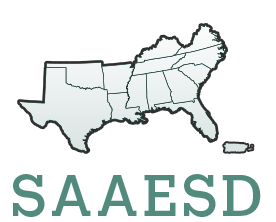
SCC84: Selection and mating strategies to improve dairy cattle performance, efficiency, and longevity
(Multistate Research Coordinating Committee and Information Exchange Group)
Status: Inactive/Terminating
Date of Annual Report: 04/12/2019
Report Information
Period the Report Covers: 10/01/2013 - 09/30/2018
Participants
John Cole – USDA-ARSAlbert De Vries – University of Florida
Chad Dechow – Penn State University
Leslie Hansen – University of Minnesota
Brad Heins – University of Minnesota
Heather Huson – Cornell University
Christian Maltecca - NCSU
Francisco Peñagaricano – University of Florida
Kent Weigel – University of Wisconsin
Joe West – University of Georgia
Lakshmi Kumar Matukumalli – USDA-NIFA
Students
Amy Hazel
Cassandra Stambuk
Michael Schmitt
Brief Summary of Minutes
Franscico Peñagaricano called to order as chair at 4:30pm
Brad Heins presented the secretaries report.
Introductions of participants and Guests.
Joe West gave a project report:
1) Impressed with number attending meeting and especially the number of graduate students.
2) Keep looking for new faculty for the project. Possible participants
James Koltes – Iowa State
Luis Brito – Purdue
Li Ma – University of Maryland
Thanks to Kent Weigel for hosting at Wisconsin
SCC84 was renewed this year.
Expired September 30, 2018
Renewed October 1, 2018 for 5 years September 30, 2023
Termination report is due 6 months from termination
March 31, 2019
Termination report includes: Impact Statement, All Publications, and Grants associated with the projects.
Everyone needs to make sure we move to new SCC84 project. Contract Ag Exp project personnel at our University to do that.
2019:
Chair- Brad Heins
Secretary – Christian Maltecca
Location – Pennsylvania
October 22-24, 2019
Lakshmi Matukumalli gave NIFA report
Respectfully submitted,
Brad Heins
Secretary SCC84
Accomplishments
<p><strong> </strong></p><br /> <p><strong>Objective 1. Share data and results from research on crossbreeding, inbreeding, and non-additive genetic effects in order to develop and recommend optimal mating strategies for commercial dairy producers</strong></p><br /> <p>Major Accomplishments and Outcomes:</p><br /> <ul><br /> <li>Identification of genomic regions impacting inbreeding depression in US dairy cattle (Christian Maltecca, North Carolina State University)</li><br /> <li>Development of Geno-Diver, a software designed to simulate complex traits with a quantitative and/or fitness component (Christian Maltecca, North Carolina State University)</li><br /> <li>Assessment of genetic diversity and genomic inbreeding in Jersey and Guernsey dairy cattle (Heather Huson, Cornell University)</li><br /> <li>Comparison of pure Holstein with Montbeliarde and Viking Red crossbreds (Leslie Hansen & Bradley Heins, University of Minnesota)</li><br /> <li>Evaluation of crossbreeding in organic dairy farms (Bradley Heins, University of Minnesota; Chad Dechow, Pennsylvania State University)</li><br /> </ul><br /> <p><strong>Objective 2. Exchange research results that will allow for refinement of USDA economic merit indexes</strong></p><br /> <p>Major Accomplishments and Outcomes:</p><br /> <ul><br /> <li>Refinement of economic selection indexes, including the incorporation of new traits (John Cole & Paul VanRaden, USDA)</li><br /> <li>Economic assessment of genetic testing and alternative reproduction strategies (Albert De Vries, University of Florida)</li><br /> <li>Evaluation of the effects of improving prediction of future cow performance on sire and calf selection decisions (Albert De Vries, University of Florida)</li><br /> <li>Genomic evaluations using single-step GBLUP (Ignacy Misztal, University of Georgia)</li><br /> </ul><br /> <p><strong>Objective 3. Capture phenotypic data for novel and economically important traits to elucidate their genetic regulation and potential for genomic selection</strong></p><br /> <p>Major Accomplishments and Outcomes:</p><br /> <ul><br /> <li>Genetic analysis of feed efficiency and related traits (Kent Weigel, University of Wisconsin; Chad Dechow, Pennsylvania State University; Rebecca Cockrum, Virginia Tech; Paul VanRaden, USDA)</li><br /> <li>Identification of genetic mechanisms underlying mastitis resistance, and relationship to mammary microbiota (Heather Huson, Cornell University)</li><br /> <li>Genetic analysis of digital cushion thickness (Heather Huson, Cornell University)</li><br /> <li>Genetic regulation of ketosis (Heather Huson, Cornell University; Kent Weigel, University of Wisconsin; Rebecca Cockrum, Virginia Tech)</li><br /> <li>Effects of dietary components on gene expression and rumen microbiome in preweaned dairy heifers (Rebecca Cockrum, Virginia Tech)</li><br /> <li>Genetic analysis of calf health and mortality (Kent Weigel, University of Wisconsin)</li><br /> <li>Development of national genetic evaluations for six postpartum disorders, namely mastitis, ketosis, milk fever, displaced abomasum, metritis, and retained placenta (John Cole & Paul VanRaden, USDA; Christian Maltecca, North Carolina State University)</li><br /> <li>Development of a national genetic evaluation for gestation length (John Cole & Paul VanRaden, USDA)</li><br /> <li>Evaluation of genotype by environment (climate) interaction (Christian Maltecca, North Carolina State University; Francisco Peñagaricano, University of Florida)</li><br /> <li>Genetic dissection of dairy bull fertility: from fine mapping to genomic prediction (Francisco Peñagaricano, University of Florida)</li><br /> </ul><br /> <p><strong>Objective 4. Coordinate with the National Animal Germplasm Program Dairy Committee to optimize its dairy collection</strong></p><br /> <p>Major Accomplishments and Outcomes:</p><br /> <ul><br /> <li>Recovery of a lost Holstein male lineage (Chad Dechow, Pennsylvania State University)</li><br /> </ul><br /> <p> </p>Publications
<p>Effect of diet energy density and genomic residual feed intake on prebred dairy heifer feed efficiency, growth, and manure excretion.</p><br /> <p>Williams KT, Weigel KA, Coblentz WK, Esser NM, Schlesser H, Hoffman PC, Su H, Akins MS. J Dairy Sci. 2019 [Epub ahead of print]</p><br /> <p>Genetic Selection for Mastitis Resistance.</p><br /> <p>Weigel KA, Shook GE.Vet Clin North Am Food Anim Pract. 2018 Nov;34(3):457-472.</p><br /> <p>Genome-wide association analyses based on a multiple-trait approach for modeling feed efficiency.</p><br /> <p>Lu Y, Vandehaar MJ, Spurlock DM, Weigel KA, Armentano LE, Connor EE, Coffey M, Veerkamp RF, de Haas Y, Staples CR, Wang Z, Hanigan MD, Tempelman RJ. J Dairy Sci. 2018 Apr;101(4):3140-3154.</p><br /> <p>A 100-Year Review: Methods and impact of genetic selection in dairy cattle-From daughter-dam comparisons to deep learning algorithms.</p><br /> <p>Weigel KA, VanRaden PM, Norman HD, Grosu H. J Dairy Sci. 2017 Dec;100(12):10234-10250.</p><br /> <p>The genetic and biological basis of feed efficiency in mid-lactation Holstein dairy cows.</p><br /> <p>Hardie LC, VandeHaar MJ, Tempelman RJ, Weigel KA, Armentano LE, Wiggans GR, Veerkamp RF, de Haas Y, Coffey MP, Connor EE, Hanigan MD, Staples C, Wang Z, Dekkers JCM, Spurlock DM. J Dairy Sci. 2017 Nov;100(11):9061-9075.</p><br /> <p>Prediction of whole-genome risk for selection and management of hyperketonemia in Holstein dairy cattle.</p><br /> <p>Weigel KA, Pralle RS, Adams H, Cho K, Do C, White HM. J Anim Breed Genet. 2017 Jun;134(3):275-285.</p><br /> <p>Relationships between body condition score change, prior mid-lactation phenotypic residual feed intake, and hyperketonemia onset in transition dairy cows.</p><br /> <p>Rathbun FM, Pralle RS, Bertics SJ, Armentano LE, Cho K, Do C, Weigel KA, White HM.</p><br /> <p>J Dairy Sci. 2017 May;100(5):3685-3696.</p><br /> <p>Use of genotype × environment interaction model to accommodate genetic heterogeneity for residual feed intake, dry matter intake, net energy in milk, and metabolic body weight in dairy cattle.</p><br /> <p>Yao C, de Los Campos G, VandeHaar MJ, Spurlock DM, Armentano LE, Coffey M, de Haas Y, Veerkamp RF, Staples CR, Connor EE, Wang Z, Hanigan MD, Tempelman RJ, Weigel KA. J Dairy Sci. 2017 Mar;100(3):2007-2016.</p><br /> <p>Assessing genomic prediction accuracy for Holstein sires using bootstrap aggregation sampling and leave-one-out cross validation.</p><br /> <p>Mikshowsky AA, Gianola D, Weigel KA. J Dairy Sci. 2017 Jan;100(1):453-464.</p><br /> <p>Modeling genetic and nongenetic variation of feed efficiency and its partial relationships between component traits as a function of management and environmental factors.</p><br /> <p>Lu Y, Vandehaar MJ, Spurlock DM, Weigel KA, Armentano LE, Staples CR, Connor EE, Wang Z, Coffey M, Veerkamp RF, de Haas Y, Tempelman RJ. J Dairy Sci. 2017 Jan;100(1):412-427.</p><br /> <p>Semi-supervised learning for genomic prediction of novel traits with small reference populations: an application to residual feed intake in dairy cattle.</p><br /> <p>Yao C, Zhu X, Weigel KA. Genet Sel Evol. 2016 Nov 7;48(1):84.</p><br /> <p>Genome-wide association mapping and pathway analysis of leukosis incidence in a US Holstein cattle population.</p><br /> <p>Abdalla EA, Peñagaricano F, Byrem TM, Weigel KA, Rosa GJ. Anim Genet. 2016 Aug;47(4):395-407.</p><br /> <p>Improving reliability of genomic predictions for Jersey sires using bootstrap aggregation sampling.</p><br /> <p>Mikshowsky AA, Gianola D, Weigel KA. J Dairy Sci. 2016 May;99(5):3632-3645.</p><br /> <p>Short communication: Genetic correlation of bovine leukosis incidence with somatic cell score and milk yield in a US Holstein population.</p><br /> <p>Abdalla EA, Weigel KA, Byrem TM, Rosa GJM. J Dairy Sci. 2016 Mar;99(3):2005-2009.</p><br /> <p>Genetic parameters between feed-intake-related traits and conformation in 2 separate dairy populations--the Netherlands and United States.</p><br /> <p>Manzanilla-Pech CI, Veerkamp RF, Tempelman RJ, van Pelt ML, Weigel KA, VandeHaar M, Lawlor TJ, Spurlock DM, Armentano LE, Staples CR, Hanigan M, De Haas Y. J Dairy Sci. 2016 Jan;99(1):443-57.</p><br /> <p>An alternative approach to modeling genetic merit of feed efficiency in dairy cattle.</p><br /> <p>Lu Y, Vandehaar MJ, Spurlock DM, Weigel KA, Armentano LE, Staples CR, Connor EE, Wang Z, Bello NM, Tempelman RJ. J Dairy Sci. 2015 Sep;98(9):6535-51.</p><br /> <p>Using a family-based structure to detect the effects of genomic inbreeding on embryo viability in Holstein cattle.</p><br /> <p>Bjelland DW, Weigel KA, Coburn AD, Wilson RD. J Dairy Sci. 2015 Jul;98(7):4934-44.</p><br /> <p>Optimization of reproductive management programs using lift chart analysis and cost-sensitive evaluation of classification errors.</p><br /> <p>Shahinfar S, Guenther JN, Page CD, Kalantari AS, Cabrera VE, Fricke PM, Weigel KA. J Dairy Sci. 2015 Jun;98(6):3717-28.</p><br /> <p>Considerations when combining data from multiple nutrition experiments to estimate genetic parameters for feed efficiency.</p><br /> <p>Hardie LC, Armentano LE, Shaver RD, VandeHaar MJ, Spurlock DM, Yao C, Bertics SJ, Contreras-Govea FE, Weigel KA. J Dairy Sci. 2015 Apr;98(4):2727-37.</p><br /> <p>Heterogeneity in genetic and nongenetic variation and energy sink relationships for residual feed intake across research stations and countries.</p><br /> <p>Tempelman RJ, Spurlock DM, Coffey M, Veerkamp RF, Armentano LE, Weigel KA, de Haas Y, Staples CR, Connor EE, Lu Y, VandeHaar MJ. J Dairy Sci. 2015 Mar;98(3):2013-26.</p><br /> <p>Short communication: Use of single nucleotide polymorphism genotypes and health history to predict future phenotypes for milk production, dry matter intake, body weight, and residual feed intake in dairy cattle.</p><br /> <p>Yao C, Armentano LE, VandeHaar MJ, Weigel KA. J Dairy Sci. 2015 Mar;98(3):2027-32.</p><br /> <p>Prediction of genetic contributions to complex traits using whole genome sequencing data.</p><br /> <p>Yao C, Leng N, Weigel KA, Lee KE, Engelman CD, Meyers KJ. BMC Proc. 2014 Jun 17;8(Suppl 1): S68.</p><br /> <p>Applied animal genomics: results from the field.</p><br /> <p>Van Eenennaam AL, Weigel KA, Young AE, Cleveland MA, Dekkers JC. Annu Rev Anim Biosci. 2014 Feb; 2:105-39.</p><br /> <p>Meta-analysis of candidate gene effects using Bayesian parametric and non-parametric approaches.</p><br /> <p>Wu XL, Gianola D, Rosa GJ, Weigel KA. J Genomics. 2014 Jan 2; 2:1-19.</p><br /> <p>Enhancing genome-enabled prediction by bagging genomic BLUP.</p><br /> <p>Gianola D, Weigel KA, Krämer N, Stella A, Schön CC. PLoS One. 2014 Apr 10;9(4): e91693.</p><br /> <p>Short communication: genetic evaluation of stillbirth in US Brown Swiss and Jersey cattle.</p><br /> <p>Yao C, Weigel KA, Cole JB. J Dairy Sci. 2014;97(4):2474-80.</p><br /> <p>Imputation of genotypes from low density (50,000 markers) to high density (700,000 markers) of cows from research herds in Europe, North America, and Australasia using 2 reference populations.</p><br /> <p>Pryce JE, Johnston J, Hayes BJ, Sahana G, Weigel KA 2nd, McParland S, Spurlock D, Krattenmacher N, Spelman RJ, Wall E, Calus MP.</p><br /> <p>J Dairy Sci. 2014 Mar;97(3):1799-811.</p><br /> <p>Three-breed rotational crossbreds of Montbéliarde, Viking Red, and Holstein compared with Holstein cows for feed efficiency, income over feed cost, and residual feed intake.</p><br /> <p>Shonka-Martin BN, Heins BJ, Hansen LB. J Dairy Sci. 2019 Apr;102(4):3661-3673.</p><br /> <p>Three-breed rotational crossbreds of Montbéliarde, Viking Red, and Holstein compared with Holstein cows for dry matter intake, body traits, and production.</p><br /> <p>Shonka-Martin BN, Hazel AR, Heins BJ, Hansen LB. J Dairy Sci. 2019 Jan;102(1):871-882.</p><br /> <p>Effects of winter housing systems on production, economics, body weight, body condition score, and bedding cultures for organic dairy cows.</p><br /> <p>Heins BJ, Sjostrom LS, Endres MI, Carillo MR, King R, Moon RD, Sorge US. J Dairy Sci. 2019 Jan;102(1):706-714.