmitter based devices) exist which can fill this need, but there remains a research challenge to find the most cost effective sensor system which will serve the locomotion needs of the autonomous equipment.
Objective 3: Design and evaluate automation systems
{CA (Slaughter), CO (Khosla), FL (Ehsani, Lee), GA (Li, Daley), HI (Gautz), IA (Darr, Steward, Tang), MA (Tao), MI (Guyer), NY (Hang), OK (Weckler, Wang), OR (Seavert), AZ (Villalobos), AL (Chin), PA (Heinemann), TX (Sui, Thomasson), WA (Pierce, Pitts, Tang, Lewis), WV (Tabb)}
A great deal of knowledge and equipment from the industrial and military use of automated equipment can be applied to specialty crops, but the wide variation and semi-chaotic nature of field operations provide a significant number of research challenges. A key challenge is adaptability to multiple crops and cropping systems. The capital investment of automated equipment is high, and the potential market is small. It is critical to spread research and development costs by developing automated systems which can easily adapt to different crops and different cropping systems.
An automated system for specialty crops is much more than an autonomous robot moving along the a row of tress or plants. A cost effective system will require information from many sources both on-site and off site, and autonomous robots or vehicles will require direction and coordination. Hence the efficient gathering of data and control of devices will be an integral part of an automated system.
It is not feasible to replace human labor with automation in all operations. In some operations the cost of replacing human dexterity and complex decision-making capability with equipment is not justified. In these operations, a semi-automated device assisting human labor may be a more optimum solution. Developing a man-machine system requires careful attention to the ergonomic (safety, productivity, comfort and intellectual engagement) needs of the human in the system. Very little research has been done in ergonomic design of specialty crop equipment, and additional research is required to design an optimum man-machine system for specialty crops.
Decisions regarding the overall plan and control of an automated system will remain with a human operator. The quantity of operator decision making information needed for autonomous or semi-autonomous operation of various equipment may potentially be unprecedented and unmanagable. There is a need to develop computer-based data systems which collect, verify, and organize raw data to present information to the operator such as the maturity of the crop, crop stress, and spatial variation. There is also a need to organize predictive models for crop needs such as pesticide application, pruning, thinning and harvesting. Finally, there is also a need to help the operator visualize the most effective use of the automated equipment.
Objective 4: Partner with equipment and technology manufacturers in commercializing
{CA (Slaughter), FL (Lee, Li), HI (Gautz), IA (Darr, Steward, Tang), MA (Tao), MI (Guyer), OK (Weckler), OR (Seavert), AL (Chin), GA (Daley), PA (Heinemann), TX (Sui, Thomasson), WA (Pierce, Pitts, Sablani, Tang, Lewis)}
The selection of a particular crop and operation covered by this project will be based on growers' needs for automation of that crop and operation. With that as a premise, we cannot consider the research complete until a piece of equipment or device is commercialized and in use. Immediately following acceptance of this project, we will initiate meetings between project participants, specialty crop growers and manufacturers to target specific crops and operations for which we will develop automation.
Commercialization of many critical automation needs of specialty crop growers will be difficult to justify using traditional business plans because the small size of the market, coupled with potentially high development costs, will result in a low return on investment (ROI). Manufacturers will need help to lessen the cost of development and risk assumed to marketing a new product. Frequent communication between growers, manufacturers and project participants will help to mitigate cost and risk to the manufacturers. We will create opportunities for partnerships between specialty crop growers, manufacturers and project participants.
Defining a set of industry standards for the test, operation and components of automated equipment is a necessity. Standards will enable the interchangeability of parts and software, decrease design time, and encourage manufacturers to build component parts such as articulating arms and end effectors. We will work with the standards committees of ASABE and SAE to propose standards related to automation in specialty crops.
The design of automation systems is a continually evolving engineering area, and the application of automation design to specialty crops is very new. Engineering concepts and techniques learned during this project should be shared among practicing engineers and engineering students. During the project we will periodically collect concepts and techniques learned from among the participants, and disseminate this knowledge through classroom and continuing education venues.
Measurement of Progress and Results
Outputs
- Engineering models relating mechanical and/or physical properties, enzymatic reactions, internal quality and plant external stress indicators to the "biological state" of selected specialty crops
- Engineering models which estimate "biological state" based on the interaction of multiple indicators
- Sensors capable of measuring the "biological state" adapted for outdoor use on automated equipment
- Sensors used in industrial and military automation adapted for use in specialty crop environments
- Sensors capable of measuring and monitoring product quality and food safety during harvest and postharvest operations.
- Specialty crop automated and semi-automated equipment available
- Wide-area specialty crop data communication systems available
- Decision-making software for use in aiding the management of automated equipment
- Integrated set of design, test and manufacturing standards
- Design, manufacturing and usage education modules for use in university and continuing education learning.
Outcomes or Projected Impacts
- Specialty crop technology development
- Research publications in the design of specialty crop equipment
- Training of graduate and undergraduate engineering students in the design of specialty crop automated equipment
- Workshops and other continuing education opportunities for practicing engineers
- Competitive advantage for domestic specialty crop producers by increasing labor efficiency with automated equipment and systems
- Healthier and safer working environment for the remaining human workforce used in specialty crop production and handling
- Manufacturing workforce (design engineers, mechanics, operators) better prepared to manufacture and use automated equipment for specialty crop production and handling
- Reduction of the impact of specialty crop production on the environment through more precise field and packinghouse operations
Milestones
(2009): Spring 2009: Meetings between researchers, growers and manufactures have identified targeted specialty crops and related operations. Summer 2009: Research, design and manufacturing responsibilities for the targeted crops / operations assigned. Fall 2009: Target crops / operations requiring longer development time (not likely to be completed within the 5 year duration of this project) identified(2010): Spring 2010: Obtaining industrial and federal grants to support team research
(2011): Spring 2011: Developing prototypes and conducting field research
(2012): Spring 2012: Filing patent application for developed technologies
(2013): Spring 2013: Organizing workshops and helping industry to commercialize and apply the technologies. Fall 2013: Project ends (renewal in place)
Projected Participation
View Appendix E: ParticipationOutreach Plan
From the user-based design nature of this project, outreach to our partners is continuous and integral to the project. The intended users will be a part of the initial meetings to select targeted crops / operations, in the prototype design and testing of equipment, in the development of business plan and marketing tools, and in the commercialization of the target equipment. Indicators of the project outreach will include the expected scientific papers, patents and publications in the trade and popular press. In addition, there will be frequent communication with manufactures, growers and the people who will be using the automated equipment, sets of standards to convey the design concepts learned to a wide audience of engineers and technicians, and educational modules which can be used in classroom and continuing education venues.
Organization/Governance
The technical committee will consist of project leaders for the contributing states, the administrative advisor, and CSREES representatives. Voting membership includes all persons with contributing projects.
A Chairperson, Vice Chairperson and Secretary will be elected from the voting membership at the first authorized committee meeting after the project has been approved. The Chair, Vice-Chair and Secretary will serve two years, if so desired by the membership. They will be responsible for meeting arrangements, annual reports, implementation of the Outreach Plan, and preparation of the renewal proposal.
Due to the number and diversity of the membership, and the user-based nature of this project, a working group and coordinator for each target crop / operation will be selected by the Chairperson from among the membership. The coordinator will be responsible for communication within the working group, developing a timeline for the targeted crop / operation, and coordinating activities among the four project objectives (as apply to the particular targeted crop / operation).