</p><br /> <p>Relationships between protein and energy consumed from milk replacer and starter and calf growth and first-lactation production of Holstein dairy cows.</p><br /> <p>Rauba J, Heins BJ, Chester-Jones H, Diaz HL, Ziegler D, Linn J, Broadwater N. J Dairy Sci. 2019 Jan;102(1):301-310.</p><br /> <p>Enhancing the fatty acid profile of milk through forage-based rations, with nutrition modeling of diet outcomes.</p><br /> <p>Benbrook CM, Davis DR, Heins BJ, Latif MA, Leifert C, Peterman L, Butler G, Faergeman O, Abel-Caines S, Baranski M. Food Sci Nutr. 2018 Feb 28;6(3):681-700.</p><br /> <p>Evaluation of a commercial vacuum fly trap for controlling flies on organic dairy farms.</p><br /> <p>Kienitz MJ, Heins BJ, Moon RD. J Dairy Sci. 2018 May;101(5):4667-4675.</p><br /> <p>Technical note: Validation of an ear-tag accelerometer sensor to determine rumination, eating, and activity behaviors of grazing dairy cattle.</p><br /> <p>Pereira GM, Heins BJ, Endres MI. J Dairy Sci. 2018 Mar;101(3):2492-2495.</p><br /> <p>Fertility, survival, and conformation of Montbéliarde × Holstein and Viking Red × Holstein crossbred cows compared with pure Holstein cows during first lactation in 8 commercial dairy herds.</p><br /> <p>Hazel AR, Heins BJ, Hansen LB. J Dairy Sci. 2017 Nov;100(11):9447-9458.</p><br /> <p>Short communication: Verifying Holstein heifer heart girth to body weight prediction equations.</p><br /> <p>Heinrichs AJ, Heinrichs BS, Jones CM, Erickson PS, Kalscheur KF, Nennich TD, Heins BJ, Cardoso FC. J Dairy Sci. 2017 Oct;100(10):8451-8454.</p><br /> <p>Relationships between early-life growth, intake, and birth season with first-lactation performance of Holstein dairy cows.</p><br /> <p>Chester-Jones H, Heins BJ, Ziegler D, Schimek D, Schuling S, Ziegler B, de Ondarza MB, Sniffen CJ, Broadwater N. J Dairy Sci. 2017 May;100(5):3697-3704.</p><br /> <p>Production and calving traits of Montbéliarde × Holstein and Viking Red × Holstein cows compared with pure Holstein cows during first lactation in 8 commercial dairy herds.</p><br /> <p>Hazel AR, Heins BJ, Hansen LB. J Dairy Sci. 2017 May;100(5):4139-4149.</p><br /> <p>Growth, behavior, and economics of group-fed dairy calves fed once or twice daily in an organic production system.</p><br /> <p>Kienitz MJ, Heins BJ, Chester-Jones H. J Dairy Sci. 2017 Apr;100(4):3318-3325.</p><br /> <p>Short communication: Relationship of activity and rumination to abundance of pest flies among organically certified cows fed 3 levels of concentrate.</p><br /> <p>Sjostrom LS, Heins BJ, Endres MI, Moon RD, Paulson JC. J Dairy Sci. 2016 Dec;99(12):9942-9948.</p><br /> <p>Crossbreeding: implications for dairy cow fertility and survival.</p><br /> <p>Buckley F, Lopez-Villalobos N, Heins BJ. Animal. 2014 May;8 Suppl 1:122-33.</p><br /> <p>Production, fertility, survival, and body measurements of Montbéliarde-sired crossbreds compared with pure Holsteins during their first 5 lactations.</p><br /> <p>Hazel AR, Heins BJ, Seykora AJ, Hansen LB. J Dairy Sci. 2014;97(4):2512-25.</p><br /> <p>Comparison of peripartum metabolic status and postpartum health of Holstein and Montbéliarde-sired crossbred dairy cows.</p><br /> <p>Mendonça LG, Abade CC, da Silva EM, Litherland NB, Hansen LB, Hansen WP, Chebel RC. J Dairy Sci. 2014 Feb;97(2):805-18.</p><br /> <p>Symposium review: The genomic architecture of inbreeding: How homozygosity affects health and performance.</p><br /> <p>Baes CF, Makanjuola BO, Miglior F, Marras G, Howard JT, Fleming A, Maltecca C. J Dairy Sci. 2019 Mar;102(3):2807-2817.</p><br /> <p>Including gene networks to predict calving difficulty in Holstein, Brown Swiss and Jersey cattle.</p><br /> <p>Tiezzi F, Arceo ME, Cole JB, Maltecca C. BMC Genet. 2018 Apr 2;19(1):20.</p><br /> <p>Effect of genetic architecture on the prediction accuracy of quantitative traits in samples of unrelated individuals.</p><br /> <p>Morgante F, Huang W, Maltecca C, Mackay TFC. Heredity (Edinb). 2018 Jun;120(6):500-514.</p><br /> <p>Rumination time as a potential predictor of common diseases in high-productive Holstein dairy cows.</p><br /> <p>Moretti R, Biffani S, Tiezzi F, Maltecca C, Chessa S, Bozzi R. J Dairy Res. 2017 Nov;84(4):385-390.</p><br /> <p>Invited review: Inbreeding in the genomics era: Inbreeding, inbreeding depression, and management of genomic variability.</p><br /> <p>Howard JT, Pryce JE, Baes C, Maltecca C. J Dairy Sci. 2017 Aug;100(8):6009-6024.</p><br /> <p>Geno-Diver: A combined coalescence and forward-in-time simulator for populations undergoing selection for complex traits.</p><br /> <p>Howard JT, Tiezzi F, Pryce JE, Maltecca C. J Anim Breed Genet. 2017 Dec;134(6):553-563.</p><br /> <p>Genotype by environment (climate) interaction improves genomic prediction for production traits in US Holstein cattle.</p><br /> <p>Tiezzi F, de Los Campos G, Parker Gaddis KL, Maltecca C. J Dairy Sci. 2017 Mar;100(3):2042-2056.</p><br /> <p>Benchmarking dairy herd health status using routinely recorded herd summary data.</p><br /> <p>Parker Gaddis KL, Cole JB, Clay JS, Maltecca C. J Dairy Sci. 2016 Feb;99(2):1298-1314.</p><br /> <p>Investigation of regions impacting inbreeding depression and their association with the additive genetic effect for United States and Australia Jersey dairy cattle.</p><br /> <p>Howard JT, Haile-Mariam M, Pryce JE, Maltecca C. BMC Genomics. 2015 Oct 19; 16:813.</p><br /> <p>Causal relationships between milk quality and coagulation properties in Italian Holstein-Friesian dairy cattle.</p><br /> <p>Tiezzi F, Valente BD, Cassandro M, Maltecca C. Genet Sel Evol. 2015 May 13; 47:45.</p><br /> <p>Genomic prediction of disease occurrence using producer-recorded health data: a comparison of methods.</p><br /> <p>Parker Gaddis KL, Tiezzi F, Cole JB, Clay JS, Maltecca C. Genet Sel Evol. 2015 May 8; 47:41.</p><br /> <p>Accounting for trait architecture in genomic predictions of US Holstein cattle using a weighted realized relationship matrix.</p><br /> <p>Tiezzi F, Maltecca C. Genet Sel Evol. 2015 Apr 2; 47:24.</p><br /> <p>Characterizing homozygosity across United States, New Zealand and Australian Jersey cow and bull populations.</p><br /> <p>Howard JT, Maltecca C, Haile-Mariam M, Hayes BJ, Pryce JE. BMC Genomics. 2015 Mar 15; 16:187.</p><br /> <p>Short communication: Genomic selection for hoof lesions in first-parity US Holsteins.</p><br /> <p>Dhakal K, Tiezzi F, Clay JS, Maltecca C.</p><br /> <p>J Dairy Sci. 2015 May;98(5):3502-7.</p><br /> <p>A genome-wide association study for clinical mastitis in first parity US Holstein cows using single-step approach and genomic matrix re-weighting procedure.</p><br /> <p>Tiezzi F, Parker-Gaddis KL, Cole JB, Clay JS, Maltecca C. PLoS One. 2015 Feb 6;10(2): e0114919.</p><br /> <p>Inferring causal relationships between reproductive and metabolic health disorders and production traits in first-lactation US Holsteins using recursive models.</p><br /> <p>Dhakal K, Tiezzi F, Clay JS, Maltecca C. J Dairy Sci. 2015 Apr;98(4):2713-26.</p><br /> <p>Genomic selection for producer-recorded health event data in US dairy cattle.</p><br /> <p>Parker Gaddis KL, Cole JB, Clay JS, Maltecca C. J Dairy Sci. 2014 May;97(5):3190-9.</p><br /> <p>Udder and teat conformational risk factors for elevated somatic cell count and clinical mastitis in New York Holsteins.</p><br /> <p>Miles AM, McArt JAA, Leal Yepes FA, Stambuk CR, Virkler PD, Huson HJ. Prev Vet Med. 2019 Feb 1; 163:7-13.</p><br /> <p>Genomic evaluation, breed identification, and population structure of Guernsey cattle in North America, Great Britain, and the Isle of Guernsey.</p><br /> <p>Cooper TA, Eaglen SAE, Wiggans GR, Jenko J, Huson HJ, Morrice DR, Bichard M, Luff WGL, Woolliams JA. J Dairy Sci. 2016 Jul;99(7):5508-5515.</p><br /> <p>Genetic differentiation of Mexican Holstein cattle and its relationship with Canadian and U.S. Holsteins.</p><br /> <p>García-Ruiz A, Ruiz-López F, Van Tassell CP, Montaldo HH, Huson HJ. Front Genet. 2015 Feb 9; 6:7.</p><br /> <p>The SLICK hair locus derived from Senepol cattle confers thermotolerance to intensively managed lactating Holstein cows.</p><br /> <p>Dikmen S, Khan FA, Huson HJ, Sonstegard TS, Moss JI, Dahl GE, Hansen PJ. J Dairy Sci. 2014 Sep;97(9):5508-20.</p><br /> <p>Genome-wide association study and ancestral origins of the slick-hair coat in tropically adapted cattle.</p><br /> <p>Huson HJ, Kim ES, Godfrey RW, Olson TA, McClure MC, Chase CC, Rizzi R, O'Brien AM, Van Tassell CP, Garcia JF, Sonstegard TS. Front Genet. 2014 Apr 29; 5:101.</p><br /> <p>Varying dietary protein and fat elicits differential transcriptomic expression within stress response pathways in preweaned Holstein heifers.</p><br /> <p>Owens CE, Geiger AJ, Akers RM, Cockrum RR. J Dairy Sci. 2019 Feb;102(2):1630-1641.</p><br /> <p>Diet shifts provoke complex and variable changes in the metabolic networks of the ruminal microbiome.</p><br /> <p>Wolff SM, Ellison MJ, Hao Y, Cockrum RR, Austin KJ, Baraboo M, Burch K, Lee HJ, Maurer T, Patil R, Ravelo A, Taxis TM, Truong H, Lamberson WR, Cammack KM, Conant GC. Microbiome. 2017 Jun 8;5(1):60.</p><br /> <p>Genetic and epigenetic architecture of paternal origin contribute to gestation length in cattle.</p><br /> <p>Fang L, Jiang J, Li B, Zhou Y, Freebern E, Vanraden PM, Cole JB, Liu GE, Ma L. Commun Biol. 2019 Mar 14; 2:100.</p><br /> <p>Comparison of gene editing versus conventional breeding to introgress the POLLED allele into the US dairy cattle population.</p><br /> <p>Mueller ML, Cole JB, Sonstegard TS, Van Eenennaam AL. J Dairy Sci. 2019 Mar 6.</p><br /> <p>Array CGH-based detection of CNV regions and their potential association with reproduction and other economic traits in Holsteins.</p><br /> <p>Liu M, Fang L, Liu S, Pan MG, Seroussi E, Cole JB, Ma L, Chen H, Liu GE. BMC Genomics. 2019 Mar 7;20(1):181.</p><br /> <p>Comparative analyses of sperm DNA methylomes among human, mouse and cattle provide insights into epigenomic evolution and complex traits.</p><br /> <p>Fang L, Zhou Y, Liu S, Jiang J, Bickhart DM, Null DJ, Li B, Schroeder SG, Rosen BD, Cole JB, Van Tassell CP, Ma L, Liu GE. Epigenetics. 2019 Feb 27:1-17.</p><br /> <p>Calling known variants and identifying new variants while rapidly aligning sequence data.</p><br /> <p>VanRaden PM, Bickhart DM, O'Connell JR. J Dairy Sci. 2019 Apr;102(4):3216-3229.</p><br /> <p>Modeling pedigree accuracy and uncertain parentage in single-step genomic evaluations of simulated and US Holstein datasets.</p><br /> <p>Bradford HL, Masuda Y, Cole JB, Misztal I, VanRaden PM. J Dairy Sci. 2019 Mar;102(3):2308-2318.</p><br /> <p>Modeling missing pedigree in single-step genomic BLUP.</p><br /> <p>Bradford HL, Masuda Y, VanRaden PM, Legarra A, Misztal I. J Dairy Sci. 2019 Mar;102(3):2336-2346.</p><br /> <p>Symposium review: Genetics, genome-wide association study, and genetic improvement of dairy fertility traits.</p><br /> <p>Ma L, Cole JB, Da Y, VanRaden PM. J Dairy Sci. 2019 Apr;102(4):3735-3743.</p><br /> <p>Genetic and nongenetic profiling of milk pregnancy-associated glycoproteins in Holstein cattle.</p><br /> <p>Santos DJA, Cole JB, Null DJ, Byrem TM, Ma L.</p><br /> <p>J Dairy Sci. 2018 Nov;101(11):9987-10000.</p><br /> <p> </p><br /> <p>Determination of quantitative trait nucleotides by concordance analysis between quantitative trait loci and marker genotypes of US Holsteins.</p><br /> <p>Weller JI, Bickhart DM, Wiggans GR, Tooker ME, O'Connell JR, Jiang J, Ron M, VanRaden PM. J Dairy Sci. 2018 Oct;101(10):9089-9107.</p><br /> <p>Differing genetic trend estimates from traditional and genomic evaluations of genotyped animals as evidence of preselection bias in US Holsteins.</p><br /> <p>Masuda Y, VanRaden PM, Misztal I, Lawlor TJ. J Dairy Sci. 2018 Jun;101(6):5194-5206.</p><br /> <p>Convergent Evolution of Slick Coat in Cattle through Truncation Mutations in the Prolactin Receptor.</p><br /> <p>Porto-Neto LR, Bickhart DM, Landaeta-Hernandez AJ, Utsunomiya YT, Pagan M, Jimenez E, Hansen PJ, Dikmen S, Schroeder SG, Kim ES, Sun J, Crespo E, Amati N, Cole JB, Null DJ, Garcia JF, Reverter A, Barendse W, Sonstegard TS. Front Genet. 2018 Feb 23; 9:57.</p><br /> <p>Invited review: Genetics and claw health: Opportunities to enhance claw health by genetic selection.</p><br /> <p>Heringstad B, Egger-Danner C, Charfeddine N, Pryce JE, Stock KF, Kofler J, Sogstad AM, Holzhauer M, Fiedler A, Müller K, Nielsen P, Thomas G, Gengler N, de Jong G, Ødegård C, Malchiodi F, Miglior F, Alsaaod M, Cole JB. J Dairy Sci. 2018 Jun;101(6):4801-4821.</p><br /> <p>Symposium review: Possibilities in an age of genomics: The future of selection indices.</p><br /> <p>Cole JB, VanRaden PM. J Dairy Sci. 2018 Apr;101(4):3686-3701.</p><br /> <p>Genotype imputation in a tropical crossbred dairy cattle population.</p><br /> <p>Oliveira Júnior GA, Chud TCS, Ventura RV, Garrick DJ, Cole JB, Munari DP, Ferraz JBS, Mullart E, DeNise S, Smith S, da Silva MVGB. J Dairy Sci. 2017 Dec;100(12):9623-9634.</p><br /> <p>Genomic evaluation of age at first calving.</p><br /> <p>Hutchison JL, VanRaden PM, Null DJ, Cole JB, Bickhart DM. J Dairy Sci. 2017 Aug;100(8):6853-6861.</p><br /> <p>Dissection of additive, dominance, and imprinting effects for production and reproduction traits in Holstein cattle.</p><br /> <p>Jiang J, Shen B, O'Connell JR, VanRaden PM, Cole JB, Ma L. BMC Genomics. 2017 May 30;18(1):425.</p><br /> <p>Economic considerations of breeding for polled dairy cows versus dehorning in the United States.</p><br /> <p>Thompson NM, Widmar NO, Schutz MM, Cole JB, Wolf CA. J Dairy Sci. 2017 Jun;100(6):4941-4952.</p><br /> <p>A single nucleotide polymorphism in COQ9 affects mitochondrial and ovarian function and fertility in Holstein cows.</p><br /> <p>Ortega MS, Wohlgemuth S, Tribulo P, Siqueira LG, Cole JB, Hansen PJ. Biol Reprod. 2017 Mar 1;96(3):652-663.</p><br /> <p>Single nucleotide variants and InDels identified from whole-genome re-sequencing of Guzerat, Gyr, Girolando and Holstein cattle breeds.</p><br /> <p>Stafuzza NB, Zerlotini A, Lobo FP, Yamagishi ME, Chud TC, Caetano AR, Munari DP, Garrick DJ, Machado MA, Martins MF, Carvalho MR, Cole JB, Barbosa da Silva MV.</p><br /> <p>PLoS One. 2017 Mar 21;12(3): e0173954.</p><br /> <p>Selecting sequence variants to improve genomic predictions for dairy cattle.</p><br /> <p>VanRaden PM, Tooker ME, O'Connell JR, Cole JB, Bickhart DM. Genet Sel Evol. 2017 Mar 7;49(1):32.</p><br /> <p>Association of single nucleotide polymorphisms in candidate genes previously related to genetic variation in fertility with phenotypic measurements of reproductive function in Holstein cows.</p><br /> <p>Ortega MS, Denicol AC, Cole JB, Null DJ, Taylor JF, Schnabel RD, Hansen PJ. J Dairy Sci. 2017 May;100(5):3725-3734.</p><br /> <p>Evaluation of genetic components in traits related to superovulation, in vitro fertilization, and embryo transfer in Holstein cattle.</p><br /> <p>Parker Gaddis KL, Dikmen S, Null DJ, Cole JB, Hansen PJ. J Dairy Sci. 2017 Apr;100(4):2877-2891.</p><br /> <p>Genomic Selection in Dairy Cattle: The USDA Experience.</p><br /> <p>Wiggans GR, Cole JB, Hubbard SM, Sonstegard TS. Annu Rev Anim Biosci. 2017 Feb 8; 5:309-327.</p><br /> <p>Phenotypic and genetic effects of recessive haplotypes on yield, longevity, and fertility.</p><br /> <p>Cole JB, Null DJ, VanRaden PM. J Dairy Sci. 2016 Sep;99(9):7274-7288.</p><br /> <p>Invited review: Opportunities for genetic improvement of metabolic diseases.</p><br /> <p>Pryce JE, Parker Gaddis KL, Koeck A, Bastin C, Abdelsayed M, Gengler N, Miglior F, Heringstad B, Egger-Danner C, Stock KF, Bradley AJ, Cole JB. J Dairy Sci. 2016 Sep;99(9):6855-6873.</p><br /> <p>Changes in genetic selection differentials and generation intervals in US Holstein dairy cattle as a result of genomic selection.</p><br /> <p>García-Ruiz A, Cole JB, VanRaden PM, Wiggans GR, Ruiz-López FJ, Van Tassell CP. Proc Natl Acad Sci U S A. 2016 Jul 12;113(28): E3995-4004.</p><br /> <p>Identification of a nonsense mutation in APAF1 that is likely causal for a decrease in reproductive efficiency in Holstein dairy cattle.</p><br /> <p>Adams HA, Sonstegard TS, VanRaden PM, Null DJ, Van Tassell CP, Larkin DM, Lewin HA. J Dairy Sci. 2016 Aug;99(8):6693-6701.</p><br /> <p>Explorations in genome-wide association studies and network analyses with dairy cattle fertility traits.</p><br /> <p>Parker Gaddis KL, Null DJ, Cole JB. J Dairy Sci. 2016 Aug;99(8):6420-6435.</p><br /> <p>Reducing animal sequencing redundancy by preferentially selecting animals with low-frequency haplotypes.</p><br /> <p>Bickhart DM, Hutchison JL, Null DJ, VanRaden PM, Cole JB. J Dairy Sci. 2016 Jul;99(7):5526-5534.</p><br /> <p>Diversity and population-genetic properties of copy number variations and multicopy genes in cattle.</p><br /> <p>Bickhart DM, Xu L, Hutchison JL, Cole JB, Null DJ, Schroeder SG, Song J, Garcia JF, Sonstegard TS, Van Tassell CP, Schnabel RD, Taylor JF, Lewin HA, Liu GE. DNA Res. 2016 Jun;23(3):253-62.</p><br /> <p>Increasing the number of single nucleotide polymorphisms used in genomic evaluation of dairy cattle.</p><br /> <p>Wiggans GR, Cooper TA, VanRaden PM, Van Tassell CP, Bickhart DM, Sonstegard TS. J Dairy Sci. 2016 Jun;99(6):4504-4511.</p><br /> <p>Use of single nucleotide polymorphisms in candidate genes associated with daughter pregnancy rate for prediction of genetic merit for reproduction in Holstein cows.</p><br /> <p>Ortega MS, Denicol AC, Cole JB, Null DJ, Hansen PJ. Anim Genet. 2016 Jun;47(3):288-97.</p><br /> <p>Practical implications for genetic modeling in the genomics era.</p><br /> <p>VanRaden PM. J Dairy Sci. 2016 Mar;99(3):2405-2412.</p><br /> <p>A simple strategy for managing many recessive disorders in a dairy cattle breeding program.</p><br /> <p>Cole JB. Genet Sel Evol. 2015 Nov 30; 47:94.</p><br /> <p>Cattle Sex-Specific Recombination and Genetic Control from a Large Pedigree Analysis.</p><br /> <p>Ma L, O'Connell JR, VanRaden PM, Shen B, Padhi A, Sun C, Bickhart DM, Cole JB, Null DJ, Liu GE, Da Y, Wiggans GR. PLoS Genet. 2015 Nov 5;11(11): e1005387.</p><br /> <p>Single nucleotide polymorphisms associated with thermoregulation in lactating dairy cows exposed to heat stress.</p><br /> <p>Dikmen S, Wang XZ, Ortega MS, Cole JB, Null DJ, Hansen PJ. J Anim Breed Genet. 2015 Dec;132(6):409-19.</p><br /> <p>Fast imputation using medium or low-coverage sequence data.</p><br /> <p>VanRaden PM, Sun C, O'Connell JR. BMC Genet. 2015 Jul 14; 16:82.</p><br /> <p>Short communication: Improving accuracy of Jersey genomic evaluations in the United States and Denmark by sharing reference population bulls.</p><br /> <p>Wiggans GR, Su G, Cooper TA, Nielsen US, Aamand GP, Guldbrandtsen B, Lund MS, VanRaden PM. J Dairy Sci. 2015 May;98(5):3508-13.</p><br /> <p>Assessment of autozygosity in Nellore cows (Bos indicus) through high-density SNP genotypes.</p><br /> <p>Zavarez LB, Utsunomiya YT, Carmo AS, Neves HH, Carvalheiro R, Ferenčaković M, Pérez O'Brien AM, Curik I, Cole JB, Van Tassell CP, da Silva MV, Sonstegard TS, Sölkner J, Garcia JF. Front Genet. 2015 Jan 29; 6:5.</p><br /> <p>Short communication: Analysis of genomic predictor population for Holstein dairy cattle in the United States--Effects of sex and age.</p><br /> <p>Cooper TA, Wiggans GR, VanRaden PM. J Dairy Sci. 2015 Apr;98(4):2785-8.</p><br /> <p>Technical note: Rapid calculation of genomic evaluations for new animals.</p><br /> <p>Wiggans GR, VanRaden PM, Cooper TA. J Dairy Sci. 2015 Mar;98(3):2039-42.</p><br /> <p>Genomic signatures reveal new evidences for selection of important traits in domestic cattle.</p><br /> <p>Xu L, Bickhart DM, Cole JB, Schroeder SG, Song J, Tassell CP, Sonstegard TS, Liu GE. Mol Biol Evol. 2015 Mar;32(3):711-25.</p><br /> <p>Invited review: overview of new traits and phenotyping strategies in dairy cattle with a focus on functional traits.</p><br /> <p>Egger-Danner C, Cole JB, Pryce JE, Gengler N, Heringstad B, Bradley A, Stock KF. Animal. 2015 Feb;9(2):191-207.</p><br /> <p>Comparison of single-trait to multi-trait national evaluations for yield, health, and fertility.</p><br /> <p>VanRaden PM, Tooker ME, Wright JR, Sun C, Hutchison JL. J Dairy Sci. 2014 Dec;97(12):7952-62.</p><br /> <p>Genome wide CNV analysis reveals additional variants associated with milk production traits in Holsteins.</p><br /> <p>Xu L, Cole JB, Bickhart DM, Hou Y, Song J, VanRaden PM, Sonstegard TS, Van Tassell CP, Liu GE. BMC Genomics. 2014 Aug 15; 15:683.</p><br /> <p>Improvement of prediction ability for genomic selection of dairy cattle by including dominance effects.</p><br /> <p>Sun C, VanRaden PM, Cole JB, O'Connell JR. PLoS One. 2014 Aug 1;9(8): e103934.</p><br /> <p>Development of a Lifetime Merit-based selection index for US dairy grazing systems.</p><br /> <p>Gay KD, Widmar NJ, Nennich TD, Schinckel AP, Cole JB, Schutz MM. J Dairy Sci. 2014 Jul;97(7):4568-78.</p><br /> <p>Genome-wide association study of reproductive efficiency in female cattle.</p><br /> <p>McDaneld TG, Kuehn LA, Thomas MG, Snelling WM, Smith TP, Pollak EJ, Cole JB, Keele JW. J Anim Sci. 2014 May;92(5):1945-57.</p><br /> <p>Genomic evaluation, breed identification, and discovery of a haplotype affecting fertility for Ayrshire dairy cattle.</p><br /> <p>Cooper TA, Wiggans GR, Null DJ, Hutchison JL, Cole JB. J Dairy Sci. 2014;97(6):3878-82.</p><br /> <p>Bovine exome sequence analysis and targeted SNP genotyping of recessive fertility defects BH1, HH2, and HH3 reveal a putative causative mutation in SMC2 for HH3.</p><br /> <p>McClure MC, Bickhart D, Null D, Vanraden P, Xu L, Wiggans G, Liu G, Schroeder S, Glasscock J, Armstrong J, Cole JB, Van Tassell CP, Sonstegard TS. PLoS One. 2014 Mar 25;9(3): e92769.</p><br /> <p>Short communication: Use of young bulls in the United States.</p><br /> <p>Hutchison JL, Cole JB, Bickhart DM. J Dairy Sci. 2014 May;97(5):3213-20.</p><br /> <p>A genome-wide association study of calf birth weight in Holstein cattle using single nucleotide polymorphisms and phenotypes predicted from auxiliary traits.</p><br /> <p>Cole JB, Waurich B, Wensch-Dorendorf M, Bickhart DM, Swalve HH. J Dairy Sci. 2014 May;97(5):3156-72</p><br /> <p>Increasing long-term response by selecting for favorable minor alleles.</p><br /> <p>Sun C, VanRaden PM. PLoS One. 2014 Feb 5;9(2): e88510.</p><br /> <p>Predicting male fertility in dairy cattle using markers with large effect and functional annotation data.</p><br /> <p>Nani JP, Rezende FM, Peñagaricano F. BMC Genomics. 2019 Apr 2;20(1):258.</p><br /> <p>Genomic prediction of bull fertility in US Jersey dairy cattle.</p><br /> <p>Rezende FM, Nani JP, Peñagaricano F. J Dairy Sci. 2019 Apr;102(4):3230-3240.</p><br /> <p>Gene Mapping and Gene-Set Analysis for Milk Fever Incidence in Holstein Dairy Cattle.</p><br /> <p>Pacheco HA, da Silva S, Sigdel A, Mak CK, Galvão KN, Texeira RA, Dias LT, Peñagaricano F. Front Genet. 2018 Oct 10; 9:465.</p><br /> <p>In Utero Heat Stress Alters the Offspring Epigenome.</p><br /> <p>Skibiel AL, Peñagaricano F, Amorín R, Ahmed BM, Dahl GE, Laporta J. Sci Rep. 2018 Oct 2;8(1):14609.</p><br /> <p>Epidemiologic and economic analyses of pregnancy loss attributable to mastitis in primiparous Holstein cows.</p><br /> <p>Dahl MO, De Vries A, Maunsell FP, Galvao KN, Risco CA, Hernandez JA. J Dairy Sci. 2018 Nov;101(11):10142-10150.</p><br /> <p>Genetic dissection of bull fertility in US Jersey dairy cattle.</p><br /> <p>Rezende FM, Dietsch GO, Peñagaricano F. Anim Genet. 2018 Oct;49(5):393-402.</p><br /> <p>RNA-Seq reveals novel genes and pathways involved in bovine mammary involution during the dry period and under environmental heat stress.</p><br /> <p>Dado-Senn B, Skibiel AL, Fabris TF, Zhang Y, Dahl GE, Peñagaricano F, Laporta J. Sci Rep. 2018 Jul 23;8(1):11096.</p><br /> <p>Whole-genome scan reveals significant non-additive effects for sire conception rate in Holstein cattle.</p><br /> <p>Nicolini P, Amorín R, Han Y, Peñagaricano F. BMC Genet. 2018 Feb 27;19(1):14.</p><br /> <p>Economic and genetic performance of various combinations of in vitro-produced embryo transfers and artificial insemination in a dairy herd.</p><br /> <p>Kaniyamattam K, Block J, Hansen PJ, De Vries A. J Dairy Sci. 2018 Feb;101(2):1540-1553.</p><br /> <p>Impact of applying sex sorted semen on the selection proportion of the sire of dams selection pathway in a nucleus program.</p><br /> <p>Joezy-Shekalgorabi S, De Vries A. Asian-Australas J Anim Sci. 2018 Sep;31(9):1387-1392.</p><br /> <p>Predicting bull fertility using genomic data and biological information.</p><br /> <p>Abdollahi-Arpanahi R, Morota G, Peñagaricano F. J Dairy Sci. 2017 Dec;100(12):9656-9666.</p><br /> <p>Evidence that mastitis can cause pregnancy loss in dairy cows: A systematic review of observational studies.</p><br /> <p>Dahl MO, Maunsell FP, De Vries A, Galvao KN, Risco CA, Hernandez JA. J Dairy Sci. 2017 Oct;100(10):8322-8329.</p><br /> <p>Season of conception is associated with future survival, fertility, and milk yield of Holstein cows.</p><br /> <p>Pinedo PJ, De Vries A. J Dairy Sci. 2017 Aug;100(8):6631-6639.</p><br /> <p>Comparison between an exclusive in vitro-produced embryo transfer system and artificial insemination for genetic, technical, and financial herd performance.</p><br /> <p>Kaniyamattam K, Block J, Hansen PJ, De Vries A. J Dairy Sci. 2017 Jul;100(7):5729-5745.</p><br /> <p>Economic trade-offs between genetic improvement and longevity in dairy cattle.</p><br /> <p>De Vries A. J Dairy Sci. 2017 May;100(5):4184-4192.</p><br /> <p>Unravelling the genomic architecture of bull fertility in Holstein cattle.</p><br /> <p>Han Y, Peñagaricano F. BMC Genet. 2016 Nov 14;17(1):143.</p><br /> <p>Economic feasibility of cooling dry cows across the United States.</p><br /> <p>Ferreira FC, Gennari RS, Dahl GE, De Vries A. J Dairy Sci. 2016 Dec;99(12):9931-9941.</p><br /> <p>Stochastic dynamic simulation modeling including multi-trait genetics to estimate genetic, technical, and financial consequences of dairy farm reproduction and selection strategies.</p><br /> <p>Kaniyamattam K, Elzo MA, Cole JB, De Vries A. J Dairy Sci. 2016 Oct;99(10):8187-8202.</p><br /> <p>Bayesian integration of sensor information and a multivariate dynamic linear model for prediction of dairy cow mastitis.</p><br /> <p>Jensen DB, Hogeveen H, De Vries A. J Dairy Sci. 2016 Sep;99(9):7344-7361.</p><br /> <p>Genome-wide association mapping and pathway analysis of leukosis incidence in a US Holstein cattle population.</p><br /> <p>Abdalla EA, Peñagaricano F, Byrem TM, Weigel KA, Rosa GJ. Anim Genet. 2016 Aug;47(4):395-407.</p><br /> <p>Economic evaluation of stall stocking density of lactating dairy cows.</p><br /> <p>De Vries A, Dechassa H, Hogeveen H. J Dairy Sci. 2016 May;99(5):3848-3857.</p><br /> <p>Effects of season and herd milk volume on somatic cell counts of Florida dairy farms.</p><br /> <p>Ferreira FC, De Vries A. J Dairy Sci. 2015 Jun;98(6):4182-97.</p><br /> <p>A study of methods for evaluating the success of the transition period in early-lactation dairy cows.</p><br /> <p>Lukas JM, Reneau JK, Wallace RL, De Vries A. J Dairy Sci. 2015 Jan;98(1):250-62.</p><br /> <p>Effect of delayed breeding during the summer on profitability of dairy cows.</p><br /> <p>Gobikrushanth M, De Vries A, Santos JE, Risco CA, Galvão KN. J Dairy Sci. 2014 Jul;97(7):4236-46.</p><br /> <p>Estimation of regional genetic parameters for mortality and 305-d milk yield of US Holsteins in the first 3 parities.</p><br /> <p>Tokuhisa K, Tsuruta S, De Vries A, Bertrand JK, Misztal I. J Dairy Sci. 2014 Jul;97(7):4497-502.</p><br /> <p>Agreement between milk fat, protein, and lactose observations collected from the Dairy Herd Improvement Association (DHIA) and a real-time milk analyzer.