Literature Cited
ALLISON, J. M., JR. PRUSSIA, S. E. DANIELL, J. W. & TOLLNER, E. W. (1987). Measuring the internal pressure of maturing pecans. In Transactions of the ASAE - American Society of Agricultural Engineers pp. 1869-1872. ANDERSON, R. E. & ABBOTT, J. A. (1975). Apple Quality after Storage in Air, Delayed-Ca or Ca [Controlled Atmosopheres]. In Hortsci pp. 255-257. ARIMA, S. KONDO, N. & NAKAMURA, H. (1996). Development of robotic system for cucumber harvesting. In Jarq-Japan Agricultural Research Quarterly pp. 233-238. BAEZ, J. 1984 "Plane wreck at Los Gatos (Deportee)" lyrics by Woodie Guthrie, music by Martin Hoffman. Published by Vanguard Recording Society New York, New York BAJEMA, R. W. BARITELLE, A. L. HYDE, G. M. & PITTS, M. J. (2000). Factors influencing dynamic mechanical properties of Red 'Delicious' apple tissue. In Transactions of the ASAE pp. 1725-1731. BARLOW, F. D. & FENSKE, L. J. (1947). Cost and utilization of power and equipment on farms in the Mississippi River Delta Cotton Area of Louisiana. p. 44. Baton Rouge, La.: Louisiana State University and Agricultural and Mechanical College Agricultural Experiment Station. BELFORTE, G. DEBOLI, R. GAY, P. PICCAROLO, P. & AIMONINO, D. R. (2006). Robot design and testing for greenhouse applications. In Biosystems engineering pp. 309-321. BELL, T. O'CONNOR, M. JONES, V. K. REKOW, A. ELKAIM, G. & PARKINSON, B. (1998). Realistic autofarming closed-loop tractor control over irregular paths using kinematic GPS. In Journal of Navigation pp. 327-335. BOYETTE, M. D. (1996). Forced-air cooling packaged blueberries. In Applied engineering in agriculture pp. 213-217 BOYETTE, M. D. SANDERS, D. C. & ESTES, E. A. (1992a). Postharvest cooling and handling of cabbage and leafy greens. In AG - North Carolina Agricultural Extension Service, North Carolina State University p. 4 p. BROWN, G. K. SCHULTE, N. L. PETERSON, D. L. TIMM, E. J. BEAUDRY, R. M. HANCOCK, J. F. & TAKEDA, F. (1993). Estimates of mechanization effects on fresh blueberry quality. In Paper p. 13 p. BROWN, G. K. SCHULTE, N. L. TIMM, E. J. BURTON, C. L. & MARSHALL, D. E. (1989). Apple packing line impact damage reduction. In Paper - American Society of Agricultural Engineers p. 14 p. BURTON, C. L. BROWN, G. K. SCHULTE PASON, N. L. & TIMM, E. J. (1989). Apple bruising related to picking and hauling impacts. In Paper - American Society of Agricultural Engineers p. 19 BYERS, R. E. HOGMIRE, H. W. FERREE, D. C. HALL, F. R. & DONAHUE, S. J. (1989). Spray chemical deposits in high-density and trellis apple orchards. In HortScience pp. 918-920. CARDENAS-WEBER, M. STROSHINE, R. L. HAGHIGHI, K. & EDAN, Y. (1991). Melon material properties and finite element analysis of melon compression with application to robot gripping. In Transactions of the ASAE pp. 920-929. CAVALIERI, R. P. HYDE, G. M. & MACQUARRIE, P. R. (1998). Hydraulic sorting of watercore apples. In Acta horticulturae pp. 103-108. CHENG, F. T. SHEN, E. DENG, J. Y. & NGUYEN, K. (1999). Development of a system framework for the computer-integrated manufacturing execution system: a distributed object-oriented approach. In International Journal of Computer Integrated Manufacturing pp. 384-402. CHO, S. I. CHANG, S. J. KIM, Y. Y. & AN, K. J. (2002). Development of a three-degrees-of-freedom robot for harvesting lettuce using machine vision and fuzzy logic control. In Biosystems engineering pp. 143-149. CHO, S. I. & KRUTZ, G. W. (1989). Fruit ripeness detection by using 1H-NMR. In Paper - American Society of Agricultural Engineers p. 11 p. CHO, Y. J. YOU, B. J. LIM, J. H. & OH, S. R. (2000). Real-time visual tracking insensitive to three-dimensional rotation of objects. In Biologically Motivated Computer Vision, Proceeding pp. 160-167. COOPER, M. R. BARTON, G. T. & BRODELL, A. P. (1947). Progress