</p><br /> <p>Kaniyamattam K, De Vries A. J Dairy Sci. 2014 May;97(5):2896-908.</p><br /> <p>Dynamics of culling for Jersey, Holstein, and Jersey × Holstein crossbred cows in large multibreed dairy herds.</p><br /> <p>Pinedo PJ, Daniels A, Shumaker J, De Vries A. J Dairy Sci. 2014 May;97(5):2886-95.</p><br /> <p>International bull evaluations by genomic BLUP with a prediction population.</p><br /> <p>Fragomeni B, Masuda Y, Bradford HL, Lourenco DAL, Misztal I. J Dairy Sci. 2019 Mar;102(3):2330-2335.</p><br /> <p>Application of single-step genomic evaluation using multiple-trait random regression test-day models in dairy cattle.</p><br /> <p>Oliveira HR, Lourenco DAL, Masuda Y, Misztal I, Tsuruta S, Jamrozik J, Brito LF, Silva FF, Schenkel FS. J Dairy Sci. 2019 Mar;102(3):2365-2377.</p><br /> <p>Genetics and genomics of reproductive disorders in Canadian Holstein cattle.</p><br /> <p>Guarini AR, Lourenco DAL, Brito LF, Sargolzaei M, Baes CF, Miglior F, Misztal I, Schenkel FS. J Dairy Sci. 2019 Feb;102(2):1341-1353.</p><br /> <p>Bias in heritability estimates from genomic restricted maximum likelihood methods under different genotyping strategies.</p><br /> <p>Cesarani A, Pocrnic I, Macciotta NPP, Fragomeni BO, Misztal I, Lourenco DAL. J Anim Breed Genet. 2019 Jan;136(1):40-50.</p><br /> <p>Comparison of genomic predictions for lowly heritable traits using multi-step and single-step genomic best linear unbiased predictor in Holstein cattle.</p><br /> <p>Guarini AR, Lourenco DAL, Brito LF, Sargolzaei M, Baes CF, Miglior F, Misztal I, Schenkel FS.</p><br /> <p>J Dairy Sci. 2018 Sep;101(9):8076-8086.</p><br /> <p>Incorporation of causative quantitative trait nucleotides in single-step GBLUP.</p><br /> <p>Fragomeni BO, Lourenco DAL, Masuda Y, Legarra A, Misztal I. Genet Sel Evol. 2017 Jul 26;49(1):59.</p><br /> <p>Genomic analysis of cow mortality and milk production using a threshold-linear model.</p><br /> <p>Tsuruta S, Lourenco DAL, Misztal I, Lawlor TJ. J Dairy Sci. 2017 Sep;100(9):7295-7305.</p><br /> <p>Selection of core animals in the Algorithm for Proven and Young using a simulation model.</p><br /> <p>Bradford HL, Pocrnić I, Fragomeni BO, Lourenco DAL, Misztal I.</p><br /> <p>J Anim Breed Genet. 2017 Dec;134(6):545-552.</p><br /> <p>Accuracy of breeding values in small genotyped populations using different sources of external information-A simulation study.</p><br /> <p>Andonov S, Lourenco DAL, Fragomeni BO, Masuda Y, Pocrnic I, Tsuruta S, Misztal I. J Dairy Sci. 2017 Jan;100(1):395-401.</p><br /> <p>Metafounders are related to Fst fixation indices and reduce bias in single-step genomic evaluations.</p><br /> <p>Garcia-Baccino CA, Legarra A, Christensen OF, Misztal I, Pocrnic I, Vitezica ZG, Cantet RJ. Genet Sel Evol. 2017 Mar 10;49(1):34.</p><br /> <p>Invited review: efficient computation strategies in genomic selection.</p><br /> <p>Misztal I, Legarra A. Animal. 2017 May;11(5):731-736.</p><br /> <p>Dimensionality of genomic information and performance of the Algorithm for Proven and Young for different livestock species.</p><br /> <p>Pocrnic I, Lourenco DA, Masuda Y, Misztal I. Genet Sel Evol. 2016 Oct 31;48(1):82.</p><br /> <p>Weighting Strategies for Single-Step Genomic BLUP: An Iterative Approach for Accurate Calculation of GEBV and GWAS.</p><br /> <p>Zhang X, Lourenco D, Aguilar I, Legarra A, Misztal I. Front Genet. 2016 Aug 19; 7:151.</p><br /> <p>Is genomic selection now a mature technology?</p><br /> <p>Misztal I. J Anim Breed Genet. 2016 Apr;133(2):81-2.</p><br /> <p>The Dimensionality of Genomic Information and Its Effect on Genomic Prediction.</p><br /> <p>Pocrnic I, Lourenco DA, Masuda Y, Legarra A, Misztal I. Genetics. 2016 May;203(1):573-81.</p><br /> <p>Implementation of genomic recursions in single-step genomic best linear unbiased predictor for US Holsteins with a large number of genotyped animals.</p><br /> <p>Masuda Y, Misztal I, Tsuruta S, Legarra A, Aguilar I, Lourenco DAL, Fragomeni BO, Lawlor TJ. J Dairy Sci. 2016 Mar;99(3):1968-1974.</p><br /> <p>Inexpensive Computation of the Inverse of the Genomic Relationship Matrix in Populations with Small Effective Population Size.</p><br /> <p>Misztal I. Genetics. 2016 Feb;202(2):401-9.</p><br /> <p>Genotype by environment interactions on culling rates and 305-day milk yield of Holstein cows in 3 US regions.</p><br /> <p>Tsuruta S, Lourenco DA, Misztal I, Lawlor TJ. J Dairy Sci. 2015 Aug;98(8):5796-805.</p><br /> <p>Ancestral Relationships Using Metafounders: Finite Ancestral Populations and Across Population Relationships.</p><br /> <p>Legarra A, Christensen OF, Vitezica ZG, Aguilar I, Misztal I. Genetics. 2015 Jun;200(2):455-68.</p><br /> <p>Hot topic: Use of genomic recursions in single-step genomic best linear unbiased predictor (BLUP) with a large number of genotypes.</p><br /> <p>Fragomeni BO, Lourenco DA, Tsuruta S, Masuda Y, Aguilar I, Legarra A, Lawlor TJ, Misztal I. J Dairy Sci. 2015 Jun;98(6):4090-4.</p><br /> <p>Use of genomic recursions and algorithm for proven and young animals for single-step genomic BLUP analyses--a simulation study.</p><br /> <p>Fragomeni BO, Lourenco DA, Tsuruta S, Masuda Y, Aguilar I, Misztal I. J Anim Breed Genet. 2015 Oct;132(5):340-5.</p><br /> <p>Assigning unknown parent groups to reduce bias in genomic evaluations of final score in US Holsteins.</p><br /> <p>Tsuruta S, Misztal I, Lourenco DA, Lawlor TJ. J Dairy Sci. 2014 Sep;97(9):5814-21.</p><br /> <p>Estimation of regional genetic parameters for mortality and 305-d milk yield of US Holsteins in the first 3 parities.</p><br /> <p>Tokuhisa K, Tsuruta S, De Vries A, Bertrand JK, Misztal I. J Dairy Sci. 2014 Jul;97(7):4497-502.</p><br /> <p>Using recursion to compute the inverse of the genomic relationship matrix.</p><br /> <p>Misztal I, Legarra A, Aguilar I. J Dairy Sci. 2014;97(6):3943-52.</p><br /> <p>Are evaluations on young genotyped animals benefiting from the past generations?</p><br /> <p>Lourenco DA, Misztal I, Tsuruta S, Aguilar I, Lawlor TJ, Forni S, Weller JI. J Dairy Sci. 2014;97(6):3930-42.</p><br /> <p>Methods for genomic evaluation of a relatively small genotyped dairy population and effect of genotyped cow information in multiparity analyses.</p><br /> <p>Lourenco DA, Misztal I, Tsuruta S, Aguilar I, Ezra E, Ron M, Shirak A, Weller JI. J Dairy Sci. 2014 Mar;97(3):1742-52.</p><br /> <p>Annual rhythms of milk and milk fat and protein production in dairy cattle in the United States.</p><br /> <p>Salfer IJ, Dechow CD, Harvatine KJ. J Dairy Sci. 2019 Jan;102(1):742-753.</p><br /> <p>DNA methylation patterns in peripheral blood mononuclear cells from Holstein cattle with variable milk yield.</p><br /> <p>Dechow CD, Liu WS. BMC Genomics. 2018 Oct 11;19(1):744.</p><br /> <p>Invited review: Learning from the future - A vision for dairy farms and cows in 2067.</p><br /> <p>Britt JH, Cushman RA, Dechow CD, Dobson H, Humblot P, Hutjens MF, Jones GA, Ruegg PS, Sheldon IM, Stevenson JS. J Dairy Sci. 2018 May;101(5):3722-3741.</p><br /> <p>Short communication: Genetic lag represents commercial herd genetic merit more accurately than the 4-path selection model.</p><br /> <p>Dechow CD, Rogers GW. J Dairy Sci. 2018 May;101(5):4312-4316.</p><br /> <p>Short communication: Two dominant paternal lineages for North American Jersey artificial insemination sires.</p><br /> <p>Dechow CD, Liu WS, Idun JS, Maness B. J Dairy Sci. 2018 Mar;101(3):2281-2284.</p><br /> <p>Genetic parameters for yield, fitness, and type traits in US Brown Swiss dairy cattle.</p><br /> <p>Gibson KD, Dechow CD. J Dairy Sci. 2018 Feb;101(2):1251-1257.</p><br /> <p>Blood plasma traits associated with genetic merit for feed utilization in Holstein cows.</p><br /> <p>Dechow CD, Baumrucker CR, Bruckmaier RM, Blum JW. J Dairy Sci. 2017 Oct;100(10):8232-8238.</p><br /> <p>Mammary immunoglobulin transfer rates following prepartum milking.</p><br /> <p>Baumrucker CR, Dechow CD, Macrina AL, Gross JJ, Bruckmaier RM. J Dairy Sci. 2016 Nov;99(11):9254-9262.</p><br /> <p>Association of calf growth traits with production characteristics in dairy cattle.</p><br /> <p>Van De Stroet DL, Calderón Díaz JA, Stalder KJ, Heinrichs AJ, Dechow CD. J Dairy Sci. 2016 Oct;99(10):8347-8355.</p><br /> <p>Quarter variation and correlations of colostrum albumin, immunoglobulin G1 and G2 in dairy cows.</p><br /> <p>Samarütel J, Baumrucker CR, Gross JJ, Dechow CD, Bruckmaier RM. J Dairy Res. 2016 May;83(2):209-18.</p><br /> <p>Within-milking variation in milk composition and fatty acid profile of Holstein dairy cows.</p><br /> <p>Rico DE, Marshall ER, Choi J, Kaylegian KE, Dechow CD, Harvatine KJ.</p><br /> <p>J Dairy Sci. 2014 Jul;97(7):4259-68.</p>Impact Statements
- The major impacts of this Multistate Research Coordinating Committee and Information Exchange Group are be summarized as follows: (i) development and implementation of national genetic evaluations for new traits, (ii) development of an updated Lifetime Net Merit (NM$) formula and related economic selection indexes, (iii) better understanding of the genetic control of both novel and economically important traits, and (iv) the confirmation that crossbreeding is an attractive option for commercial dairy producers.
Date of Annual Report: 02/17/2020
Report Information
Period the Report Covers: 10/01/2018 - 09/30/2019
Participants
De Vries, Albert, devries@ufl.edu, University of FloridaMisztal, Ignacy, ignacy@uga.edu, University of Georgia
Ma, Li, lima@umd.edu, University of Maryland
Lopez-Villalobos, Nicolas, N.Lopez-Villalobos@massey.ac.nz, Massey University, New Zealand
Taxis, Tasia, taxistas@msu.edu, Michigan State University
Heins, Bradley, hein0106@umn.edu, University of Minnesota
Huson, Heather J, hjh3@cornell.edu, Cornell University
Dechow, Chad, cdd1@psu.edu, Pennsylvania State University
Cockrum, Rebecca R, rcockrum@vt.edu, Virginia Tech
Cole, John, john.cole@ars.usda.gov, USDA-ARS
Peñagaricano, Francisco, fpenagaricano@ufl.edu, University of Florida
Weigel, Kent, kweigel@wisc.edu, University of Wisconsin
Students: Glenda Pereira, Isaac Hagen, Allison Herrick, Asha Miles, Lydia Hardie, Yutaka Masuda, Yvette Steyn
Brief Summary of Minutes
AGENDA
Tuesday, October 29 – Station reports, Tour and discussion with Hershey executives, presentation by Isaac Hagen and Hershey staff
Wednesday, October 30 – Station reports, presentation by Nicolas Lopez-Villalobos, farm tours (Wanner’s Pride and Joy farm, Warwick Manor Farm)
Thursday, October 31 – Business meeting
Business Meeting
Discussions
- Potential transition from coordinating committee to multi-state project
- Review NIMSS project website
- Report by Lakshmi Matukumalli from NIFA
- Discussion on next years meeting in New Zealand
- Joe West will be retiring
Resolutions
- Albert DeVries was nominated as the new secretary
- Christian Maltecca was nominated as the new chair
- The next meeting will be held October 25-29, 2020 in New Zealand
Submitted by Brad Heins, Chair and acting secretary
Accomplishments
<p><strong>Objective 1:</strong> Recommend breeding strategies for optimal use of breed resources, maintenance and (or) exploitation of within-breed (additive and non-additive) genetic variation</p><br /> <ul><br /> <li>Comparison of pure Holstein with Montbéliarde and Viking Red crossbreds (Leslie Hansen and Bradley Heins, University of Minnesota)</li><br /> <li>Comparison of pure Holstein with Jersey, Montbéliarde, Normande and Viking Red crossbreds (Bradley Heins, University of Minnesota)</li><br /> <li>Evaluation of crossbreeding in organic dairy farms (Bradley Heins, University of Minnesota; Chad Dechow, Pennsylvania State University)</li><br /> <li>Genomic predictions for crossbred dairy cattle (Paul VanRaden, USDA-ARS; Bradley Heins, University of Minnesota; Chad Dechow, Pennsylvania State University)</li><br /> </ul><br /> <p><strong>Objective 2: </strong>Capture phenotypic data for novel and economically important traits to elucidate their genetic regulation and potential for genomic selection</p><br /> <ul><br /> <li>Genetic analysis of feed efficiency (Kent Weigel, University of Wisconsin; Paul VanRaden, USDA)</li><br /> <li>Identification of genetic mechanisms underlying mastitis resistance and relationship to mammary microbiota (Heather Huson, Cornell University)</li><br /> <li>Genetic analysis of digital cushion thickness in Holstein and Jersey breeds (Heather Huson, Cornell University)</li><br /> <li>Effects of dietary components on gene expression and rumen microbiome in preweaned dairy heifers (Rebecca Cockrum, Virginia Tech)</li><br /> <li>Genetic analysis of bovine respiratory disease (Kent Weigel, University of Wisconsin)</li><br /> <li>Elucidating the genetic basis and mechanism of complex diseases and traits (Li Ma, University of Maryland)</li><br /> <li>Genomic prediction for milk-production and feed-efficiency traits within North American dairy herds (Kent Weigel, University of Wisconsin)</li><br /> </ul><br /> <p><strong>Objective 3:</strong> Collaborate with the National Animal Germplasm Program (NAGP) Dairy Committee, Animal Genomics and Improvement Laboratory (AGIL), and the Council on Dairy Cattle Breeding (CDCB) to improve genetic variation of dairy and optimize economic merit indices</p><br /> <ul><br /> <li>Genomic evaluations using single-step GBLUP (Ignacy Misztal, University of Georgia)</li><br /> <li>Recovery of a lost Holstein male lineage (Chad Dechow, Pennsylvania State University)</li><br /> <li>Genetic dissection of dairy bull fertility: from fine mapping to genomic prediction (Francisco Peñagaricano, University of Florida)</li><br /> <li>New reference map ARS-UCD1 replaced UMD3 and 80,000 instead of 60,000 markers and QTLs used in genomic predictions since December 2018 (Paul VanRaden, USDA-ARS)</li><br /> <li>Early first calving (EFC) as a new trait for selection. (Paul VanRaden, USDA-ARS)</li><br /> <li>Revision of Net Merit $ to include feed intake, early first calving, heifer livability, increased body weight maintenance cost, and reduced value of productive life due to faster genetic trend (Paul VanRaden, USDA-ARS)</li><br /> </ul><br /> <p><strong>Objective 4:</strong> Develop variant discovery strategies to incorporate functional –omics data into breeding schemes for economically important traits</p><br /> <ul><br /> <li>To review historic and current genetic diversity in the populations with particular interest in inbreeding levels and signatures of selection (Li Ma, University of Maryland)</li><br /> <li>Developing statistical approaches and computing tools to boost the power of current and next-generation sequencing-based genetic studies (Li Ma, University of Maryland)</li><br /> </ul><br /> <p><strong>Objective 5:</strong> Carry out interdisciplinary collaborations to improve dairy cow and calf health through increased partnerships with researchers, industry and stakeholders</p><br /> <ul><br /> <li>The crossbred cattle project is a collaboration among 3 multistate groups (NY, MN, and PA) as well as a commercial AI company in New Zealand.</li><br /> <li>Lactation curves of Crossbred and Holstein Diary cattle (MN and NZ)</li><br /> </ul>Publications