of farm mechanization. p. 101. Washington, D.C.: U.S. Dept. of Agriculture. CROWE, T. G. & DELWICHE, M. J. (1996). Real-time defect detection in fruit. II. An algorithm and performance of a prototype system. In Transactions of the ASAE pp. 2309-2317. DAVIS, D. C. & PITTS, M. J. (1985). Measurement of cherry firmness. In Postharvest pomology newsletter pp. 10-12. DE JANVRY, A. LEVEEN, P. & RUNSTEN, D. (1980). Mechanization in California agriculture : the case of canning tomatoes. p. 220 leaves. Berkeley, Calif.: s.n. DECK, S. H. & STIKELEATHER, L. F. (1994). Evaluations of a semi-automated vegetable sorting concept. In Applied engineering in agriculture pp. 815-821. DELWICHE, M. J. (1987). Grader performance using a peach ground color maturity chart. In HortScience pp. 87-89. DELWICHE, M. J. AREVALO, H. & MEHLSCHAU, J. (1996). Second generation impact force response fruit firmness sorter. In Transactions of the ASAE pp. 1025-1033. DELWICHE, M. J. & BAUMGARDNER, R. A. (1986). Conversion between southeastern and California peach sizes. In HortScience pp. 1238-1239. DELWICHE, M. J. MCDONALD, T. & BOWERS, S. V. (1987). Determination of peach firmness by analysis of impact forces. In Transactions of the ASAE - American Society of Agricultural Engineers pp. 249-254. DELWICHE, M. J. TANG, S. & RUMSEY, J. W. (1987). Color and optical properties of Clingstone peaches related to maturity. In Transactions of the ASAE - American Society of Agricultural Engineers pp. 1873-1879. DELWICHE, M. J. TANG, S. & THOMPSON, J. F. (1990). Prune defect detection by line-scan imaging. In Transactions of the ASAE pp. 950-954. EDAN, Y. HAGHIGHI, K. STROSHINE, R. & CARDENAS-WEBER, M. (1992). Robot gripper analysis: finite element modeling and optimization. In Applied engineering in agriculture pp. 563-570. FINNEY, E. E. J. ABBOTT, J. A. WATADA, A. E. & MASSIE, D. R. (1978). Nondestructive Sonic Resonance and the Texture of Apples [Quality Evaluation]. In J Am Soc Hortic Sci pp. 158-162 FREELAND, R. S. YODER, R. E. AMMONS, J. T. & LEONARD, L. L. (2002). Integration of real-time global positioning with ground-penetrating radar surveys. In Applied engineering in agriculture pp. 647-650. FRIDLEY, R. B. & HOLTMAN, J. B. (1974). Predicting the Socio-Economic Implications of Mechanization by Systems Analysis. [Harvesting Strawberries]. In Trans ASAE Gen Ed (Am Soc Agric Eng) pp. 821-825. GAUTZ, L. D. & BHAMBARE, D. N. (1990). Relationship between viscoelastic properties and maturity of mangoes. In Paper - American Society of Agricultural Engineers p. 10 p. GAUTZ, L. D. & WONG, C. W. (1993). Device for opening and closing magenta vessels for micropropagation. In HortTechnology pp. 340-342 GILES, D. K. DELWICHE, M. J. & DODD, R. B. (1987). Control of orchard spraying based on electronic sensing of target characteristics. In Transactions of the ASAE - American Society of Agricultural Engineers pp. 1624-1630, 1636. GRISE, V. N. & JOHNSON, S. S. (1973). An economic analysis of cling peach production with emphasis on harvest mechanization. pp. vi, 38. Washington, D.C.: U.S. Dept. of Agriculture. GUYER, D. E. SCHULTE, N. L. TIMM, E. J. & BROWN, G. K. (1991). Minimizing apple bruising in the packingline. In Tree fruit postharvest journal pp. 14-20. HANDSCHIN, W. F. ANDREWS, J. B. & RAUCHENSTEIN, E. (1921). The horse and the tractor : an economic study of their use on farms in central Illinois. pp. [169]-223. Urbana, Ill.: University of Illinois Agricultural Experiment Station. HEINEMANN, P. H. HUGHES, R. MORROW, C. T. SOMMER, H. J., III BEELMAN, R. B. & WUEST, P. J. (1994). Grading of mushrooms using a machine vision system. Transactions of the ASAE 37(5), 1671-1677. HEINEMANN, P. H. & MORROW, C. T. (1986). An artificial apple bud for frost temperature sensing. Transactions of the ASAE - American Society of Agricultural Engineers 29(5), 1338-1341. HUNG, Y. C. PRUSSIA, S. E. & EZEIKE, G. O. I. (1999). Nondestructive firmness sensing using a laser air-puff detector. In Postharvest biology and technology pp. 15-25. HUNG, Y. C. TOLLNER, E. W. UPCHURCH, B. L. & PRUSSIA, S. E. (1989). Physical properties of apples affected by watercore disorder. In Paper - American Society of Agricultural Engineers p. 15 p. HYDE, G. M. ZHANG, W. & CAVALIERI, R. P. (1990). Bruise detection using the instrumented sphere and a high speed video camera. In Paper - American Society of Agricultural Engineers p. ill. IKEDIALA, J. N. TANG, J. DRAKE, S. R. & NEVEN, L. G. (2000). Dielectric properties of apple cultivars and codling moth larvae. In Transactions of the ASAE pp. 1175-1184. KLEYNEN, O. LEEMANS, V. & DESTAIN, M. F. (2003). Selection of the most efficient wavelength bands for 'Jonagold' apple sorting. In Postharvest biology and technology pp. 221-232. KONDO, N. FUJIURA, T. MONTA, M. SHIBANO, Y. MOHRI, K. & YAMADA, H. (1995). End-effectors for petty-tomato harvesting robot. In Acta horticulturae pp. 239-245. KONDO, N. MONTA, M. SHIBANO, Y. MOHRI, K. YAMASHITA, J. & FUJIURA, T. (1992). Agricultural robots(2): manipulators and fruits harvesting hands. In Paper p. 19 p. KONDO, N. NISHITSUJI, Y. LING, P. P. & TING, K. C. (1996). Visual feedback guided robotic cherry tomato harvesting. In Transactions of the ASAE pp. 2331-2338. KONDO, N. OGAWA, Y. MONTA, M. & SHIBANO, Y. (1996). Visual sensing algorithm for chrysanthemum cutting sticking robot system. In Acta horticulturae pp. 383-388. KONDO, N. & TING, K. C. (1998). Robotics for plant production. In Artificial Intelligence Review pp. 227-243. KRAUSE, K. R. (1982). Indirect farm labor and management costs. In Agricultural economic report - United States Dept of Agriculture p. 65 p. KRUTZ, G. W. (1984). Future use of robots in agriculture. In Robotics and intelligent machines in agriculture : proceedings, First International Conference on Robotics and Intelligent Machines in Agriculture, October 2-4 1983, Tampa, Florida pp. 15-29 ill. St. Joseph, Mich.: American Society of Agricultural Engineers. KUTZ, L. J. MILES, G. E. HAMMER, P. A. & KRUTZ, G. W. (1987). Robotic transplanting of bedding plants. In Transactions of the ASAE - American Society of Agricultural Engineers pp. 586-590. LANHAM, B. T. (1947). Farm power and equipment costs in northern Alabama. p. 31. Auburn, Ala.: Agricultural Experiment Station of the Alabama Polytechnic Institute. LI, C., AND P.H. HEINEMANN. (2007). ANN-integrated electronic nose and zNoseTM system for apple quality evaluation. Transactions of ASABE. 50(6): 2285-2294. LONG, L. E. (1931). Farm power in the Yazoo-Mississippi Delta. p. 30. A. & M. College, Miss.: Mississippi Agricultural Experiment Station. LU, R. (2001). Predicting firmness and sugar content of sweet cherries using near-infrared diffuse reflectance spectroscopy. In Transactions of the ASAE pp. 1265-1271. LU, R. & ABBOTT, J. A. (1996). Finite element analysis of models of vibration in apples. In Journal of texture studies pp. 265-286. LU, R. & ABBOTT, J. A. (1997). Finite element modeling of transient responses of apples to impulse excitation. In Transactions of the ASAE pp. 1395-1406. LU, R. GUYER, D. E. & BEAUDRY, R. M. (2000). Determination of firmness and sugar content of apples using near-infrared diffuse reflectance. In Journal of texture studies pp. 615-630. MARRAZZO, W. N. HEINEMANN, P. H. CRASSWELLER, R. E. & LEBLANC, E. 2007. Electronic nose chemical sensor feasibility study for the differentiation of apple cultivars. Transactions of the ASAE 48(5), 1995-2002. MILLER, B. K. & DELWICHE, M. J. (1989). Automatic grading of fresh peaches using color computer