<p>Abdalla, E.A., F.B. Lopes, T.M. Byrem, K.A. Weigel, and G.J.M. Rosa. 2019. Genomic prediction of bovine leukosis incidence in a US Holstein population. Livestock Science 225:73–77. doi:10.1016/j.livsci.2019.05.004. </p><br /> <p>Abdollahi-Arpanahi, R., M.R. Carvalho, E.S. Ribeiro, and F. Peñagaricano. 2019. Association of lipid-related genes implicated in conceptus elongation with female fertility traits in dairy cattle. Journal of Dairy Science 102:10020–10029. doi:10.3168/jds.2019-17068.</p><br /> <p>Bradford, H. L., Y. Masuda, J. B. Cole, I. Misztal, and P. M. VanRaden. 2019. Modeling pedigree accuracy and uncertain parentage in single-step genomic evaluations of simulated and US Holstein datasets. J. Dairy Sci. 102:2308–2318. https://doi.org/10.3168/jds.2018-15419</p><br /> <p>Bradford, H. L., Y. Masuda, P. M. VanRaden, A. Legarra, I. Misztal. 2019. Modeling missing pedigree in single-step genomic BLUP. J. Dairy Sci. 102:2336–2346. <a href="https://doi.org/10.3168/jds.2018-15434">https://doi.org/10.3168/jds.2018-15434</a></p><br /> <p>Carvalho, M.R., F. Peñagaricano, J.E.P. Santos, T.J. DeVries, B.W. McBride, and E.S. Ribeiro. 2019. Long-term effects of postpartum clinical disease on milk production, reproduction, and culling of dairy cows. Journal of Dairy Science 102:11701–11717. doi:10.3168/jds.2019-17025.</p><br /> <p>Cole, J.B. and Null, D.J. Short communication: Phenotypic and genetic effects of the polled haplotype on yield, longevity, and fertility in US Brown Swiss, Holstein, and Jersey cattle. J. Dairy Sci. 102 (9):8247-8250. 2019.</p><br /> <p>Cole. J.B., and VanRaden, P.M. <em>Symposium review:</em> Possibilities in an age of genomics: The future of selection indices. J. Dairy Sci. 101(4):3686–3701. 2018.</p><br /> <p>Costa, A., N. Lopez-Villalobos, N.W. Sneddon, L. Shalloo, M. Franzoi, M. De Marchi, and M. Penasa. 2019. Invited review: Milk lactose—Current status and future challenges in dairy cattle. Journal of Dairy Science 102:5883–5898. doi:10.3168/jds.2018-15955.</p><br /> <p>Guarini, A. R., D. A. L. Lourenco, L. F. Brito, M. Sargolzaei, C. F. Baes, F. Miglior, I. Misztal, and F. S. Schenkel. 2019. Genetics and genomics of reproductive disorders in Canadian Holstein cattle. J. Dairy Sci. 102:1341-1353. https://doi.org/10.3168/jds.2018-15038</p><br /> <p>Guarini, A. R., D. A. L. Lourenco, L. F. Brito, M. Sargolzaei, C. F. Baes, F. Miglior, S. Tsuruta, I. Misztal, and F. S. Schenkel. 2019. Use of a single-step approach for integrating foreign information into national genomic evaluation in Holstein cattle. J. Dairy Sci. 102:8175-8183. https://doi.org/10.3168/jds.2018-15819</p><br /> <p>Guarini, A. R., M. Sargolzaei, L. F. Brito, V. Kroezen, D. A. L. Lourenco, C. F. Baes, F. Miglior, J. B. Cole, and F. S. Schenkel. 2019. Estimating the effect of the deleterious recessive haplotypes AH1 and AH2 on reproduction performance of Ayrshire cattle. J. Dairy Sci. 102:5315-5322. <a href="https://doi.org/10.3168/jds.2018-15366">https://doi.org/10.3168/jds.2018-15366</a></p><br /> <p>Handcock, R.C., N. Lopez-Villalobos, L.R. McNaughton, P.J. Back, G.R. Edwards, and R.E. Hickson. 2019. Positive relationships between body weight of dairy heifers and their first-lactation and accumulated three-parity lactation production. Journal of Dairy Science 102:4577–4589. doi:10.3168/jds.2018-15229.</p><br /> <p>Jiang, J., Ma, L., Prakapenka, D., VanRaden, P.M., Cole, J.B., and Da, Y. A large-scale genome-wide association study in U.S. Holstein cattle. Front. Genet. 10:412. 2019.</p><br /> <p>Li, B., L. Fang, D.J. Null, J. Hutchison, E. Connor, P.M. VanRaden, M. Vandehaar, R. Tempelman, and K.A. Weigel. 2019. High-density genome-wide association study for residual feed intake in Holstein dairy cattle. Journal of Dairy Science 102. doi:10.3168/jds.2019-16645.</p><br /> <p>Ma, L., Sonstegard, T.S., Cole, J.B., Van Tassell, C.P., Wiggans, G.R., Crooker, B.A., Tan, C., Prakapenka, D., Liu, G.E., and Da, Y. Genome changes due to artificial selection in U.S. Holstein cattle. BMC Genomics 20:128. 2019.</p><br /> <p>Ma, L., Sonstegard, T.S., Cole, J.B. et al. Genome changes due to artificial selection in U.S. Holstein cattle. BMC Genomics 20, 128 (2019). <a href="https://doi.org/10.1186/s12864-019-5459-x">https://doi.org/10.1186/s12864-019-5459-x</a></p><br /> <p>Ma, L., J.B. Cole, Y. Da, and P.M. VanRaden. 2019. Symposium review: Genetics, genome-wide association study, and genetic improvement of dairy fertility traits. Journal of Dairy Science 102:3735–3743. doi:10.3168/jds.2018-15269.</p><br /> <p>Miles, A.M., McArt, J.A.A., Leal Yepes, F.A., Stambuk, C.R., Virkler, P.D., Huson, H.J., (2018) Udder and teat conformational risk factors for elevated somatic cell count and clinical mastitis in New York Holsteins. Preventative Veterinary Medicine epub Dec 15; Feb 163:7-13.</p><br /> <p>Mueller, M.L., Cole, J.B., Sonstegard, T.S., and Van Eenennaamn, A.L. Comparison of gene editing versus conventional breeding to introgress the <em>POLLED</em> allele into the US dairy cattle population. J. Dairy Sci. 102(5):4215–4226. 2019.</p><br /> <p>Nani, J.P., Rezende, F.M. & Peñagaricano, F. Predicting male fertility in dairy cattle using markers with large effect and functional annotation data. BMC Genomics 20, 258 (2019). https://doi.org/10.1186/s12864-019-5644-y</p><br /> <p>Oliveira, H. R., L. F. Brito, D. A. L. Lourenco, F. F. Silva, J. Jamrozik, L. R. Schaeffer, and F. S. Schenkel. 2019. Invited review: Advances and applications of random regression models: From quantitative genetics to genomics. J. Dairy Sci. 102:7664-7683. https://doi.org/10.3168/jds.2019-16265</p><br /> <p>Oliveira, H. R., L. F. Brito, M. Sargolzaei, F. F. Silva, J. Jamrozik, D. A. L. Lourenco, and F. S. Schenkel. 2019. Impact of including information from bulls and their daughters in the training population of multiple‐step genomic evaluations in dairy cattle: a simulation study. J. Anim. Breed. Genet. In Press. https://doi.org/10.1111/jbg.12407</p><br /> <p>Oliveira H. R., L. F. Brito, F. F. Silva, D. A. L. Lourenco, J. Jamrozik, and F. S. Schenkel. 2019. Genomic prediction of lactation curves for milk, fat, protein, and somatic cell score in Holstein cattle. J. Dairy Sci. 102:452-463. https://doi.org/10.3168/jds.2018-15159</p><br /> <p>Oliveira, H. R., D. A. L. Lourenco, Y. Masuda, I. Misztal, S. Tsuruta, J. Jamrozik, L. F. Brito, F. F. Silva, and F. S. Schenkel. 2019. Application of single-step genomic evaluation using multiple-trait random regression test-day models in dairy cattle. J. Dairy Sci. 102:2365–2377. https://doi.org/10.3168/jds.2018-15466</p><br /> <p>Oliveira, H. R., D. A. L. Lourenco, Y. Masuda, I. Misztal, S. Tsuruta, J. Jamrozik, L. F. Brito, F. F. Silva, J. P. Cant, and F. S. Schenkel. 2019. Single-step genome-wide association for longitudinal traits of Canadian Ayrshire, Holstein, and Jersey dairy cattle. J. Dairy Sci. In Press. https://doi.org/10.3168/jds.2019-16821</p><br /> <p>Oliveira, H. R., J. P. Cant, L. F. Brito, F. L. B. Feitosa, T. C. S. Chud, P. A. S. Fonseca, J. Jamrozik, F. F. Silva, D. A. L. Lourenco, and F. S. Schenkel. 2019. Genome-wide association for milk production traits and somatic cell score in different lactation stages of Ayrshire, Holstein, and Jersey dairy cattle. J. Dairy Sci. 102:8159-8174. <a href="https://doi.org/10.3168/jds.2019-16451">https://doi.org/10.3168/jds.2019-16451</a></p><br /> <p>Pacheco, H.A., S. da Silva, A. Sigdel, C.K. Mak, K.N. Galvão, R.A. Texeira, L.T. Dias, and F. Peñagaricano. 2018. Gene Mapping and Gene-Set Analysis for Milk Fever Incidence in Holstein Dairy Cattle. Frontiers in Genetics 9:465. doi:10.3389/fgene.2018.00465.</p><br /> <p>Pereira, G.M., and B.J. Heins. 2019. Activity and rumination of Holstein and crossbred cows in an organic grazing and low-input conventional dairy herd. Translational Animal Science 3:txz106. <a href="https://doi.org/10.1093/tas/txz106">https://doi.org/10.1093/tas/txz106</a></p><br /> <p>Rezende, F.M., J.P. Nani, and F. Peñagaricano. 2019. Genomic prediction of bull fertility in US Jersey dairy cattle. Journal of Dairy Science 102:3230–3240. doi:10.3168/jds.2018-15810.</p><br /> <p>Santos, D.J., Cole, J.B., Lawlor, T.J., VanRaden, P.V., Tonhati, H., and Ma, L. Variance of gametic diversity and its use in selection programs. J. Dairy Sci. 102(6):5279-5294. 2019.</p><br /> <p>Schmitt, M.R., VanRaden, P.M., and De Vries, A. Ranking sires using genetic selection indices based on financial investment methods versus lifetime net merit. J. Dairy Sci. 102(10):9060–9075. 2019.</p><br /> <p>Shonka-Martin, B., A. Hazel, B. Heins, and L.B. Hansen. 2019a. Three-breed rotational crossbreds of Montbéliarde, Viking Red, and Holstein compared with Holstein cows for dry matter intake, body traits, and production. Journal of dairy science 102:871–882. https://doi.org/10.3168/jds.2018-15318</p><br /> <p>Shonka-Martin, B., B.J. Heins, and L.B. Hansen. 2019b. Three-breed rotational crossbreds of Montbéliarde, Viking Red, and Holstein compared with Holstein cows for feed efficiency, income over feed cost, and residual feed intake. Journal of dairy science 102:3661–3673. <a href="https://doi.org/10.3168/jds.2018-15682">https://doi.org/10.3168/jds.2018-15682</a></p><br /> <p>Sigdel, A., R. Abdollahi-Arpanahi, I. Aguilar, and F. Peñagaricano. 2019. Whole Genome Mapping Reveals Novel Genes and Pathways Involved in Milk Production Under Heat Stress in US Holstein Cows. Frontiers in Genetics 10:928. doi:10.3389/fgene.2019.00928.</p><br /> <p>Stambuk, C.R., McArt, J.A.A., Bicalho, R.C., Miles, A.M., Huson, H.J., (2018) A longitudinal study of digital cushion thickness and its function as a predictor for compromised locomotion and hoof lesions in Holstein cows. Translational Animal Science epub Sept 27, 2018; Jan 3:1:9</p><br /> <p>VanRaden, P.M., Bickhart, D.M., and O'Connell, J.R. Calling known variants and identifying new variants while rapidly aligning sequence data. J. Dairy Sci. 102(4):3216–3229. 2019.</p><br /> <p>Veronese, A., O. Marques, R. Moreira, A.L. Belli, R.S. Bisinotto, T.R. Bilby, F. Peñagaricano, and R.C. Chebel. 2019a. Genomic merit for reproductive traits. I: Estrous characteristics and fertility in Holstein heifers. Journal of Dairy Science 102:6624–6638. doi:10.3168/jds.2018-15205.</p><br /> <p>Veronese, A., O. Marques, F. Peñagaricano, R.S. Bisinotto, K.G. Pohler, T.R. Bilby, and R.C. Chebel. 2019b. Genomic merit for reproductive traits. II: Physiological responses of Holstein heifers. Journal of Dairy Science 102:6639–6648. doi:10.3168/jds.2018-15245.</p><br /> <p>Williams, K.T., K.A. Weigel, W.K. Coblentz, N.M. Esser, H. Schlesser, P.C. Hoffman, H. Su, and M.S. Akins. 2019. Effect of diet energy density and genomic residual feed intake on prebred dairy heifer feed efficiency, growth, and manure excretion. Journal of Dairy Science 102:4041–4050. doi:10.3168/jds.2018-15504.</p>Impact Statements
- The major impacts of this SCC84 group are be summarized as follows: (i) development and implementation of national genetic evaluations for new traits, (ii) development of crossbreeding rotations for dairy farm profitability and feed efficiency, (iii) better understanding of the genetic control of health traits for calves and cows, (iv) improvement in genomic evaluation for future traits, and (v) training of graduate student in dairy cattle genetics.
Date of Annual Report: 01/27/2021
Report Information
Period the Report Covers: 10/01/2020 - 09/30/2021
Participants
De Vries, Albert, devries@ufl.edu, University of FloridaMa, Li, lima@umd.edu, University of Maryland
Lopez-Villalobos, Nicolas, N.Lopez-Villalobos@massey.ac.nz, Massey University, New Zealand
Taxis, Tasia, taxistas@msu.edu, Michigan State University
Heins, Bradley, hein0106@umn.edu, University of Minnesota
Huson, Heather J, hjh3@cornell.edu, Cornell University
Dechow, Chad, cdd1@psu.edu, Pennsylvania State University
Cockrum, Rebecca R, rcockrum@vt.edu, Virginia Tech
Cole, John, john.cole@ars.usda.gov, USDA-ARS
Peñagaricano, Francisco, fpenagaricano@ufl.edu, University of Florida
Weigel, Kent, kweigel@wisc.edu, University of Wisconsin
Hansen, Les, hanse009@umn.edu, University of Minnesota
Godfrey, Bob, rgodfre@uvi.edu, University of the Virgin Islands, Administrative Advisor
Students: Yvette Steyn
Brief Summary of Minutes
AGENDA
Thursday, November 12 –
20 minutes: Welcome, introductions
20 minutes: Update from USDA-NIFA and administrative advisor.
10 minutes: Discussion – 2021 Annual Meeting.
10 minutes: Election of new SCC84 secretary.