vision. In Acta horticulturae pp. 161-166. MILLER, B. K. & DELWICHE, M. J. (1991). Peach defect detection with machine vision. In Transactions of the ASAE pp. 2588-2597. MILLER, W. M. & DROUILLARD, G. P. (2001). Multiple feature analysis for machine vision grading of Florida citrus. In Applied engineering in agriculture pp. 627-633 MONTA, M. KONDO, N. SHIBANO, Y. & MOHRI, K. (1995). End-effectors for agricultural robot to work in vineyard. In Acta horticulturae pp. 247-254. NATIONAL RURAL CENTER. (1980). Production efficiency and technology for small farms. p. 160 in various pagings. Washington, D.C. (1828 L Street, NW, Washington, D.C. 20036): The National Rural Center. OKAMURA, N. K. DELWICHE, M. J. & THOMPSON, J. F. (1993). Raisin grading by machine vision. In Transactions of the ASAE pp. 485-492. OLIVEIRA, V. J. & UNITED STATES. DEPT. OF AGRICULTURE. ECONOMIC RESEARCH SERVICE. (1993). Hired farm labor use on fruit, vegetable, and horticultural specialty farms. In Agricultural Economic Report ; no 676 pp. iv, 28. Washington, D.C.: U.S. Dept. of Agriculture Economic Research Service. OZER, N. ENGEL, B. A. & SIMON, J. E. (1998). A multiple impact approach for non-destructive measurement of fruit firmness and maturity. In Transactions of the ASAE pp. 871-876. PEARSON, T. C. & SLAUGHTER, D. C. (1996). Machine vision detection of early split pistachio nuts. In Transactions of the ASAE pp. 1203-1207. PEARSON, T. C. SLAUGHTER, D. C. & STUDER, H. E. (1996). Hull adhesion characteristics of early-split and normal pistachio nuts. In Applied engineering in agriculture pp. 219-221. PELLERIN, R. A. MILLIER, W. F. LAKSO, A. N. REHKUGLER, G. E. THROOP, J. A. & ALLPORT, T. E. (1978). Apple Harvesting with an Inertial Vs. Impulse Trunk Shaker on Open-Center and Central-Leader Trees. I. In Trans Asae (Am Soc Agric Eng) pp. 407-413 PETERSON, W. L. & KISLEV, Y. (1981). The cotton harvester in retrospect : labor displacement or replacement? p. 15 leaves. St. Paul, Minn.: Dept. of Agricultural and Applied Economics University of Minnesota Institute of Agriculture Forestry and Home Economics. PILARSKI, T. HAPPOLD, M. PANGELS, H. OLLIS, M. FITZPATRICK, K. & STENTZ, A. (2002). The demeter system for automated harvesting (Reprinted from Proceedings of the American Nuclear Society: 8th International Topical Meeting on Robotics Remote Systems, Pittsburgh, PA, April 25-29, 1999.). In Autonomous Robots pp. 9-20. PINTO, F. A. C. REID, J. F. ZHANG, Q. & NOGUCHI, N. (2000). Vehicle guidance parameter determination from crop row images using principal component analysis. In Journal of agricultural engineering research pp. 257-264. PITTS, M. J. & CAVALIERI, R. P. (1988). Objective assessment of apple maturity based on starch location. In Transactions of the ASAE pp. 962-966. PITTS, M. J. HYDE, G. M. & CAVALIERI, R. P. (1987). Modeling potato tuber mass with tuber dimensions. In Transactions of the ASAE - American Society of Agricultural Engineers pp. 1154-1159. PRICE, B. L. (1983). The political economy of mechanization in U.S. agriculture. pp. xiv, 108. Boulder, Colo.: Westview Press. RAY, J. A. STROSHINE, R. L. KRUTZ, G. W. & WAI, W. K. (1993). Quality sorting of sweet cherries using magnetic resonance. In Paper p. 11 p. REHKUGLER, G. E. MILLIER, W. F. PELLERIN, R. A. & THROOP, J. A. (1976). Analysis of a Reciprocating Panel Fruit Lowering Device. In Trans Asae (Am Soc Agric Eng) pp. 30-34. REHKUGLER, G. E. STIEFVATER, T. L. & THROOP, J. A. (1971). An Optical Bruise Detection Technique. [Mechanical Harvest,Apples]. In Amer Soc Agr Eng Trans Asae pp. 1189-1194. REHKUGLER, G. E. & THROOP, J. A. (1986). Apple sorting with machine vision. In Transactions of the ASAE - American Society of Agricultural Engineers pp. 1388-1397. REID, J. F. ZHANG, Q. NOGUCHI, N. & DICKSON, M. (2000). Agricultural