10 minutes: Other business
Business Meeting
Discussions
- Bob Godfrey, New Administrative Advisor
- Lakshmi Matukumalli – Primary NIFA contact
- Frank Siewerdt – Secondary NIFA contact
- Review NIMSS project website
- Report by Debora Hamernik from NIFA
- Brad, Chad, Albert, Heather and Nicolas for committee for prepare for USDA NIFA conference session
- Discussion on next years meeting in Michigan
- The next meeting will be held Michigan in 2021
Submitted by Brad Heins, Chair and acting secretary
Accomplishments
<p><strong>Objective 1:</strong> Recommend breeding strategies for optimal use of breed resources, maintenance and(or) exploitation of within-breed (additive and non-additive) genetic variation</p><br /> <ul><br /> <li>Comparison of pure Holstein with Montbéliarde and Viking Red crossbreds (Leslie Hansen and Bradley Heins, University of Minnesota)</li><br /> <li>Comparison of pure Holstein with Jersey, Montbéliarde, Normande and Viking Red crossbreds (Bradley Heins, University of Minnesota)</li><br /> <li>Evaluation of crossbreeding in organic dairy farms (Bradley Heins, University of Minnesota; Chad Dechow, Pennsylvania State University)</li><br /> <li>Genomic predictions for crossbred dairy cattle (Paul VanRaden, USDA-ARS; Bradley Heins, University of Minnesota; Chad Dechow, Pennsylvania State University)</li><br /> <li>Genetic studies have been performed for digital cushion thickness comparing variation within and across the Holstein and Jersey breeds and between sexes (Heather Huson, Cornell, Brad Heins, University of Minnesota)</li><br /> <li>Genetic studies have been performed for mastitis related traits including udder and teat morphology and somatic cell counts across lactation (Heather Huson, Cornell)</li><br /> <li>A comparison of Jersey cattle from Jersey Island (Heather Huson, Cornell)</li><br /> <li>Breeds and within cows for colostrum production (Rebecca Cockrum, Virginia Tech)</li><br /> </ul><br /> <p><strong>Objective 2: </strong>Capture phenotypic data for novel and economically important traits to elucidate their genetic regulation and potential for genomic selection</p><br /> <ul><br /> <li>Genetic analysis of feed efficiency (Kent Weigel and</li><br /> <li>Francisco Penagaricano, University of Wisconsin; Paul VanRaden, USDA)</li><br /> <li>Identification of the genetic mechanisms underlying mastitis resistance/susceptibility in dairy cattle and relationship to mammary microbiota (Heather Huson, Cornell University)</li><br /> <li>Reducing dairy cattle lameness with improved genetic understanding and selection for digital cushion thickness (Heather Huson, Cornell University)</li><br /> <li>Effects of dietary components on gene expression and rumen microbiome in preweaned dairy heifers (Rebecca Cockrum, Virginia Tech)</li><br /> <li>Predicting sperm production of young dairy bulls using collection history and management factors (Kent Weigel and Francisco Penagaricano , University of Wisconsin)</li><br /> <li>Prediction of feed intake and residual feed intake in mid-lactation dairy cows using descriptive, performance, behavioral, and blood metabolite data (Francisco Penagaricano, University of Wisconsin).</li><br /> <li>Elucidating the genetic basis and mechanism of complex diseases and traits (Li Ma, University of Maryland)</li><br /> <li>Genomic prediction for milk-production and feed-efficiency traits within North American dairy herds (Kent Weigel, University of Wisconsin)</li><br /> </ul><br /> <p><strong>Objective 3:</strong> Collaborate with the National Animal Germplasm Program (NAGP) Dairy Committee, Animal Genomics and Improvement Laboratory (AGIL), and the Council on Dairy Cattle Breeding (CDCB) to improve genetic variation of dairy and optimize economic merit indices</p><br /> <ul><br /> <li>Reconstitution and modernization of lost Holstein male lineages using samples from a gene bank. (Chad Dechow, Pennsylvania State University)</li><br /> <li>Genetic dissection of dairy bull fertility: from fine mapping to genomic prediction (Francisco Peñagaricano, University of Florida)</li><br /> <li>Early first calving (EFC) as a new trait for selection. (Paul VanRaden, USDA-ARS)</li><br /> <li>Revision of Net Merit $ to include feed intake, early first calving, heifer livability, increased body weight maintenance cost, and reduced value of productive life due to faster genetic trend (Paul VanRaden, USDA-ARS)</li><br /> </ul><br /> <p> </p><br /> <p><strong>Objective 4:</strong> Develop variant discovery strategies to incorporate functional –omics data into breeding schemes for economically important traits</p><br /> <ul><br /> <li>To review historic and current genetic diversity in the populations with particular interest in inbreeding levels and signatures of selection (Li Ma, University of Maryland)</li><br /> <li>Developing statistical approaches and computing tools to boost the power of current and next-generation sequencing-based genetic studies (Li Ma, University of Maryland)</li><br /> <li>Admixture and population structure analysis to examine the effect of ancestry and breeding effects (Heather Huson, Cornell; Bradley Heins, University of Minnesota)</li><br /> </ul><br /> <p><strong>Objective 5:</strong> Carry out interdisciplinary collaborations to improve dairy cow and calf health through increased partnerships with researchers, industry and stakeholders</p><br /> <ul><br /> <li>The crossbred cattle project is a collaboration among 3 multistate groups (NY, MN, and PA) as well as a commercial AI company in New Zealand.</li><br /> <li>Lactation curves of Crossbred and Holstein Diary cattle (MN and NZ)</li><br /> <li>Mastitis and lameness projects (NY and MN)</li><br /> </ul><br /> <p> </p><br /> <p> </p><br /> <p><strong>Objective 6:</strong> Create a pipeline of diverse graduate students in the fields of quantitative and functional genetics and bioinformatics via outreach and educational opportunities</p><br /> <ul><br /> <li>Graduate research opportunities (NY, MN, VT, WI, PA, MI)</li><br /> <li>Undergraduate research opportunities (NY, MN, VT, WI, PA)</li><br /> <li>Teaching collaboration with Neogen-Geneseek (NY)</li><br /> </ul>Publications
<ol><br /> <li>Carvalho MR, C Aboujaoude, F Peñagaricano, JEP Santos, TJ DeVries, BW McBride, and ES Ribeiro (2020) Associations between maternal characteristics and health, survival, and performance of dairy heifers from birth through to first lactation. Journal of Dairy Science 103:823-839.</li><br /> <li>Cole, J.B., Eaglen, A.E., Maltecca, C., Mulder, H.A., and Pryce, J.E. The future of phenomics in dairy cattle breeding. Anim. Front. 10(2):37–44. 2020.</li><br /> <li>Cousillas-Boam, G., W.J. Weber, A. Benjamin, S. Kahl, B.J. Heins, T. Elsasser, D. Kerr, and B.A. Crooker. 2020. Effect of Holstein genotype on innate immune and metabolic responses of heifers to lipopolysaccharide (LPS) administration. Domestic Animal Endocrinology 70:106374. https://doi.org/10.1016/j.domaniend.2019.07.002</li><br /> <li>Dechow, C.D., W.S. Liu, L.W. Specht, and H. Blackburn. 2020. Reconstitution and modernization of lost Holstein male lineages using samples from a gene bank. Journal of Dairy Science 103:4510–4516. doi:10.3168/jds.2019-17753.</li><br /> <li>Dechow, C.D., K.S. Sondericker, A.A. Enab, and L.C. Hardie. 2020b. Genetic, farm, and lactation effects on behavior and performance of US Holsteins in automated milking systems. Journal of Dairy Science 103:11503–11514. doi:10.3168/jds.2020-18786.</li><br /> <li> Freebern, E., D.J.A. Santos, L. Fang, J. Jiang, K.L. Parker Gaddis, G.E. Liu, P.M. VanRaden, C. Maltecca, J.B. Cole, and L. Ma. 2020a. GWAS and fine-mapping of livability and six disease traits in Holstein cattle. BMC Genomics 21:41. doi:10.1186/s12864-020-6461-z.</li><br /> <li> Freebern, E., D.J.A. Santos, L. Fang, J. Jiang, K.L. Parker Gaddis, G.E. Liu, P.M. VanRaden, C. Maltecca, J.B. Cole, and L. Ma. 2020b. GWAS and fine-mapping of livability and six disease traits in Holstein cattle. BMC Genomics 21:41. doi:10.1186/s12864-020-6461-z.</li><br /> <li> Gross N, F Peñagaricano, and H Khatib (2020) Integration of whole-genome DNA methylation data with RNA sequencing data to identify markers for bull fertility. Animal Genetics 51: 502-510.</li><br /> <li> Handcock, R.C., N. Lopez-Villalobos, L.R. McNaughton, P.J. Back, G.R. Edwards, and R.E. Hickson. 2020. Body weight of dairy heifers is positively associated with reproduction and stayability. Journal of Dairy Science 103:4466–4474. doi:10.3168/jds.2019-17545.</li><br /> <li> Hazel, A., B. Heins, and L. Hansen. 2020. Fertility and 305-day production of Viking Red-, Montbéliarde-, and Holstein-sired crossbred cows compared with Holstein cows during their first 3 lactations in Minnesota dairy herds. J. Dairy Sci. 103:8683–8697. https://doi.org/10.3168/jds.2020-18196</li><br /> <li>Hazel, A., B. Heins, and L. Hansen. 2020. Health treatment cost, stillbirth, survival, and conformation of Viking Red-, Montbéliarde-, and Holstein-sired crossbred cows compared with pure Holstein cows during their first 3 lactations. J. Dairy Sci. 103:10917–10939. https://doi.org/10.3168/jds.2020-18604</li><br /> <li> Hurst, T.S., N. Lopez-Villalobos, and J.P. Boerman. 2021. Predictive equations for early-life indicators of future body weight in Holstein dairy heifers. Journal of Dairy Science 104:736–749. doi:10.3168/jds.2020-18560</li><br /> <li><strong> </strong>Huson, H.J.*, Sonstegard, T.S., Godfrey, J., Hambrook, D., Wolfe, C., Wiggans, G., Blackburn, H., Van Tassell, C.P.*, (2020) A genetic investigation of Island Jersey Cattle, the foundation of the Jersey breed. <em>Frontiers in Genetics</em>, 17 April 2020 <a href="https://doi.org/10.3389/fgene.2020.00366">https://doi.org/10.3389/fgene.2020.00366</a></li><br /> <li> Leal Yepes, F.A.†, Nydam, D.V., Mann, S., Caixeta, L., McArt, J.A.A., Overton, T.R., Wakshlag, J.J., Huson, H.J.*, 2019 Longitudinal phenotypes improve genotype association for hyperketonemia in dairy cattle. <em>Animals</em> Dec 1, 2019, 9(12), 1059, https://doi.org/10.3390/ani9121059</li><br /> <li>Li, B., VanRaden, P.M., Guduk, E., O'Connell, J.R., Null, D.J., Connor, E.E., VandeHaar, M.J., Tempelman, R.J., Weigel, K.A., and Cole, J.B. <a href="https://aipl.arsusda.gov/publish/jds/2020/JDS_103_2477-2486_LiEtAl.pdf">Genomic prediction of residual feed intake in US Holstein dairy cattle</a>. J. Dairy Sci. 103(3):2477–2486. 2020. </li><br /> <li>Lima FS, FT Silvestre, F Peñagaricano, and WW Thatcher (2020) Early genomic prediction of daughter pregnancy rate is associated with improved reproductive performance in Holstein dairy cows. Journal of Dairy Science 103:3312-3324.</li><br /> <li> Lacey, E.K., K.J. Harvatine, and C.D. Dechow. 2020. Short communication: Diet digestibility measured from fecal samples and associations with phenotypic and genetic merit for milk yield and composition. Journal of Dairy Science 103:5270–5274. doi:10.3168/jds.2019-17450.</li><br /> <li> Lopez-Villalobos, N., R.J. Spelman, J. Melis, S.R. Davis, S.D. Berry, K. Lehnert, N.W. Sneddon, S.E. Holroyd, A.K. MacGibbon, and R.G. Snell. 2020. Genetic correlations of milk fatty acid contents predicted from milk mid-infrared spectra in New Zealand dairy cattle. Journal of Dairy Science 103:7238–7248. doi:10.3168/jds.2019-17971.</li><br /> <li> Makanjuola, B.O., C. Maltecca, F. Miglior, F.S. Schenkel, and C.F. Baes. 2020a. Effect of recent and ancient inbreeding on production and fertility traits in Canadian Holsteins. BMC Genomics 21:605. doi:10.1186/s12864-020-07031-w.</li><br /> <li> Makanjuola, B.O., F. Miglior, E.A. Abdalla, C. Maltecca, F.S. Schenkel, and C.F. Baes. 2020b. Effect of genomic selection on rate of inbreeding and coancestry and effective population size of Holstein and Jersey cattle populations. Journal of Dairy Science 103:5183–5199. doi:10.3168/jds.2019-18013.</li><br /> <li> Maltecca, C., F. Tiezzi, J.B. Cole, and C. Baes. 2020. Symposium review: Exploiting homozygosity in the era of genomics—Selection, inbreeding, and mating programs. Journal of Dairy Science 103:5302–5313. doi:10.3168/jds.2019-17846.</li><br /> <li> McWhorter, T.M., Hutchison, J.L., Norman, H.D., Cole, J.B., Fok, G.C., Lourenco, D.A.L., and VanRaden, P.M. <a href="https://aipl.arsusda.gov/publish/jds/2020/JDS_103_10374-10382_McWhorterEtAl.pdf">Investigating conception rate for beef service sires bred to dairy cows and heifers</a>. J. Dairy Sci. 103(11):10374–10382. 2020. </li><br /> <li>Miglior, F., C. Baes, D. Lourenco, F. Penagaricano, and B. Heins. 2020. Introduction: ADSA and Interbull Joint Breeding and Genetics Symposia. J. Dairy Sci. 103:5275–5277. https://doi.org/10.3168/jds.2020-18666</li><br /> <li> Miles, A.M. and Huson, H.J., (2020) Graduate student literature review: Understanding the genetics underlying mastitis. <em>Journal of Dairy Science </em>6 November. https://doi.org/10.3168/jds.2020-18297</li><br /> <li> Miles, A.M. and Huson, H.J., (2020) Time- and population dependent genetic patterns underlie bovine milk somatic cell count. <em>Journal of Dairy Science </em>103(9): 1-13, 1 July. https://doi.org/10.3168/jds.2020-18322</li><br /> <li><em> </em>Nani JP and F Peñagaricano (2020) Whole-genome homozygosity mapping reveals candidate regions affecting bull fertility in US Holstein cattle. BMC Genomics 21:338.</li><br /> <li>Nani, J.P., Bacheller, L.R., Cole, J.B., and VanRaden, P.M. <a href="https://aipl.arsusda.gov/publish/jds/2020/JDS_103_1729-1734_NaniEtAl.pdf">Discovering ancestors and connecting relatives in large genomic databases</a>. J. Dairy Sci. 103(2):1729–1734. 2020.</li><br /> <li>HA Pacheco, FM Rezende, and F Peñagaricano (2020) Gene mapping and genomic prediction of bull fertility using sex chromosome markers. Journal of Dairy Science 103:3304-3311.</li><br /> <li>Parker Gaddis, K.L., VanRaden, P.M., Cole, J.B., Norman, H.D., Nicolazzi, E., and Dürr, J.W. <a href="https://aipl.arsusda.gov/publish/jds/2020/JDS_103_5354-5365_ParkerGaddisEtAl.pdf"><em>Symposium review:</em> Development, implementation, and perspectives of health evaluations in the United States</a> J. Dairy Sci. 103(6):5354–5365. 2020.</li><br /> <li>Pralle RS, NE Schultz, HM White, and KA Weigel (2020) Hyperketonemia GWAS and parity dependent SNP associations in Holstein dairy cows intensively sampled for blood β-hydroxybutyrate concentration. Physiological Genomics 52(8):347-357.</li><br /> <li>Quick AE, TL Ollivett, BW Kirkpatrick, and KA Weigel (2020) Genomic analysis of bovine respiratory disease and lung consolidation in pre-weaned Holstein calves using clinical scoring and lung ultrasound. Journal of Dairy Science 103:1632-1641.</li><br /> <li>Rezende FM, M Haile-Mariam, JE Pryce, and F Peñagaricano (2020) Across-country genomic prediction of bull fertility in Jersey dairy cattle. Journal of Dairy Science 103:11618-11627.</li><br /> <li>Sigdel A, L Liu, R Abdollahi-Arpanahi, I Aguilar, and F Peñagaricano (2020) Genetic dissection of reproductive performance of dairy cows under heat stress. Animal Genetics 51:511–520.</li><br /> <li>Stambuk, C., E. Staiger, B. Heins, and H. Huson. 2020. Exploring physiological and genetic variation of digital cushion thickness in Holstein and Jersey cows and bulls. J. Dairy Sci. 103:9177–9194. https://doi.org/10.3168/jds.2020-18290</li><br /> <li>Stambuk, C.R., E.A. Staiger, A. Nazari-Ghadikolaei, B.J. Heins, and H.J. Huson. 2020. Phenotypic characterization and genome-wide association studies of digital cushion thickness in Holstein cows. J. Dairy Sci. 103:3289–3303. https://doi.org/10.3168/jds.2019-17409</li><br /> <li>Uddin EM, O Santana, KA Weigel, and MA Wattiaux (2020) Enteric methane, lactation performance, digestibility, and metabolism of nitrogen and energy of Holsteins and Jerseys fed 2 levels of forage fiber from alfalfa silage or corn silage. Journal of Dairy Science 103:6087-6099.</li><br /> <li>VanRaden, P.M., Tooker, M.E., Chud, T.C.S., Norman, H.D., Megonigal, Jr., J.H., Haagen, I.W., and Wiggans, G.R. <a href="https://aipl.arsusda.gov/publish/jds/2020/JDS_103_1620-1631_VanRadenEtAl.pdf">Genomic predictions for crossbred dairy cattle</a>. J. Dairy Sci. 103(2):1620–1631. 2020.</li><br /> <li>VanRaden, P.M. <a href="https://aipl.arsusda.gov/publish/jds/2020/JDS_103_5291-5301_VanRaden.pdf"><em>Symposium review:</em> How to implement genomic selection</a>. J. Dairy Sci. 103(6):5291–5301. 2020. </li><br /> <li>Whitt, C.E.†, Tauer, L., Huson, H.J., (2019) Bull efficiency using dairy genetic traits. <em>PLOS ONE</em> Nov 11, 2019; 14:11 e0223436. https://doi.org/10.1371/journal.pone.0223436</li><br /> </ol>Impact Statements
- The major impacts of this SCC84 group are be summarized as follows: (i) development and implementation of national genetic evaluations for new traits, (ii) development of crossbreeding rotations for dairy farm profitability and feed efficiency, (iii) better understanding of the genetic control of health traits for calves and cows, (iv) improvement in genomic evaluation for future traits, and (v) training of graduate student in dairy cattle genetics. Collaborated with the National Animal Germplasm Program (NAGP) Dairy Committee, Animal Genomics and Improvement Laboratory (AGIL), and the Council on Dairy Cattle Breeding (CDCB) to improve genetic variation of dairy and optimize economic merit indices
Date of Annual Report: 02/15/2021
Report Information
Period the Report Covers: 10/01/2020 - 09/30/2021
Participants
Participants:De Vries, Albert, devries@ufl.edu, University of Florida Ma,
Li, lima@umd.edu, University of Maryland,
Tasia, Kendrick taxistas@msu.edu, Michigan State University,
Heins, Bradley, hein0106@umn.edu, University of Minnesota
Huson, Heather J, hjh3@cornell.edu, Cornell University
Cockrum, Rebecca R, rcockrum@vt.edu, Virginia Tech
VanRaden, Paul, paul.vanraden@ars.usda.gov, USDA-ARS
Miles, Ashs, asha.miles@ars.usda.gov, USDA-ARS
Peñagaricano, Francisco, fpenagarican@wisc.edu, UW Madison
Weigel, Kent, kweigel@wisc.edu, University of Wisconsin
Hansen, Les, hanse009@umn.edu, University of Minnesota
Maltecca, Christian, cmaltec@ncsu, North Carolina State University
Jiang, Jicai, jjiang26@ncsu.edu, North Carolina State University
Brito, Luiz, britol@purdue.edu, Purdue University
Misztal, Ignacy, ignacy@uga.eduL, University of Georgia
Lourenco, Daniela, danilino@uga.edu, University of Georgia
Cole, John, URUS
John Arthington
Brief Summary of Minutes
Brief Summary of Minutes of Annual Meeting AGENDA
November 20 –
10 minutes: Welcome, introductions
15 minutes: Update from USDA-NIFA and administrative advisor.