automatic guidance research in North America. In Computers and electronics in agriculture pp. 155-167. REYES, M. U. PAULL, R. E. ARMSTRONG, J. W. FOLLETT, P. A. & GAUTZ, L. D. (2000). Non-destructive inspection of mango fruit (Mangifera indica L.) with soft X-ray imaging. In Acta horticulturae pp. 787-792. REYES, M. U. PAULL, R. E. WILLIAMSON, M. R. & GAUTZ, L. D. (1996). Ripeness determination of "Solo" papaya (Carica papaya L.) by impact force. In Applied engineering in agriculture pp. 703-708. REYNOLDSON, L. A. (1933). Utilization and cost of power on corn belt farms. p. 60. Washington: U.S. Dept. of Agriculture. ROBINSON, T. L. MILLIER, W. F. THROOP, J. A. CARPENTER, S. G. & LAKSO, A. N. (1990). Mechanical harvestability of Y-shaped and pyramid-shaped 'Empire' and 'Delicious' apple trees. In Journal of the American Society for Horticultural Science pp. 368-374. RODRIGUEZ, M. AYALA, A. HERRERA, F. & PRIANO, F. H. (1996). Greenhouse automation through computer and hertzian link. In International Journal of Electrical Engineering Education pp. 66-88. SAKAUE, O. (1996). Development of seedling production robot and automated transplanter system. In Jarq-Japan Agricultural Research Quarterly pp. 221-226. SAPERS, G. M. ABBOTT, J. MASSIE, D. WATADA, A. & FINNEY, E. E. J. (1977). Volatile Composition of Mcintosh Apple Juice as a Function of Maturity and Ripeness Indices [Abstract Only]. In Food Technol p. 122. SARIG, Y. (1993). Robotics of fruit harvesting: a state-of-the-art review. In Journal of agricultural engineering research pp. 265-280. SINGH, P. C. BHAMIDIPATI, S. SINGH, R. K. SMITH, R. S. & NELSON, P. E. (1996). Evaluation of in-line sensors for prediction of soluble and total solids/moisture in continuous processing of fruit juices. In Food Control pp. 141-148. SCHULTE, N. L. TIMM, E. J. & BROWN, G. K. (1994). 'Redhaven' peach impact damage thresholds. In HortScience : a publication of the American Society for Horticultural Science pp. 1052-1055. SCHULTE, N. L. TIMM, E. J. BROWN, G. K. MARSHALL, D. E. & BURTON, C. L. (1989). Apple damage assessment during intrastate transportation. In Paper - American Society of Agricultural Engineers p. 18 p SIVARAMAN, B. & BURKS, T. F. (2006). Geometric performance indices for analysis and synthesis of manipulators for robotic harvesting. In Transactions of the ASABE pp. 1589-1597. SLAUGHTER, D. C. (1995). Nondestructive determination of internal quality in peaches and nectarines. In Transactions of the ASAE pp. 617-623. SLAUGHTER, D. C. CHEN, P. & CURLEY, R. G. (1999). Vision guided precision cultivation. In Precision agriculture pp. 199-216. SLAUGHTER, D. C. & CRISOSTO, C. H. (1998). Nondestructive internal quality assessment of kiwifruit using near-infrared spectroscopy. In Seminars in food analysis pp. 131-140. SLAUGHTER, D. C. & HARRELL, R. C. (1987). Color vision in robotic fruit harvesting. In Transactions of the ASAE - American Society of Agricultural Engineers pp. 1144-1148. SLAUGHTER, D. C. HINSCH, R. T. & THOMPSON, J. F. (1993). Assessment of vibration injury to Bartlett pears. In Transactions of the ASAE pp. 1043-1047. STENTZ, A. DIMA, C. WELLINGTON, C. HERMAN, H. & STAGER, D. (2002). A system for semi-autonomous tractor operations. In Autonomous Robots pp. 87-104. STEINMETZ, V. U. DELWICHE, M. J. GILES, D. K. & EVANS, R. (1994). Sorting cut roses with machine vision. In Transactions of the ASAE pp. 1347-1353. SULLIVAN, G. H. WILCOX, G. E. UYESHIRO, R. Y. & ROBERTSON, J. L. (1974). Precipitation and Economic Feasibility of Harvest Mechanization for Processing Tomatoes. In Hortsci pp. 389-391. TANG, S. DELWICHE, M. J. & THOMPSON, J. F. (1990). Development and evaluation of a defect sorter for prunes. In Paper - American Society of Agricultural Engineers