Business Meeting
Report by Frank Siewerdt from NIFA
Albert Devries elected chair for the 2022 meeting
The next meeting will be held in Michigan in 2022
Discussion on next year’s meeting in Michigan
Election of new SCC84 secretary will happen at the beginning of the next meeting (2022)
Station Reports
Submitted by Christian Maltecca, Chair and acting secretary
Accomplishments
<p><strong>Accomplishments</strong></p><br /> <p> </p><br /> <p><strong>Objective 1:</strong> Recommend breeding strategies for optimal use of breed resources, maintenance and(or) exploitation of within-breed (additive and non-additive) genetic variation</p><br /> <p> </p><br /> <ul><br /> <li>Analyzing data on genome variation in crossbred cattle from three different types of crossbreeding programs (rotational crossbreeding, creation of a composite breed, and F1 generation hybrid with backcrossing to ancestry breed). <strong>(Cornell)</strong></li><br /> <li>Comparison of crossbreds of Holstein, Jersey, Montbéliarde, Normande and Viking Red to Holsteins for feed efficiency fed two different diets. <strong>(UofM, PennState)</strong></li><br /> <li>Determine milk, fat, protein production and SCS, fertility, and survival for beta casein A2 genotypes of organic Holstein cows. <strong>(UofM, PennState)</strong></li><br /> <li>Fatty acid profiles of Holstein, Grazecross and ProCROSS cows in an organic and conventional dairy herd <strong>(UofM)</strong></li><br /> <li>Estimation and Management of Inbreeding and Inbreeding Depression in North American Cattle under Genomic Selection <strong>(NCSU)</strong></li><br /> <li>Current state of inbreeding, genetic diversity, and selective histories in 5 breeds of U.S. Dairy Cattle <strong>(NCSU)</strong></li><br /> </ul><br /> <p> </p><br /> <p> </p><br /> <p><strong>Objective 2:</strong> Capture phenotypic data for novel and economically important traits to elucidate their genetic regulation and potential for genomic selection</p><br /> <p> </p><br /> <ul><br /> <li>Signatures of selection for thermal stress <strong>(Purdue)</strong></li><br /> <li>Milking temperament in Holstein cattle <strong>(Purdue)</strong></li><br /> <li>Livestock behavioral genomics <strong>(Purdue)</strong></li><br /> <li>Purdue Animal Sciences Research Data Ecosystem <strong>(Purdue)</strong></li><br /> <li>Functional longevity in Holstein based on RRM <strong>(Purdue)</strong></li><br /> <li>Calf health (including disease transmission ability in pens) <strong>(Purdue)</strong></li><br /> <li>Lactation persistency and extended lactations <strong>(Purdue)</strong></li><br /> <li>Analysis of milk microbiota using 16SrRNA sequence data – This project will assess correlations between milk microbiota and milk components and production or health parameters. It will also establish normal variation of milk microbiota within cow across lactation and across cows. <strong>(Cornell)</strong></li><br /> <li>Inheritance of DNA methylation patterns and influence of breeding strategies and reproductive technologies – A new project is being developed to explore this concept. It will include correlation of performance traits with DNA methylation and inheritance patterns. <strong>(Cornell)</strong></li><br /> <li>Circulating metabolites indicate differences in high and low residual feed intake Holstein dairy cows. <strong>(UW)</strong></li><br /> <li>Predicting daily milk yield for primiparous cows using data of within herd relatives to capture genotype-by-environment interactions. <strong>(UW).</strong></li><br /> <li>Effect of diet energy level and genomic residual feed intake on bred Holstein dairy heifer growth and feed efficiency. <strong>(UW)</strong>.</li><br /> <li>Gene mapping, gene-set analysis, and genomic prediction of postpartum blood calcium in Holstein cows. <strong>(UW)</strong></li><br /> <li>Comparison of methods to predict feed intake and residual feed intake using behavioral and metabolite data in addition to classical performance variables. <strong>(UW)</strong></li><br /> <li>Comparison of single-breed and multi-breed training populations for infrared predictions of novel phenotypes in Holstein cows <strong>(UW)</strong></li><br /> <li>Evaluation of bull fertility in Italian Brown Swiss dairy cattle using cow field data. <strong>(UW)</strong></li><br /> <li>Assessment of the relationship between postpartum health and mid-lactation performance, behavior, and feed efficiency in Holstein dairy cows. <strong>(UW)</strong></li><br /> <li>Evaluating the performance of machine learning methods and variable selection methods for predicting difficult-to-measure traits in Holstein dairy cattle using milk infrared spectral data. <strong>(UW)</strong></li><br /> <li>Integrating genomic and infrared spectral data improves the prediction of milk protein</li><br /> <li>composition in dairy cattle. <strong>(UW)</strong></li><br /> <li>Assessing feed efficiency in early and mid-lactation and its associations with performance and health in Holstein <strong>(UW)</strong></li><br /> <li></li><br /> <li>Response to ad libitum milk allowance of Holstein and crossbred dairy and dairy-beef calves in an automated feeding system <strong>(UofM)</strong></li><br /> <li>Optimization of multi-period planning problems <strong>(UFL)</strong></li><br /> </ul><br /> <p>Update dynamic programming model </p><br /> <ul><br /> <li>Extend with more traits, including multiple service sires</li><br /> <li>Separate current from future lactations </li><br /> <li>Calculate insemination values </li><br /> </ul><br /> <p>Improve prediction of fertility with data from precision dairy farming sensors and machine learning methods </p><br /> <ul><br /> <li>Embrio Transfer and fertility evaluations <strong>(USDA AGIL)</strong></li><br /> <li>Flexible Testing and Milk-Only Records <strong>(USDA AGIL)</strong></li><br /> </ul><br /> <p> </p><br /> <p> </p><br /> <p> </p><br /> <p><strong>Objective 3:</strong> Collaborate with the National Animal Germplasm Program (NAGP) Dairy Committee, Animal Genomics and Improvement Laboratory (AGIL), and the Council on Dairy Cattle Breeding (CDCB) to improve genetic variation of dairy and optimize economic merit indices.</p><br /> <p> </p><br /> <ul><br /> <li>Evaluation of Jersey breed and NAGP repository sample collection of Jersey breed – a collaborative project with the USDA’s – NAGP and AGIL departments, American Jersey Association, and Cornell University is comparing the NAGP sample collection representing Jersey cattle and conducting a general overview of the breed’s inbreeding, admixture, and adaptation. This project uses genotypes obtained from the CDCB. <strong>(Cornell)</strong></li><br /> <li>National single-step evaluation of Holsteins and all dairy breeds <strong>(UGA)</strong></li><br /> <li>Multibreed ssGBLUP evaluations <strong>(UGA)</strong></li><br /> <li>Flexible Testing and Milk-Only Records <strong>(USDA AGIL)</strong></li><br /> <li>International Bull Rankings <strong>(USDA AGIL)</strong></li><br /> <li>Prediction Factors for Yield Traits <strong>(USDA AGIL)</strong></li><br /> <li>Develop Hoof Health Data Pipeline <strong>(USDA AGIL, UofM)</strong></li><br /> <li>Genetic evaluations of stillbirth for five US dairy breeds: a data-resource feasibility study <strong>(UW)</strong></li><br /> </ul><br /> <p> </p><br /> <p><strong>Objective 4:</strong> Develop variant discovery strategies to incorporate functional –omics data into breeding schemes for economically important traits</p><br /> <p> </p><br /> <ul><br /> <li>Genomic analysis of visceral fat accumulation in Holstein cows. <strong>(UW)</strong></li><br /> <li>Effect of natural pre-luteolytic prostaglandin F2α pulses on the bovine luteal transcriptome during spontaneous luteal regression <strong>(UW)</strong></li><br /> <li>Genes and pathways associated with pregnancy loss in dairy cattle <strong>(UW)</strong></li><br /> <li>Targeted sequencing revealscandidate causal variants for dairy bull subfertility <strong>(UW)</strong></li><br /> <li>Histological and transcriptomic analysis of adipose and muscle of dairy calves supplemented with 5-hydroxytryptophan. <strong>(UW)</strong></li><br /> <li>My research design for multiple projects incorporate intensive phenotypic characterization of traits directly on the animals being genotyped and used in the genomic association studies. My team has conducted multiple longitudinal field trials to evaluate phenotypic variation over time and better understand biological mechanisms. <strong>(Cornell)</strong></li><br /> <li>Crossbred dairy cattle- A new crossbred cattle evaluation is ongoing and uses admixture and population structure analysis to examine the effect of ancestry and breeding effects on crossbred cattle performance and future selection. <strong>(Cornell)</strong></li><br /> <li>Genomic Partitioning of inbreeding by age and functional annotation in U.S. Dairy Cattle <strong>(NCSU)</strong></li><br /> <li>Two new software tools for large-scale genetic analyses <strong>(NCSU)</strong><br /> <ul><br /> <li>SSGP: million-scale mixed-model associations</li><br /> <li>MPH: partitioning heritability into many components in related individuals</li><br /> </ul><br /> </li><br /> </ul><br /> <p><strong>Objective 5: </strong>Carry out interdisciplinary collaborations to improve dairy cow and calf health through increased partnerships with researchers, industry, and stakeholders </p><br /> <p> </p><br /> <p> </p><br /> <p> </p><br /> <p><strong>Objective 6:</strong> Create a pipeline of diverse graduate students in the fields of quantitative and functional genetics and bioinformatics via outreach and educational opportunities</p><br /> <p> </p><br /> <p>Graduate research opportunities (NY, MN, VT, WI, PA, MI, NCSU)</p><br /> <p>Undergraduate research opportunities (NY, MN,</p>Publications
<p><strong>Publications</strong></p><br /> <p> </p><br /> <ol><br /> <li>Landaeta-Hernández*, A.J., Zambrano-Nava, S., Verde, O., Pinto-Santini, L., Montero-Urdaneta, M., Hernández-Fonseca, J.P., Fuenmayor-Morales, C., Sonstegard, T.S., Huson, H.J.,</li><br /> <li>Olson, T.A., (2021) Heat stress response in slick vs normal-haired Criollo Limonero heifers. Tropical Animal Health and Production 53, 445. https://doi.org/10.1007/s11250-021-02856-3</li><br /> <li>Miles, A.M. †, Posbergh, C.J. †, Huson, H.J., (2021) Direct Phenotyping and Principal Component Analysis of Type Traits Implicate Novel QTL in Bovine Mastitis through Genome-Wide Association. Animals April 11(4), 1147. <a href="https://doi.org/10.3390/ani11041147">https://doi.org/10.3390/ani11041147</a></li><br /> <li>Basiel, B.L., L.C. Hardie, B.J. Heins+, C.D. Dechow. 2021. Genetic parameters and genomic regions associated with horn fly resistance in organic Holstein cattle. J. Dairy Sci. 104:12724-12740. https://doi.org/10.3168/jds.2021-20366</li><br /> <li>Haagen, I.W., L.C. Hardie, B.J. Heins, and C.D. Dechow. 2021. Genetic parameters of passivetransfer of immunity for US organic Holstein calves. J. Dairy Sci. 104:2018–2026.https://doi.org/10.3168/jds.2020-19080</li><br /> <li>Haagen, I.W. L.C. Hardie, B.J. Heins, C.D. Dechow. 2021. Genetic parameters of calf</li><br /> </ol><br /> <p>morbidity and stayability for US organic Holstein calves J. Dairy Sci. 104: 11770-11778.</p><br /> <p>https://doi.org/10.3168/jds.2021-20432</p><br /> <ol start="7"><br /> <li>Hardie, L., B. Heins, and C. Dechow. 2021. Genetic parameters for stayability of Holsteins in US organic herds. J. Dairy Sci. Volume 104, Issue 4, 4507 – 4515 https://doi.org/10.3168/jds.2020-19399</li><br /> <li>Hazel, A.R., B.J. Heins, and L.B. Hansen. 2021. Herd life, lifetime production, and profitability of Viking Red-sired and Montbéliarde-sired crossbred cows compared with their Holstein herdmates. J. Dairy Sci. Volume 104, Issue 3, 3261 – 3277 https://doi.org/10.3168/jds.2020-19137</li><br /> <li>WM Brown, MJ Martin, C Siberski, JE Koltes, F Peñagaricano, KA Weigel, and HM White (2021) Predicting dry matter intake in mid-lactation Holstein cows using point-in-time data streams available on dairy farms. Journal of Dairy Science (in review).</li><br /> <li>MJ Martin, RS Pralle, IR Bernstein, MJ VandeHaar, KA Weigel, Z Zhou, and HM White (2021)</li><br /> <li>Circulating metabolites indicate differences in high and low residual feed intake Holstein dairy cows. Metabolites (in review).</li><br /> <li>F Zhang, KA Weigel, and VE Cabrera (2021) Predicting daily milk yield for primiparous cows using data of within herd relatives to capture genotype-by-environment interactions. Journal of Dairy Science (in review).</li><br /> <li>K Williams, KA Weigel, W Coblentz, NM Esser, H Schlesser, PC Hoffman, R Ogden, H Su, and MSAkins (2021) Effect of diet energy level and genomic residual feed intake on bred Holstein dairy heifer growth and feed efficiency. Journal of Dairy Science (in press).</li><br /> </ol><br /> <p> </p><br /> <ol start="14"><br /> <li>L Cavani, MB Poindexter, CD Nelson, JEP Santos, and F Peñagaricano (2021) Gene mapping, gene-set analysis, and genomic prediction of postpartum blood calcium in Holstein cows. Journal of Dairy Science (in press)</li><br /> <li>MJ Martin, JRR Dorea, MR Borchers, RL Wallace, SJ Bertics, SK DeNise, KA Weigel, and HM White (2021) Comparison of methods to predict feed intake and residual feed intake using behaviora and metabolite data in addition to classical performance variables. Journal of Dairy Science 104:8765-8782.</li><br /> <li>LFM Mota, S Pegolo, T Baba, G Morota, F Peñagaricano, G Bittante, and A Cecchinato (2021) Comparison of single-breed and multi-breed training populations for infrared predictions of novel phenotypes in Holstein cows. Animals 11: 1993.</li><br /> <li>HA Pacheco, M Battagin, A Rossoni, A Cecchinato, and F Peñagaricano (2021) Evaluation of bull fertility in Italian Brown Swiss dairy cattle using cow field data. Journal of Dairy Science 104:10896-10904.</li><br /> <li>MJ Martin, KA Weigel, and HM White (2021). Assessment of the relationship between</li><br /> <li>postpartum health and mid-lactation performance, behavior, and feed efficiency in Holstein dairy cows. Animals 11, 1385.</li><br /> <li>LFM Mota, S Pegolo, T Baba, F Peñagaricano, G Morota, G Bittante, and A Cecchinato (2021) Evaluating the performance of machine learning methods and variable selection methods for predicting difficult-to-measure traits in Holstein dairy cattle using milk infrared spectral data. Journal of Dairy Science 104: 8107-8121.</li><br /> <li>T Baba, S Pegolo, LFM Mota, F Peñagaricano, G Bittante, A Cecchinato, and G Morota (2021) Integrating genomic and infrared spectral data improves the prediction of milk protein composition in dairy cattle. Genetics Selection Evolution 53: 29.</li><br /> <li>M Nehme Marinho, R Zimpel, F Peñagaricano, and JEP Santos (2021) Assessing feed efficiency in early and mid-lactation and its associations with performance and health in Holstein. Journal of Dairy Science 104: 5493-5507.</li><br /> <li>A Sigdel, XL Wu, K Parker Gaddis, HD Norman, JA Carrillo, J Burchard, F Peñagaricano and J Dürr (2021) Genetic evaluations of stillbirth for five US dairy breeds: a data-resource feasibility study. Frontiers in Genetics (in review).</li><br /> <li>LC Novo, L Cavani, P Pinedo, P Melendez, and F Peñagaricano (2021) Genomic analysis of visceral fat accumulation in Holstein cows. Frontiers in Genetics (in review).</li><br /> <li>MA Mezera, W Li, L Liu, R Meidan, F Peñagaricano, and MC Wiltbank (2021) Effect of natural pre-luteolytic prostaglandin F2α pulses on the bovine luteal transcriptome during spontaneous luteal regression. Biology of Reproduction 105: 1016-1029.</li><br /> <li>A Sigdel, RS Bisinotto, and F Peñagaricano (2021) Genes and pathways associated with pregnancy loss in dairy cattle. Scientific Reports 11: 13329.</li><br /> <li>R Abdollahi-Arpanahi, HA Pacheco, and F Peñagaricano (2021) Targeted sequencing reveals candidate causal variants for dairy bull subfertility. Animal Genetics 52: 509-513.</li><br /> <li>SL Field, MG Marrero, L Liu, F Peñagaricano, and J Laporta (2021) Histological and transcriptomic analysis of adipose and muscle of dairy calves supplemented with 5-hydroxytryptophan. Scientific Reports 11: 9665.</li><br /> <li>LF Brito, N Bedere, F Douhard, HR Oliveira, M Arnal, F Peñagaricano, AP Schinckel, CF Baes, and F Miglior (2021) Genetic selection of high-yielding dairy cattle towards sustainable farming systems in a rapidly changing world. Animal (in press) </li><br /> <li>AE Quick, J Meronek, K Amburn, K Rozeboom, and KA Weigel (2021) Predicting sperm production of young dairy bulls using collection history and management factors. Journal of Dairy Science 104:5817-5826. </li><br /> <li>B Shen, E Freebern, J Jiang, C Maltecca, JB Cole, GE Liu, L Ma Effect of Temperature and Maternal Age on Recombination Rate in Cattle Frontiers in Genetics, 1307</li><br /> <li>MC Fabbri, C Dadousis, F Tiezzi, C Maltecca, E Lozada-Soto, S Biffani.</li><br /> <li>Genetic diversity and population history of eight Italian beef cattle breeds using measures of autozygosity PloS one 16 (10), e0248087</li><br /> <li>BO Makanjuola, C Maltecca, F Miglior, G Marras, EA Abdalla, ... Identification of unique ROH regions with unfavorable effects on production and fertility traits in Canadian Holsteins Genetics selection evolution 53 (1), 1-11</li><br /> <li>LF Brito, N Bedere, F Douhard, HR Oliveira, M Arnal, F Peñagaricano, AP Schinckel, CF Baes, and F Miglior (2021) Genetic selection of high-yielding dairy cattle towards sustainable farming systems in a rapidly changing world. Animal (in press) </li><br /> <li>AE Quick, J Meronek, K Amburn, K Rozeboom, and KA Weigel (2021) Predicting sperm production of young dairy bulls using collection history and management factors. Journal of Dairy Science 104:5817-5826. </li><br /> </ol><br /> <p> </p><br /> <p> </p>Impact Statements
- (vi) Collaborated with the National Animal Germplasm Program (NAGP) Dairy Committee, Animal Genomics and Improvement Laboratory (AGIL), and the Council on Dairy Cattle Breeding (CDCB) to improve genetic variation of dairy and optimize economic merit indices
Date of Annual Report: 12/21/2022
Report Information
Period the Report Covers: 10/01/2021 - 09/30/2022
Participants
ParticipantsMembers:
Tasia Kendrick, Michigan State University, taxistas@msu.edu
Heather Huson, Cornell University, hjh3@cornell.edu
Luiz Brito, Purdue University, britol@purdue.edu
Ignacy Misztal, University of Georgia, ignacy@uga.edu
Albert De Vries, University of Florida, devries@ufl.edu
Bradley Heins, University of Minnesota WCROC, hein0106@umn.edu
Francisco Penagaricano, University of Wisconsin, shelley.prudom@wisc.edu
Kent Weigel, University of Wisconsin, kweigel@wisc.edu
Students/postdocs:
Samrawit Gebeyehu, University of Minnesota
Kathryn Bosley, University of Minnesota
Tanya Muratori, Penn State
Wasim Yousaf, Penn State
Emmanuel Lozada-Soto, North Carolina State University
Srikanth Krishnamoorthy, Cornell University
Azwan Jaafar, Cornell University
Sydney Jewell, Cornell University
Maddy Sokacz, Michigan State University
Ligia Cavani, UW Madison
Barbara Nascimento, UW Madison
Larissa Novo, UW Madison
Hendyel Pacheco, UW Madison
Fiona Louise Guinan, UW Madison
Barbara Mazetti Nascimento, UW Madison
Partners:
Sajjad Toghiani, USDA-ARS-AGIL, sajjad.toghiani@usda.gov
Ahmed Al-Khudhair, USDA-ARS-AGIL, ahmed.al-khudhair@usda.gov
Kristen Gaddis, CDCB, kristen.gaddis@uscdcb.com
Xiao-Lin (Nick) Wu, CDCB, nick.wu@uscdcb.com
Virtual reports:
Neal Schrick, Administrative Advisor, fschrick@utk.edu
Frank Siewerdt, USDA-NIFA, Frank.Siewerdt@USDA.gov
Brief Summary of Minutes
AGENDA
Monday, October 17, 2022
Meet at Kellogg Hotel and Conference Center Lobby, Michigan State University
Tour Sand Creek Dairy
Tour and Lunch at MooVille Creamery
Tour CentralStar Cooperative Diagnostic Laboratory
Return to Kellogg Hotel and Conference Center
Dinner at Harrison Roadhouse (networking with industry supporters)
Tuesday, October 18, 2022
Meet in private dining room in the Brody Café, Michigan State University
Administrative Advisor update
USDA-NIFA update
SCC-84 Project rewrite discussion
Station Reports
Lunch at Brody Square
Station Reports
Dinner at Lansing Brewing Company (networking with MSU affiliated individuals)
Wednesday, October 19, 2022
Meet in private dining room in the Brody Café, Michigan State University
Business meeting: 2023 host will be Heather Huson, Cornell University. Albert De Vries will repeat as chair. The group thanked Tasia Kendrick for the excellent organization of the meeting.
Station Reports
SCC-84 Project rewrite discussion: Brad Heins will lead the rewrite. Aim is a full multi-state project (S-1096)
Conference Concludes at noon
Submitted by Albert De Vries, Chair and acting secretary
Accomplishments
<p>Outcomes, outputs, activities and milestones reported by the following stations: </p><br /> <p><strong>Objective 1</strong>: Recommend breeding strategies for optimal use of breed resources, maintenance and(or) exploitation of within-breed (additive and non-additive) genetic variation</p><br /> <ul><br /> <li>Reasons for disposal and cull cow value of Holstein and crossbred dairy cattle (UMN)</li><br /> <li>Fatty Acids of Holsteins and Crossbred Cows (UMN)</li><br /> <li>RFI of Pre-Weaned Holstein Calves (UMN)</li><br /> <li>Development of insemination values to assist dairy producers making breeding decisions (UFL)</li><br /> <li>Managing Bovine Leukemia Virus by Integrating Surveillance of Youngstock and Monitoring of the Milking Herd (MSU)</li><br /> <li>Assessment of inbreeding and strategies to safeguard genetic diversity in US dairy cattle (NCSU)</li><br /> <li>Strategies for accuracy of imputation from 7K to 50K single nucleotide polymorphism chips in US crossbred dairy cattle (NCSU)</li><br /> </ul><br /> <p> </p><br /> <p><strong>Objective 2:</strong> Capture phenotypic data for novel and economically important traits to elucidate their genetic regulation and potential for genomic selection</p><br /> <ul><br /> <li>Milk microbiome analysis (Cornell)</li><br /> <li>The effect of selection on the genome architecture of crossbred dairy cattle (Cornell)</li><br /> <li>Local Ancestry Inference (Cornell)</li><br /> <li>Investigating epigenetic inheritance and associations with development, disease, and performance (Cornell)</li><br /> <li>Diet digestibility (PSU)</li><br /> <li>Organic calf growth (PSU)</li><br /> <li>Calf recumbency (PSU)</li><br /> <li>Beef x Dairy (PSU)</li><br /> <li>Telomere length (PSU)</li><br /> <li>Lost Y chromosome lineages (PSU)</li><br /> <li>Precision Dairy Research Data Ecosystem (Purdue)</li><br /> <li>Milkability and behavior traits in automated milking systems (Purdue)</li><br /> <li>Udder conformation traits based on XYZ coordinates from AMS (Purdue)</li><br /> <li>Modelling overall resilience in lactating cows (Purdue)</li><br /> <li>Meta-analysis of genetic parameters of resilience and efficiency traits (Purdue)</li><br /> <li>Novel traits from automatic milk feeders: calf feeding behavior and resilience (Purdue)</li><br /> <li>Feed efficiency in dairy cows (UW)</li><br /> <li>Investigating resilience indicators in U.S. dairy cattle (UW)</li><br /> <li>Feasibility study of genetic evaluation for Johne’s disease on US Holstein cows (UW)</li><br /> <li>Probability of survival in Jersey cattle (UW)</li><br /> <li>Genomic prediction of bull fertility in Italian Brown Swiss cattle (UW)</li><br /> <li>Genetic analysis of estrus expression in dairy cows (UW)</li><br /> </ul><br /> <p> </p><br /> <p><strong>Objective 3</strong>: Collaborate with the National Animal Germplasm Program (NAGP) Dairy Committee, Animal Genomics and Improvement Laboratory (AGIL), and the Council on Dairy Cattle Breeding (CDCB) to improve genetic variation of dairy and optimize economic merit indices.</p><br /> <ul><br /> <li>Genomic comparison between Jersey bulls in the National Animal Germplasm Program (NAGP) and Jersey bulls in the population (Cornell)</li><br /> <li>Progress in genetic evaluation for CDCB (UGA)</li><br /> <li>Why spurious signals in GWAS measures? (UGA)</li><br /> <li>Why do we look at 1 M windows? (UGA)</li><br /> <li>Are p-values worth anything? (UGA)</li><br /> <li>Negative effects of genomic selection (UGA)</li><br /> <li>Embryo Transfer & fertility evaluation (USDA)</li><br /> <li>Milking Speed Research Project (USDA)</li><br /> <li>Increasing the Accuracy of Genomic Prediction, Developing Algorithms, Selecting Markers, and Evaluating New Traits to Improve Dairy Cattle (USDA, UGA, NCSU)</li><br /> </ul><br /> <p> </p><br /> <p><strong>Objective 4</strong>: Develop variant discovery strategies to incorporate functional –omics data into breeding schemes for economically important traits</p><br /> <ul><br /> <li>Inheritance of a mutation causing Jersey neuropathy with splayed forelimbs (USDA)</li><br /> </ul><br /> <p> </p><br /> <p><strong>Objective 5</strong>: Carry out interdisciplinary collaborations to improve dairy cow and calf health through increased partnerships with researchers, industry, and stakeholders</p><br /> <ul><br /> <li>Precision crossbreeding for African Dairy Production Systems (UMN)</li><br /> <li>REML for Scalable Genome-Partitioning of Quantitative Genetic Variation (NCSU)</li><br /> </ul><br /> <p> </p><br /> <p><strong>Objective 6</strong>: Create a pipeline of diverse graduate students in the fields of quantitative and functional genetics and bioinformatics via outreach and educational opportunities</p><br /> <ul><br /> <li>Graduate research opportunities (Cornell, UMN, UW, PSU, MSU, NCSU, UFL, Purdue)</li><br /> <li>Undergraduate research opportunities (Cornell, UMN)</li><br /> </ul>Publications
<p>Basiel, B.L., L.C. Hardie, B.J. Heins+, C.D. Dechow. 2021. Genetic parameters and genomic regions associated with horn fly resistance in organic Holstein cattle. J. Dairy Sci. 104:12724-12740. https://doi.org/10.3168/jds.2021-20366</p><br /> <p>Haagen, I.W. L.C. Hardie, B.J. Heins, C.D. Dechow. 2021. Genetic parameters of calf morbidity and stayability for US organic Holstein calves J. Dairy Sci. 104: 11770-11778. https://doi.org/10.3168/jds.2021-20432</p><br /> <p>Hardie, L. C., I. W. Haagen, B. J. Heins, and C. D. Dechow. 2022. Genetic parameters and association of national evaluations with breeding values for health traits in US organic Holstein cows J. Dairy Sci. 105:495-508. https://doi.org/10.3168/jds.2021-20588</p><br /> <p>Wu, X.-L., Gaddis, K. L. P., Burchard, J., Norman, H. D., Nicolazz, E. Connor, E. E., Cole, J. B., Durr, J. (2021) An alternative interpretation of residual feed intake by phenotypic recursive relationships in dairy cattle. JDS Communications. 2(6):371-375.</p><br /> <p>Wu XL, Wiggans GR, Norman HD, Miles AM, Van Tassell CP, Baldwin RL, Burchard J, Dürr J. (2022) Statistical Methods Revisited for Estimating Daily Milk Yields: How Well do They Work? Front Genet. 13:943705.</p><br /> <p>Sigdel A, Wu XL, Parker Gaddis KL, Norman HD, Carrillo JA, Burchard J, Peñagaricano F, Dürr J. (2022) Genetic Evaluations of Stillbirth for Five United States Dairy Breeds: A Data-Resource Feasibility Study. Front Genet. 13:819678.</p><br /> <p>Gorr, A., V. E. Cabrera, J. Meronek, and K. A. Weigel. 2022. Decision-support tool for global allocation of dairy sire semen based on regional demand, supply constraints, and genetic profiles. Journal of Dairy Science (in press)</p><br /> <p>Liang, Z., D. Prakapenka, K. L. Parker Gaddis, M. J. VandeHaar, K. A. Weigel, R. J. Tempelman, J. E. Koltes, J. E. P. Santos, H. M. White, F. Peñagaricano, R. L. Baldwin, and Y. Da. 2022. Impact of epistasis effects on the accuracy of predicting phenotypic values of residual feed intake in U.S. Holstein cows. Frontiers in Genetics (in press)</p><br /> <p>Sigdel, A., R. S. Bisinotto, and F. Peñagaricano (2022) Genetic analysis of fetal loss in Holstein cattle. Journal of Dairy Science 105: 9012-9020 (https://doi.org/10.3168/jds.2022-22000)</p><br /> <p>Brown, W., M. J. Martin, C. Siberski, J. E. Koltes, F. Peñagaricano, K. A. Weigel, and H. M. White. 2022. Predicting dry matter intake in mid-lactation Holstein cows using point-in-time data streams available on dairy farms. Journal of Dairy Science 105: 8130-8142 (https://doi.org/10.3168/jds.2022-22093)</p><br /> <p>Pacheco, H. A., A. Rossoni, A. Cecchinato, and F. Peñagaricano (2022) Deciphering the genetic basis of male fertility in Italian Brown Swiss dairy cattle. Scientific Reports 12: 10575. (https://doi.org/10.1038/s41598-022-14889-1)</p><br /> <p>Cavani, L., W. E. Brown, K. L. Parker Gaddis, R. J. Tempelman, M. J. VandeHaar, H. M. White, F. Peñagaricano, and K. A. Weigel. 2022. Estimates of genetic parameters for feeding behavior traits and their associations with feed efficiency in Holstein cows. Journal of Dairy Science 105: 7564-7574 (https://doi.org/10.3168/jds.2022-22066)</p><br /> <p>Zhang, F., K. A. Weigel, and V. E. Cabrera. 2022. Predicting daily milk yield for primiparous cows using data of within herd relatives to capture genotype-by-environment interactions. Journal of Dairy Science 105: 6739-6748 (https://doi.org/10.3168/jds.2021-21559)</p><br /> <p>Sigdel, A., X. L. Wu, K. L. Parker Gaddis, H. D. Norman, J. A. Carrillo, J. F. Burchard, F. Peñagaricano, and J. W. Dürr (2022) Genetic evaluations of stillbirth for five US dairy breeds: a data-resource feasibility study. Frontiers in Genetics 13: 819678 (https://doi.org/10.3389/fgene.2022.819678)</p><br /> <p>Khanal, P., K. L, Parker Gaddis, M. J. VandeHaar, K. A. Weigel, H. White, F. Peñagaricano, J. E. Koltes, J. E. P. Santos, R. L. Baldwin, J. F. Burchard, J. W. Dürr, and R. J. Tempelman. 2022. Multiple trait random regression modeling of feed efficiency in US Holsteins. Journal of Dairy Science 105: 5954-5971 (https://doi.org/10.3168/jds.2021-21739)</p><br /> <p>Williams, K., K. A. Weigel, W. Coblentz, N. M. Esser, H. Schlesser, P. C. Hoffman, R. Ogden, H. Su, and M. S. Akins. 2022. Effect of diet energy level and genomic residual feed intake on bred Holstein dairy heifer growth and feed efficiency. Journal of Dairy Science 105: 2201-2214 (https://doi.org/10.3168/jds.2020-19982)</p><br /> <p>Novo, L. C., L. Cavani, P. Pinedo, P. Melendez, and F. Peñagaricano (2022) Genomic analysis of visceral fat accumulation in Holstein cows. Frontiers in Genetics 12: 803216. (https://doi.org/10.3389/fgene.2021.803216)</p><br /> <p>Cavani, L., M. B. Poindexter, C. D. Nelson, J. E. P. Santos, and F. Peñagaricano (2022) Gene mapping, gene-set analysis, and genomic prediction of postpartum blood calcium in Holstein cows. Journal of Dairy Science 105: 525-534 (https://doi.org/10.3168/jds.2021-20872)</p><br /> <p>Martin, M. J., R. S. Pralle, I. R. Bernstein, M. J. VandeHaar, K. A. Weigel, Z. Zhou, and H. M. White. 2021. Circulating metabolites indicate differences in high and low residual feed intake Holstein dairy cows. Metabolites 11, 868 (https://doi.org/10.3390/metabo11120868)</p><br /> <p>Martin, M. J., J. R. R. Dorea, M. R. Borchers, R. L. Wallace, S. J. Bertics, S. K. DeNise, K. A. Weigel, and H. M. White. 2021. Comparison of methods to predict feed intake and residual feed intake using behavioral and metabolite data in addition to classical performance variables. Journal of Dairy Science 104: 8765-8782 (https://doi.org/10.3168/jds.2020-20051)</p><br /> <p>Quick, A. E., J. Meronek, K. Amburn, K. Rozeboom, and K. A. Weigel. 2021. Predicting sperm production of young dairy bulls using collection history and management factors. Journal of Dairy Science 104: 5817-5826 (https://doi.org/10.3168/jds.2020-19617)</p><br /> <p>Martin, M. J., K. A. Weigel, and H. M. White. 2021. Assessment of the relationship between postpartum health and mid-lactation performance, behavior, and feed efficiency in Holstein dairy cows. Animals 11, 1385. (https://doi.org/10.3390/ani11051385)</p><br /> <p>Harbowy, R, Niles D, Bartlett P, Taxis TM. (2022) Controlling Bovine Leukemia Virus in a Large Dairy Herd by Selective Culling Based on Diagnostic Testing. Applied Animal Science, Under Review September 2022.</p><br /> <p>LaHuis, C, Benitez O, Droscha C, Singh S, Borgman A, Lohr C, Bartlett P, Taxis TM. (2022) Identification of BoLA Alleles Associated with BLV Disease Progression in US Beef Cows. Pathogens, 11(10), 1093. https://doi.org/10.3390/pathogens11101093.</p><br /> <p>Lohr CE, Sporer KRB, Brigham KA, Pavliscak LA, Mason MM, Borgman A, Ruggiero VJ, Taxis TM, Bartlett PC, Droscha CJ. (2022) Phenotypic Selection of Dairy Cattle Infected with Bovine Leukemia Virus Demonstrates Immunogenetic Resilience Through NGS-based Genotyping of BoLA MHC Class II genes. Pathogens 11(1), 104. https://doi.org/10.3390/pathogens11010104.</p><br /> <p> </p>Impact Statements
- (vi) Collaboration with the National Animal Germplasm Program (NAGP) Dairy Committee, Animal Genomics and Improvement Laboratory (AGIL), and the Council on Dairy Cattle Breeding (CDCB) to improve genetic variation of dairy and optimize economic merit indices