p. 22 p. TAO, C. V. & LI, J. (2007). Advances in mobile mapping technology. In International Society for Photogrammetry and Remote Sensing (ISPRS) book series pp. xv, 176. London ; New York: Taylor & Francis. TAO, Y. MORROW, C. T. HEINEMANN, P. H. & SOMMER, H. J., III (1995). Fourier-based separation technique for the shape grading of potatoes using machine vision. In Transactions of the ASAE pp. 949-957. THAI, C. N. & SHEWFELT, R. L. (1990). Peach quality changes at different constant storage temperatures: empirical models. In Transactions of the ASAE pp. 227-233. THAI, C. N. SHEWFELT, R. L. & GARNER, J. C. (1990). Tomato color changes under constant and variable storage temperatures: empirical models. In Transactions of the ASAE pp. 607-614. THOMPSON, J. F. GRANT, J. A. KUPFERMAN, E. M. & KNUTSON, J. (1997). Reducing sweet cherry damage in postharvest operations. In HortTechnology pp. 134-138. THROOP, J. A. ANESHANSLEY, D. J. & UPCHURCH, B. L. (1994a). Camera system effects on detecting watercore in 'Red Delicious' apples. In Transactions of the ASAE pp. 873-877. TILLETT, N. (2003). Robots on the farm. In Industrial Robot-an International Journal pp. 396-397. TIMM, E. J. ARMSTRONG, P. R. BROWN, G. K. & BEAUDRY, R. M. (1993). A portable instrument for measuring firmness of cherries and berries. In Paper p. 17 p. TIMM, E. J. BROWN, G. K. ARMSTRONG, P. R. BEAUDRY, R. M. & SHIRAZI, A. (1996). Portable instrument for measuring firmness of cherries and berries. In Applied engineering in agriculture pp. 71-77. TIMM, E. J. BROWN, G. K. SCHULTE, N. L. & GUYER, D. E. (1993). Color measurements for dark sweet cherry maturity estimation in Michigan. In Paper p. 12 p. TIMM, E. J. GILLILAND, P. V. BROWN, G. K. & AFFELDT, H. A., JR. (1991). Potential methods for detecting pits in tart cherries. In Applied engineering in agriculture pp. 103-109. TIMM, E. J. & GUYER, D. E. (1998). Tart cherry firmness and quality changes during mechanical harvesting and handling. In Applied engineering in agriculture pp. 153-158. TOLLNER, E. W. HUNG, Y. C. UPCHURCH, B. L. & PRUSSIA, S. E. (1992a). Relating X-ray absorption to density and water content in apples. In Transactions of the ASAE pp. 1921-1928. TORII, T. (2000). Research in autonomous agriculture vehicles in Japan. In Computers and electronics in agriculture pp. 133-153. UPCHURCH, B. L. AFFELDT, H. A. HRUSCHKA, W. R. NORRIS, K. H. & THROOP, J. A. (1990). Spectrophotometric study of bruises on whole, 'Red Delicious' apples. In Transactions of the ASAE pp. 585-589. UPCHURCH, B. L. AFFELDT, H. A. HRUSCHKA, W. R. & THROOP, J. A. (1991). Optical detection of bruises and early frost damage on apples. In Transactions of the ASAE pp. 1004-1009. UPCHURCH, B. L. MILES, G. E. STROSHINE, R. L. FURGASON, E. S. & EMERSON, F. H. (1987). Ultrasonic measurement for detecting apple bruises. In Transactions of the ASAE - American Society of Agricultural Engineers pp. 803-809. WANG, Z., P.H. HEINEMANN, P. N. WALKER, AND C. HEUSER. (1999).Automated separation of micropropagated sugarcane shoots. Transactions of the American Society of Agricultural Engineers. 42(1): 247-254. WATADA, A. E. ABBOTT, J. A. & FINNEY, E. E. J. (1976). Firmness of Peaches Measured Nondestructively [Grading]. In J Am Soc Hortic Sci pp. 404-406. WU, N. Q. PITTS, M. J. DAVIS, D. C. CAVALIERI, R. P. & DRAKE, S. R. (1994). Modeling mechanical behavior of apple with the finite element method. In Paper p. 20 p. XIE, L. J. YING, Y. B. YU, H. Y. & FU, X. P. (2007). Application of near infrared spectroscopy technique to nondestructive measurement of vegetable quality. In Spectroscopy and Spectral Analysis pp. 1131-1135. ZHAO, K. CAVALIERI, R. P. HYDE, G. M. & PITTS, M. J. (1993). Apparent density of air-water mixtures. In Transactions of the ASAE pp. 103-111.